- 1The Department of Orthopaedics, The Second Xiangya Hospital of Central South University, Changsha, China
- 2Hunan Key Laboratory of Tumor Models and Individualized Medicine, The Second Xiangya Hospital of Central South University, Changsha, China
- 3Xiangya School of Medicine, Central South University, Changsha, China
- 4The Department of Radiology, The Second Xiangya Hospital of Central South University, Changsha, China
Background: As a research hotspot, deep learning has been continuously combined with various research fields in medicine. Recently, there is a growing amount of deep learning-based researches in orthopedics. This bibliometric analysis aimed to identify the hotspots of deep learning applications in orthopedics in recent years and infer future research trends.
Methods: We screened global publication on deep learning applications in orthopedics by accessing the Web of Science Core Collection. The articles and reviews were collected without language and time restrictions. Citespace was applied to conduct the bibliometric analysis of the publications.
Results: A total of 822 articles and reviews were finally retrieved. The analysis showed that the application of deep learning in orthopedics has great prospects for development based on the annual publications. The most prolific country is the USA, followed by China. University of California San Francisco, and Skeletal Radiology are the most prolific institution and journal, respectively. LeCun Y is the most frequently cited author, and Nature has the highest impact factor in the cited journals. The current hot keywords are convolutional neural network, classification, segmentation, diagnosis, image, fracture, and osteoarthritis. The burst keywords are risk factor, identification, localization, and surgery. The timeline viewer showed two recent research directions for bone tumors and osteoporosis.
Conclusion: Publications on deep learning applications in orthopedics have increased in recent years, with the USA being the most prolific. The current research mainly focused on classifying, diagnosing and risk predicting in osteoarthritis and fractures from medical images. Future research directions may put emphasis on reducing intraoperative risk, predicting the occurrence of postoperative complications, screening for osteoporosis, and identification and classification of bone tumors from conventional imaging.
Introduction
As a subset of machine learning, deep learning has broken the limitations of traditional machine learning, and can implement more accurate classification and segmentation of images to extract feature elements (1, 2). It is known that deep learning surpasses the performance of other machine learning techniques in several aspects, such as predicting the potential activity of drug molecules (3) and the alternative splicing patterns in tissues (4). Convolutional neural networks (CNNs), consisting of multiple neural layers, are powerful learning structures in deep learning. They are effectively used to solve related problems in computer vision and images. By varying the depth and breadth of the neural layers, the capacity of the CNNs can be expanded to accommodate tens of thousands of images and allow them to interpret correctly (1). Currently, deep learning has been demonstrated to have the potential to identify diseases such as skin lesions and diabetic retinopathy, and it has achieved greater accuracy that comparable to experienced physicians (5, 6).
Most musculoskeletal diseases in orthopedics require a large degree of help from images. Given the advantages of deep learning in image processing, there has been a growing number of researches on the application of deep learning in orthopedics in recent years (7). Bibliometrics is a statistical and quantitative method to analyze the academic characteristics of a body of literatures in certain scientific fields. It can help researchers to grasp the priorities and trends, and predict its prospects. Therefore, it has been applied in multiple research areas, including immunology (8), oncology (9, 10), nursing (11), vaccine (12), and orthopedics (13). The software Citespace can quantitatively analyze the literature database to evaluate the distribution of countries (or regions) and institutions, as well as excavate the literature that plays a central position in the research field, and visualize the research hotspots and development trends (14).
Currently, no bibliometric analysis has been conducted to quantitatively analyze the progress and current status of deep learning in this emerging field. Herein, this study aimed to elucidate the research hotspots, key fields, and trends of deep learning applications in orthopedics in recent years by using Citespace, Moreover, the research direction and references for further exploration were shown as well.
Methods
Data source and collection
Since the details of the documents in the Web of Science are more accurate than other databases, such as Scopus, PubMed, Embase, etc. We retrieved all literature from the Web of Science Core Collection. The retrieval was completed within 1 day on March 29, 2022 to reduce changes due to frequent updates to the bibliographic database. The searching strategy was as follows: #1 and #2 (#1:WC = (orthopedics) OR TS = (orthopedic* OR orthopedic* OR “sports medicine”) OR TS = (“bone disease*” OR TS = “bone age” OR bone fracture* OR “joint disease*” OR “joint disorder*” OR TS = “arthritis” OR TS = “joint dislocations” OR TS = “joint dislocations” OR TS = “musculoskeletal system” OR “musculoskeletal disease*” OR “musculoskeletal disorder*” OR “musculoskeletal trauma*” OR TS = “bone tumor*” OR TS = “bone cancer” OR TS = “bone metastasis” OR TS = “bone cyst*” OR TS = “soft tissue tumor*” OR TS = “soft tissue neoplasm*” OR TS = “joint replacement*” OR TS = “arthroplasty” OR TS = “arthroscope*”), #2: TS =“deep learning” OR TS = “convolutional neural network*”). There is no limit to the publication year. The type of literature was selected as articles and reviews without language restriction. Literature not relevant to this topic was excluded, and duplicate literature was removed by Using Citespace (5.8 R3). Finally, a total of 822 articles were retrieved and exported for records in the format of plain text files. Meanwhile, we also obtained the number of annual publications and the amount of publication of the journal.
Statistical analysis and visualization
The number of annual publications was imported into Microsoft Office Excel 2021 and the trend was further analyzed. CiteSpace is a visual bibliometrics tool based on Java language for quantitative analysis of literature in specific research areas. The conception of “co-citation analysis theory” is as follows: when two documents appeared in the reference list of a third cited document, these two documents form a co-citation relationship. By mining the “co-introduction relationship”, it is possible to reveal important turning points, the evolution of keywords, and the frontiers in related research fields. In this study, the time spans were set to 2015 to 2022 based on the publication date of all 822 literature. Time slices were set to 1 year per slice, and selection criteria was selected as g-index. Authors, countries, and institutions were selected to perform the cooperative network analysis. Keywords were selected for co-occurrence, burst, cluster analysis, and timeline viewer. Reference, cited-authors, and cited journals were chosen for citation analysis. Cosine was select for the link strength. Besides, pathfinder, pruning sliced networks, and pruning the merged network in pruning algorithm were selected. In the visual network maps, node colors change from cold to warm from the inside to the outside, representing the year from the original to the most recent. The purple circle on the outside represented high centrality, and the node connection indicated cooperation, co-occurrence, and co-citation.
Results
Trends of annual publications
Overall, a total of 822 publications (759 articles and 63 reviews) were identified. The number of studies published in each year can help us understand the general trends in the relevant research. Since 2022 has just begun, the number of documents in 2022 cannot show the overall publication situation. Therefore, the analysis only included the publication from 2015 to 2021. As shown in Figure 1, the number of studies concerning the application of deep learning in orthopedics has increased year by year since 2017, indicating that deep learning has received high attention from orthopedics in this period. Compared with publications in 2019, the total number of articles published in 2020 were 227, showing an explosive growth. To further understand the trend of annual publications in this field between 2015 and 2021, a trend line of publications in this period was plotted, and the results showed the exponential function Y = 0.2941e1.0998x (R2 = 0.8983, Y is the annual publication, and X is the year). It is clear that the application of deep learning in orthopedics has great potential, and research trends are likely to continue.
Distribution of countries/ regions and institutions
We used Citespace to conduct co-citation analysis of the countries (or regions) and institutions. The country or region distribution map consisted of 56 nodes and 53 links. As demonstrated in Figure 2A and Table 1, the most significant number of publications came from USA (211 time) and China (196 time), which were three times higher than those of other countries or regions. The countries of the top ten centrality were Greece (0.76), Switzerland (0.75), Spain (0.74), France (0.51), Estonia (0.5), England (0.45), Australia (0.45), Egypt (0.45), Canada (0.42), and Belgium (0.41) (Table 2). Analyzing nodes reveals that they have more connections to other countries and continents. England, Australia, and Canada had high centrality and publications. The institution distribution map consisted of 225 nodes and 239 links. As shown in Figure 2B and Table 2, the top ten prolific institutions were University of California San Francisco (USA), Stanford University (USA), Johns Hopkins University (USA), Harvard Medical School (USA), Shanghai Jiao Tong University (China), Seoul National University (South Korea), University of Chinese Academy of Sciences (China), Sun Yat-sen University (China), China Medical University (China), and Yonsei University (South Korea). Nodes with a centrality >0.1 indicate a good key role. Sun Yat-sen University (China), Harvard Medical School (USA), Shanghai Jiao Tong University (China), and University of Chinese Academy of Sciences (China) had high centrality and publication.
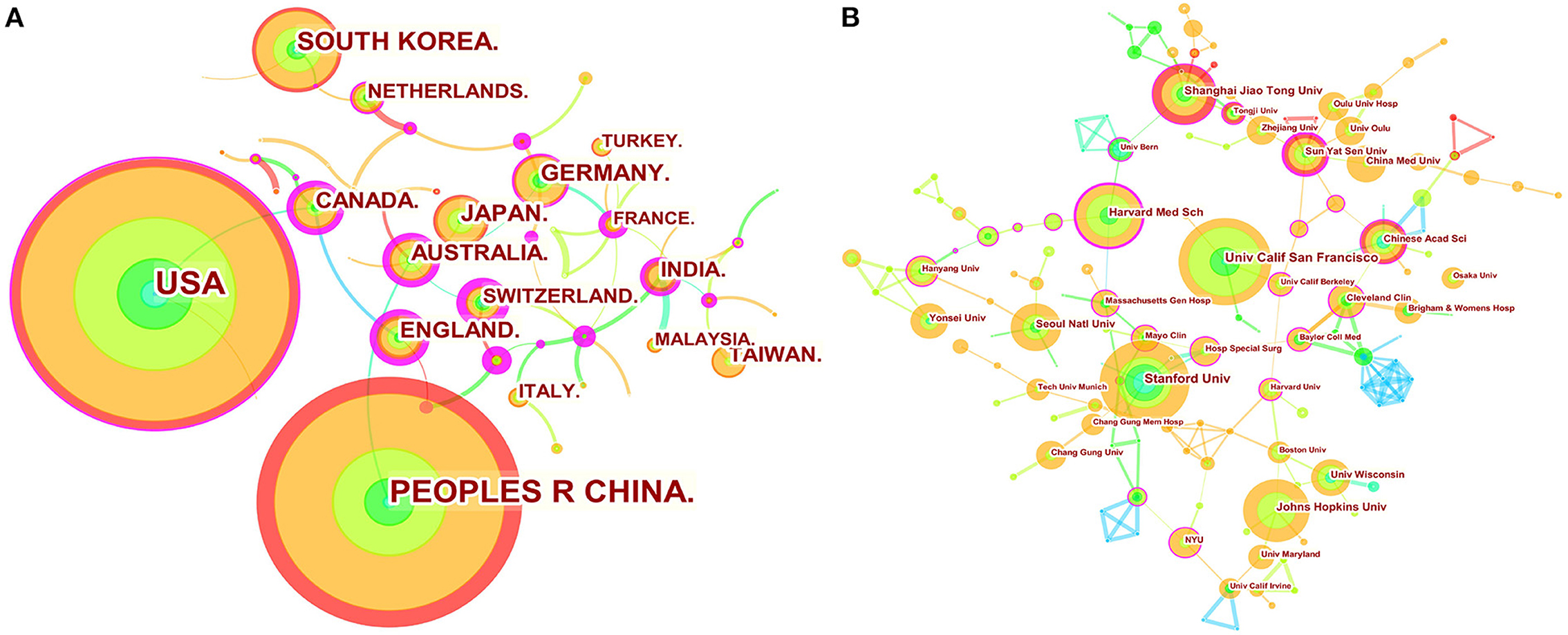
Figure 2. Map of countries (or regions) cooperation networks (A) and institution cooperation networks (B). The nodes represent country (or region) or institution. The lines represent cooperation relationships. The colors in the nodes represent the years, and the purple ring represents centrality.
Analysis of authors and cited authors
A total of 250 authors were involved in the cooperative map (Figure 3A). As shown in Table 3, VALENTINA PEDOIA was the author with the most published literature. Interestingly, among the top ten authors, we found three major collaborative networks. They were VALENTINA PEDOIA (University of California System, USA) and SHARMILA MAJUMDAR (University of California System, USA), PAUL H YI (Johns Hopkins University, USA) and JAN FRITZ (New York University, USA), JARET M KARNUTA (Cleveland Clinic Foundation, USA), PREM N RAMKUM AR (Cleveland Clinic Foundation, USA) and HEATHER S HAEBERLE (Cleveland Clinic Foundation, USA), separately. Co-cited authors are two (or more authors) who are cited in one or more subsequent papers at the same time, the two or more authors constitute a co-cited relationship. The co-cited author map consisted of 487 notes and 747 links (Figure 3B). As displayed in Table 3, LeCun Y was the most frequently co-cited author. Among the top ten co-cited authors, Litjens G had the most centrality.
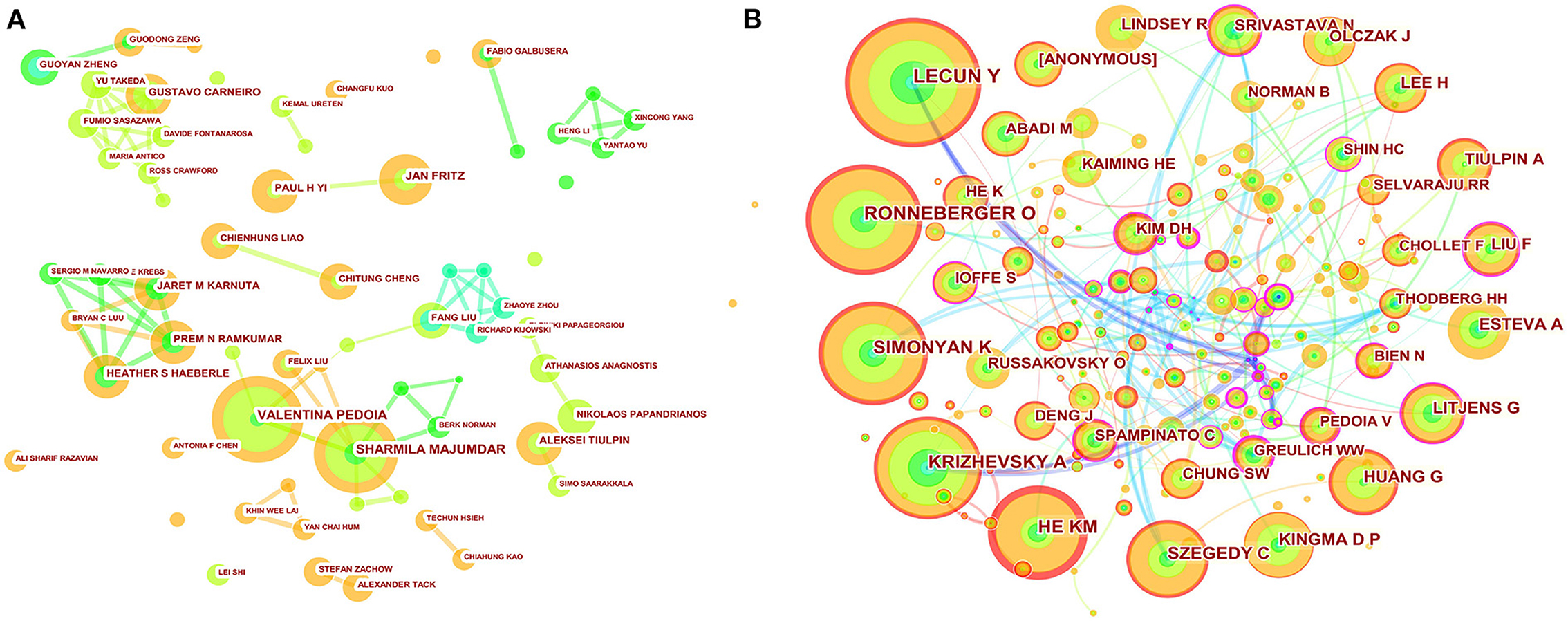
Figure 3. Map of author's cooperative relationship (A) and co-citation network (B). The nodes represent author or co-cited author, and the lines represent cooperation or co-citation relationships, respectively. The colors in the nodes represent the years, and the purple ring represents centrality.
Journals and co-cited academic journals
The 822 articles retrieved in this study were published in 308 journals. As shown in Table 4, The journal Skeletal Radiology (33 times) had the highest number of outputs, followed by IEEE Access (28 times), Scientific Reports (25 times), Computer Methods and Programs in Biomedicine (19 times), Applied Sciences Basel (17 times), Diagnostics (17 times), International Journal of Computer Assisted Radiology and Surgery (15 times), Journal of Digital Imaging (14 times), Medical Image Analysis (14 times), and Sensors (14 times). The co-occurrence analysis of cited journals obtained from Citespace was shown in Figure 4A and Table 4, with 532 nodes and 817 links. The greater the node, the higher the co-citation frequency of journals. Through the analysis of cited journals, the distribution of journals that focus on this field can be obtained. Co-citation frequency reflects the quality and influence of journals. Journals with high co-citation frequency are often regarded as mainstream journals. The top ten cited journals were Lecture Notes in Computer Science (345 times), In Proceedings of The IEEE Conference on Computer Vision and Pattern Recognition (324 times), Medical Image Analysis (287 times), Radiology (280 times), IEEE Transactions on Medical Imaging (274 times), Scientific Reports (197 times), Nature (190 times), Journal of Digital Imaging (185 times), PLoS One (179 times), and American Journal of Roentgenology (149 times).
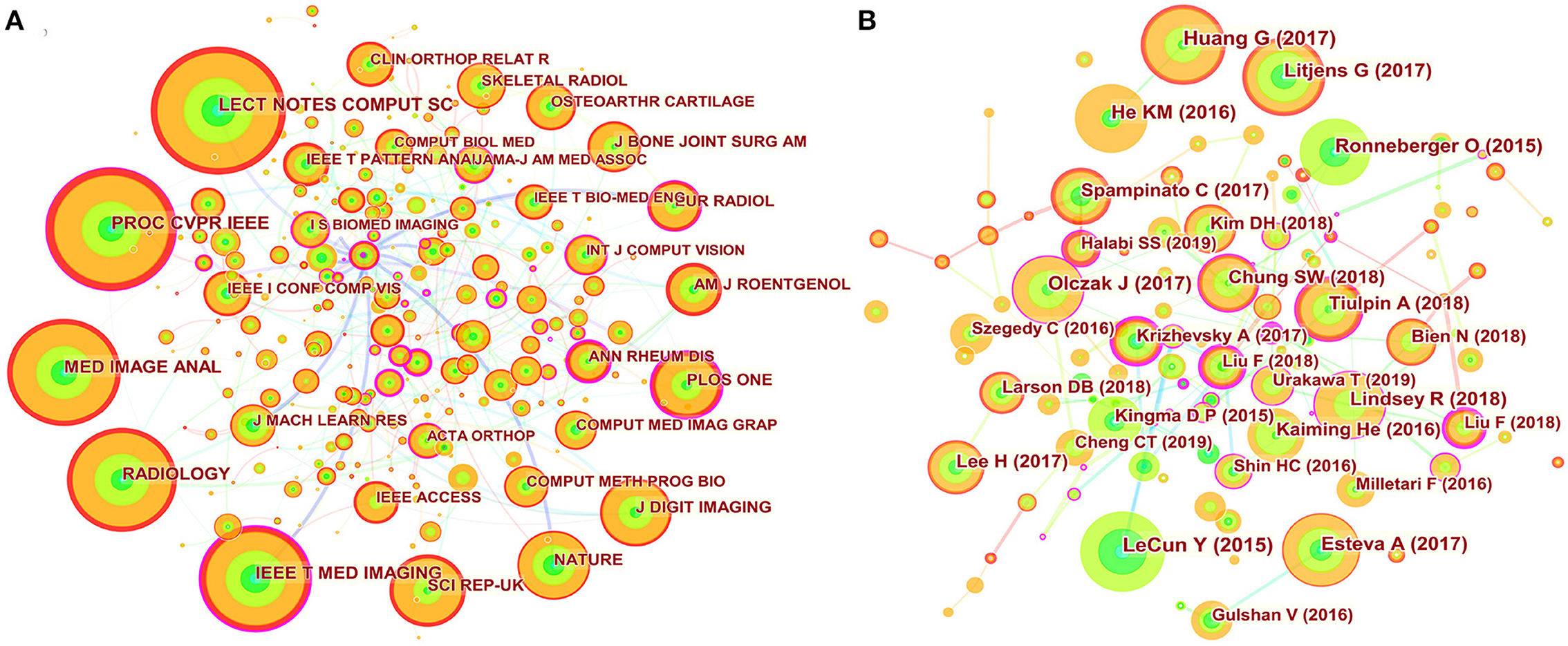
Figure 4. Map of journal co-citation and cited references. (A) The nodes represent journal. The lines represent co-citation relationships. The colors in the nodes represent the years, and the purple ring represents centrality. (B) The nodes represent cited reference. The lines represent co-citation relationships. The colors in the nodes represent the years, and the purple ring represents centrality.
Analysis of cited references
A total of 464 nodes and 733 links presenting the co-citation relationship of the references formed the cited reference network in Figure 4B. Co-citation means that two or more articles are cited by one or more papers at the same time, and important references have higher co-citation frequency. As shown in Table 5, among the top ten most frequently cited references, “Deep learning” had the most frequency. Meanwhile, there were three proceedings papers, namely the “Densely Connected Convolutional Networks,” “Deep Residual Learning for Image Recognition,” and “U-Net: Convolutional Networks for Biomedical Image Segmentation.” Most of these articles were preliminary explorations of the neighborhood, and the publication of these articles was related to the gradual increase in annual publications that began in 2017.
Analysis of keywords, burst value, clustering, and time evolution
The high-frequency keywords the article can help us understand the main research hotspots of the filed. Keyword co-occurrence analysis was performed by Citespcace. The co-occurrence network of keywords had a total of 265 nodes and 404 links (Figure 5A). The high-frequency keywords were listed in Table 6. The top 20 keywords were as follows: classification, segmentation, convolutional neural network, MRI, system, diagnosis, osteoarthritis, artificial intelligence, image, neural network, CT, model, hip, knee, bone, fracture, children, reliability, disease, and prediction. From these keywords, we found that the main research methods were artificial intelligence, neural network, and convolutional neural network. The main research tasks were classification, segmentation, diagnosis, and prediction. The main research content was image, and the main research objects were bone, hip, knee, osteoarthritis, fracture, and disease. To sum up, classification, diagnosis, and risk prediction of various types of fractures and osteoarthritis by image were major research hot-spots.
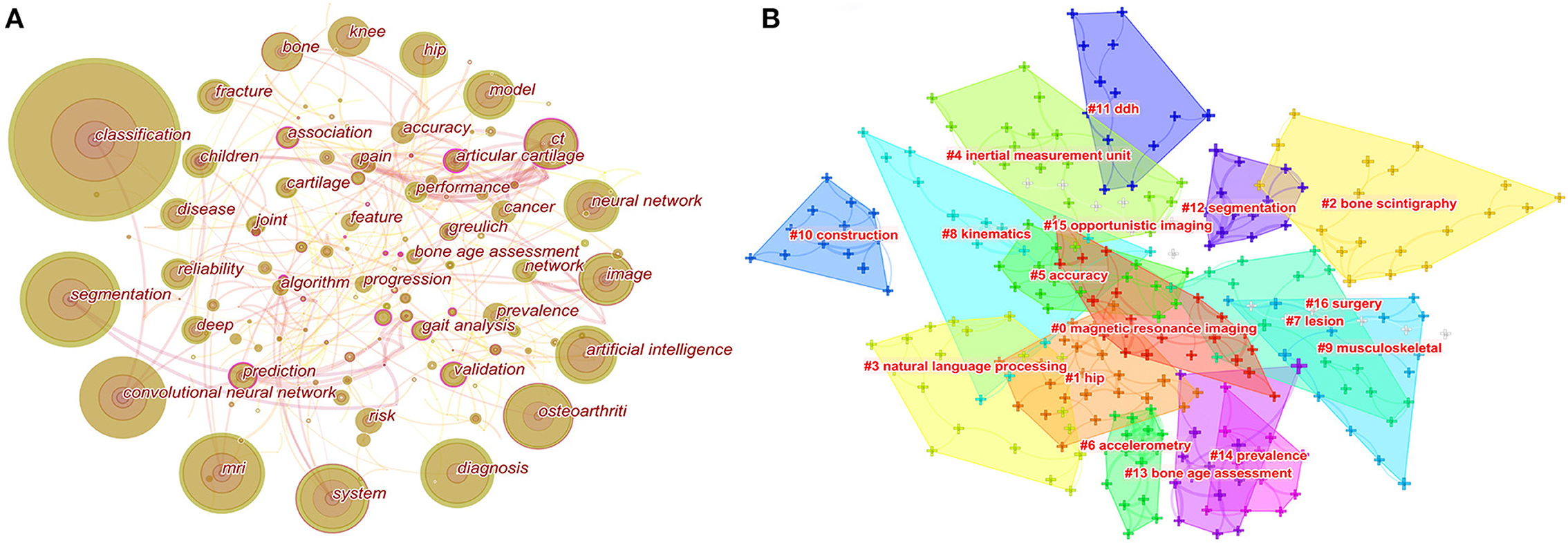
Figure 5. Map of keywords occurrence (A) and the clustering of keywords (B). For keywords occurrence, the nodes represent keywords. The lines represent co-occurrence relationships, and the colors in the nodes represent the years.
The clustering of keywords can present the structural system of related research fields. The sixteen different clusters made through Citespace were shown in Figure 5B: #0 magnetic resonance imaging, #1 hip, #2 bone scintigraphy, #3 natural language processing, #4 inertial measurement unit, #5 accuracy, #6 accelerometry, #7 lesion, #8 kinematics, #9 musculoskeletal, #10 construction, #11 DDH, #12 segmentation, #13 bone age assessment, #14 prevalence, #15 opportunistic imaging, and #16 surgery. The ordinal number of clusters was arranged by cluster size, and the smaller the ordinal number, the larger the cluster.
The strong burst keywords can help us explore the development trends of the filed. We use Citespace's burst function to analyze keywords that have attracted much attention from academia. The burst keywords were shown in Figure 6. Keywords with higher burst values over a period mean that they have received special attention during the corresponding time intervals and may become a new research trend. In the burst keywords after 2020, we paid attention to the following main keywords: risk factor, identification, localization, and surgery. At the same time, a timeline viewer of keywords was plotted, which may help to visualize the phased hotspots and directions from the temporal dimension (Figure 7).
Discussion
This study utilized the principles of bibliometrics analysis and the method of Citespace visualization. In the application of deep learning in orthopedic filed, the annual number of articles, countries (or regions) and institutions, authors and cited authors, journals and cited journals, and keywords were extensively analyzed to reveal the current research hot-spots and trends in this field.
Based on an analysis of countries (or regions) and institutions, the USA and China were the two most documented countries, but there was a lack of international cooperation between them. More international cooperation is needed for China (0.00 centrality) to jointly promote development in this field. England, Australia, and Canada had high centrality and publications, suggesting that these countries might play an essential role in bridging research. At the same time, most of the top ten institutions with the most centrality and publications are from China and the USA, but these institutions basically cooperate with other institutions in their countries. Therefore, from the perspective of cooperation networks, we hope that there will be more cooperation between different countries.
Through the analysis of the author cooperation network, we found that the top ten authors mainly constituted three cooperative networks. In the cooperation between the two authors in the deep learning field, VALENTINA PEDOIA (University of California System) and Sharmila MAJUMDAR (University of California System), the most cited literature showed that the improved U-net can automatically segment cartilage and meniscus from MRI (15). PAUL H YI (Johns Hopkins University, USA) and JAN FRITZ (New York University, USA) have reported that deep learning can significantly address the identification of implants after knee replacement surgery and help with subsequent revision surgeries (16). JARET M KARNUTA (Cleveland Clinic Foundation, USA), PREM N RAMKUMAR (Cleveland Clinic Foundation, USA) and HEATHER S HAEBERLE (Cleveland Clinic Foundation, USA) summarized some application of artificial intelligence in orthopedics (17). However, it is worth noting that in the entire network of cooperative map, none of the authors had a centrality <0.1, indicating a lack of international cooperation among these authors. The entire network presented a very weak partnership.
As to the analysis of a network of cited authors, the most frequently cited author-LeCun Y believed that deep learning is good at processing high-dimensional data, and can accurately identify and classify targets in image recognition tasks (18). Similarly, Litjens G et al. with the most centrality in the top ten co-cited authors published “A survey on deep learning in medical image analysis (19),” which plays a key role in the field.
According to the journals and co-cited journals in Table 4. The journal Skeletal Radiology had the most publications. The average impact factor of the top ten journals in 2020 was 4.086, and the impact factor of Medical Image Analysis was the highest (8.545). Furthermore, seventy percent of journals had an impact factor over three, and fifty percent of journals belonged to Q1 (JCR). The journal Nature had the highest impact factor in the cited journals. In addition to the cited journals that cannot obtain impact factor and JCR partitions, seventy-five percent of the cited journals belonged to Q1. These data suggested that this area has attracted academic attention from high-scoring journals.
Among top ten co-cited references, these references are usually the basis of relevant knowledge fields and play an important role in knowledge structure. For three proceedings papers, Ronneberger O, He KM, and Huang G built new models for deep learning in 2015, 2016, and 2017, respectively, which strengthened the ability of deep learning to process images. These models are deep residual learning, densely connected convolutional networks, and U-net (20–22). Article entitled “Deep learning” by LeCun Y et al. indicated that deep learning can precisely solve tasks including image recognition and speech recognition (18). Therefore, these articles laid the foundation of this field. “A survey on deep learning in medical image analysis” by Litjens G et al. summarized the research on the application of deep learning in various medical fields before Feb 1, 2017 in details, and these researches were basically published in 2016 and early 2017. We found that these studies on the application of deep learning in musculoskeletal disease were basically belong to proceedings papers (19). Today, the trend of publication in the journals is increasing year by year (Figure 1), suggesting that research in this field will still be a fertile area in the next few years. Among the top ten most frequently cited reference, a study by Olczak J et al. was the first article about deep learning application in fracture, and the performance of deep learning was like that of senior orthopedic surgeons (23).
Keywords are the cores of a paper that reflecting the concerns of relevant field. By analyzing the high-frequency keywords and strong-burst keywords, we can explore the hot-spots and research trends. The main research hotspots and research trends are as follows:
For hot keyword-fracture
After the first report of deep learning in fracture by Olczak et al. (23), the number of studies concerning this field increased with years.
For upper extremity fracture, deep learning is commonly applied to distal radius fracture and humeral fracture (24–26). Gan et al. applied two deep learning models to identify distal radius fracture (24). They trained a Faster R-CNN with the ability to automatically annotate the regions of interest (ROIs), and then another CNN model for diagnostic was trained with images that were annotated by Faster R-CNN Model; The average intersection of the union (IOU) value of Faster R-CNN was 0.87, and the AUC of inception-v4 was 0.96. However, their data consisted only of anterior-posterior (AP) image, whereas images in both the AP and lateral directions were often clinically required, and there was no adequate amount of data from multiple centers. Recently, Suzuki et al. addressed these two issues, and the results showed that the CNN model had better accuracy than orthopedic surgeons (25). In addition to identification of fractures, deep learning can also directly classify fracture types. Chung et al. shown that CNN was superior to orthopedic surgeons in the classification of proximal humeral three- and four-part fracture, with an AUC exceeding 90%, while both fractures are easily misjudged in clinical work (27). Besides, Langerhuizen et al. tried to identify scaphoid bone fracture, a common fracture of the wrist, by using a small data set and found that deep learning performed inferior than surgeons in 2020 (28). However, Yoon et al. later established two model, namely the Apparent Fracture Model and the Occult Fracture Model, which excelled in identifying obvious and potential scaphoid fractures, respectively. Their research used more datasets and escalated deep learning algorithms, and both models showed higher sensitivity and specificity than that of Langerhuizen et al. (29).
In lower limb, hip fractures attracted much attention. Cheng et al. proved that deep learning model can automatically identify femoral neck fracture and trochanteric fracture through pelvic X-rays, with 98% sensitivity and 91% accuracy (30). Mutasa et al. and Bae et al. designed deep learning models for identifying garden-type femoral neck fractures on hip or pelvic X-rays, respectively (31, 32). Similarly, Urakawa et al. reported that deep learning also outperformed clinicians in identifying intertrochanteric fractures (33). In addition, Badgeley et al. incorporated patient characteristics into the deep learning model to predict hip fractures (34). Zdolsek et al. also identified atypical femoral fractures from normal femoral shaft fractures on conventional X-rays by deep learning models, and the ResNet had the best performance (35). Ankle fractures are considered as one of the most common fractures in clinical practice. Currently, classifying ankle fractures using the AO Foundation/Orthopedic Trauma Association (AO/OTA) system is not easy to grasp. The ResNet network trained by Olczak et al. can classify ankle fractures based on the AO/OTA system, with an AUC of 0.90 (36). In addition to these common lower extremity fractures, deep learning can also assist in diagnosing and identifying calcaneus fractures by accurately evaluating Bohler's angle (BA) and critical angle of Gissane (CAG) on X-rays (37).
Moreover, studies showed that deep learning can also help to reveal vertebrae fracture as well. Murata et al. accurately identified vertebral fractures on plain thoracolumbar radiography (38). Usually, compression fractures of the vertebrae are associated with osteoporosis. Improving the recognition of compression fractures is also the direction of the current solution. Now, deep learning can identify vertebral compression fractures on radiography and can help distinguish between fresh and old compression fractures, solving the challenge of identifying fresh compression fractures on radiography (39). Similarly, on MRI images, deep learning has also reached the level of specialists in identifying fresh compressed bones (40). More recently, a study by Suri et al. also adopted deep learning to accurately segment and identify the vertebral body and intervertebral discs on MRI, CT, and X-rays, which can offer substantial help in the clinical practice concerning spine-related diseases (41).
For hot keyword-osteoarthritis
Osteoarthritis is the most common musculoskeletal disorder, which mainly affects the hip and knee joints with large weight bearings, especially the knee joint. Initially, a proceedings paper showed the use of CNN to analyze Kellgren & Lawrence (K&L) grades based on knee radiographs (42). Then, Tiulpin et al. incorporated additional disease-related features (such as the joint space) into the deep learning model and ultimately achieved better result (43). In addition, a deep learning model developed by Tiulpin et al. can automatically assess Osteoarthritis Research Society International (OARSI) and K&L grading (44). Leung et al. demonstrated the possibility of deep learning in predicting total knee replacement in patients with osteoarthritis. In addition to routine X-rays, current research suggests that deep learning can enable MRI to become an effective tool in osteoarthritis recognition and clinical application by significantly reducing the acquisition time and automatically precise segmentation (15, 45).
For the burst keywords-risk factor, identification, localization, and surgery
Deep learning is often applied to the classification and diagnosis of diseases. However, with the development of deep learning, this technique will also be applied to perioperative management of orthopedic surgery. Nowadays, deep learning is being gradually explored in the study of orthopedic surgery. Since the visual field in arthroscopic surgery is often affected by different angles of the joint, which requires repositioning in different visual fields, automatic localization under the arthroscopic field of view is urgently needed. Recently, Banach A et al. collected arthroscopic videos and performed deep learning on video sequences at four different knee angles, and the results showed that deep learning performed well in arthroscopic field of view (46). Besides, Sarin JK et al. argued that deep learning can detect joint degeneration and help determine the boundaries between diseased cartilage and normal cartilage in arthroscopic surgery (47). In addition, Seibold M et al. combined acoustic sensing and deep learning to help reduce complications such as soft tissue damage caused by excessive bone drilling in surgery (48). These studies demonstrate the potential of deep learning in assisting orthopedic surgery. For the postoperative aspect of orthopedic surgery, the prediction of postoperative complications is important. Zhu WB et al. used deep learning to infer the possibility of bone necrosis after femoral neck fracture internal fixation from postoperative X-rays, which can help clinicians make timely treatment (49).
For the timeline viewer of keywords
From the timeline viewer of keywords, we found that the study of fractures and osteoarthritis gradually began to study in-depth from 2016 to 2019. We also paid special attention to the direction of gradual in-depth research after 2019, such as osteoporosis, and bone tumors (osteosarcoma). Osteoporosis often occurs in older or postmenopausal women, and is usually detected because of fractures, thereby screening for osteoporosis may help prevent osteoporotic fractures in many patients. Deep learning can help predict osteoporosis and the possible fracture risk (50, 51). Although dual energy X-ray absorptiometry is the current gold standard for osteoporosis diagnosis, the lack of universality limits its clinical application. Accordingly, Loffler et al. used conventional CT combined with deep learning to predict osteoporosis (52). In addition, the present studies on bone tumor rely on radiographic imaging and histopathology. Deep learning can help improve the segmentation of bone tumor in imaging (53), and can also be used in histopathology to quickly distinguish between normal tumor areas and necrotic tumor areas, thereby assisting to evaluate response to neojuvant chemotherapy (54, 55). Therefore, the use of deep learning based on conventional imaging and histopathology to accurately screen osteoporosis, identify and classify bone tumors may become future research directions.
Several limitations remain in this study. First, as the literature in the Web of Science Core Collection is constantly updated, and there is currently no uniform regulation on keywords related to the literature, the results of this study may differ from the actual number of documents included. Second, deep learning first appeared in seminars or conferences, and then gradually appeared in journals in the form of article. Since we consider that article has more systematic research, so it is strictly regulated in literature inclusion, which may introduce certain bias to this study. However, it is believed that literature-based visual analysis has undoubtedly laid a foundation for investigators to quickly understand the research hot-spots and development trends of deep learning applied in orthopedics.
Conclusion
In conclusion, according to the annual publication curve, the research on the application of deep learning in orthopedics has developed rapidly, and there is a good research prospect. Accordingly, researchers in the field of deep learning and orthopedics may benefit this study. The USA is the country with the largest amounts of articles in this field. University of California San Francisco, the institution with the largest amounts of articles, is also from the USA. However, cooperation between different countries is markedly insufficient. The application of deep learning in orthopedic research has been published in journals of various discipline categories, and multidisciplinary communication conforms to the mainstream of today's world. The current research hot-spots mainly focused on the classification and diagnosis of orthopedic diseases that rely heavily on medical images, such as fractures and osteoarthritis. The application of deep learning to reduce intraoperative risk, predict postoperative risk, screen osteoporosis, and identify, classify, and segment bone tumors may be at the fore-front of the future. At the same time, with the rapid development of deep learning applications in orthopedics, researches in this field will continue to evolve toward multicentric data and more perfect deep learning algorithms.
Data availability statement
The original contributions presented in the study are included in the article/supplementary material, further inquiries can be directed to the corresponding author.
Author contributions
CF: investigation, data analysis and visualization, and writing—original draft preparation. XZ, HW, YH, and ZL: investigation and editing. CT: conceptualization, supervision, and writing and revision. All authors have read and agreed to the published version of the manuscript.
Funding
This work was supported by grant from the National Natural Foundation of China (81902745), Hunan Provincial Natural Science Foundation of China (2022JJ30843), the Science and Technology Development Fund Guided by Central Government (2021Szvup169), Hunan Provincial Administration of Traditional Chinese Medicine Project (No. D2022117), and Clinical Research Center for Medical Imaging in Hunan Province (2020SK4001).
Conflict of interest
The authors declare that the research was conducted in the absence of any commercial or financial relationships that could be construed as a potential conflict of interest.
Publisher's note
All claims expressed in this article are solely those of the authors and do not necessarily represent those of their affiliated organizations, or those of the publisher, the editors and the reviewers. Any product that may be evaluated in this article, or claim that may be made by its manufacturer, is not guaranteed or endorsed by the publisher.
References
1. Krizhevsky A, Sutskever I, Hinton GE. Imagenet classification with deep convolutional neural networks. Commun ACM. (2017) 60:84–90. doi: 10.1145/3065386
2. Girshick R, Donahue J, Darrell T, Malik J IEEE (editors). Rich feature hierarchies for accurate object detection and semantic segmentation. 27th IEEE Conference on Computer Vision and Pattern Recognition (CVPR) Columbus, OH (2014).
3. Ma J, Sheridan RP, Liaw A, Dahl GE, Svetnik V. Deep neural nets as a method for quantitative structure-activity relationships. J Chem Inf Model. (2015) 55:263–74. doi: 10.1021/ci500747n
4. Leung MK, Xiong HY, Lee LJ, Frey BJ. Deep learning of the tissue-regulated splicing code. Bioinformatics. (2014) 30:i121–9. doi: 10.1093/bioinformatics/btu277
5. Esteva A, Kuprel B, Novoa RA, Ko J, Swetter SM, Blau HM, et al. Dermatologist-Level classification of skin cancer with deep neural networks. Nature. (2017) 542:115–8. doi: 10.1038/nature21056
6. Ting DSW, Cheung CY-L, Lim G, Tan GSW, Quang ND, Gan A, et al. Development and validation of a deep learning system for diabetic retinopathy and related eye diseases using retinal images from multiethnic populations with diabetes. JAMA. (2017) 318:2211–23. doi: 10.1001/jama.2017.18152
7. Kijowski R, Liu F, Caliva F, Pedoia V. Deep learning for lesion detection, progression, and prediction of musculoskeletal disease. J Magn Reson Imaging. (2020) 52:1607–19. doi: 10.1002/jmri.27001
8. Ma D, Yang B, Guan B, Song L, Liu Q, Fan Y, et al. A bibliometric analysis of pyroptosis from 2001 to 2021. Front Immunol. (2021) 12:731933. doi: 10.3389/fimmu.2021.731933
9. Li G, Liang Y, Yang H, Zhang W, Xie T. The research landscape of ferroptosis in cancer: a bibliometric analysis. Front Cell Dev Biol. (2022) 10:841724. doi: 10.3389/fcell.2022.841724
10. Tozzi AE, Fabozzi F, Eckley M, Croci I. Dell'Anna VA, Colantonio E, et al. Gaps and opportunities of artificial intelligence applications for pediatric oncology in european research: a systematic review of reviews and a bibliometric analysis. Front Oncol. (2022) 12:905770. doi: 10.3389/fonc.2022.905770
11. Kokol P. Meta approaches in knowledge synthesis in nursing: a bibliometric analysis. Nurs Outlook. (2021) 69:815–25. doi: 10.1016/j.outlook.2021.02.006
12. Fernandes S, Jit M, Bozzani F, Griffiths UK, Scott JAG, Burchett HED, et al. Bibliometric analysis of systematic reviews on vaccines and immunisation. Vaccine. (2018) 36:2254–61. doi: 10.1016/j.vaccine.2018.02.049
13. He J, He L, Geng B, Xia Y. Bibliometric analysis of the top-cited articles on unicompartmental knee arthroplasty. J Arthroplasty. (2021) 36:1810–8 e3. doi: 10.1016/j.arth.2020.11.038
14. Synnestvedt MB, Chen C, Holmes JH. Citespace Ii: Visualization and Knowledge Discovery in Bibliographic Databases. AMIA Annual Symposium proceedings AMIA Symposium (2005):724–8.
15. Norman B, Pedoia V, Majumdar S. Use of 2d U-Net convolutional neural networks for automated cartilage and meniscus segmentation of knee Mr Imaging data to determine relaxometry and morphometry. Radiology. (2018) 288:177–85. doi: 10.1148/radiol.2018172322
16. Yi PH, Wei JC, Kim TK, Sair HI, Hui FK, Hager GD, et al. Automated detection & classification of knee arthroplasty using deep learning. Knee. (2020) 27:535–42. doi: 10.1016/j.knee.2019.11.020
17. Helm JM, Swiergosz AM, Haeberle HS, Karnuta JM, Schaffer JL, Krebs VE, et al. Machine learning and artificial intelligence: definitions, applications, and future directions. Curr Rev Musculoskelet Med. (2020) 13:69–76. doi: 10.1007/s12178-020-09600-8
19. Litjens G, Kooi T, Bejnordi BE, Setio AAA, Ciompi F, Ghafoorian M, et al. A survey on deep learning in medical image analysis. Med Image Anal. (2017) 42:60–88. doi: 10.1016/j.media.2017.07.005
20. Ronneberger O, Fischer P, Brox T editors. U-Net: convolutional networks for biomedical image segmentation. 18th International Conference on Medical Image Computing and Computer-Assisted Intervention (MICCAI) Munich, Germany (2015).
21. He K, Zhang X, Ren S, Sun J IEEE (editors). Deep Residual Learning for Image Recognition. 2016 IEEE Conference on Computer Vision and Pattern Recognition (CVPR) Seattle, WA (2016).
22. Huang G, Liu Z, van der Maaten L, Weinberger KQ IEEE (editors). Densely Connected Convolutional Networks. 30th IEEE/CVF Conference on Computer Vision and Pattern Recognition (CVPR) Honolulu, HI (2017).
23. Olczak J, Fahlberg N, Maki A, Razavian AS, Jilert A, Stark A, et al. Artificial intelligence for analyzing orthopedic trauma radiographs deep learning algorithms-are they on par with humans for diagnosing fractures? Acta Orthop. (2017) 88:581–6. doi: 10.1080/17453674.2017.1344459
24. Gan KF, Xu DL, Lin YM, Shen YD, Zhang T, Hu KQ, et al. Artificial intelligence detection of distal radius fractures: a comparison between the convolutional neural network and professional assessments. Acta Orthop. (2019) 90:394–400. doi: 10.1080/17453674.2019.1600125
25. Suzuki T, Maki S, Yamazaki T, Wakita H, Toguchi Y, Horii M, et al. Detecting distal radial fractures from wrist radiographs using a deep convolutional neural network with an accuracy comparable to hand orthopedic surgeons. J Digit Imaging. (2022) 35:39–46. doi: 10.1007/s10278-021-00519-1
26. Tobler P, Cyriac J, Kovacs BK, Hofmann V, Sexauer R, Paciolla F, et al. Ai-Based detection and classification of distal radius fractures using low-effort data labeling: evaluation of applicability and effect of training set size. Eur Radiol. (2021) 31:6816–24. doi: 10.1007/s00330-021-07811-2
27. Chung SW, Han SS, Lee JW, Oh KS, Kim NR, Yoon JP, et al. Automated detection and classification of the proximal humerus fracture by using deep learning algorithm. Acta Orthop. (2018) 89:468–73. doi: 10.1080/17453674.2018.1453714
28. Langerhuizen DWG, Bulstra AEJ, Janssen SJ, Ring D, Kerkhoffs G, Jaarsma RL, et al. Is deep learning on par with human observers for detection of radiographically visible and occult fractures of the scaphoid? Clin Orthop Rel Res. (2020) 478:2653–9. doi: 10.1097/CORR.0000000000001318
29. Yoon AP, Lee YL, Kane RL, Kuo CF, Lin CH, Chung KC. Development and validation of a deep learning model using convolutional neural networks to identify scaphoid fractures in radiographs. JAMA Netw Open. (2021) 4:11. doi: 10.1001/jamanetworkopen.2021.6096
30. Cheng CT, Ho TY, Lee TY, Chang CC, Chou CC, Chen CC, et al. Application of a deep learning algorithm for detection and visualization of hip fractures on plain pelvic radiographs. Eur Radiol. (2019) 29:5469–77. doi: 10.1007/s00330-019-06167-y
31. Mutasa S, Varada S, Goel A, Wong TT, Rasiej MJ. Advanced deep learning techniques applied to automated femoral neck fracture detection and classification. J Digit Imaging. (2020) 33:1209–17. doi: 10.1007/s10278-020-00364-8
32. Bae J, Yu S, Oh J, Kim TH, Chung JH, Byun H, et al. External validation of deep learning algorithm for detecting and visualizing femoral neck fracture including displaced and non-displaced fracture on plain X-ray. J Digit Imaging. (2021) 34:1099–109. doi: 10.1007/s10278-021-00499-2
33. Urakawa T, Tanaka Y, Goto S, Matsuzawa H, Watanabe K, Endo N. Detecting intertrochanteric hip fractures with orthopedist-level accuracy using a deep convolutional neural network. Skeletal Radiol. (2019) 48:239–44. doi: 10.1007/s00256-018-3016-3
34. Badgeley MA, Zech JR, Oakden-Rayner L, Glicksberg BS, Liu M, Gale W, et al. Deep learning predicts hip fracture using confounding patient and healthcare variables. Npj Digital Medicine (2019) 2:31. doi: 10.1038/s41746-019-0105-1
35. Zdolsek G, Chen YP, Bogl HP, Wang CL, Woisetschlager M, Schilcher J. Deep neural networks with promising diagnostic accuracy for the classification of atypical femoral fractures. Acta Orthop. (2021) 92:394–400. doi: 10.1080/17453674.2021.1891512
36. Olczak J, Emilson F, Razavian A, Antonsson T, Stark A, Gordon M. Ankle fracture classification using deep learning: automating detailed AO foundation/orthopedic trauma association (Ao/Ota) 2018 malleolar fracture identification reaches a high degree of correct classification. Acta Orthop. (2020) 92:102–8. doi: 10.1080/17453674.2020.1837420
37. Guo J, Mu YX, Xue D, Li HQ, Chen JX, Yan HX, et al. Automatic analysis system of calcaneus radiograph: rotation-invariant landmark detection for calcaneal angle measurement, fracture identification and fracture region segmentation. Comput Meth Programs Biomed. (2021) 206:13. doi: 10.1016/j.cmpb.2021.106124
38. Murata K, Endo K, Aihara T, Suzuki H, Sawaji Y, Matsuoka Y, et al. Artificial intelligence for the detection of vertebral fractures on plain spinal radiography. Sci Rep. (2020) 10:8. doi: 10.1038/s41598-020-76866-w
39. Chen WJ, Liu X, Li KH, Luo Y, Bai SW, Wu JF, et al. A deeplearning model for identifying fresh vertebral compression fractures on digital radiography. Eur Radiol. (2022) 32:1496–505. doi: 10.1007/s00330-021-08247-4
40. Yabu A, Hoshino M, Tabuchi H, Takahashi S, Masumoto H, Akada M, et al. Using artificial intelligence to diagnose fresh osteoporotic vertebral fractures on magnetic resonance images. Spine J. (2021) 21:1652–8. doi: 10.1016/j.spinee.2021.03.006
41. Suri A, Jones BC, Ng G, Anabaraonye N, Beyrer P, Domi A, et al. A deep learning system for automated, multi-modality 2d segmentation of vertebral bodies and intervertebral discs. Bone. (2021) 149:9. doi: 10.1016/j.bone.2021.115972
42. Antony J, McGuinness K, O'Connor NE, Moran K IEEE (editors). Quantifying radiographic knee osteoarthritis severity using deep convolutional neural networks. 23rd International Conference on Pattern Recognition (ICPR) Mexico (2016).
43. Tiulpin A, Thevenot J, Rahtu E, Lehenkari P, Saarakkala S. Automatic knee osteoarthritis diagnosis from plain radiographs: a deep learning-based approach. Sci Rep. (2018) 8:10. doi: 10.1038/s41598-018-20132-7
44. Tiulpin A, Saarakkala S. Automatic grading of individual knee osteoarthritis features in plain radiographs using deep convolutional neural networks. Diagnostics. (2020) 10:12. doi: 10.3390/diagnostics10110932
45. Caliva F, Namiri NK, Dubreuil M, Pedoia V, Ozhinsky E, Majumdar S. Studying osteoarthritis with artificial intelligence applied to magnetic resonance imaging. Nat Rev Rheumatol. (2022) 18:112–21. doi: 10.1038/s41584-021-00719-7
46. Banach A, Strydom M, Jaiprakash A, Carneiro G, Eriksson A, Crawford R, et al. Visual localisation for knee arthroscopy. Int J Comput Assist Radiol Surg. (2021) 16:2137–45. doi: 10.1007/s11548-021-02444-8
47. Sarin JK, Nykanen O, Tiitu V, Mancini IAD, Brommer H, Visser J, et al. Arthroscopic determination of cartilage proteoglycan content and collagen network structure with near-infrared spectroscopy. Ann Biomed Eng. (2019) 47:1815–26. doi: 10.1007/s10439-019-02280-7
48. Seibold M, Maurer S, Hoch A, Zingg P, Farshad M, Navab N, et al. Real-Time acoustic sensing and artificial intelligence for error prevention in orthopedic surgery. Sci Rep. (2021) 11:11. doi: 10.1038/s41598-021-83506-4
49. Zhu WB, Zhang XZ, Fang SY, Wang B, Zhu C. Deep learning improves osteonecrosis prediction of femoral head after internal fixation using hybrid patient and radiograph variables. Front Med. (2020) 7:12. doi: 10.3389/fmed.2020.573522
50. Jang R, Choi JH, Kim N, Chang JS, Yoon PW, Kim C-H. Prediction of osteoporosis from simple hip radiography using deep learning algorithm. Sci Rep. (2021) 11:19997. doi: 10.1038/s41598-021-99549-6
51. Hsieh C-I, Zheng K, Lin C, Mei L, Lu L, Li W, et al. Automated bone mineral density prediction and fracture risk assessment using plain radiographs via deep learning. Nat Commun. (2021) 12:5472. doi: 10.1038/s41467-021-25779-x
52. Loffler MT, Jacob A, Scharr A, Sollmann N, Burian E, El Husseini M, et al. Automatic opportunistic osteoporosis screening in routine Ct: improved prediction of patients with prevalent vertebral fractures compared to Dxa. Eur Radiol. (2021) 31:6069–77. doi: 10.1007/s00330-020-07655-2
53. Zhang R, Huang L, Xia W, Zhang B, Qiu BS, Gao X. Multiple supervised residual network for osteosarcoma segmentation in Ct images. Comput Med Imaging Graph. (2018) 63:1–8. doi: 10.1016/j.compmedimag.2018.01.006
54. Fu Y, Xue P, Ji HZ, Cui WT, Dong EQ. Deep model with siamese network for viable and necrotic tumor regions assessment in osteosarcoma. Med Phys. (2020) 47:4895–905. doi: 10.1002/mp.14397
Keywords: orthopedics, deep learning, bibliometric analysis, research trends, Citespace
Citation: Feng C, Zhou X, Wang H, He Y, Li Z and Tu C (2022) Research hotspots and emerging trends of deep learning applications in orthopedics: A bibliometric and visualized study. Front. Public Health 10:949366. doi: 10.3389/fpubh.2022.949366
Received: 20 May 2022; Accepted: 27 June 2022;
Published: 19 July 2022.
Edited by:
Peter Kokol, University of Maribor, SloveniaReviewed by:
Shuogui Xu, Second Military Medical University, ChinaArsene Daniel Nyalundja, Association of Future African Neurosurgeons (AFAN), Cameroon
Copyright © 2022 Feng, Zhou, Wang, He, Li and Tu. This is an open-access article distributed under the terms of the Creative Commons Attribution License (CC BY). The use, distribution or reproduction in other forums is permitted, provided the original author(s) and the copyright owner(s) are credited and that the original publication in this journal is cited, in accordance with accepted academic practice. No use, distribution or reproduction is permitted which does not comply with these terms.
*Correspondence: Chao Tu, dHVjaGFvQGNzdS5lZHUuY24=