- 1Department of Urology, Affiliated Zhongda Hospital of Southeast University, Nanjing, China
- 2Department of Medical College, Southeast University, Nanjing, China
- 3Department of Chemotherapy, Affiliated the Second Hospital of Nanjing, Nanjing University of Chinese Medicine, Nanjing, China
- 4Department of Urology, Nanjing Lishui People's Hospital, Zhongda Hospital Lishui Branch of Southeast University, Nanjing, China
Background: Renal sarcoma (RS) is rarely seen in clinical practice. The purpose of this study was to develop a prognostic nomogram model, which could predict the probability of overall survival (OS) and cancer-specific survival (CSS) in adult patients with RS.
Methods: Patients diagnosed with RS were recruited from the SEER database between 2004 and 2015, and randomized to two cohorts: the training cohort and the validation cohort. Uni- and multivariate Cox regression analyses in the training cohort were used to screen independent prognostic factors for OS and CSS. Prognostic nomograms for OS and CSS were created separately for adult RS patients based on independent risk factors. The area under the receiver operating characteristic (ROC) curves, calibration curves, and decision curve analysis (DCA) were used to validate the nomograms.
Results: A total of 232 eligible patients were recruited, including 162 in the training cohort and 70 in the validation cohort. Sex, histological type, SEER stage, and surgery were independent prognostic factors for OS, while histological type, SEER stage, surgery, chemotherapy were independent prognostic factors for CSS. Based on the above independent prognostic factors, prognostic nomograms for OS and CSS were created respectively. In the training cohort, the AUCs of the nomograms for OS and CSS were 0.742 and 0.733, respectively. In the validation cohort, the AUCs of the nomograms for OS and CSS were 0.837 and 0.758, respectively. The calibration curves of the nomograms showed high consistencies between the predicted and actual survival rates. Finally, the DCA demonstrated that the nomograms in the wide high-risk threshold had a higher net benefit than the SEER stage.
Conclusion: A prognostic nomogram for renal sarcoma was created and validated for reliability and usefulness in our study, which assisted urologists in accurately assessing the prognosis of adult RS patients.
Introduction
Sarcomas are a heterogeneous group of tumors arising in the embryonic mesoderm, accounting for approximately 1% of all malignant tumors, of which <5% occur in the urogenital tract (1). Primary renal sarcoma (RS) accounts for around 24.6% of all genitourinary sarcomas and <1% of all primary kidney tumors (1, 2). Renal sarcoma is not only very rare but also leads to a poor prognosis: the overall 1-, 3-, and 5-year survival rate was 86.3, 40.7, and 14.5%, respectively, and the median survival was 28 months (3). According to previous reviews and case reports, renal sarcoma could be classified into the following pathological types: liposarcoma (4), leiomyosarcoma (5), carcinosarcoma (6), rhabdomyosarcoma (7), clear cell sarcoma (8), fibrosarcoma (9) and others, and different pathological types predict distinct prognosis.
RS is currently poorly studied as it is such a rare malignancy. As a result, an accurate prognostic model for RS is essential for both urologists and patients. In fact, the SEER stage grading system was employed by urologists to measure the progression of RS, which includes localized, regional, distant, and unstaged (10, 11). However, other factors including sex, age, year of diagnosis, race, marital status, radiation, chemotherapy, surgery, etc. may also have an impact on prognosis due to individual variances. In recent years, nomograms have been increasingly employed in clinical practice for cancer prognosis. It has been regarded as a useful statistical prediction tool for benefiting both clinicians and patients (12, 13). So far, there is no report on the application of nomograms in predicting the prognosis of renal sarcoma in adults. In the present study, based on data from the SEER database between 2004 and 2015, nomograms were set up to predict survival outcomes for adult patients with RS and their reliability was also validated.
Materials and methods
Data sources
Data were extracted from the Surveillance Epidemiology and End Results (SEER) database (https://seer.cancer.gov/), which is supported by the Surveillance Research Program (SRP) in NCI's Division of Cancer Control and Population Sciences (DCCPS). SEER statistics are collected on a national scale, with information from 18 states that represent all regions of the country covering 28% of the US population, including sociodemographic factors, geographic variables, clinical factors, cancer-specific factors, pathologic variables, treatment factors, and outcomes (14). The SEER database is openly accessed, and all authors have obtained permission. SEER*Stat software [Version 8.3.9.2 - August 20, 2021, SEER*Stat Software (cancer.gov)] was used to extract the data.
Patients
A total of 367 patients diagnosed with RS between 2004 and 2015 were established according to the International Classification of Disease for Oncology, Third Edition [ICD-O-3] site codes, including liposarcoma (8850/3, 8851/3, 8852/3, 8853/3, 8858/3, 8860/3), leiomyosarcoma (8890/3, 8891/3, 8896/3), carcinosarcoma (8980/3), rhabdomyosarcoma (8900/3, 8901/3, 8910/3), clear cell sarcoma (8964/3), fibrosarcoma (8810/3), sarcoma, NOS (8800/3). The exclusion criteria are based on the following principles: (1) age at diagnosis is below 18 years old, n = 62; (2) unknown marital status at diagnosis, n = 18; (3) unknown Race, n = 1; (4) unknown Survival months, n = 1; (5) not the first malignant primary tumor, n = 53. Finally, 232 eligible patients were included in the analytic cohort. The flow chart of the selection process was presented in Figure 1.
Variables and endpoints
The following variables were filtered from the SEER database: age, year of diagnosis, sex, marital status, race, grade, histological type, SEER stage, surgery, radiotherapy, chemotherapy. To facilitate the next step of data analysis, the categorical variables were coded directly, and for continuous variables, they were first converted to categorical variables before coding. Some of the variables are explained below:
1. Regarding age, patients were divided into two categories: older than 60 years and ≤60.
2. Regarding year of diagnosis, it was divided into two phases: 2004–2009, 2010–2015.
3. Regarding grade, it was defined as follows: well-differentiated (Grade I); moderately differentiated (Grade II); poorly differentiated (Grade III); undifferentiated (Grade IV); and unknown grade.
4. Regarding the stage of SEER, patients were classified into four subgroups according to the progression of the sarcoma, including localized, regional, distant, and unstaged.
The death and RS-specific death were regarded as observed endpoints. OS refers to the period between the start of the study and death from any cause, and survivors are censored as of the last follow-up. CSS refers to the period between the commencement of the study and the death due to RS, with deaths due to other causes or survivors omitted.
Statistical methods
Categorical data were described as numbers (n) and percentages (%), and chi-square tests were used to assess differences in categorical variables. The sample was divided into a training cohort and a validation cohort (in a ratio of 7:3) using a no-replacement random sampling method. The training cohort was used to create nomograms and filter factors for nomograms, while the validation cohort was used to validate the results of the training cohort. Univariate Cox regression was used to identify factors associated with OS and CSS, and multivariate Cox regression to identify associated independent risk factors. Variables with P values <0.05 in univariate Cox regression analysis were included in multivariate Cox regression analysis, and associated hazard ratios (HR) and 95% confidence intervals (CI) were calculated. Based on the results of multivariate Cox regression analysis, independent risk factors were used to create prognostic nomograms to predict the probability of OS and CSS at 3 and 5 years. In addition, receiver operating characteristic (ROC) curves, decision curve analysis (DCA), and calibration curves were used to assess the predictive performance of the nomogram and SEER stage.
A vertical line was drawn on the scale for each variable for a given adult RS patient, and the intersection with the “dot” line represented the score for that variable. The total score is calculated by adding up the scores for each variable. Matching scores were found on the “total score” line and projected onto the OS and CSS lines below, resulting in 3- and 5-OS and CSS probabilities for that individual.
In ROC curve analysis, the area under the curve (AUC) is defined as the area enclosed by the ROC curve and the coordinate axes. The value of the AUC usually ranges between 0.5 and 1, and the diagnostic value of the nomogram is represented by the AUC. In the calibration curve analysis, a bootstrap method with 1,000 resamples was used for testing.
SPSS 26.0 (IBM Corp. Released 2019. IBM SPSS Statistics for Windows, Version 26.0. Armonk, NY: IBM Corp.) was applied to conduct statistical analysis for univariate and multivariate Cox regression. The nomograms were developed and validated by exerting the rms, hmisc, lattice, survival, formula, ggplot2, pROC, timeROC, and rmda packages in R version 4.1.2 (http://www.r-project.org/). P <0.05 (two-sided) was considered statistically significant.
Results
Baseline demographic and clinical characteristics
A total of 232 eligible patients diagnosed with RS between 2004 and 2015 were included in our study, which were divided into two cohorts randomly: the training cohort (162, 70.0%) and the validation cohort (70, 30.0%). The number of RS patients aged over or equal to 60 and under 60 was similar in the total cohort. Similarly, the number of patients with the year of diagnosis in 2004–2009 and in 2010–2015 was approximately equal. Most RS patients were female (54.7%), married (57.3%), and white (81.1%). Grade IV accounts for the largest proportion of known grades. Of the other general type, the majority were leiomyosarcoma (40.9%) and localized (34.1%). Most RS adult patients received radiotherapy (85.3%) and chemotherapy (76.7%), but only a few had undergone surgery (22.4%). Specific baseline demographic and clinical characteristics information are represented in Table 1.
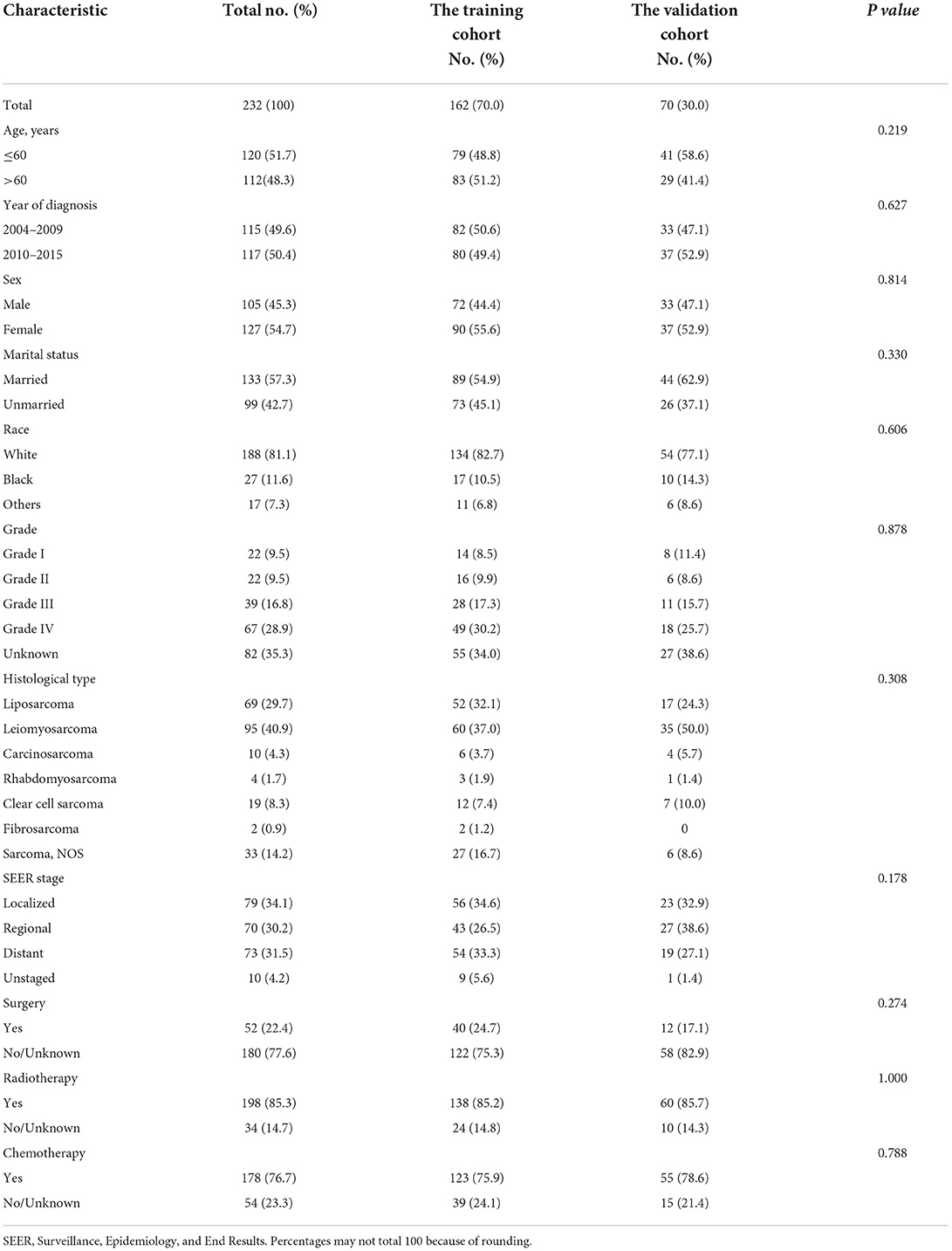
Table 1. Baseline demographic and clinical characteristics with adult renal sarcoma patients in our study.
Univariate and multivariate analysis of OS and CSS
The univariate and multivariate Cox regression analysis of OS and CSS rates in the training cohort was carried out for screening independent prognostic variables. Age, year of diagnosis, sex, marital status, race, grade, histological type, SEER stage, surgery, radiotherapy, and chemotherapy were included in our analysis. By univariate regression analysis, it was shown that all variables mentioned above might be substantially linked with OS and CSS. Meanwhile, it was also shown that sex, histological type, SEER stage, and surgery were independent predictive variables for OS by multivariate analysis, while histological type, SEER stage, surgery, and chemotherapy were independent prognostic variables for CSS. Confidence intervals (CI) and corresponding p-values for specific variables in the univariate and multivariate analyses of OS and CSS were summarized in Tables 2, 3, respectively.
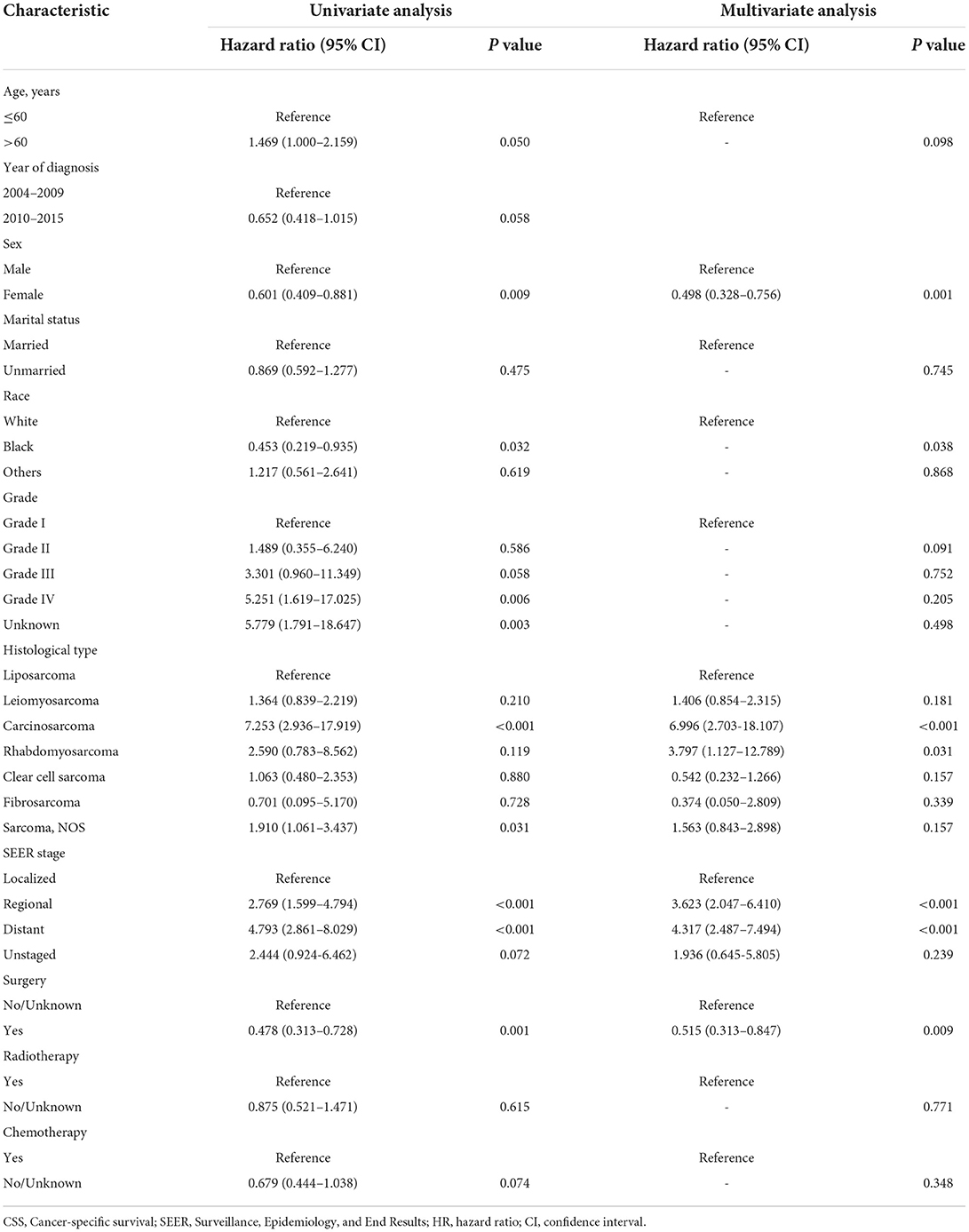
Table 2. Univariate and multivariate analysis of overall survival (OS) rates in the training cohort.
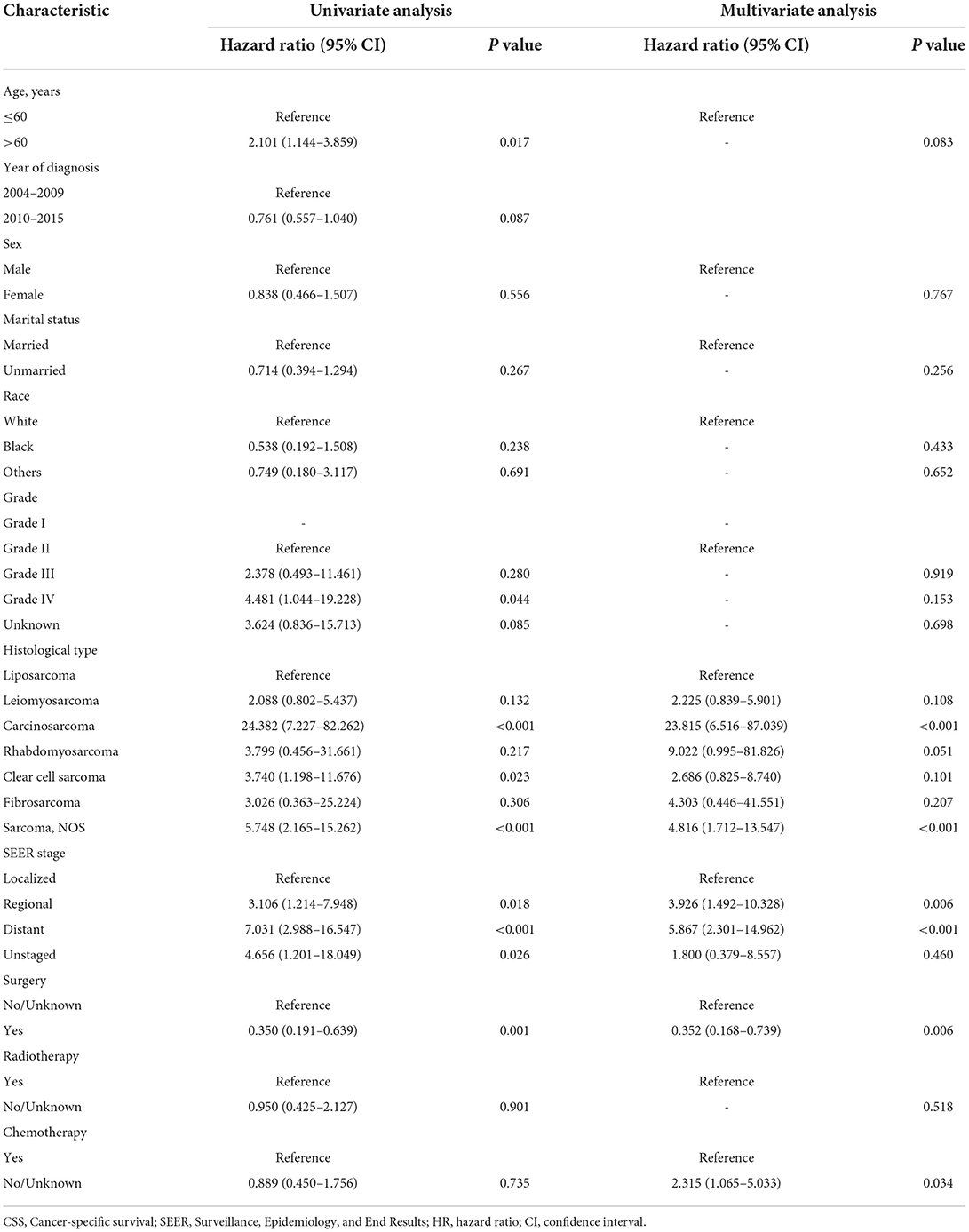
Table 3. Univariate and multivariate analysis of cancer-specific survival (CSS) rates in the training cohort.
Nomogram development and validation
According to the independent prognostic variables of OS and CSS, the nomograms were established, respectively (Figure 2). In the OS nomogram, the SEER stage contributed the most to survival outcome, while the histological type contributed the least. In the CSS nomogram, the SEER stage was the most significant predictor of survival, followed by histological type.
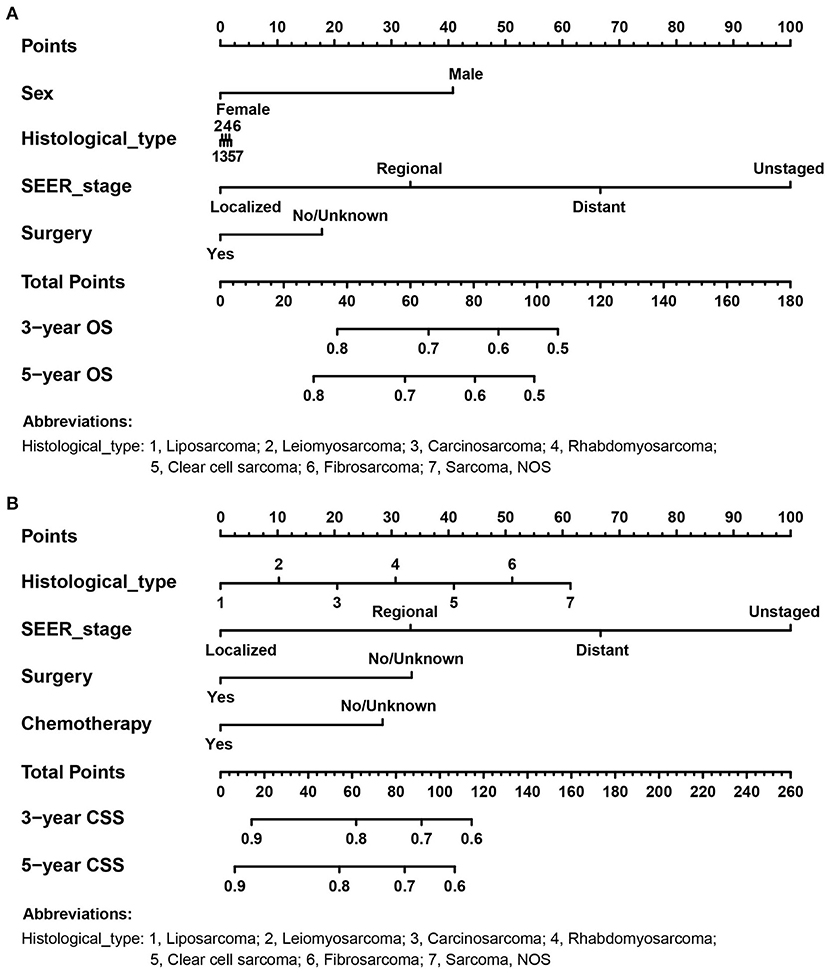
Figure 2. The prognostic nomograms for predicting 3- and 5- OS and CSS probabilities of adult RS patients in the training cohort. (A) OS nomogram; (B) CSS nomogram.
As shown in Figure 3, the ROC curves were drawn, and the AUC of the OS nomogram was significantly greater than that of the SEER stage in the training cohort (nomogram 0.742, SEER stage 0.698), while in the validation cohort the AUC of the OS nomogram was similar to SEER stage (nomogram 0.837, SEER 0.833). However, the AUCs of the nomograms for CSS were considerably higher than those of the SEER stage both in the training cohort (nomogram 0.733, SEER stage 0.656) and validation cohort (nomogram 0.758, SEER stage 0.656). By comprising of the above ROC curves, it was demonstrated that the nomogram had more diagnostic value than the SEER stage to discriminate the survival probability of adult RS patients.
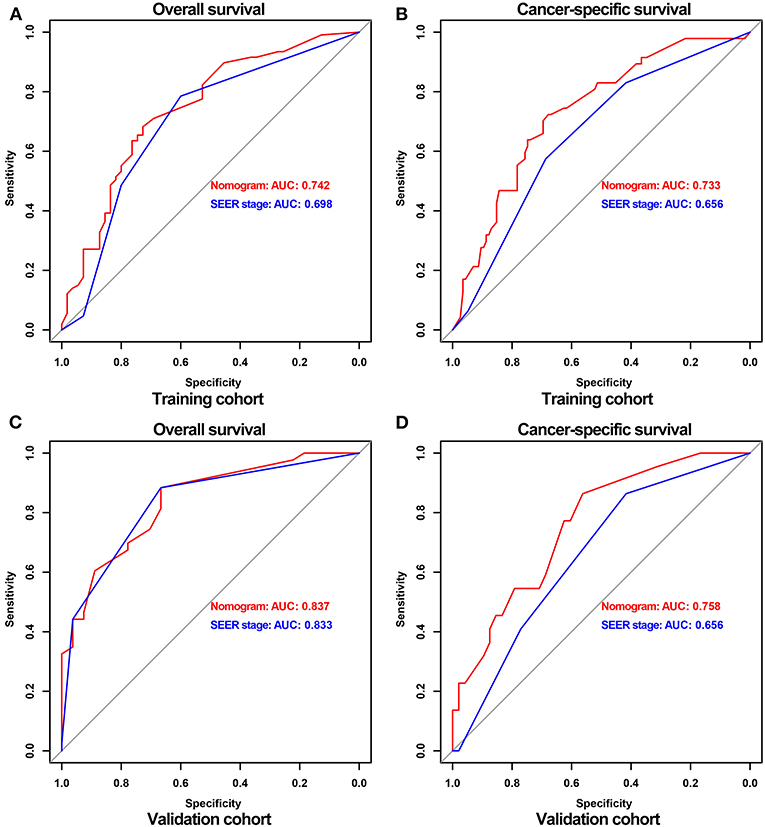
Figure 3. ROC curves of nomograms and the SEER stage for predicting OS and CSS probabilities in the training and validation cohort. ROC for OS (A) and CSS (B) in the training cohort, respectively; ROC for OS (C) and CSS (D) in the validation cohort, respectively.
The AUCs for 3- and 5-OS were 0.751 and 0.757, respectively, and 0.779 and 0.750 for 3- and 5-CSS, respectively, in the training cohort. The validation cohort AUCs for 3- and 5-OS were 0.775 and 0.829, respectively, and 0.807 and 0.855, respectively, for 3- and 5-CSS. As shown in Figure 4, the nomograms accurately predict the probability of 3- and 5- OS and CSS for adult RS patients.
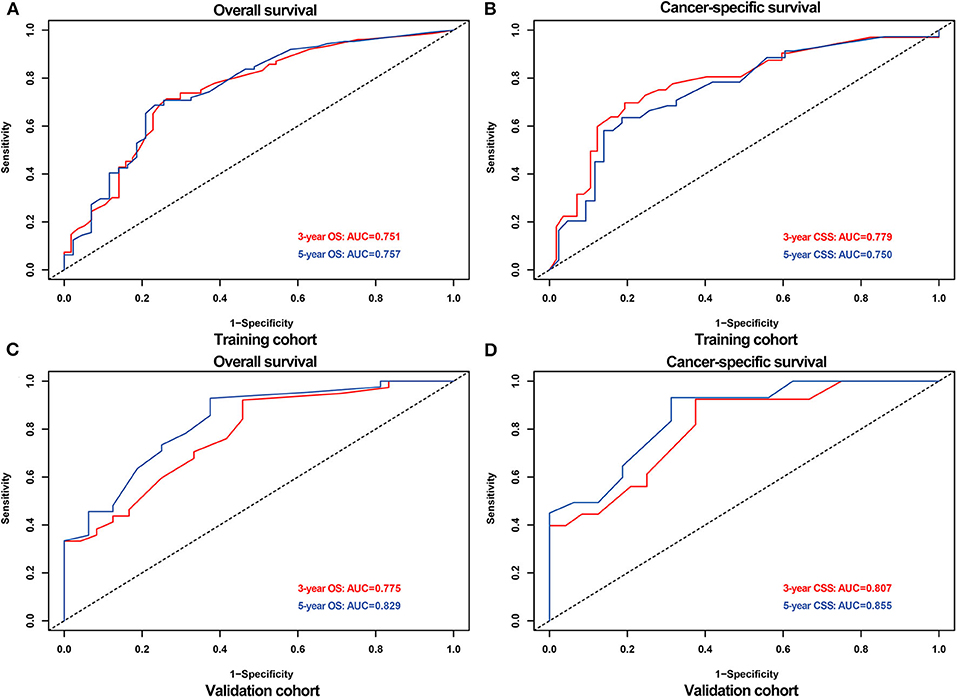
Figure 4. ROC curves for predicting 3-,5- OS and CSS probabilities in the training and validation cohort. ROC for 3-,5- OS (A) and CSS (B) in the training cohort; ROC for 3-,5- OS (C) and CSS (D) in the validation cohort.
The calibration curves of the nomograms showed high consistencies between the predicted and actual survival rates both in the training and validation cohorts, illustrated in Figure 5 and Supplementary Figure 1. The gray line in the calibration curves represents the ideal reference line, where the predicted survival probability matches the actual survival probability. The presentation of the nomograms was represented by red dots. The DCA demonstrated that the nomograms in the wide high-risk threshold had a higher net benefit than the SEER stage (Figure 6), which validated the superiority of the nomogram utility over the SEER stage in clinical practice.
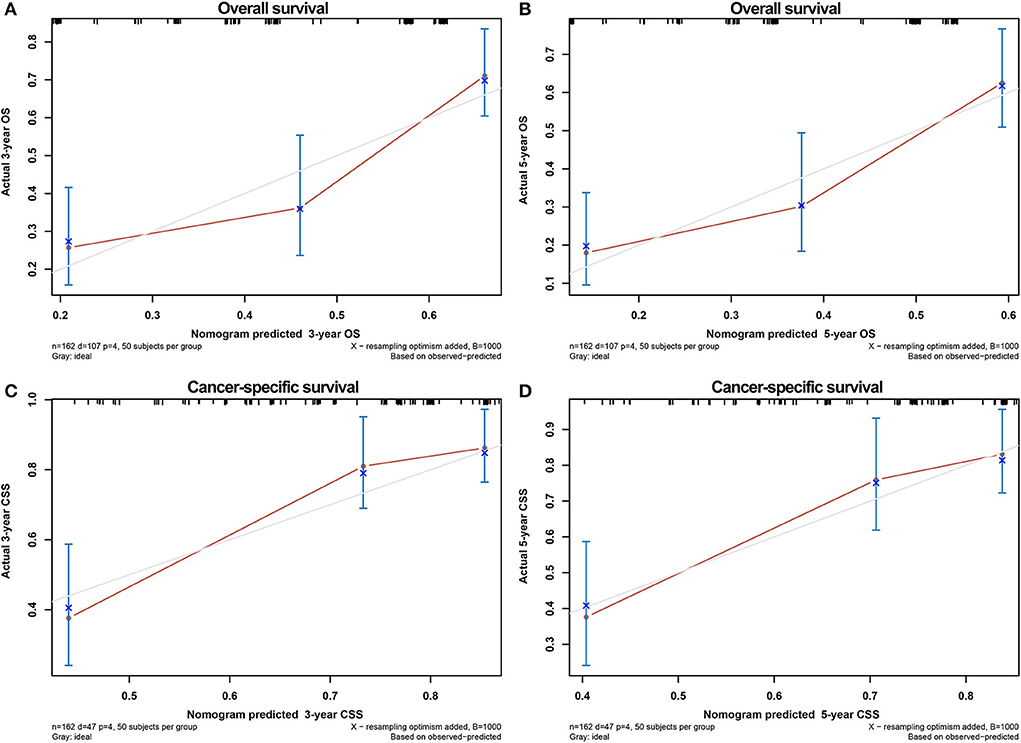
Figure 5. Calibration curves for verifying the consistency between predicted 3-,5- OS and CSS and actual 3-,5- OS and CSS in the training cohort. 3- OS (A) and 5- OS (B) calibration curves; 3- CSS (C) and 5- CSS (D) calibration curves.
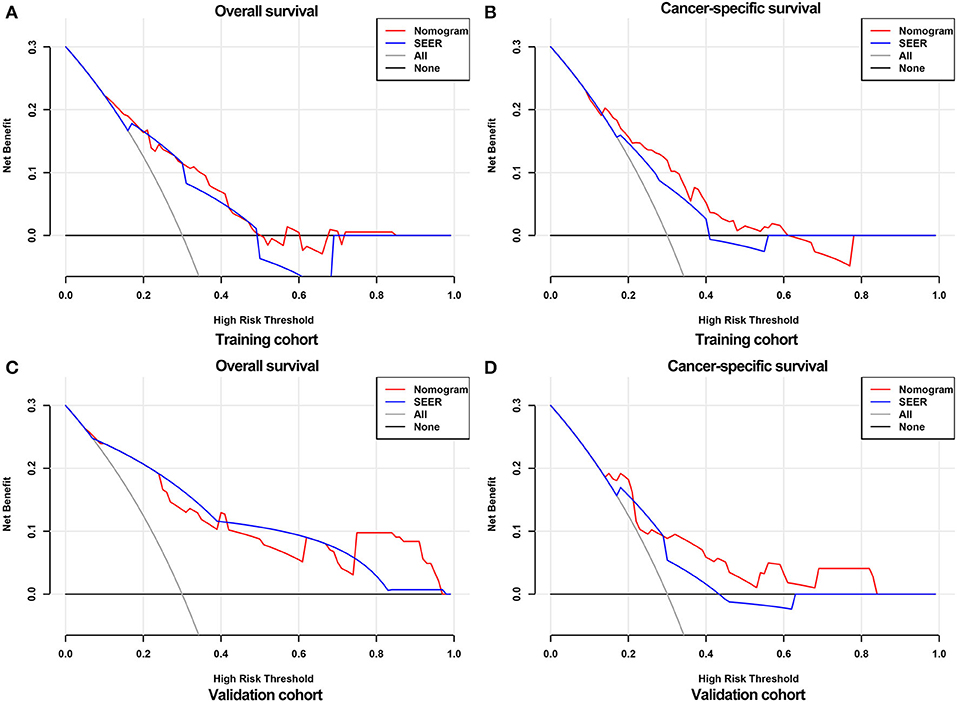
Figure 6. DCA curves for validating the clinical utility of the nomograms. DCA curves for OS (A) and CSS (B) in the training cohort. DCA curves for OS (C) and CSS (D) in the validation cohort.
Discussion
As mentioned above, adult renal sarcomas are an extremely rare group of tumors, accounting for only 0.8% of primary renal tumors (3). The SEER stage grading system was used by urologists to evaluate the progression of renal sarcomas. Sarcomas are classified into different grades based on the location and the extent to which it invades organs, blood vessels, and lymph nodes, including localized, regional, distant, and unstaged. However, due to the influence of individual differences, such as sex, age, race, marital status, radiation, chemotherapy, surgery, etc., it is not comprehensive enough to use the extent of tumor invasion alone to evaluate the prognosis for adult RS patients.
The nomogram is a graphical representation of a clinical prediction model that calculates a total score based on the values of individual predictor variables, and then predicts the risk of an event or the probability of survival based on the total score (15, 16). It is a novel prediction model that is gradually sought after by clinicians. In recent years, predictions for the prognosis of various urinary cancer with nomograms have been reported more and more. For instance, Wu et al. employed a genomic-clinicopathologic nomogram to predict preoperative lymph node metastasis in bladder cancer (17); A nomogram was conducted by Mao et al. to predict prognosis in patients with lung metastatic renal cell carcinoma (18). Zhang et al. established a radiomics nomogram to predict bone metastasis in newly diagnosed prostate cancer patients (19). The nomogram and Aggtrmmns scoring system were utilized by Zhou et al. for predicting overall survival and cancer-specific survival of kidney cancer patients (20).
As it is known that compared with the SEER stage, nomogram has the following advantages: (1) By combining various independent risk factors according to the patient's condition, it allows for a more intuitive assessment and individualization of the patient's prognosis (21). (2) It quantifies the possibility of OS and CSS in patients, permitting a more precise prognostic evaluation (22). Therefore, for the first time, the prognostic nomograms were developed for adult RS patients to obtain personalized and accurate prognostic predictions in this study.
We extracted data from the SEER database for adult RS patients and used COX univariate and subsequent multivariate regression analysis to conclude that histological type, SEER stage, surgery were independent risk factors for OS and CSS. Based on the multivariate regression analysis, the OS and CSS nomograms were constructed, respectively. Subsequently, we validated the nomograms. The area under the ROC curves for 3-,5- OS were 0.775 and 0.829, respectively, and 0.807 and 0.855 for 3-, 5- CSS, respectively, which depicted that the nomograms accurately predict the probability of 3- and 5- OS and CSS for adult RS patients. The calibration curves showed high consistencies between the predicted and actual survival rates.
From the nomograms, it was suggested that RS patients without surgery, with distant SEER stage grade, and histological type of carcinosarcoma had the poorest prognosis. According to the Kaplan-Meier overall and disease-specific survival analysis of patients with RS established by Nazemi et al. (1), liposarcoma had the greatest prognosis, followed by leiomyosarcoma and clear cell sarcoma, while carcinosarcoma had the worst prognosis. Some studies have shown that carcinosarcoma had the worst prognosis, which was consistent with our analysis. In addition, in our study, univariate Cox regression analysis found that Sarcoma, NOC patients had poorer OS compared to liposarcoma patients (HR = 1.910, 95% CI 1.061–3.437, p = 0.031). However, after multivariate Cox regression analysis, there was no difference between the two groups, which could be explained by the inclusion of other confounding variables, which led to biased results.
In addition, our study demonstrated that surgical treatment for adult RS patients may effectively reduce the risk of death. This is in line with the findings of Moreira et al. (14) and Öztürk (23). Moreover, it is also found that chemotherapy may also improve the prognosis of adult RS patients. Chemotherapy has now been applied clinically to treat advanced or recurrent renal sarcoma, although not standardized (24), and the latest research of Yakirevich et al. suggested that comprehensive genomic analysis of adult RS patients may provide new opportunities for targeted therapy (25).
To our surprise, our data suggested radiotherapy was not an independent prognostic factor for the adult patient with renal sarcoma, which was in accordance with the findings of Li et al. (26). However, Gamboa et al. reported that preoperative radiotherapy may improve the prognosis by making some tumors easier to resect (27). Thus, the prognostic impact of radiotherapy on patients with renal sarcomas should be further explored. The clinical outcome of primary adult renal sarcoma is extremely poor and the optimal treatment remains to be debated. Further studies are needed to verify whether it is surgery or combination therapy that works best. Furthermore, our data also suggested that female patients had a better prognosis than male patients, which could be attributed to differences in female anatomy or hormone levels.
We appraised the prognosis of adult RS patients with nomograms for the first time, which adds a new dimension to our research. Simultaneously, using the SEER database excluded the influencing factors of single-center. Even so, there are still a few flaws in our study: (1) Because of the rarity of renal sarcoma, limited sample size is inevitable and therefore our findings may not be representative; (2) As our study is retrospective, there is a lack of multicenter data for external validation. (3) Due to the lack of data in the SEER database, genetic factors, laboratory findings, and medication history were not included in our study.
Conclusions
In conclusion, a prognostic nomogram was created to predict overall survival (OS) and cancer-specific survival (CSS) for adult patients with RS, and their reliability and usefulness were also validated in our study. We anticipate that our study will facilitate urologists in accurately assessing the prognosis of adult RS patients and provide support for further clinical trials.
Data availability statement
The datasets presented in this study can be found in online repositories. The names of the repository/repositories and accession number(s) can be found in the article/Supplementary material.
Author contributions
YZ, MC, JW, YM, and QW: study designing. MC, JW, YM, and QW: administrative support. YZ, WM, GZ, SS, ST, and TJ: data collection. YZ, WM, GZ, and SS: statistical analysis and graphs production. YZ, WM, and GZ: draft writing. YZ, WM, GZ, SS, ST, TJ, QW, YM, JW, and MC: final revision. All authors contributed to the article and approved the submitted version.
Funding
This study was funded by Natural Science Foundation of China (82170703 to GZ and 82100732), Natural Science Foundation of Jiangsu Province (BK20200360), and Excellent Youth Development Fund of Zhongda Hospital, SEU (2021ZDYYYQPY04).
Conflict of interest
The authors declare that the research was conducted in the absence of any commercial or financial relationships that could be construed as a potential conflict of interest.
Publisher's note
All claims expressed in this article are solely those of the authors and do not necessarily represent those of their affiliated organizations, or those of the publisher, the editors and the reviewers. Any product that may be evaluated in this article, or claim that may be made by its manufacturer, is not guaranteed or endorsed by the publisher.
Supplementary material
The Supplementary Material for this article can be found online at: https://www.frontiersin.org/articles/10.3389/fpubh.2022.942608/full#supplementary-material
Supplementary Figure 1. Calibration curves for verifying the consistency between predicted 3-,5- OS and CSS and actual 3-,5- OS and CSS in the validation cohort. 3- OS (A) and 5- OS (B) calibration curves; 3- CSS (C) and 5- CSS (D) calibration curves.
Abbreviations
RS, Renal sarcoma; SEER, Surveillance, Epidemiology, and End Results; OS, Overall survival; CSS, Cancer-specific survival; DCA, Decision curve analysis; ICD-O, The International Classification of Diseases for Oncology; ROC, Receiver operating characteristic; AUC, Area under the curve; HR, Hazard ratios; CI, Confidence intervals.
References
1. Nazemi A, Daneshmand S. Adult genitourinary sarcoma: a population-based analysis of clinical characteristics and survival. Urol Oncol. (2020) 38:334–43. doi: 10.1016/j.urolonc.2019.12.004
2. Vogelzang NJ, Fremgen AM, Guinan PD, Chmiel JS, Sylvester JL, Sener SF. Primary renal sarcoma in adults. A natural history and management study by the American Cancer Society, Illinois Division. Cancer. (1993) 71:804–10. doi: 10.1002/1097-0142(19930201)71:3<804::aid-cncr2820710324>3.0.co
3. Wang X, Xu R, Yan L, Zhuang J, Wei B, Kang D, et al. Adult renal sarcoma: clinical features and survival in a series of patients treated at a high-volume institution. Urology. (2011) 77:836–41. doi: 10.1016/j.urology.2010.09.028
4. Mayes DC, Fechner RE, Gillenwater JY. Renal liposarcoma. Am J Surg Pathol. (1990) 14:268–73. doi: 10.1097/00000478-199003000-00008
5. Ding Y, Quan C. Primary renal leiomyosarcoma. J Coll Physicians Surg Pak. (2021) 30:725–7. doi: 10.29271/jcpsp.2021.06.725
6. Chiu KC, Lin MC, Liang YC, Chen CY. Renal carcinosarcoma: case report and review of literature. Ren Fail. (2008) 30:1034–9. doi: 10.1080/08860220802403192
7. Sola JE, Cova D, Casillas J, Alvarez OA, Qualman S, Rodriguez MM. Primary renal botryoid rhabdomyosarcoma: diagnosis and outcome. J Pediatr Surg. (2007) 42:e17–20. doi: 10.1016/j.jpedsurg.2007.08.011
8. Aw SJ, Chang KTE. Clear cell sarcoma of the kidney. Arch Pathol Lab Med. (2019) 143:1022–6. doi: 10.5858/arpa.2018-0045-RS
9. Agarwal K, Singh S, Pathania OP. Primary renal fibrosarcoma: a rare case report and review of literature. Indian J Pathol Microbiol. (2008) 51:409–10. doi: 10.4103/0377-4929.42541
10. Chen C, Jiang X, Xia F, Chen X, Wang W. Clinicopathological Characteristics and Survival Outcomes of Primary Renal Leiomyosarcoma. Front Surg. (2021) 8:704221. doi: 10.3389/fsurg.2021.704221
11. Zhuge Y, Cheung MC, Yang R, Perez EA, Koniaris LG, Sola JE. Pediatric non-Wilms renal tumors: subtypes, survival, and prognostic indicators. J Surg Res. (2010) 163:257–63. doi: 10.1016/j.jss.2010.03.061
12. Chen JY, Chen JJ, Yang BL, Liu ZB, Huang XY, Liu GY, et al. Predicting sentinel lymph node metastasis in a Chinese breast cancer population: assessment of an existing nomogram and a new predictive nomogram. Breast Cancer Res Treat. (2012) 135:839–48. doi: 10.1007/s10549-012-2219-x
13. Lee CK, Asher R, Friedlander M, Gebski V, Gonzalez-Martin A, Lortholary A, et al. Development and validation of a prognostic nomogram for overall survival in patients with platinum-resistant ovarian cancer treated with chemotherapy. Eur J Cancer. (2019) 117:99–106. doi: 10.1016/j.ejca.2019.05.029
14. Moreira DM, Gershman B, Thompson RH, Okuno SH, Robinson SI, Leibovich BC, et al. Clinicopathologic characteristics and survival for adult renal sarcoma: a population-based study. Urol Oncol. (2015) 33:505 e15–20. doi: 10.1016/j.urolonc.2015.07.022
15. Iasonos A, Schrag D, Raj GV, Panageas KS. How to build and interpret a nomogram for cancer prognosis. J Clin Oncol. (2008) 26:1364–70. doi: 10.1200/JCO.2007.12.9791
16. Mao W, Wang K, Xu B, Zhang H, Sun S, Hu Q, et al. ciRS-7 is a prognostic biomarker and potential gene therapy target for renal cell carcinoma. Mol Cancer. (2021) 20:142. doi: 10.1186/s12943-021-01443-2
17. Wu SX, Huang J, Liu ZW, Chen HG, Guo P, Cai QQ, et al. A genomic-clinicopathologic nomogram for the preoperative prediction of lymph node metastasis in bladder cancer. Ebiomedicine. (2018) 31:54–65. doi: 10.1016/j.ebiom.2018.03.034
18. Mao W, Fu Z, Wang K, Wu J, Xu B, Chen M. Prognostic nomogram for patients with lung metastatic renal cell carcinoma: a SEER-based study. Ann Palliat Med. (2021) 10:2791–804. doi: 10.21037/apm-20-1488
19. Zhang W, Mao N, Wang Y, Xie H, Duan S, Zhang X, et al. A Radiomics nomogram for predicting bone metastasis in newly diagnosed prostate cancer patients. Eur J Radiol. (2020) 128:109020. doi: 10.1016/j.ejrad.2020.109020
20. Zhou Y, Zhang R, Ding Y, Wang Z, Yang C, Tao S, et al. Prognostic nomograms and Aggtrmmns scoring system for predicting overall survival and cancer-specific survival of patients with kidney cancer. Cancer Med. (2020) 9:2710–22. doi: 10.1002/cam4.2916
21. Hou G, Zheng Y, Wei D, Li X, Wang F, Tian J, et al. Development and validation of a SEER-based prognostic nomogram for patients with bone metastatic prostate cancer. Medicine (Baltimore). (2019) 98:e17197. doi: 10.1097/MD.0000000000017197
22. Wang K, Xu X, Xiao R, Du D, Wang L, Zhang H, et al. Development and validation of a nomogram to predict cancer-specific survival in patients with hypopharyngeal squamous cell carcinoma treated with primary surgery. J Int Med Res. (2021) 49:3000605211067414. doi: 10.1177/03000605211067414
23. Ozturk H. Prognostic features of renal sarcomas (Review). Oncol Lett. (2015) 9:1034–8. doi: 10.3892/ol.2014.2838
24. Blas L, Roberti J. Primary renal synovial sarcoma and clinical and pathological findings: a systematic review. Curr Urol Rep. (2021) 22:25. doi: 10.1007/s11934-021-01038-w
25. Yakirevich E, Madison R, Fridman E, Mangray S, Carneiro BA, Lu S, et al. Comprehensive genomic profiling of adult renal sarcomas provides insight into disease biology and opportunities for targeted therapies. Eur Urol Oncol. (2021) 4:282–8. doi: 10.1016/j.euo.2019.04.002
26. Li L, Liang J, Song T, Yin S, Zeng J, Zhong Q, et al. A nomogram model to predict prognosis of patients with genitourinary sarcoma. Front Oncol. (2021) 11:656325. doi: 10.3389/fonc.2021.656325
Keywords: adult patients, renal sarcoma, nomogram, SEER, prognosis
Citation: Zhu Y, Mao W, Zhang G, Sun S, Tao S, Jiang T, Wang Q, Meng Y, Wu J and Chen M (2022) Development and validation of a prognostic nomogram for adult patients with renal sarcoma: A retrospective study based on the SEER database. Front. Public Health 10:942608. doi: 10.3389/fpubh.2022.942608
Received: 12 May 2022; Accepted: 22 August 2022;
Published: 12 September 2022.
Edited by:
Yi-Ju Tseng, National Yang Ming Chiao Tung University, TaiwanReviewed by:
Yuliuming Wang, The Second Affiliated Hospital of Harbin Medical University, ChinaMarla Weetall, PTC Therapeutics, United States
Shigao Huang, Air Force Medical University, China
Shiva Basnet, Tongji University, China
Copyright © 2022 Zhu, Mao, Zhang, Sun, Tao, Jiang, Wang, Meng, Wu and Chen. This is an open-access article distributed under the terms of the Creative Commons Attribution License (CC BY). The use, distribution or reproduction in other forums is permitted, provided the original author(s) and the copyright owner(s) are credited and that the original publication in this journal is cited, in accordance with accepted academic practice. No use, distribution or reproduction is permitted which does not comply with these terms.
*Correspondence: Ming Chen, mingchenseu@126.com; Jianping Wu, doctorwujianping@126.com; Yuan Meng, lishumy@live.cn
†These authors have contributed equally to this work