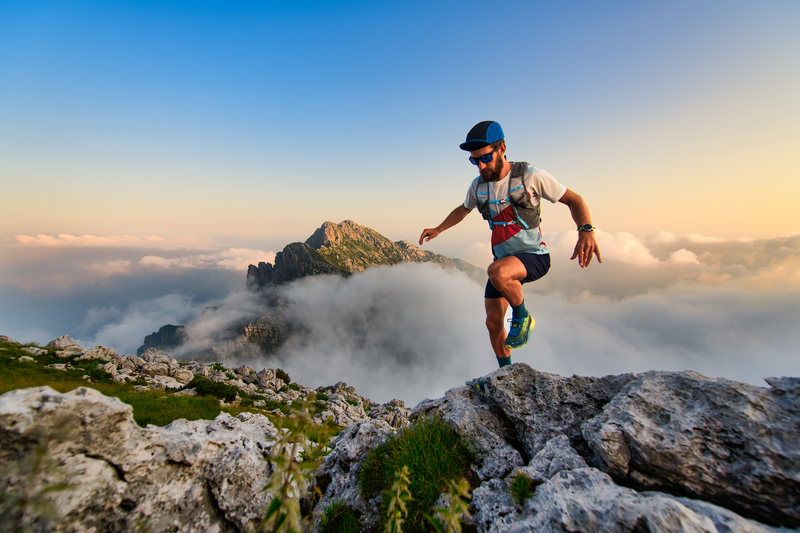
94% of researchers rate our articles as excellent or good
Learn more about the work of our research integrity team to safeguard the quality of each article we publish.
Find out more
ORIGINAL RESEARCH article
Front. Public Health , 05 August 2022
Sec. Infectious Diseases – Surveillance, Prevention and Treatment
Volume 10 - 2022 | https://doi.org/10.3389/fpubh.2022.940905
This article is part of the Research Topic Diagnosis, Treatment and Prognosis of Viral Hepatitis, Volume II View all 20 articles
Background: The main objective of this study is to explore the effects of hepatitis C (HCV) on the prevalence rate of kidney stones in US women.
Method: Dates for HCV infection and kidney stones were collected from National Health and Nutrition Examination Survey (NHANES) database, a cross-sectional study. The analysis samples included adults aged ≥20 years and women from six consecutive cycles of the NHANES 2007–2018. The association between HCV infection and kidney stones was performed by using logistic regression models. Subgroup analyses were conducted to find sensitive crowds.
Results: A total of 13,262 participants were enrolled, including 201 infected with HCV. After adjustment for potential confounders, we revealed a positive relationship between HCV and kidney stones (OR = 1.70, 95%CI:1.13–2.56). The crowds' statistically significant difference was characterized by other races (OR = 8.17, 95%CI:1.62–41.22) and BMI within 25–29.9 kg/m2 (OR = 2.45, 95%CI:1.24–4.83).
Conclusions: HCV infection may affect the prevalence of urolithiasis in US women, even the causal relationship remains unclear, the relation deserves special attention. We considered such a study an ideal way to begin exploring the effects of HCV on kidney stones.
When it comes to the urinary system, nephrolithiasis (NL), also known as kidney stones, is a prevalent problem in the study field, with high rates of occurrence and recurrence (1–3). The morbidity of patients with nephrolithiasis is increasing as a result of global warming, including a fast-paced lifestyle and an unhealthy or irregular diet, among other factors (4). Kidney stones have become increasingly widespread all around the globe over the years, regardless of gender, race, or age of the patient. Note that the rate of kidney stone production varies significantly across different geographical locations and nations. The prevalence of kidney stones in the United States (5) was more than 10%, according to a recent multi-country survey study, although the rates were 9 percent and 5.8 percent in Europe (6) and China (7), respectively, according to the same study. We also found that gender plays a very important role in the epidemiology of renal calculi. According to a 2020 NHANES-based survey study (5), it was found that the prevalence of kidney stones increased significantly in women during 2008–2018, while it did not increase significantly in men. In another retrospective study that surveyed 500,000 Navy personnel, it was found that the prevalence of kidney stones was way higher in women than in men (8). To treat it, minimally invasive endoscopic procedures, such as percutaneous nephrolithotomy, flexible ureteroscopy lithotripsy, and other endoscopic procedures, are routinely performed. Although therapy has been completed, patients still have a substantial risk of recurrence of their condition (9). If it is not treated effectively, it may progress to serious consequences, such as irreversible kidney damage and end-stage renal disease. Nephrolithiasis has grown in importance as a public health concern, as well as a significant financial burden on medical healthcare systems (10).
Hepatitis C virus (HCV) is a single-stranded RNA virus with an envelope that belongs to the Flaviviridae viral family (11). It is responsible for the transmission of the disease. HCV infection is a serious public health hazard in many parts of the globe, including the United States. Worldwide, around 130–150 million individuals are estimated to be chronically infected with HCV (12), according to the WHO. HCV infection is diagnosed with the use of anti-HCV antibodies and HCV RNA (13). It is one of the most significant contributors to liver fibrosis, organ failure, and even liver cancer (14). Not only that but there is also a gender gap in HCV infection rates. HCV surveillance data typically show lower HCV detection rates in women than in men (15). However, a recent survey of HCV prevalence among drug users found a higher prevalence among women drug users (15). A Pakistani study also found significantly higher rates of HCV infection in women than in men (16). Recent investigations have shown that HCV may infect macrophage cells, which may explain the extrahepatic symptoms. Chronic HCV infection has the potential to harm other organs, including the lung (17), heart (18), and kidney. Almost all of the research conducted so far has focused only on the impact of HCV on renal function (19). The most typically documented kidney ailment linked with HCV is HCV-associated glomerular disease, which is characterized by the presence of mixed cryoglobulinemia. Although glomerular damage is often believed to be the cause of kidney disease, tubular injury has also been identified as a contributing factor. It has been found that individuals with HCV infection suffer from both glomerular and tubule damage, respectively (20). One of the most serious risks associated with kidney stone prevalence is tubular epithelial cell injury (2). Is there a correlation between the increased prevalence of kidney stones and HCV infection in women?
As a consequence, we undertook a large population-based cross-sectional investigation using the National Health and Nutrition Examination Survey database to determine if there is a link between HCV and the occurrence of kidney stones in the general population. Subgroup studies were conducted at the same time to identify the most vulnerable population. All of the studies aimed to provide some kind of proof for the prevention of renal illness on some level.
Data for this study were obtained from the NHANES database based on big data mining methods and were conducted by the Centers for Disease Control and Prevention (CDC) (21, 22). NHANES is a nationally representative, cross-sectional survey meant to produce nationally representative estimates of the population's health and nutritional status. It is utilized for cross-sectional investigations. From 2007 through 2018, survey data was used six times in a row. The NHANES website (www.cdc.gov/nchs/nhanes/) has further information on the data.
In this research, all of the subjects were between the ages of 20 and 80. The current research has a total of 59,841 participants. The following were the criteria for exclusion: (1) unknown kidney stone (n = 25,163); (2) undetermined HCV infection status (n = 8,869); and (3) men (n = 12,547) (Figure 1). A total of 13,262 participants were included in the final analysis. The Institutional Review Committee of the National Center for Health Statistics(NCHS) gave its approval to the procedure.
Figure 1. Flowchart of the sample selection from National Health and Nutrition Examination Survey (NHANES) 2007–2018.
To identify kidney stones, the results from the KIQ026 (Do you have kidney stones?) questionnaire were used. To confirm the diagnosis of HCV infection, laboratory tests were completed. The methodologies for detecting HCV-Ab and HCV-RNA were developed from the laboratory data column's “Description of Laboratory Methodology.” HCV-Ab(+)or HCV-RNA(+) were used to define positive HCV infection. Age, race, education level, marital status, PIR (Ratio of Family Income to Poverty), physical activity, total water consumption, high blood pressure, diabetes, and alcohol users were all included in the questionnaires. The laboratory test yielded the BMI.
Regarding the selection of weights, the principle of the official guidelines provided by NHANES is to first specify the variable that examines the smallest population and then proceed with selecting the weights corresponding to that variable. In this study, our data included MEC examination data, and according to the recommendations of the weight selection guidelines, we selected the sub-weights corresponding to MEC. According to the NHANES analysis guidelines, the new sampling weights for the combined survey cycles were constructed by dividing the 2-year weights for each cycle by six (23).
Categorical, dichotomous, and continuous variables were used to record the data. Continuous variables were expressed as mean standard deviation (SD), and dichotomous and categorical variables were expressed as counts proportions. Weighted Chi-square tests (categorical variables) and weighted one-way analysis of variance (ANOVA) (normal distribution continuous variables) or weighted Kruskal-H Wallis's test (skewed distribution continuous variables) were used to evaluate differences in clinical characteristics between groups. When <10% of the data were missing, the missing item value was replaced by means. For variables with an additional category, missing indications were produced. Because HCV-Ab data was unavailable from 2015–2016, the infection status gap was filled by the total HCV infection prevalence from 2007 to 2018.
We employed machine learning to predict the effect of each research variable on kidney stone prevalence to assess the risk of the factors linked to kidney stone prevalence (Figure 3). To investigate the independent connection, three logical regression models were created in our study: (1) unadjusted; (2) adjusted for age and race; and (3) adjusted for all factors. In addition, we used stratified multivariate logistic regression to conduct subgroup analyses to find acceptable groups. Statistical analyses were carried out using R 3.5.3 (http://www.r-project.org/) and Empower Stats software (http://www.empowerstats.com), with a P-value of 0.05 being considered statistically significant.
A total of 13,262 people were included in our study, and the necessary variables are listed in Table 1. In general, the morbidity rate associated with kidney stone development has been increasing (P for trend<0.001). However, this tendency did not hold for HCV infection prevalence (P for trend >0.05). In comparison to the nonstone former group, the stone former group had a greater prevalence of HCV infection (p < 0.0001). The results are depicted in Figure 2, as well as in Supplementary Tables 1, 2. Additionally, we applied machine learning (24) to predict the effect of each research variable on the production of kidney stones. The result indicates that HCV infection is one of the several factors influencing the production of calculi (Figure 3).
The major objective of this study was to establish a causal relationship between HCV infection status and the prevalence of kidney stone development. We conducted multivariate logistic regression analysis. Three models were developed in accordance with the guidelines of the Strengthening the Reporting of Observational Studies in Epidemiology (STROBE) statement (25). In an unadjusted model, kidney stone development was associated with HCV infection in US women (OR = 1.96, 95%CI:1.31–2.92). Following that, after adjusting for age and race, we developed a second model, the pattern was similar to the first. Surprisingly, the results were substantially equal before and after adjustment for all variables (OR = 1.70, 95%CI:1.13–2.56). Subgroup analysis revealed that this negative connection trend was more evident in subgroups that comprised other races (OR=8.17, 95% CI:1.62–41.22) and with BMI between 25 and 29.9 kg/m2 (OR = 2.45, 95% CI:1.24–4.83) (Table 2).
Nephrolithiasis is characterized by a high prevalence, a high recurrence rate, and a varied prognosis (4, 26). Procrastinating the start of treatment can result in serious impairment of renal function. Earlier research suggested that kidney stones are a complicated chronic systemic condition (27). At the moment, the treatment regimen for renal calculi includes surgery, rehabilitation, and medication. Rapid advancements in science and technology aided in the evolution of the surgical approach from open surgery to a variety of less invasive endoscopic procedures. Despite this, current treatment for kidney stones focuses mostly on clinical symptoms, with no commonly available etiological medication. The basic cause of kidney stone production and recurrence remains unknown (28). As a result, understanding important risk factors for kidney stones is of critical clinical importance.
Our investigation indicated that the prevalence of kidney stones was increasing every 2 years, and the findings in the literature corroborated our findings (3, 5). However, because the morbidity of HCV-Ab did not follow the epidemiology of nephrolithiasis from 2007 to 2018, we believe that it may eventually come down to effective prevention and treatment measures (29). Although HCV infection is curable, due to the large number of people who are ignorant of their illness, HCV infection continues to be a significant public health problem (30).
The purpose of this initiative was to investigate the association between HCV infection and renal calculi. The NHANES database was mined and analyzed for massive, organized, population-based cross-sectional data. We discovered that the number of HCV-infected people in the kidney stone group was significantly higher than the number of HCV-infected subjects in the non-stone group. The most important finding of our current investigation is the identification of HCV virus status as a risk factor for kidney stone prevalence using multivariate logistic models. This connection persisted after correcting for all the confounding variables. According to the link between HCV(+) and nephrolithiasis, the most significant crowds were those with BMI of 25–29.9 kg/m2 and other races. Overweight (BMI 25–29.9 kg/m2) and obesity (BMI ≥ 30 kg/m2) are risk factors for the development of kidney stones, and Parvin (31) found a higher probability of kidney stones in the overweight and obese population in a study in 2021. The results of a cohort study on BMI and prevalence of kidney stones by Korean scholar Kim (32) in 2019 showed that in the metabolically healthy population, overweight and obesity have OR = 1.12, 95%: 13–1.22 and OR = 1.72, 95%:1.21–2.44, while in the metabolically unhealthy population, overweight and obesity have OR = 1.27, 95%. 1.20–1.34 and OR = 1.36, 95%:1.22–1.51. The results of this paper showed that in the female population with BMI of 25–29.9 kg/m2, OR = 2.45, 95%CI:1.24–4.83, suggesting that HCV infection is positively associated with the prevalence of kidney stones, and in the female population with BMI > 30 kg/m2, OR = 1.24, 95%CI:0.64–2.40, suggesting that HCV infection was positively associated with the prevalence of kidney stones, but not significantly, which may be related to the small sample size and needs to be verified in a large sample multicenter ready control trial.
Simultaneously, machine learning was utilized to determine whether certain variables have an effect on the occurrence of kidney stones in this study. Data may be ranked using machine learning according to their influence. The most relevant factors in the model of kidney stone development were the albumin creatinine ratio, BMI, serum creatinine, age, non-Hispanic white, high blood pressure, HCV infection status, and so on. Some research have been carried out to compare the advantages and disadvantages of machine learning approaches to traditional regression techniques. As of yet, however, the findings have been considerably disparate. Studies have revealed that logistic regression may be as accurate as, or, perhaps, more accurate than other machine learning algorithms in certain situations (20). Other research, on the other hand, have shown that machine learning approaches are more trustworthy than traditional regression analysis (24). Consequently, according to Wolpert's “No Free Lunch Theorem,” no single strategy will be the most accurate in every situation, thus, comparisons of strategies across various study topics and datasets may provide different findings. Both machine learning and logistic regression models in this study indicated HCV infection as a risk factor for kidney stone development, which is a strong start in this area of research.
Our research shows for the first time that HCV infection may have an effect on kidney stone development, utilizing a cross-sectional study. As a result, there has been no research on its putative mechanism. We hypothesize the following causes based on past research on its correlation: It is widely established that renal tubular epithelial cell injury is a critical stage in the processing of kidney stones prevalence. Although chronic kidney disease (CKD) is now recognized as an extrahepatic manifestation of hepatitis C virus, it is often advanced. Researchers are reporting that tubulointerstitial damage is the early stage of renal manifestation rather than glomerular damage (20), which is positive. An Italian study of 98 cirrhotic individuals found tubular involvement to be the most prevalent kidney abnormality (33). The HCV core protein has also been shown to be more prevalent in the renal tubules of patients with HCV infection (34). In the proximal renal tubular epithelial cells, activation of caspases 3, 8, and 9 by HCV has been found to impact tubular barrier function directly in renal epithelial cells, favoring apoptotic cascades (35). As a result of the damage to the renal tubular cells, crystals are readily formed on them. Crystals adhering to the surface of renal tubular cells are taken up by the cells. In due course, a stone is created from the crystals and crystal aggregates that have grown in the preceding step.
The following are some of our study's limitations: (a) Because this was a cross-sectional research, it cannot prove a causal link between HCV infection and the likelihood of kidney stone development; (b) It should be noted that the NHANES only included “self-reported” data on kidney stone history, which excluded asymptomatic stones. Another drawback is that we were unable to determine the sort of renal calculi that were present; and (c) Eating habits may have an impact on kidney stone development. However, no dietary information or characteristics related with kidney stone production were collected.
Participants with HCV infection had a higher chance of acquiring kidney stones. Infection with HCV may be a risk factor for the production of kidney stones in US women.
The datasets presented in this study can be found in online repositories. The names of the repository/repositories and accession number(s) can be found in the article/Supplementary material.
The studies involving human participants were reviewed and approved by NCHS's Institutional Review Board reviewed and approved the study protocol. The patients/participants provided their written informed consent to participate in this study.
YC: conceptualization, methodology, and software. XS: data curation and writing—original draft. HL and GL: visualization and investigation. KH: supervision and software. CL and ZH: writing—review and editing. All authors contributed to the article and approved the submitted version.
This work was supported by the National Natural Science Foundation of China (82070724) and Natural Science Foundation of Anhui Province (1908085MH246).
The authors are grateful for the invaluable support and useful discussions with other members of the urological department.
The authors declare that the research was conducted in the absence of any commercial or financial relationships that could be construed as a potential conflict of interest.
All claims expressed in this article are solely those of the authors and do not necessarily represent those of their affiliated organizations, or those of the publisher, the editors and the reviewers. Any product that may be evaluated in this article, or claim that may be made by its manufacturer, is not guaranteed or endorsed by the publisher.
The Supplementary Material for this article can be found online at: https://www.frontiersin.org/articles/10.3389/fpubh.2022.940905/full#supplementary-material
HCV, Hepatitis C virus; NHANES, National Health and Nutrition Examination Survey; HCV-Ab, Hepatitis C virus antibody; HCV-RNA, Hepatitis C virus RibonucleicAcid; OR, Odds ratio; BMI, Body mass index; US, United States; PIR, Ratio of family income to poverty.
1. Ferraro PM, Bargagli M, Trinchieri A, Gambaro G. Risk of kidney stones: influence of dietary factors, dietary patterns, and vegetarian-vegan diets. Nutrients. (2020) 12:779. doi: 10.3390/nu12030779
2. Chen JY, Sun XY, Ouyang JM. Modulation of calcium oxalate crystal growth and protection from oxidatively damaged renal epithelial cells of corn silk polysaccharides with different molecular weights. Oxid Med Cell Longev. (2020) 2020:6982948. doi: 10.1155/2020/6982948
3. Hill AJ, Basourakos SP, Lewicki P, Wu X, Arenas-Gallo C, Chuang D, et al. Incidence of kidney stones in the United States: the continuous national health and nutrition examination survey. J Urol. (2022) 207:851–6. doi: 10.1097/JU.0000000000002331
4. Scales CD Jr, Smith AC, Hanley JM, Saigal CS. Prevalence of kidney stones in the United States. Eur Urol. (2012) 62:160–5. doi: 10.1016/j.eururo.2012.03.052
5. Abufaraj M, Xu T, Cao C, Waldhoer T, Seitz C, D'andrea D, et al. Prevalence and trends in kidney stone among adults in the USA: analyses of National Health and Nutrition Examination survey 2007-2018 data. Eur Urol Focus. (2021) 7:1468-75. doi: 10.1016/j.euf.2020.08.011
6. Sorokin I, Mamoulakis C, Miyazawa K, Rodgers A, Talati J, Lotan Y. Epidemiology of stone disease across the world. World J Urol. (2017) 35:1301–20. doi: 10.1007/s00345-017-2008-6
7. Zeng G, Mai Z, Xia S, Wang Z, Zhang K, Wang L, et al. Prevalence of kidney stones in China: an ultrasonography based cross-sectional study. BJU Int. (2017) 120:109–16. doi: 10.1111/bju.13828
8. Masterson JH, Phillips CJ, Crum-Cianflone NF, Krause RJ, Sur RL, L'Esperance JO. A 10-year retrospective review of nephrolithiasis in the navy and navy pilots. J Urol. (2017) 198:394–400. doi: 10.1016/j.juro.2017.02.074
9. Chen WC, Chou WH, Chu HW, Huang CC, Liu X, Chang WP, et al. The rs1256328 (ALPL) and rs12654812 (RGS14) polymorphisms are associated with susceptibility to calcium nephrolithiasis in a Taiwanese population. Sci Rep. (2019) 9:17296. doi: 10.1038/s41598-019-53261-8
10. Bobulescu IA, Park SK, Xu L, Blanco F, Poindexter J, Adams-Huet B, et al. Net acid excretion and urinary organic anions in idiopathic uric acid nephrolithiasis. Clin J Am Soc Nephrol. (2019) 14:411–20. doi: 10.2215/CJN.10420818
11. Liang Y, Cao X, Ding Q, Zhao Y, He Z, Zhong J. Hepatitis C virus NS4B induces the degradation of TRIF to inhibit TLR3-mediated interferon signaling pathway. PLoS Pathog. (2018) 14:e1007075. doi: 10.1371/journal.ppat.1007075
12. Wang Q, Li Y, Liu SA, Xie W, Cheng J. Cell culture-adaptive mutations in hepatitis C virus promote viral production by enhancing viral replication and release. World J Gastroenterol. (2018) 24:1299–311. doi: 10.3748/wjg.v24.i12.1299
13. Hassanin TM, Abdelraheem EM, Abdelhameed S, Abdelrazik M, Fouad YM. Detection of hepatitis C virus core antigen as an alternative method for diagnosis of hepatitis C virus infection in blood donors negative for hepatitis C virus antibody. Eur J Gastroenterol Hepatol. (2020) 32:1348–51. doi: 10.1097/MEG.0000000000001647
14. Grimm J, Peschel G, Müller M, Schacherer D, Wiest R, Weigand K, et al. Rapid Decline of serum proprotein convertase Subtilisin/Kexin 9 (PCSK9) in non-cirrhotic patients with chronic hepatitis C infection receiving direct-acting antiviral therapy. J Clin Med. (2021) 10:1621. doi: 10.3390/jcm10081621
15. Esmaeili A, Mirzazadeh A, Carter GM, Esmaeili A, Hajarizadeh B, Sacks HS, et al. Higher incidence of HCV in females compared to males who inject drugs: a systematic review and meta-analysis. J Viral Hepat. (2017) 24:117–27. doi: 10.1111/jvh.12628
16. Ahmed MZ, Shahzad H, Rao T, Ali A, Samad N. Seroprevalence of hepatitis C virus (HCV) and hepatitis B Virus (HBV) in district Vehari, Pakistan. J Coll Physicians Surg Pak. (2020) 30:550–1. doi: 10.29271/jcpsp.2020.05.550
17. Moorman JP, Fitzgerald SM, Prayther DC, Lee SA, Chi DS, Krishnaswamy G. Induction of p38- and gC1qR-dependent IL-8 expression in pulmonary fibroblasts by soluble hepatitis C core protein. Respir Res. (2005) 6:105. doi: 10.1186/1465-9921-6-105
18. Ramos-Casals M, Zignego AL, Ferri C, Brito-Zerón P, Retamozo S, Casato M, et al. Evidence-based recommendations on the management of extrahepatic manifestations of chronic hepatitis C virus infection. J Hepatol. (2017) 66:1282–99. doi: 10.1016/j.jhep.2017.02.010
19. Fabrizi F, Verdesca S, Messa P, Martin P. Hepatitis C virus infection increases the risk of developing chronic kidney disease: a systematic review and meta-analysis. Dig Dis Sci. (2015) 60:3801–13. doi: 10.1007/s10620-015-3801-y
20. Kaartinen K, Vuoti S, Honkanen E, Löyttyniemi E, Singh R, Färkkilä M. Tubular cell damage may be the earliest sign of renal extrahepatic manifestation caused by Hepatitis C. PLoS ONE. (2021) 16:e0251392. doi: 10.1371/journal.pone.0251392
21. Yang J, Li Y, Liu Q, Li L, Feng A, Wang T, et al. Brief introduction of medical database and data mining technology in big data era. J Evid Based Med. (2020) 13:57–69. doi: 10.1111/jebm.12373
22. Wu WT Li YJ, Feng AZ Li L, Huang T, Xu AD, et al. Data mining in clinical big data: the frequently used databases, steps, and methodological models. Mil Med Res. (2021) 8:44. doi: 10.1186/s40779-021-00338-z
23. Johnson CL, Paulose-Ram R, Ogden CL, Carroll MD, Kruszon-Moran D, Dohrmann SM, et al. National health and nutrition examination survey: analytic guidelines, 1999-2010. Vital Health Stat. (2013) 2:1–24.
24. Churpek MM, Yuen TC, Winslow C, Meltzer DO, Kattan MW, Edelson DP. Multicenter Comparison of Machine Learning Methods and Conventional Regression for Predicting Clinical Deterioration on the Wards. Crit Care Med. (2016) 44:368–74. doi: 10.1097/CCM.0000000000001571
25. von Elm E, Altman DG, Egger M, Pocock SJ, Gøtzsche PC, Vandenbroucke JP. The Strengthening the Reporting of Observational Studies in Epidemiology (STROBE) statement: guidelines for reporting observational studies. Bull World Health Organ. (2007) 85:867–72. doi: 10.2471/BLT.07.045120
26. Rule AD, Lieske JC, Pais VM Jr. Management of kidney stones in 2020. JAMA. (2020) 323:1961–2. doi: 10.1001/jama.2020.0662
27. Tang J, Mettler P, McFann K, Chonchol M. The association of prevalent kidney stone disease with mortality in US adults: the National Health and Nutrition Examination Survey III, 1988-1994. Am J Nephrol. (2013) 37:501–6. doi: 10.1159/000350691
28. Liang L, Li L, Tian J, Lee SO, Dang Q, Huang CK, et al. Androgen receptor enhances kidney stone-CaOx crystal formation via modulation of oxalate biosynthesis and oxidative stress. Mol Endocrinol. (2014) 28:1291–303. doi: 10.1210/me.2014-1047
29. Blach S, Zeuzem S, Manns M, Altraif I, Duberg AS, Muljono DH, et al. Global prevalence and genotype distribution of hepatitis C virus infection in 2015: a modelling study. Lancet Gastroenterol Hepatol. (2017) 2:161–76. doi: 10.1016/S2468-1253(16)30181-9
30. Hepatitis C. virus prevalence and level of intervention required to achieve the WHO targets for elimination in the European Union by 2030: a modelling study. Lancet Gastroenterol Hepatol. (2017) 2:325–36.
31. Khalili P, Jamali Z, Sadeghi T, Esmaeili-Nadimi A, Mohamadi M, Moghadam-Ahmadi A, et al. Risk factors of kidney stone disease: a cross-sectional study in the southeast of Iran. BMC Urol. (2021) 21:141. doi: 10.1186/s12894-021-00905-5
32. Kim S, Chang Y, Yun KE, Jung HS, Kim I, Hyun YY, et al. Metabolically healthy and unhealthy obesity phenotypes and risk of renal stone: a cohort study. Int J Obes. (2019) 43:852–61. doi: 10.1038/s41366-018-0140-z
33. Biliotti E, Palazzo D, Tinti F, D’Alessandro MD, Esvan R, et al. HCV cirrhotic patients treated with direct-acting antivirals: detection of tubular dysfunction and resolution after viral clearance. Liver Int. (2021) 41:158–67. doi: 10.1111/liv.14672
34. Sansonno D, Lauletta G, Montrone M, Grandaliano G, Schena FP, Dammacco F. Hepatitis C virus RNA and core protein in kidney glomerular and tubular structures isolated with laser capture microdissection. Clin Exp Immunol. (2005) 140:498–506. doi: 10.1111/j.1365-2249.2005.02778.x
Keywords: kidney stone, hepatitis C virus (HCV), National Health and Nutrition Examination Survey(NHANES), nephrolithiasis, cross-section study
Citation: Chen Y, Shen X, Liang H, Li G, Han K, Liang C and Hao Z (2022) Relationship between hepatitis C and kidney stone in US females: Results from the National Health and Nutrition Examination Survey in 2007–2018. Front. Public Health 10:940905. doi: 10.3389/fpubh.2022.940905
Received: 10 May 2022; Accepted: 14 July 2022;
Published: 05 August 2022.
Edited by:
Jian Wu, Zhejiang University, ChinaReviewed by:
Jun Lyu, First Affiliated Hospital of Jinan University, ChinaCopyright © 2022 Chen, Shen, Liang, Li, Han, Liang and Hao. This is an open-access article distributed under the terms of the Creative Commons Attribution License (CC BY). The use, distribution or reproduction in other forums is permitted, provided the original author(s) and the copyright owner(s) are credited and that the original publication in this journal is cited, in accordance with accepted academic practice. No use, distribution or reproduction is permitted which does not comply with these terms.
*Correspondence: Chaozhao Liang, bGlhbmdfY2hhb3poYW9AYWhtdS5lZHUuY24=; Zongyao Hao, aGFvem9uZ3lhb0AxNjMuY29t
†These authors have contributed equally to this work
Disclaimer: All claims expressed in this article are solely those of the authors and do not necessarily represent those of their affiliated organizations, or those of the publisher, the editors and the reviewers. Any product that may be evaluated in this article or claim that may be made by its manufacturer is not guaranteed or endorsed by the publisher.
Research integrity at Frontiers
Learn more about the work of our research integrity team to safeguard the quality of each article we publish.