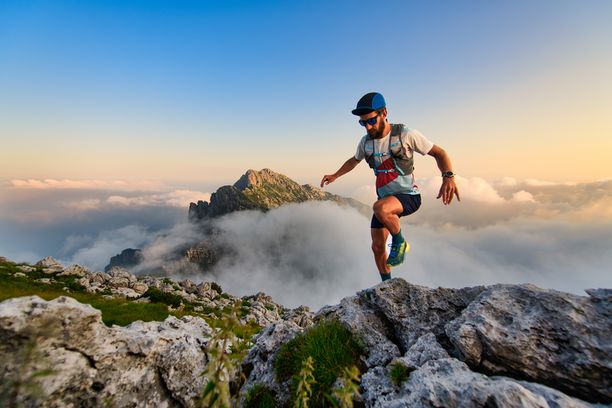
94% of researchers rate our articles as excellent or good
Learn more about the work of our research integrity team to safeguard the quality of each article we publish.
Find out more
ORIGINAL RESEARCH article
Front. Public Health, 11 August 2022
Sec. Digital Public Health
Volume 10 - 2022 | https://doi.org/10.3389/fpubh.2022.944790
Objective: In an era of increasingly expensive intensive care costs, it is essential to evaluate early whether the length of stay (LOS) in the intensive care unit (ICU) of obesity patients with sepsis will be prolonged. On the one hand, it can reduce costs; on the other hand, it can reduce nosocomial infection. Therefore, this study aimed to verify whether ICU prolonged LOS was significantly associated with poor prognosis poor in obesity patients with sepsis and develop a simple prediction model to personalize the risk of ICU prolonged LOS for obesity patients with sepsis.
Method: In total, 14,483 patients from the eICU Collaborative Research Database were randomized to the training set (3,606 patients) and validation set (1,600 patients). The potential predictors of ICU prolonged LOS among various factors were identified using logistic regression analysis. For internal and external validation, a nomogram was developed and performed.
Results: ICU prolonged LOS was defined as the third quartile of ICU LOS or more for all sepsis patients and demonstrated to be significantly associated with the mortality in ICU by logistic regression analysis. When entering the ICU, seven independent risk factors were identified: maximum white blood cell, minimum white blood cell, use of ventilation, Glasgow Coma Scale, minimum albumin, maximum respiratory rate, and minimum red blood cell distribution width. In the internal validation set, the area under the curve was 0.73, while in the external validation set, it was 0.78. The calibration curves showed that this model predicted probability due to actually observed probability. Furthermore, the decision curve analysis and clinical impact curve showed that the nomogram had a high clinical net benefit.
Conclusion: In obesity patients with sepsis, we created a novel nomogram to predict the risk of ICU prolonged LOS. This prediction model is accurate and reliable, and it can assist patients and clinicians in determining prognosis and making clinical decisions.
With modern intensive care medicine headed toward an era where economy and efficiency are valued, it is becoming increasingly necessary to accelerate the turnover of intensive care unit (ICU) beds and implement planned bed management (1). Intensive care medicine will transition from a reactive to a proactive discipline in a few years, becoming predictive, personalized, preventive, and participatory (P4) (2), to effectively prevent the adverse prognosis of various diseases in ICU.
Sepsis in the ICU continues to be one of the leading causes of life-threatening conditions, leading to dysfunction of vital organs due to a dysregulated host response to infection, and remains a major global public health problem (3–6). It is estimated that ~15–19 million people die from sepsis worldwide each year (7). The incidence and cost of sepsis has been steadily increasing in recent years due to several factors, one being the emergence of drug-resistant and more lethal pathogens, the other being the aging of society and the malnutrition, poverty and lack of access to medicines in developing countries. Often sepsis, especially severe sepsis, requires transfer to an ICU for appropriate medical care. In the United States, the number of severe sepsis cases rose by 71% between 2003 and 2007, and the total cost of all severe sepsis patients grew by 57% over the same period (8). In 2008, the treatment cost of sepsis in the United States was ~$14.6 billion (9). In most cases, the treatment of sepsis is ranked as one of the costliest diseases in any hospital (10–12).
Body Mass Index (BMI) is defined as obesity that is calculated as weight (kg)/height2 (m2). The World Health Organisation uses the definition of overweight and obesity as a disorder of excess or abnormal fat, which will increase health risks. The prevalence of obesity is constantly increasing throughout the world, with ~20% of ICU patients (13). Compared with normal or underweight, overweight or obesity is associated with significantly higher survival rates, called the obesity paradox. This has been observed in a variety of diseases, including coronary artery disease (14), coronavirus disease 2019 (COVID-19) (15), acute respiratory diseases (16), infection diseases (5, 17), or critical illness in general (13, 18–23). Similarly, this phenomenon also exists in sepsis (6, 24–31). In addition, many studies showed that obesity patients with sepsis have longer hospital or ICU length of stay (LOS) than non-obesity groups (19, 24, 31, 32). For instance, a large retrospective cohort study showed that obesity patients with sepsis tended to ICU prolonged LOS (p-LOS) than non-obesity groups (24). In general, p-LOS not only leads to an increase in hospitalization or ICU costs but also implies an increase in the risk of hospital-acquired infections (33). In the United States and Canada, researchers have confirmed that a reduction in ICU service levels can result in hospital cost savings (34). Early identification and correction of potential risk factors for ICU p-LOS in obesity patients with sepsis is therefore critical from a clinical and financial standpoint.
All in all, the purpose of our retrospective analysis was two-fold. First, based on an extensive database, we evaluated the differences of ICU LOS among different BMI groups and verified whether ICU p-LOS was significantly associated with adverse prognosis in obesity patients with sepsis. Second, if ICU p-LOS does be an independent predictor of poor prognosis in obesity patients with sepsis, we will develop a predictive nomogram, a simple graphical representation of the scoring model. By constructing a multiple variable regression model, such as logistic regression, a score is assigned to each value level of each influencing factor according to the degree of influence of each influencing factor on the outcome variable (the magnitude of the regression coefficient), and then the scores are summed to obtain the total score. Finally, the predicted probability of the outcome event for the individual is calculated through a functional transformation relationship between the total score and the probability of the outcome event. The nomogram transforms complex regression equations into simple and visual graphs, making the results of the prediction model more readable and of greater use. This advantage has led to an increased interest and use of nomograms in medical research and clinical practice in recent years (35). The nomogram can be customized to predict the likelihood of ICU p-LOS in obesity sepsis patients. Thus, assisting physicians and nurses in selecting the appropriate treatment plan, increasing ICU bed turnover, and reducing sepsis-related ICU medical costs.
We initiated an observational study using data from the eICU Collaborative Research Database (eICU-CRD), which contains deidentified health-related data from over 200,000 admissions to ICUs monitored by The Philips eICU Program at 208 hospitals between 2014 and 2015 across the United States (36). To comply with the US Health Insurance Portability and Accountability Act (HIPAA), all tables in this database are deidentified. Furthermore, our external validation set was derived from the Medical Information Mart for Intensive Care IV database (MIMIC-IV), which contains comprehensive and high-quality hospitalization data admitted to the Higher Medical Center in Boston, Massachusetts, from 2008 to 2019 (37). The requirement for individual patient consent is irrelevant because all data is anonymous. The author, Chen, extracted the data in our study after completing a National Institutes of Health web-based training course and the Protection of Human Research Participants Examination (No. 36328122).
The Third International Consensus Definition of Sepsis (Sepsis-3) is the currently accepted diagnostic criteria for sepsis (4), but the eICU-CRD was established well before 2016, and microbiological culture results were largely unavailable. Therefore, using the Acute Physiology and Chronic Health Evaluation IV (APACHE IV) diagnostic system in eICU-CRD, we included all patients with a first admission diagnosis of sepsis coded by trained eICU-CRD clinicians (38, 39). The exclusion criteria were as follows: I. missing APACHE IV score; II. missing accurate weight and height records on admission; III. admission < 24 h; IV. sequential organ failure assessment (SOFA) score < 2; V. missing underlying information such as sex. Subsequently, we excluded non-obesity patients (BMI < 30 kg/m2) from the first cohort as the second cohort. In addition, to independently assess predictive model performance, the MIMIC-IV obesity sepsis cohort was used as an external validation set, with similar exclusion criteria.
We used PgAdmin (version 4.24) to run structure query language (SQL) to extract data from eICU-CRD. We retrospectively collected the following data: (1) demographic data: age, gender, ethnicity, admission height and admission weight; (2) sites of infection: pulmonary, renal, gastrointestinal tract, skin/soft tissue; (3) vital signs: heart rates, respiratory rates, mean arterial pressure, temperature; (4) severity score: APACHE IV, SOFA, Glasgow coma scale (GCS); (5) comorbidities: chronic obstructive pulmonary disease, chronic kidney disease, shock history, liver disease, hypertension, congestive heart failure, coronary heart disease, malignant tumors, diabetes, which were encoded and defined in the APACHE IV diagnosis system or in the International Classification of Diseases, Ninth/Ten Revision (ICD-9/10); (6) laboratory results: sodium, potassium, hemoglobin, calcium, red cell volume distribution width (RDW), white blood cell, creatinine, lactate, blood urea nitrogen (BUN), albumin, red blood cell (RBC), platelet, mean red cell volume (MCV), glucose, bilirubin, bicarbonate, chloride; (7) intervention-associated information: ventilation, vasopressors, dialysis. Our inclusion period is 24 h before and after entering the ICU. For vital signs and laboratory results, we kept their maximum and minimum values.
We extracted the following outcome variables: (1) ICU LOS; (2) hospital LOS; (3) all-cause ICU mortality; (4) all-cause hospital mortality. ICU LOS was the primary endpoint; others were the secondary endpoints. Furthermore, the secondary endpoints were extracted solely for the purpose of descriptive analysis.
Our statistical analyses and graphs were conducted in SPSS for Windows (Version 26.0), MedCalc (Version 20.015), GraphPad (Version 9.0.0) and R (Version 4.0.3). Continuous variables were described as mean ± standard deviation (SD) or median with interquartile range (IQR). Patient's demographic and clinical characteristics were compared using Student' t-test, Mann–Whitney U test or chi-squared test, as appropriate.
The first cohort was the cohort obtained by excluding all sepsis admissions from the EICU database by our exclusion criteria. In the first cohort, we compared the ICU LOS of four groups by one-way analysis of variance (ANOVA) to confirm whether sepsis patients in the obesity group had a trend toward longer ICU LOS.
The second cohort was the cohort obtained by including obesity patients of the first cohort. In the second cohort, logistic regression analysis was used to see if ICU p-LOS was an independent risk factor for sepsis-related ICU mortality. In addition, to reduce the interference of data deviation and confounding factors, propensity score matching (PSM) was performed between the ICU p-LOS and non-ICU p-LOS cohorts (40).
Obesity patients with sepsis in the second cohort were randomly assigned to a training set and an internal validation set in a 7:3 proportion during the nomogram development process. Then, in the training set, we used univariate logistic regression analysis to screen for variables linked to ICU p-LOS, and the magnitude of the relationship was measured using an odds ratio (OR) with a 95% confidence interval (CI). APACHE IV and SOFA were excluded from the nomogram because they were collinear with other variables in our study. Furthermore, the infection sites were left out of the model because they were dependent on microbial culture, which was a time-consuming process. After that, a stepwise multivariate logistic regression analysis was used to identify independent risk factors for predicting ICU p-LOS by selecting variables with univariate P-values 0.05. We used the variance inflation factor (VIF) to test for collinearity between continuous variables, and arithmetic square root of VIF 2 was considered non-collinearity. The Hosmer-Lemeshow test was used to assess the logistic regression model's fitness, with a P-value of >0.05 indicating a good fit. Based on the multivariate logistic regression analysis result, we would build a model to achieve the research purpose with fewer variables according to Occam's razor law (41). Finally, based on our model, using R software with RMS package (version 6.2-0) and DynNom package (version 5.0.1), the conventional nomogram and the newly developed interactive web dynamic nomogram were obtained by the training set. The area under the curve (AUC) of the receiver operating characteristic (ROC) curves and calibration with the bootstrap method with 1,000 resampling were used to evaluate the nomogram's performance in all sets. In addition, to compare discrimination slopes and evaluate model fitness, we calculated the integrated discrimination improvement (IDI). Then, in all sets, we used Decision Curve Analysis (DCA) and generated Clinical Impact Curves (CIC) to assess the net benefit of medical interventions that conformed to the nomogram at various threshold probabilities. All analyses were presented in the form of a transparent multivariable prediction model for individual prognosis or diagnosis (TRIPOD) (42). The maximum missing value of all variables did not exceed 25%. Multiple interpolation processes the missing values in logistic regression and model construction. All reported P-values were two-tailed, and statistical significance was defined as P < 0.05.
After being screened using the inclusion and exclusion criteria (Figure 1), a total of 14,483 sepsis patients were included in the first cohort (Table 1). We divided all sepsis patients into four groups based on the WHO BMI classification standard for obesity categories: underweight (18.5 kg/m2), normal weight (18.5–24.9 kg/m2), overweight (25–29.9 kg/m2), and obesity (30 kg/m2). We found that the median ICU LOS of obesity group was [2.97 days (IQR, 1.87–5.56 days)] which was significantly longer than the other three groups (P < 0.001) (Supplementary Table S1). In EICU, the third quartile value of ICU LOS for all sepsis patients was about 5 days; thus, patients with ICU LOS of 5 days or more were considered to have ICU p-LOS in training set and internal validation set. The results showed that the proportion of ICU p-LOS in the obesity group was 28.43% which was also significantly more than the other groups (p < 0.001). Furthermore, the results revealed that the obesity group made up the largest proportion of the overall cohort, accounting for more than half of the total. Obesity patients were younger and had the lowest male-to-female ratio. Notably, the obesity group had significantly lower ICU and hospital mortality rates than the non-obesity groups.
Table 1 showed the characteristics of all obesity participants at baseline and on the first day of ICU admission, as well as participants in the ICU p-LOS and non-ICU p-LOS groups. ICU LOS was prolonged in ~28% of patients, with no significant difference between the two groups in terms of gender, with males accounting for a lower proportion than females. And that patients with ICU p-LOS were more likely to suffer from chronic obstructive pulmonary disease (COPD), chronic kidney disease (CKD), liver disease, hypertension and congestive heart failure (CHF). We also found that ICU mortality of ICU p-LOS and non-ICU p-LOS groups was 188 (12.70%) and 268 (7.19%), respectively, with a statistical difference of p < 0.001, while hospital mortality was similar. Furthermore, clinical variables such as the majority of vital signs, severity scores, laboratory results, and intervention use were significantly different between the two groups, indicating significant heterogeneity (Table 2).
Table 2. Baseline clinical and laboratory characteristics of obesity patients with sepsis (n = 5,206).
After adjusting for baseline characteristics, vital signs, laboratory tests, and infection site, the results of the univariate and multivariate logistic regressions revealed that ICU p-LOS was an independent risk factor for ICU mortality of the participants [odds ratio (OR) = 1.88, 95% confidence interval (CI) 1.54–2.29, p < 0.001; OR = 1.39, 95% CI 1.08–1.79, p = 0.012; respectively] (Table 3). Following PSM between ICU p-LOS and non-ICU p-LOS groups based on differences in baseline characteristics, vital signs, laboratory results, and infection sites (Supplementary Table S2), results of univariate and multivariate logistic regression after adjusting for baseline characteristics, vital signs, laboratory results, and infection sites revealed that ICU p-LOS was an independently risk factor for ICU mortality of the participants [OR = 1.34, 95% CI 1.03–1.73, p = 0.027; OR = 1.41, 95% CI 1.04–1.91, p = 0.026; respectively] (Table 3). Above all, the results showed that whether PSM was used or not, there were significant differences in ICU mortality between the ICU p-LOS and non-ICU p-LOS groups. Therefore, constructing a comprehensive nomogram provides the accurate and straightforward personalized prediction of ICU p-LOS with clinical utility.
Table 3. Correlation of ICU p-LOS with ICU mortality of obesity patients with sepsis in the original and post-PSM cohort.
The training set (3,606 patients) and internal validation set (1,600) of 5,206 obesity patients with sepsis were assigned at random. The model was created to predict the likelihood of ICU p-LOS in obesity patients with sepsis. In Supplementary Table S3, all variables of the patients in each set were listed. The results revealed that there was no statistical difference between the two groups in all variables. Figure 2 showed the results of the univariate logistic analysis using the training set.
Figure 2. The univariate logistic analysis of obese patients with sepsis in the training set. BMI, body mass index; LOS, length of stay; ICU, intensive care unit; GCS, Glasgow coma scale; COPD, chronic obstructive pulmonary diseases; CKD, chronic kidney disease; LD, liver disease; CHF, congestive heart failure; RDW, red cell volume distribution width; WBC, white blood cell; BUN, blood urea nitrogen.
Following that, we used variables with p < 0.05 in the univariable logistic analysis, those with clinical significance, or categorical variables with a set of meaningful values in a multivariate logistic regression. Figure 3 showed the risk factors identified by multivariable logistic regression that were independently related to ICU p-LOS of obesity patients with sepsis. Regarding the collinearity of the variables, the VIF was calculated and visualized in Supplementary Figure S1. The result was <2, which means no collinearity in the regression analysis. Then we calculated the relative importance of predictor variables (Supplementary Figure S2). According to the Occam's Law of Razor (41), we excluded chronic kidney disease, minimum serum creatinine, maximum mean blood pressure, age, hypertension, and chronic obstructive pulmonary disease, since they only explained <10% of Logistic regression cumulative deviance explained. Finally, a model integrating maximum WBC, minimum WBC, use of ventilation, GCS, minimum albumin, maximum respiratory rate, and minimum RDW was established. The Hosmer-Lemeshow test yielded a P-value of 0.432, indicating that the model was well fitted. A nomogram was also plotted based on this model to predict the probability of ICU p-LOS in obesity patients with sepsis (Figure 4).
Figure 3. The multivariable logistic regression of obese patients with sepsis in the training set. GCS, Glasgow coma scale; CKD, chronic kidney disease; RDW, red cell volume distribution width; WBC, white blood cell; COPD, chronic obstructive pulmonary diseases.
Figure 4. Nomogram for predicting the probability of ICU p-LOS in obese patients with sepsis. RDW, red cell distribution width; GCS, Glasgow coma scale; WBC, white blood cell; ICU, intensive care unit; p-LOSz, prolonged length of stay.
Our external validation set, derived from the MIMIC IV database, was based on similar inclusion criteria and extracted relevant seven variables, excluding the samples with missing values, and 2,424 samples were finally obtained. In the external validation set, the third quartile value of ICU LOS was about 6 days, so patients with an ICU LOS of 6 days or more were considered to have ICU p-LOS in the external validation set.
The ROC curves in Figures 5A–C showed that our nomogram not only had an excellent discriminative ability in internal validation set (AUC = 0.73, 95% CI 0.71–0.75), but also a good discriminative ability in external validation (AUC = 0.78, 95% CI 0.76–0.80).
Figure 5. The receiver operating characteristic curves (ROC) in the training set (A), internal validation set (B) and external validation set (C). AUC, area under curve; Cl, confidence interval.
For ICU performance benchmarking and quality improvement analysis, APACHE IV was used to risk-adjust ICU patients. Besides, APACHE IV had been validated for predicting ICU LOS (38, 39). SOFA, like APACHE IV, was also shown to be a predictor of ICU LOS alone (43). By comparing their AUC and IDI to judge the performance of nomogram, we found that the discrimination performance of nomogram for ICU p-LOS was significantly better than APACHE IV and SOFA (P < 0.001), our nomogram improved the performance of them by about 10–15% (Table 4).
Table 4. Comparison of models in predicting the probability of ICU p-LOS of obesity patients with sepsis.
For all of the training, internal validation, and external validation sets, the calibration curves were described using the bootstrap method. In all sets, the apparent curve and bias-corrected curve deviated slightly from the reference line, but there was still good agreement between observation and prediction. Figures 6A–C depicted the details. Furthermore, the nomogram's Brier scores in the training set were 0.172, 0.178 in the internal validation set, and 0.197 in the external validation set, indicating that the nomogram's prediction calibration was good.
Figure 6. The calibration curves for the nomogram in the training set (A), internal validation set (B) and external validation set (C).
To perform a clinical application of this nomogram, we plotted the DCA curve. In three sets of circumstances, medical intervention guided by this nomogram could provide an excellent net benefit. Figures 7A–C depicted the details. We presented the nomogram's clinical impact curve (CIC) based on the DCA. Figures 8A–C depicts the outcomes. The solid red curve (number of high-risk individuals) represented the number of patients classified as positive (high risk) by the nomogram at each 1,000-patient threshold, while the dotted blue curve (number of true positive patients) represented the number of true positive patients under each risk threshold. CIC confirmed the clinical value of the nomogram by visually indicating that it provided a high clinical net benefit. Besides, to facilitate convenient clinical use, an online dynamic nomogram (https://cy19940626.shinyapps.io/DynNomapp/) based on this model was built.
Figure 7. The decision curves analysis (DCA) for the nomogram in the training set (A), internal validation set (B) and external validation set (C).
Figure 8. The clinical impact curves (CIC) for the nomogram in the training set (A), internal validation set (B) and external validation set (C).
The nomogram was found to be a useful predictive model with high sensitivity, specificity, positive predictive value, and negative predictive value in determining whether the ICU LOS of obesity patients with sepsis was relatively prolong, with 0.68 (95% CI: 0.65, 0.71), 0.69 (95% CI: 0.67, 0.71), 0.46 (95% CI: 0.44, 0.50) and 0.85 (95% CI: 0.83, 0.86) in the training set; 0.70 (95% CI: 0.65, 0.74), 0.68 (95% CI: 0.65, 0.71), 0.47 (95% CI: 0.44, 0.53), and 0.84 (95% CI: 0.82, 0.86) in the internal validation set; 0.69 (95% CI: 0.67, 0.72), 0.76 (95% CI: 0.73, 0.78), 0.72 (95% CI: 0.69, 0.74), 0.74 (95% CI: 0.71, 0.96) in the external validation set; respectively.
In our retrospective overall sepsis cohort of the EICU database (14,483 sepsis patients), the obesity group had a longer ICU LOS than the other three non-obesity groups. Meanwhile, there was a trend of ICU p-LOS in the obesity group, which was consistent with the conclusions of previous reports. Moreover, the findings revealed that the obesity group in our sepsis cohort had lower mortality than the other three non-obesity groups, providing new clinical evidence for the obesity paradox of sepsis.
In the obesity patients with sepsis cohort, we found that ICU p-LOS was an independent risk factor for ICU mortality regardless of PSM implementation. To identify the independent risk factor associated with the ICU p-LOS of obesity patients with sepsis in the ICU, we used univariate and multivariate logistic regression analyses. Finally, seven clinical variables were identified and incorporated into the best-fitting model, which was visualized as a prediction nomogram, that is, maximum WBC, minimum WBC, use of ventilation, GCS, minimum albumin, maximum respiratory rate, and minimum RDW. To our knowledge, this is the first study to examine the relationship between ICU p-LOS and ICU mortality in obesity patients with sepsis, as well as to identify relevant independent risk factors for ICU p-LOS and to develop a nomogram to predict it.
Among these seven included factors, the WBC count accounted for the most prominent weight in the nomogram. As we all recognize, the principal function of white blood cells is defense. Sepsis can be thought of as a death race between pathogens and the immune system of the host (3). An empirical model revealed that losing lymphocytes could lead to heightened mortality from sepsis. Patients with a higher NLCR had a higher white blood cell count, higher neutrophil count, lower lymphocyte count, and higher 28-day mortality or longer ICU LOS, according to a large cohort study (44). Another study found that monocyte PDL1 expression is an independent predictor of sepsis-related 28-day mortality in patients (45). Above all, we can conclude that WBC counts are considerably associated with poor prognosis in patients with sepsis or septicemia. The smaller the WBC count, the stronger the immunosuppressive response. Likewise, the conclusion was also applied to obesity patients. The innate immune system has been a primary element in established obesity (46). Furuncuoglu et al. and Maurizi et al. showed that BMI was significantly positively correlated with neutrophil, lymphocyte and WBC counts (47, 48). Several possible reasons could reveal the interrelationship between the WBC and adverse prognosis for sepsis or obesity patients, despite the immune mechanisms involved being unclear. Numerous studies have determined that many patients who died of sepsis or MODS had immunosuppressive features (49). The delayed apoptosis of neutrophils and the appearance of immature band-like neutrophils in peripheral blood with antimicrobial effector function deficits, including oxidative burst capacity, is a key finding in sepsis (50). Moreover, increased lymphocyte apoptosis in the thymus and spleen contributes to immunosuppression, sepsis and obesity (51, 52).
During sepsis, patients required invasive ventilation, and among these patients, supplemental oxygen was also essential (53). A single-center RCT pointed out that mechanical ventilation and usual oxygen therapy could have a clinically meaningful effect on the poor prognosis of patients. Meanwhile, they found that when compared to standard oxygen therapy, mechanical ventilation did not result in a statistically significant reduction in 90-day mortality (54). Currently, RDW is considered to be a crucial factor for human mortality in various diseases: hematological malignancies (55), cardiovascular diseases (56), and critical illness (57). RDW was associated with mortality in patients with sepsis, according to Zhang et al.'s findings, and it could be a useful and simple prognostic marker for patients with sepsis (58). Furthermore, RDW was found to be associated with traditional inflammatory biomarkers independently by Lippi et al. (59). In a recent cohort study that concluded RDW was more related to the prognosis of patients whose BMI was >25, there was also a significant interaction between RDW and BMI in terms of all-cause mortality (60). In addition, some vital signs and scores are used to predict sepsis. As demonstrated by Wijdicks et al., Glasgow Coma Scale score was an independent predictor of mortality in ICU patients (61). GCS and an abnormal respiratory rate, according to Lane et al., can identify patients with a higher morbidity and mortality of sepsis (62). In a randomized controlled trial, administration of albumin may have decreased the risk of death, which indicated it was associated with albumin with the prognostics of sepsis patients (63). In short, it strongly associated all seven of these inclusion factors in the nomogram with poor prognosis in sepsis or obesity patients.
Our nomogram is concise, practical containing only seven clinical variables. Based on the AUROC and calibration curve, it also shows acceptable discrimination and good calibration ability. It effectively calculates the risk probability of ICU p-LOS for obesity patients with sepsis; therefore, it provides early information on ICU p-LOS for sepsis with obesity patients admitted to the ICU and helps ICU clinicians to develop strategies and plans accordingly in advance. When considering that a particular sepsis with obesity patient is at high risk of ICU p-LOS (at least above 50%), on the one hand, there may be a possibility of shortening the ICU LOS by targeting the corresponding indicators based on the independent risk factors for ICU p-LOS in sepsis with obesity patients identified in this study, but further rigorous clinical studies are needed to verify the effectiveness. On the other hand, based on experience in the treatment and management of sepsis in the ICU, we recommend that (1) The ICU physician should develop a preferred empirical treatment strategy based on local patterns of resistance, the most prevalent pathogens associated with the known or suspected sites of sepsis infection, any host parameters associated with the risk of uncommon or drug-resistant pathogens, and considering both local and national guidelines and local antimicrobial sensitivities, and when a high risk of ICU p-LOS in sepsis with obesity patient is identified, initiate appropriate empirical antibiotic therapy to reduce ICU LOS and improve clinical outcomes in the ICU. (2) Gram staining and culture, molecular diagnostics and calcitoninogen monitoring are novel tools whose application has the potential to enhance the ability of clinical practitioners to administer antibiotics and can facilitate more effective, targeted antibiotic therapy for sepsis. (3) Therapeutic drug monitoring is a useful strategy to facilitate more accurate application of antibiotic doses to avoid under-dosing leading to possible antibiotic resistance, while encouraging clinicians to reduce the total duration of antibiotic therapy and lower antibiotic levels during a course of treatment. (4) Given the distribution of patients in the ICU, changes in elimination and the risk of resistance, an individual approach to dosing should also be adopted and, if possible, clinicians should consult the ICU pharmacist to further refine antibiotic regimens to sepsis.
Notwithstanding that it based our study on a combination of many ICUs over the entire mainland United States, it even retains several limitations. Firstly, our data were from the USA, therefore the results may not apply to ICUs in other countries. Second, this was a retrospective analysis in which recall bias was necessary. Thus, a prospective cohort study was required for further validation. Third, as seen in most of previous studies, the absolute days of stay in ICU varied from one hospital to another. Although our nomogram demonstrated a promising prediction capability within the internal eICU-CRD and MIMIC IV. However, scalability to other hospitals remains an issue and needs to be used with caution. Therefore, when other hospitals would apply this nomogram, they should start by surveying the ICU LOS across the institution and calculating the third quartile of sepsis pat to determine their own LOS prolongation threshold. Fourth, BMI is not the only way to define obesity, other indicators that can be used to define obesity include: waist to hip ratio, visceral obesity index and waist to height ratio. However, as this is a retrospective study with data from EICU and MIMIC IV, the lack of these indicators leaves us with BMI as the only way to define obesity. Therefore, there is a need for a prospective study in the future to define obesity by various indicators in order to study the role of obesity in sepsis or other diseases, which may lead to some new findings. To sum up, our nomogram is quite promising and worthy of further exploration in future clinical work and research.
We investigated specific predictors of ICU p-LOS in obesity patients with sepsis. We constructed a new nomogram to predict the risk of ICU p-LOS in obesity patients with sepsis using seven risk factors (maximum WBC, minimum WBC, use of ventilation, GCS, minimum albumin, maximum respiratory rate, and minimum RDW). This prediction model is accurate and reliable, and it can assist patients and clinicians in determining prognosis and clinical decisions.
The raw data supporting the conclusions of this article will be made available by the authors, without undue reservation.
Ethical review and approval was not required for the study on human participants in accordance with the local legislation and institutional requirements. Written informed consent for participation was not required for this study in accordance with the national legislation and the institutional requirements.
Research was designed by YaC, ML, YH, and QH. ML and YaC conducted experiments and analyzed data. The main manuscript text and figures were written by YaC, ML, YH, and YuC. The manuscript was edited and revised by YaC and QH. All authors contributed to the article and gave their approval to the final version.
China Education and Research Network (NGII20190706 and NGII20180703) funded this study.
We appreciate all of the investigators and subjects who took part in this study.
The authors declare that the research was conducted in the absence of any commercial or financial relationships that could be construed as a potential conflict of interest.
All claims expressed in this article are solely those of the authors and do not necessarily represent those of their affiliated organizations, or those of the publisher, the editors and the reviewers. Any product that may be evaluated in this article, or claim that may be made by its manufacturer, is not guaranteed or endorsed by the publisher.
The Supplementary Material for this article can be found online at: https://www.frontiersin.org/articles/10.3389/fpubh.2022.944790/full#supplementary-material
1. Ward NS, Chong DH. Critical care beds and resource utilization: current trends and controversies. Semin Respir Crit Care Med. (2015) 36:914–20. doi: 10.1055/s-0035-1564876
2. Nabbout R, Kuchenbuch M. Impact of predictive, preventive and precision medicine strategies in epilepsy. Nat Rev Neurol. (2020) 16:674–88. doi: 10.1038/s41582-020-0409-4
3. Evans L, Rhodes A, Alhazzani W, Antonelli M, Coopersmith CM, French C, et al. Surviving sepsis campaign: international guidelines for management of sepsis and septic shock 2021. Intensive Care Med. (2021) 47:1181–247. doi: 10.1007/s00134-021-06506-y
4. Singer M, Deutschman CS, Seymour CW, Shankar-Hari M, Annane D, Bauer M, et al. The third international consensus definitions for sepsis and septic shock (sepsis-3). JAMA. (2016) 315:801–10. doi: 10.1001/jama.2016.0287
5. Huttunen R, Syrjanen J. Obesity and the risk and outcome of infection. Int J Obes. (2013) 37:333–40. doi: 10.1038/ijo.2012.62
6. Jagan N, Morrow LE, Walters RW, Plambeck RW, Wallen TJ, Patel TM, et al. Sepsis and the obesity paradox: size matters in more than one way. Crit Care Med. (2020) 48:e776–82. doi: 10.1097/CCM.0000000000004459
7. Adhikari NKJ, Fowler RA, Bhagwanjee S, Rubenfeld GD. Critical care and the global burden of critical illness in adults. Lancet. (2010) 376:1339–46. doi: 10.1016/S0140-6736(10)60446-1
8. Lagu T, Rothberg MB, Shieh MS, Pekow PS, Steingrub JS, Lindenauer PK. Hospitalizations, costs, and outcomes of severe sepsis in the United States 2003 to 2007. Crit Care Med. (2012) 40:754–61. doi: 10.1097/CCM.0b013e318232db65
9. Hall MJ, Williams SN, DeFrances CJ, Golosinskiy A. Inpatient care for septicemia or sepsis: a challenge for patients and hospitals. NCHS Data Brief. (2011) 62:1–8.
10. Arefian H, Heublein S, Scherag A, Brunkhorst FM, Younis MZ, Moerer O, et al. Hospital-related cost of sepsis: a systematic review. J Infect. (2017) 74:107–17. doi: 10.1016/j.jinf.2016.11.006
11. Burchardi H, Schneider H. Economic aspects of severe sepsis: a review of intensive care unit costs, cost of illness and cost effectiveness of therapy. Pharmacoeconomics. (2004) 22:793–813. doi: 10.2165/00019053-200422120-00003
12. Paoli CJ, Reynolds MA, Sinha M, Gitlin M, Crouser E. Epidemiology and costs of sepsis in the United States-an analysis based on timing of diagnosis and severity level. Crit Care Med. (2018) 46:1889–97. doi: 10.1097/CCM.0000000000003342
13. Schetz M, De Jong A, Deane AM, Druml W, Hemelaar P, Pelosi P, et al. Obesity in the critically ill: a narrative review. Intensive Care Med. (2019) 45:757–69. doi: 10.1007/s00134-019-05594-1
14. Romero-Corral A, Montori VM, Somers VK, Korinek J, Thomas RJ, Allison TG, et al. Association of bodyweight with total mortality and with cardiovascular events in coronary artery disease: a systematic review of cohort studies. Lancet. (2006) 368:666–78. doi: 10.1016/S0140-6736(06)69251-9
15. Cho Y, Cho Y, Choi HJ, Lee H, Lim TH, Kang H, et al. The effect of BMI on COVID-19 outcomes among older patients in South Korea: a nationwide retrospective cohort study. Ann Med. (2021) 53:1292–301. doi: 10.1080/07853890.2021.1946587
16. Ni YN, Luo J, Yu H, Wang YW, Hu YH, Liu D, et al. Can body mass index predict clinical outcomes for patients with acute lung injury/acute respiratory distress syndrome? A meta-analysis. Crit Care. (2017) 21:36. doi: 10.1186/s13054-017-1615-3
17. Gribsholt SB, Pedersen L, Richelsen B, Sorensen HT, Thomsen RW. Body mass index and 90-day mortality among 35,406 Danish patients hospitalized for infection. Mayo Clin Proc. (2021) 96:550–62. doi: 10.1016/j.mayocp.2020.06.062
18. Abhyankar S, Leishear K, Callaghan FM, Demner-Fushman D, McDonald CJ. Lower short- and long-term mortality associated with overweight and obesity in a large cohort study of adult intensive care unit patients. Crit Care. (2012) 16:R235. doi: 10.1186/cc11903
19. Akinnusi ME, Pineda LA, El Solh AA. Effect of obesity on intensive care morbidity and mortality: a meta-analysis. Crit Care Med. (2008) 36:151–8. doi: 10.1097/01.CCM.0000297885.60037.6E
20. Alipoor E, Hosseinzadeh-Attar MJ, Rezaei M, Jazayeri S, Chapman M. White adipose tissue browning in critical illness: a review of the evidence, mechanisms and future perspectives. Obes Rev. (2020) 21:e13085. doi: 10.1111/obr.13085
21. Karampela I, Chrysanthopoulou E, Christodoulatos GS, Dalamaga M. Is there an obesity paradox in critical illness? Epidemiologic and metabolic considerations. Curr Obes Rep. (2020) 9:231–44. doi: 10.1007/s13679-020-00394-x
22. Pickkers P, de Keizer N, Dusseljee J, Weerheijm D, van der Hoeven JG, Peek N. Body mass index is associated with hospital mortality in critically ill patients: an observational cohort study. Crit Care Med. (2013) 41:1878–83. doi: 10.1097/CCM.0b013e31828a2aa1
23. Sakr Y, Alhussami I, Nanchal R, Wunderink RG, Pellis T, Wittebole X, et al. Being overweight is associated with greater survival in ICU patients: results from the intensive care over nations audit. Crit Care Med. (2015) 43:2623–32. doi: 10.1097/CCM.0000000000001310
24. Li S, Hu X, Xu J, Huang F, Guo Z, Tong L, et al. Increased body mass index linked to greater short- and long-term survival in sepsis patients: a retrospective analysis of a large clinical database. Int J Infect Dis. (2019) 87:109–16. doi: 10.1016/j.ijid.2019.07.018
25. Mewes C, Bohnke C, Alexander T, Buttner B, Hinz J, Popov AF, et al. Favorable 90-day mortality in obesity Caucasian patients with septic shock according to the sepsis-3 definition. J Clin Med. (2019) 9:46. doi: 10.3390/jcm9010046
26. Pepper DJ, Demirkale CY, Sun J, Rhee C, Fram D, Eichacker P, et al. Does obesity protect against death in sepsis? A retrospective cohort study of 55,038 adult patients. Crit Care Med. (2019) 47:643–50. doi: 10.1097/CCM.0000000000003692
27. Pepper DJ, Sun J, Welsh J, Cui X, Suffredini AF, Eichacker PQ. Increased body mass index and adjusted mortality in ICU patients with sepsis or septic shock: a systematic review and meta-analysis. Crit Care. (2016) 20:181. doi: 10.1186/s13054-016-1360-z
28. Prescott HC, Chang VW, O'Brien JM Jr., Langa KM, Iwashyna TJ. Obesity and 1-year outcomes in older Americans with severe sepsis. Crit Care Med. (2014) 42:1766–74. doi: 10.1097/CCM.0000000000000336
29. Wang S, Liu X, Chen Q, Liu C, Huang C, Fang X. The role of increased body mass index in outcomes of sepsis: a systematic review and meta-analysis. BMC Anesthesiol. (2017) 17:118. doi: 10.1186/s12871-017-0405-4
30. Weng L, Fan J, Yu C, Guo Y, Bian Z, Wei Y, et al. Body-mass index and long-term risk of sepsis-related mortality: a population-based cohort study of 0.5 million Chinese adults. Crit Care. (2020) 24:534. doi: 10.1186/s13054-020-03229-2
31. Papadimitriou-Olivgeris M, Aretha D, Zotou A, Koutsileou K, Zbouki A, Lefkaditi A, et al. The role of obesity in sepsis outcome among critically ill patients: a retrospective cohort analysis. Biomed Res Int. (2016) 2016:5941279. doi: 10.1155/2016/5941279
32. Zhao Y, Li Z, Yang T, Wang M, Xi X. Is body mass index associated with outcomes of mechanically ventilated adult patients in intensive critical units? A systematic review and meta-analysis. PLoS ONE. (2018) 13:e0198669. doi: 10.1371/journal.pone.0198669
33. Khullar OV, Fernandez FG, Perez S, Knechtle W, Pickens A, Sancheti MS, et al. Time is money: hospital costs associated with video-assisted thoracoscopic surgery lobectomies. Ann Thorac Surg. (2016) 102:940–7. doi: 10.1016/j.athoracsur.2016.03.024
34. Evans J, Kobewka D, Thavorn K, D'Egidio G, Rosenberg E, Kyeremanteng K. The impact of reducing intensive care unit length of stay on hospital costs: evidence from a tertiary care hospital in Canada. Can J Anaesth. (2018) 65:627–35. doi: 10.1007/s12630-018-1087-1
35. Balachandran VP, Gonen M, Smith JJ, DeMatteo RP. Nomograms in oncology: more than meets the eye. Lancet Oncol. (2015) 16:e173–80. doi: 10.1016/S1470-2045(14)71116-7
36. Pollard TJ, Johnson AEW, Raffa JD, Celi LA, Mark RG, Badawi O. The eICU Collaborative Research Database, a freely available multi-center database for critical care research. Sci Data. (2018) 5:180178. doi: 10.1038/sdata.2018.178
37. Johnson A, Bulgarelli L, Pollard T, Horng S, Celi LA, Mark R. MIMIC-IV (version 0.4). PhysioNet (2020). doi: 10.13026/a3wn-hq05
38. Yonekura H, Kawasaki Y, Nakamori Y, Kamei M. The use of sodium bicarbonate for metabolic acidosis in patients with acute kidney injury. Crit Care Med. (2020) 48:e251–2. doi: 10.1097/CCM.0000000000004111
39. Zimmerman JE, Kramer AA, McNair DS, Malila FM. Acute physiology and chronic health evaluation (APACHE) IV: hospital mortality assessment for today's critically ill patients. Crit Care Med. (2006) 34:1297–310. doi: 10.1097/01.CCM.0000215112.84523.F0
40. Elze MC, Gregson J, Baber U, Williamson E, Sartori S, Mehran R, et al. Comparison of propensity score methods and covariate adjustment: evaluation in 4 cardiovascular studies. J Am Coll Cardiol. (2017) 69:345–57. doi: 10.1016/j.jacc.2016.10.060
41. Van Den Berg HA. Occam's razor: from Ockham's via moderna to modern data science. Sci Prog. (2018) 101:261–72. doi: 10.3184/003685018X15295002645082
42. Moons KG, Altman DG, Reitsma JB, Ioannidis JP, Macaskill P, Steyerberg EW, et al. Transparent reporting of a multivariable prediction model for individual prognosis or diagnosis (TRIPOD): explanation and elaboration. Ann Intern Med. (2015) 162:W1–73. doi: 10.7326/M14-0698
43. Raith EP, Udy AA, Bailey M, McGloughlin S, MacIsaac C, Bellomo R, et al. Prognostic accuracy of the SOFA score, SIRS criteria, and qSOFA score for in-hospital mortality among adults with suspected infection admitted to the intensive care unit. JAMA. (2017) 317:290–300. doi: 10.1001/jama.2016.20328
44. Ye W, Chen X, Huang Y, Li Y, Xu Y, Liang Z, et al. The association between neutrophil-to-lymphocyte count ratio and mortality in septic patients: a retrospective analysis of the MIMIC-III database. J Thorac Dis. (2020) 12:1843–55. doi: 10.21037/jtd-20-1169
45. Shao R, Fang Y, Yu H, Zhao L, Jiang Z, Li CS. Monocyte programmed death ligand-1 expression after 3-4 days of sepsis is associated with risk stratification and mortality in septic patients: a prospective cohort study. Crit Care. (2016) 20:124. doi: 10.1186/s13054-016-1301-x
46. Bekkering S, Saner C, Riksen NP, Netea MG, Sabin MA, Saffery R, et al. Trained immunity: linking obesity and cardiovascular disease across the life-course? Trends Endocrinol Metab. (2020) 31:378–89. doi: 10.1016/j.tem.2020.01.008
47. Furuncuoglu Y, Tulgar S, Dogan AN, Cakar S, Tulgar YK, Cakiroglu B. How obesity affects the neutrophil/lymphocyte and platelet/lymphocyte ratio, systemic immune-inflammatory index and platelet indices: a retrospective study. Eur Rev Med Pharmacol Sci. (2016) 20:1300–6.
48. Maurizi G, Della Guardia L, Maurizi A, Poloni A. Adipocytes properties and crosstalk with immune system in obesity-related inflammation. J Cell Physiol. (2018) 233:88–97. doi: 10.1002/jcp.25855
49. Boomer JS, To K, Chang KC, Takasu O, Osborne DF, Walton AH, et al. Immunosuppression in patients who die of sepsis and multiple organ failure. JAMA. (2011) 306:2594–605. doi: 10.1001/jama.2011.1829
50. van der Poll T, van de Veerdonk FL, Scicluna BP, Netea MG. The immunopathology of sepsis and potential therapeutic targets. Nat Rev Immunol. (2017) 17:407–20. doi: 10.1038/nri.2017.36
51. Sell H, Habich C, Eckel J. Adaptive immunity in obesity and insulin resistance. Nat Rev Endocrinol. (2012) 8:709–16. doi: 10.1038/nrendo.2012.114
52. Cao C, Yu M, Chai Y. Pathological alteration and therapeutic implications of sepsis-induced immune cell apoptosis. Cell Death Dis. (2019) 10:782. doi: 10.1038/s41419-019-2015-1
53. Girardis M, Alhazzani W, Rasmussen BS. What's new in oxygen therapy? Intensive Care Med. (2019) 45:1009–11. doi: 10.1007/s00134-019-05619-9
54. Young P, Mackle D, Bellomo R, Bailey M, Beasley R, Deane A, et al. Conservative oxygen therapy for mechanically ventilated adults with sepsis: a post hoc analysis of data from the intensive care unit randomized trial comparing two approaches to oxygen therapy (ICU-ROX). Intensive Care Med. (2020) 46:17–26. doi: 10.1007/s00134-019-05857-x
55. Ai L, Mu S, Hu Y. Prognostic role of RDW in hematological malignancies: a systematic review and meta-analysis. Cancer Cell Int. (2018) 18:61. doi: 10.1186/s12935-018-0558-3
56. Turcato G, Serafini V, Dilda A, Bovo C, Caruso B, Ricci G, et al. Red blood cell distribution width independently predicts medium-term mortality and major adverse cardiac events after an acute coronary syndrome. Ann Transl Med. (2016) 4:254. doi: 10.21037/atm.2016.06.35
57. Salciccioli JD, Marshall DC, Pimentel MA, Santos MD, Pollard T, Celi LA, et al. The association between the neutrophil-to-lymphocyte ratio and mortality in critical illness: an observational cohort study. Crit Care. (2015) 19:13. doi: 10.1186/s13054-014-0731-6
58. Zhang L, Yu CH, Guo KP, Huang CZ, Mo LY. Prognostic role of red blood cell distribution width in patients with sepsis: a systematic review and meta-analysis. BMC Immunol. (2020) 21:40. doi: 10.1186/s12865-020-00369-6
59. Lippi G, Targher G, Montagnana M, Salvagno GL, Zoppini G, Guidi GC. Relation between red blood cell distribution width and inflammatory biomarkers in a large cohort of unselected outpatients. Arch Pathol Lab Med. (2009) 133:628–32. doi: 10.5858/133.4.628
60. Pan J, Borne Y, Engstrom G. The relationship between red cell distribution width and all-cause and cause-specific mortality in a general population. Sci Rep. (2019) 9:16208. doi: 10.1038/s41598-019-52708-2
61. Wijdicks EF, Kramer AA, Rohs T Jr., Hanna S, Sadaka F, et al. Comparison of the full outline of unresponsiveness score and the Glasgow coma scale in predicting mortality in critically ill patients. Crit Care Med. (2015) 43:439–44. doi: 10.1097/CCM.0000000000000707
62. Lane DJ, Wunsch H, Saskin R, Cheskes S, Lin S, Morrison LJ, et al. Epidemiology and patient predictors of infection and sepsis in the prehospital setting. Intensive Care Med. (2020) 46:1394–403. doi: 10.1007/s00134-020-06093-4
Keywords: nomogram, obesity, sepsis, ICU stay, eICU, MIMIC-IV
Citation: Chen Y, Luo M, Cheng Y, Huang Y and He Q (2022) A nomogram to predict prolonged stay of obesity patients with sepsis in ICU: Relevancy for predictive, personalized, preventive, and participatory healthcare strategies. Front. Public Health 10:944790. doi: 10.3389/fpubh.2022.944790
Received: 15 May 2022; Accepted: 18 July 2022;
Published: 11 August 2022.
Edited by:
Jiang Bian, University of Florida, United StatesReviewed by:
Zhe He, Florida State University, United StatesCopyright © 2022 Chen, Luo, Cheng, Huang and He. This is an open-access article distributed under the terms of the Creative Commons Attribution License (CC BY). The use, distribution or reproduction in other forums is permitted, provided the original author(s) and the copyright owner(s) are credited and that the original publication in this journal is cited, in accordance with accepted academic practice. No use, distribution or reproduction is permitted which does not comply with these terms.
*Correspondence: Qing He, MTIzaHFAMTYzLmNvbQ==
†These authors have contributed equally to this work
Disclaimer: All claims expressed in this article are solely those of the authors and do not necessarily represent those of their affiliated organizations, or those of the publisher, the editors and the reviewers. Any product that may be evaluated in this article or claim that may be made by its manufacturer is not guaranteed or endorsed by the publisher.
Research integrity at Frontiers
Learn more about the work of our research integrity team to safeguard the quality of each article we publish.