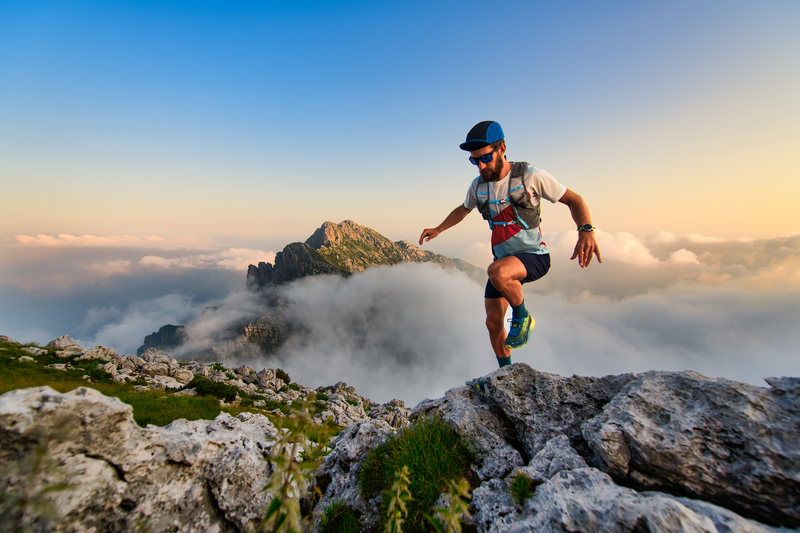
94% of researchers rate our articles as excellent or good
Learn more about the work of our research integrity team to safeguard the quality of each article we publish.
Find out more
SYSTEMATIC REVIEW article
Front. Public Health , 06 July 2022
Sec. Life-Course Epidemiology and Social Inequalities in Health
Volume 10 - 2022 | https://doi.org/10.3389/fpubh.2022.923744
This article is part of the Research Topic Physical Activity, Health Equity and Health-Related Outcomes, Volume II View all 14 articles
Background: Non-communicable diseases among children are serious consequences of childhood obesity. However, less is known about the disparities in childhood obesity comorbidities burden. This review describes the salient pattern of disparities in the prevalence of childhood obesity-related non-communicable diseases and relevant inequalities in both high- and low/medium-income countries.
Method: A systematic literature search was performed in MEDLINE, Embase, CINAHL, PsycInfo, Scopus, and Web of Science databases by two independent reviewers. Inclusion criteria were as follows: age 2–18 years; the prevalence or incidence of childhood obesity comorbidities reported; and studies published in English from January 2010 to date. No restrictions on the setting. The prevalence data were analyzed using range and median for subgroups based on the country's development status, gender, and geographical region.
Results: Our search identified 6,837 articles, out of which we examined 145 full-text articles and included 54 articles in the analysis. The median prevalence of childhood obesity-related hypertension was 35.6 vs. 12.7% among middle- and low-income countries compared with high-income countries; 37.7 vs. 32.9% among boys compared with girls; and 38.6, 25.3, and 20.1% in Asia, South America, and Europe, respectively. For metabolic syndrome, the median prevalence was 26.9 vs. 5.5% among middle- and low-income countries compared with high-income countries; 55.2 vs. 12.0% among boys compared with girls; and 40.3, 25.8, and 7.7% in South America, Asia, and Europe, respectively. The prevalence of childhood obesity-related non-alcoholic fatty liver disease was 47.5 vs. 23% among middle- and low-income countries compared with high-income countries; and 52.1, 39.7, and 23.0% in Asia, South America, and Europe, respectively. The median prevalence of dyslipidemia was 43.5 vs. 63% among middle- and low-income countries compared with high-income countries; 55.2 vs. 12.0% among boys compared to girls; and 73.7 and 49.2% in Australia and Europe, respectively.
Conclusion: There are disparities in the prevalence of childhood obesity-related hypertension, metabolic syndrome, and non-alcoholic fatty liver disease, with middle- and low-income countries, boys, and Asian region having higher prevalence. Implementing targeted interventions for childhood obesity comorbidities should consider socioeconomic disparities and strengthening of research surveillance methods for a better understanding of non-communicable disease burden in the pediatric population.
Systematic Review Registration: https://www.crd.york.ac.uk/PROSPERO, identifier: CRD42021288607.
There is a growing burden of non-communicable diseases (NCD) among children and adolescents worldwide (1). Over 2.1 billion children and adolescents under the age of 20 years were estimated to be affected by NCD globally. These include cardiovascular diseases (CVD) (13.9 million), cancers (5.9 million), chronic respiratory disorders (108.9 million), type 2 diabetes mellitus (T2DM) (8.8 million), mental health disorders (231.3 million), and injuries and violence (170.4 million). Children with NCD face lifelong burden of illness and contribute to over one-thirds of adulthood NCD incidence (2). The rise in the risk of NCD in children has been associated with the increase in childhood obesity prevalence (3, 4). Childhood obesity rate increased over 3-fold worldwide over the past three decades (5), indicating a growing global epidemic of childhood obesity. It is estimated that about 10% of school-aged children worldwide contribute to childhood obesity with an increased risk of developing chronic NCD (2). Besides the increased risk of NCD, childhood obesity causes wider societal impact such as stigma and discrimination, increased care-related cost to the affected families, cost to the community through the diversion of resources for the treatment of obesity and related conditions, and the direct healthcare cost for treating obesity and related conditions. It is estimated that obesity constitutes l−5% of total healthcare costs in various countries (6).
The American Medical Association (AMA) and World Health Organization (WHO) recognized obesity as a distinct disease (7, 8). Moreover, the World Obesity Federation identifies it as a “chronic, relapsing, progressive, disease process” (9). Obesity as a disease entity may coexist with other conditions as part of multimorbidity (MM) or as an index condition in comorbidity (10–14). MM and comorbidity increase the complexities of long-term care of affected individuals (15). In this regard, the most commonly reported obesity comorbidities include hypertension, T2DM, non-alcoholic fatty liver disease (NAFLD), CVD, and a cluster of NCD such as metabolic syndrome.
The mechanism through which obesity leads to the development of NCD is complex, but it involves its inflammatory, oxidative stress, and insulin resistance effects (16). Insulin resistance leads to compensatory hyperinsulinemia, which in turn is responsible for most of the metabolic and cardiovascular comorbidities associated with obesity (17, 18). Insulin resistance is the first step in T2DM pathogenesis followed by impaired insulin secretion, which ultimately manifests as clinical T2DM (17–19). Insulin resistance is also part of the metabolic syndrome, characterized by hyperinsulinemia, insulin resistance, dyslipidemia, hypertension, and central obesity (20), and is implicated in gastrointestinal comorbidities such as NAFLD (21).
The association between obesity and NCD has been demonstrated in several observational studies (22–27). For example, Pantalone et al. (24) found that there was a higher prevalence of T2DM, pre-diabetes, hypertension, and CVD in higher body mass index (BMI) categories compared with lower BMI, p < 0.0001. A recent systematic review and meta-analysis of 52 studies demonstrated the association between childhood obesity and NCD, with prevalence ratios of 1.4 for hyperlipidemia, 21.2 for CVD, 26.1 for NAFLD, 1.7 for pulmonary disorders, and 4.0 for hypertension (23). The Bogalusa Heart Study, a long-term epidemiological study in Louisiana, USA, also showed that hypertension increased 8.5-fold and dyslipidemia increased from 3.1- to 8.3-fold in overweight adolescents as compared with healthy weight adolescents (28, 29). Therefore, obesity in childhood that persists in adolescence has a causal link with multiple NCD.
Disparities in childhood obesity prevalence are well documented, especially those related to ethnicity and socioeconomic status. For example, in a study of ethnic and race disparity in early childhood obesity, Zilanawala et al. (30) reported that, compared with white children, the odd of obesity was higher among black Caribbean children, odds ratio (OR) = 1.7 (95% CI: 1.1–2.6), whereas Pakistani children had a lower OR = 0.60 (95%CI: 0.37–0.96). On the contrary, black African children were more likely to be overweight, OR = 1.40 (95% CI: 1.04–1.88) (30). Falconer et al. (31) found that among school-aged children in England, a higher percentage of Asian and black children than white children were overweight or obese (21–27 vs. 16%), lived in the most deprived areas (24–47 vs. 14%), and reported lifestyle that leads to obesity (38 vs. 16%). With regard to socioeconomic factors, studies show that socioeconomic disadvantage is associated with childhood obesity that is sustained in subsequent generations (32). In a cohort of 22,810 participants, BMI was found to be higher in those in the lowest socioeconomic class than those in the highest socioeconomic class by 2.0 kg/m2 (p < 0.001) (33). Analysis of a nationally representative data in the United States (USA) showed that children from middle- and high-income households were 0.78 (95% CI: 0.72–0.83) and 0.68 (95% CI: 0.59–0.77) times more likely to be overweight or obese compared with those from low-income households (34). The United Kingdom's (UK) Millennium Cohort Study shows that at the age of 17 years, 27.6% of those from the lowest income quintile classify as obese compared with 13.7% (p = 0.001) of those from the richest households (35). These studies provide evidence of disparities in childhood obesity by ethnicity and socioeconomic status.
Despite ample evidence of disparities in childhood obesity, there is a lack of literature on disparities in the prevalence of childhood obesity-related comorbidities. Often, literature on childhood obesity-related comorbidities describes the association between overweight/obesity and comorbidities (23). In a few instances, gender disparity in prevalence is reported (36–38). Nevertheless, there are some indications of ethnic disparity in the prevalence of NCD among children with obesity. For example, Cheung et al. (39) showed that the prevalence of hypertension among obese adolescents differed by ethnicity, with a significant difference among Hispanic (3.1%), African Americans (2.7%), and white (2.6%) adolescents (p = 0.02). Although it is not clear whether and how disparities in childhood obesity-related comorbidities prevalence are based on socioeconomic status, recent systematic reviews have shown disparities in MM burden between low- and middle-income countries (LMICs) and high-income countries (HICs) (14, 40–42). Such analyses are essential for devising effective preventative approaches in community and primary care settings (41).
Because many kinds of NCD are associated with obesity, prevention and management of obesity and its related NCD have become the focus of attention worldwide (43). A good understanding of disparities in childhood obesity-related NCD prevalence among different population groups provides insight into potential reasons for the differences and helps to address the common risk factors. This supports the WHO global strategy of reducing NCD burden through an integrated prevention approach of risk factors at individual, family, community, and population level (44). However, currently, there is no systematic review that has analyzed disparities in childhood obesity-related comorbidities prevalence. While there is evidence of disparity in childhood obesity burden, such evidence cannot be extrapolated to explain the disparity in childhood obesity-related NCD prevalence. Therefore, this review aims to describe the salient pattern of disparities in the prevalence of childhood obesity-related NCD comorbidities between HICs and LMICs, by gender and geographical region, with a view to potentially inform the development of interventions that address the most at-risk groups worldwide.
The protocol for this review was registered with the International Prospective Register of Systematic Reviews (PROSPERO: CRD42021288607), and the Preferred Reporting Items for Systematic Reviews and Meta-Analyses (PRISMA) statement was followed (45). We conducted a systematic search in MEDLINE, Embase, CINAHL, PsycInfo, Scopus, and Web of Science using a combination of free text and medical subheadings (MeSH) terms. We also searched the general Internet using the Discovery portal and a list of reference of relevant articles. Initially, a specific search strategy for MEDLINE was developed (Search strategies are available via the PROSPERO registry). This was then adapted to other databases. The search terms used were as follows: (children or adolescents or pediatric or students or school pupils or youth or boys or girls or school age or juvenile or preteens or teens) AND (obesity or body weight or adiposity or body mass index or waist circumference or neck circumference) AND (comorbidity or Type 2 Diabetes Mellitus or hypertension or high blood pressure or cardiovascular disease or CVD or metabolic syndrome or non-alcoholic fatty liver disease or NAFLD or depression or psychological problem or anxiety or self-esteem or sleep apnea or asthma or respiratory problem or dyslipidemia or musculoskeletal problems) AND (prevalence or incidence or odds ratio or risk ratio or occurrence or epidemiology).
The inclusion criteria for selecting studies were as follows: (1) Participants were children or adolescents aged 2–18 years, based on the WHO definition of childhood obesity (3); however, some countries used above 18 years as childhood cutoff point (46). (2) Data that were reported on the prevalence or incidence of comorbidities or relevant information could be used to generate reliable prevalence estimates of childhood obesity-related NCD comorbidities. (3) The studies used a cross-sectional or case–control or prospective cohort design or randomized control trial where prevalence or incidence data were reported. (4) Only studies published in English or translated into English from January 2010 were included to reflect more current information. (5) There were no restrictions on the setting. Studies reporting prevalence/incidence of childhood obesity comorbidity at national or specific setting such as the community, school setting, or primary care worldwide were included.
Studies were excluded if they (1) were conducted in selected population groups, such as those identified through special clinics and children with obesity as a symptom of an underlying condition, for example, Prader-Willi syndrome, Cushing syndrome, hypothyroidism, and Hashimoto disease, or a side effect from medication such as antipsychotics; (2) were case series, opinion papers, and all types of qualitative studies. Whenever a particular dataset was published more than once, the most recent publication was used.
Two reviewers screened the title and abstract of retrieved articles to exclude studies that were not eligible. The two reviewers independently appraised the full text of studies that met the inclusion criteria. Any discrepancies were resolved through consensus. Search results are reported according to the Preferred Reporting Items for Systematic Reviews and Meta-analyses (PRISMA) flow diagram (47), including reasons for excluding a full-text study. The reviewers were not blind to the journal titles.
The two reviewers assessed the quality of selected studies independently using the Joanna Briggs Institute's (JBI) Critical Appraisal Checklist for Studies Reporting Prevalence Data (48, 49), adopting a scoring system of 0–5 for poor quality and 6–10 for high quality. The checklist assesses sample representativeness, reliability of measurement, and whether sufficient details of weight classification and comorbidity definition were reported. Each of the two reviewers independently assessed the quality of each selected article. Discrepancies were resolved through consensus. Poor-quality studies were excluded from the analysis.
A single reviewer extracted data regarding the first author, year of publication, study design, country, sample size, participants' age group, type of comorbidity, diagnosis criteria, and the reported prevalence or incidence into a spread sheet. The second reviewer examined the extracted data and any discrepancies were resolved through discussions.
The extracted data were first grouped by the countries' developmental/income status (HICs vs. LMICs, according to the United Nations development status, 1999 and World Bank classification of countries by income) (50, 51); geographical region (North America, South America, Europe, Asia, Africa, and Australasia); and gender (male vs. female). Comorbidities were grouped into the following categories: (1) Metabolic syndrome, (2) dyslipidemia, (3) hypertension, (4) NAFLD, (5) pulmonary disorder, (6) psychological comorbidities, and (7) other comorbidities. To compare the prevalence of a specific comorbidity between subgroups, descriptive statistics of range and median were used to summarize the prevalence estimate of the subgroups within development/income status, geographical region, and gender (52). SPSS version 26 was used to calculate the median based on the formula: [(n + 1) ÷ 2]th, where “n” is the number of items in the set and “th” just means the (n)th number (52). Meta-analysis was not possible because the studies were not sufficiently homogeneous in terms of methodology, participants' age groups, and measurement.
The search identified a total of 6,868 articles, 301 of which were duplicates. Titles and abstracts of 6,837 articles were screened, resulting in 145 full texts being examined for eligibility. Totally, 54 of the examined full texts met our inclusion criteria (Figure 1).
Figure 1. Flowchart of study selection based on Preferred Reporting Items for Systematic Reviews and Meta-Analysis (PRISMA) guidelines.
The 54 selected studies that described the prevalence of childhood obesity comorbidities in the obese weight categories included a total of 651,659 participants. The 54 studies were conducted in 27 countries across five continents, with 27 articles from Asia, 17 from Europe, six from South America, two from North America, one from Africa, and one from Australasia (Table 1). The most commonly reported comorbidity was hypertension, followed by metabolic syndrome (NB includes hypertension) and dyslipidemia. The least reported comorbidities were asthma and emotional disorders (Table 1).
While a few studies provided separate estimates for children (≤ 10 years) and adolescents (≥10 years) (53, 54), the majority reported combined prevalence, with ages ranging from 2 to 18 years. This means that the prevalence of different age subgroups could not be compared. Eleven papers reported assessing prevalence by gender, but only two provided breakdowns by gender and age group. All the studies were observational in design with the majority being cross-sectional surveys. Table 2 summarizes the characteristics of included studies.
There was evidence of disparities in childhood obesity-related comorbidity prevalence between HICs and LMICs as shown in Table 3. The prevalence of hypertension in LMICs ranged from 13.8 to 60.7%, with a median of 35.6%, whereas in the HICs it ranged from 3 to 26% with a median of 12.7%. The highest hypertension prevalence, 60.7%, was reported among Chinese males (109), followed by Indian girls at 50% (86), then Thai children at 49.5 % (80), and Turkish males at 43.5% (110).
Table 3. Childhood obesity comorbidity prevalence range in children aged 2–18 years by development status.
Similarly, the prevalence of metabolic syndrome among children with obesity was shown to be higher among the LMICs, with a range of 12 to 72.8% and a median of 26.9%, compared with a range of 3 to 8% and a median of 5.5% in HICs. Colombian children had the highest prevalence of metabolic syndrome at 72.8% (108), followed by Chinese children with a prevalence of 41% and Mexican children at 40% (59, 85). Among HICs, Italian children had the highest prevalence of metabolic syndrome at 8.2% and Danish children had the lowest prevalence at 3.1% (96, 102).
The prevalence of childhood obesity-related NAFLD was higher among children from LMICs with a prevalence range of 31.6 to 56.4% and a median of 47.5%, compared with a range of 9.5–35.4% and a median of 23% in HICs. Up to 56.4% of Turkish children were reported to have NAFLD (82). The highest prevalence among HICs, 35.4%, was reported among Italian children (97).
The prevalence pattern of dyslipidemia, however, differed from the other comorbidities. Higher prevalence of dyslipidemia was reported among the HICs with a range of 52 to 74% and a median of 63% compared with a range of 43 to 52% and a median of 43.5% in LMICs. Canadian and Australian children had a prevalence of 52 and 74%, respectively (101, 103), while Turkish and Chilean children had a prevalence of 43 and 54%, respectively (82, 108).
A limited number of studies reported respiratory problems (asthma and wheezes) among children with obesity (95, 100), as well as the prevalence of psychological problems of anxiety and depression (90). However, disparity was difficult to discern for these comorbidities due to the small number of studies that met the inclusion criteria.
In total, eleven of the included studies disaggregated data by gender for the comorbidities of hypertension and metabolic syndrome. Nine reported on hypertension disaggregated by gender (53, 56, 62, 73, 79, 86, 87, 99, 111). Hypertension prevalence among girls ranged from 11.0 to 58.2% with a median of 32.9%. Among boys, the range was 21.0 to 60.7% with a median of 37.6% (Table 4). Only two studies reported metabolic syndrome by gender (87, 88). One of the studies showed a marked difference in the prevalence of metabolic syndrome between boys and girls (55.2 vs. 12.0%) (88).
The range of the prevalence of hypertension among obese children in Asia was 11.0 to 60.7%, median 38.6%, compared with 18.8 to 30.9%, median 25.3% in South America, and 8.6 to 55.0%, median of 20.1% in Europe. North America had only one study that report on hypertension, whereas Africa, Australia, and Antarctica had none.
The prevalence range of metabolic syndrome was 26.3 to 72.8%, median 40.3% in South America; 6.6 to 52.1%, median 25.8% in Asia; and 7.7 to 33.0%, median 7.7% in Europe. One study reported on metabolic syndrome from North Africa with a prevalence of 14.3% (63), while none was reported from the remaining global regions (Australasia and North America).
With regard to NAFLD, the prevalence ranged from 45.0 to 52.1%, median 52.1% in Asia; 26.0 to 50.0%, median 39.7% in South America; and 9.5 to 36.4%, median 23% in Europe. The other regions had no studies reporting on NAFLD. For anxiety/depression, only one study was reported from Asia, with a prevalence of 30.9%, and one from Europe, with a prevalence of 16.8%. Similarly, two studies reported dyslipidemia from Europe, prevalence 43.5 to 54%, median 48.8%, and two from South America, prevalence 52.0 to 74%, median 63%. One study in North America reported on Asthma, prevalence 11.6% (95).
The main findings of this review were that the prevalence of childhood obesity-related hypertension, metabolic syndrome, and NAFLD were higher among populations in LMICs than those in HICs. Gender disparity was also apparent among children with obesity-related hypertension and dyslipidemia, where the prevalence was higher among boys than girls. Globally, the Asian region had the highest prevalence of childhood obesity-related hypertension followed by South America and then Europe. The prevalence of childhood obesity-related metabolic syndrome was highest in South America, followed by Asia and then Europe.
Explaining the observed disparities in childhood obesity-related comorbidities is expected to be as complex as defining populations' obesity disparity because of the intricate interplay between adiposity and known NCD risk factors, such as biological, environmental, lifestyle, genetic, socioeconomic, racial, and cultural factors. Nevertheless, understanding the prevalence of obesity-related NCD across pediatric populations, through the review of studies that described childhood obesity comorbidities (Table 2), is likely to provide evidence that could help develop strategies to ameliorate obesity and associated health risks in the pediatric population.
The review showed that LMICs and global regions with lower income status had higher prevalence of the common childhood obesity-related NCD than HICs (Tables 1, 3). This is consistent with reports that populations living in LMICs or having low socioeconomic status in HICs are at increased risk of developing NCD such as CVD, NAFLD, and T2DM (112–114). For example, the adjusted incidence of stroke was reported to have doubled from 52 to 117 per 100,000 person-years in LMICs, but decreased in HICs by about 42% over a 4-year period (113). Furthermore, Sposato et al. (114) also found that lower per capita gross domestic product adjusted for purchasing power parity correlated with a higher incident risk of stroke (p = 0.027, R2 = 0.32) (114). While it is estimated that the global NCD burden will increase by 17% in the next 10 years, in the African region it is projected to increase by up to 27% (5). Similarly, almost half of all deaths of all ages in Asia are now attributable to NCD, accounting for 47% of the global burden of disease (5). At present, India is projected to have the highest global number of patients with diabetes, at 79.4 million in 2030 (115). Therefore, understanding NCD burdens specific to LMICs in the pediatric populations could help in devising effective prevention strategies for childhood obesity and its consequences based on the understanding of challenges found in LMICs. Such prevention challenges that may be shared with high-risk adult population include poverty, increased urbanization, lifestyle factors, and poor air quality, which are common in LMICs (14).
Although evidence suggests that living in LMICs is associated with an increased risk of developing NCD, the causal pathway between socioeconomic status and NCD is complex and not well understood (116). Some studies suggest that earlier adoption of healthy behaviors by advantaged socioeconomic groups is followed by an increased prevalence of risky behaviors among the disadvantaged socioeconomic groups (117, 118). For example, Marins et al. (119) reported a strong association between a low level of schooling and cardiovascular risk factors in an urban center in Brazil (OR1·77, 95% CI 1·39–2·26). While there is no conclusive explanation of the causal pathway between socioeconomic status and NCD, our analysis shows that children from LMICs are at more risk of NCD (Table 4). The reasons for this observed pattern may include material deprivation, exposure to unhealthy living conditions and unsafe environment, and limited access to high-quality health services and prevention interventions (120, 121). Adopting NCD prevention through awareness and behavioral interventions in LMICs should therefore adopt an economic-based model, in which community disparities are addressed. Such an approach was recently suggested for preventing MM in LMICs through targeting a cluster of NCD with a multipronged intervention approach (14). This may be adopted for specific age groups, including children with obesity, especially personalized interventions that prevent several metabolic risk factors (122, 123).
Several studies have reported that rapid urbanization and air pollution are linked to NCD in LMICs for all adults (124–130), and hence, children might be particularly disadvantaged in this regard and likely to benefit from early interventions that are appropriate for LMICs. In addition, relative poverty and its associated adverse effects on intrauterine environment affect the functional development of a fetus and lead to an increased risk of development of NCD in adolescence and later life (131). Epidemiological studies have linked small size births with increased risk of CVD and other NCD (132). Insufficient nutrition in the intrauterine life may result in increased susceptibility to lifestyle-related NCD risk factors (133). This is an important factor in childhood NCD burden in many LMICs, where the realities are increased urbanization associated with high levels of urban poverty and marginalization of the rural poor (134, 135). These might, in part, explain the observed differences in childhood obesity-related hypertension, metabolic syndrome, and NAFLD prevalence between LMICs and HICs reported in this review (Table 3).
In terms of ethnic and cultural disparity, ethnic minority groups within HICs are known to have a higher risk of obesity and associated NCD such as insulin resistance and metabolic disease (118). Several studies have reported the interplay among lifestyle, environmental, and genetic factors to explain a higher degree of hyperinsulinemia and correlation with adiposity, among South Asian compared with white children of similar age (131, 136–138). However, explaining disparities due to geographical ethnicity or country found in this review (Table 2) is complex. Biologically mediated racial or ethnic differences in NCD risks are reported but the actual genetic differences remain unclear as putative genes or gene variants have not yet been identified (139). However, exposure to NCD during fetal life and infancy is reported to increase the risk of developing childhood NCD (140). The Bogalusa Heart Study, for example, shows that the offspring of diabetic parents displays quicker progression to insulin resistance characteristics in the early years to adolescence (25), providing plausible evidence of biological factors at play. High prevalence of adult NCD among some population groups, therefore, increases the risk of childhood NCD in their offspring.
Interestingly, the trend in the prevalence of dyslipidemia departed from other childhood obesity-related NCD between HICs and LMICs in this review (Table 3). Although some previous studies in adults have shown lower levels of lipids in ethnic minority groups than in white counterparts, for instance, the mean plasma triglyceride concentration was estimated to be 20 mg/dl lower in African-Americans compared with their white counterparts (141), several studies have also shown that dyslipidemia and excessive body fat occur at lower levels of BMI in South Asians than in white ethnic group (131, 142–144). Given that there were only four studies that reported on dyslipidemia in this review, the differences may not reflect the true picture of disparity.
Our results on gender disparity in childhood comorbidity (hypertension and metabolic syndrome) in boys than girls are irrespective of whether they occurred within LMICs or HICs. This is consistent with previous reports on gender disparity in obesity among children. World Obesity Federation Atlas of Childhood Obesity (2019) showed that about 65% of countries reported a higher prevalence of obesity among boys than girls aged 5–19 years, most of which were in high- and middle-income countries (3). Furthermore, the national Canadian data 2004–2013 showed a 2-fold higher prevalence of obesity among boys than girls (145). Similarly, in China, among children aged 7–18 years, boys had a higher prevalence of obesity than girls (146). In the UK, the National Child Measurement Program (NCMP) shows that boys, children from most deprived areas, and ethnic minorities have a disproportionately higher prevalence of obesity (147). These differences are attributed to risk factors shared with other NCD such as biological differences in body composition and sociocultural differences between sexes. For example, girls in the HICs are reported to prefer food lower in energy, while boys consume more meat and energy-dense food (148). The findings of the review are therefore consistent with the expected gender disparities in obesity-related NCD.
Given the disparity in burden and underlying risks factors of childhood obesity comorbidities, the key implications for practice and research are summarized in Box 1.
Box 1. Implications for practice and research.
• The need for interventions that target at-risk population groups to be culturally and contextually sound through the involvement of specific population group. This should take into consideration the socioeconomic realities of the specific population, informed by local evidence and cultural appropriateness.
• The need to design and conduct research studies on how best to reach the at-risk population with an individualized approach in countries with resource constraints and weak health systems.
• The need to strengthen methods for surveillance of childhood obesity-related NCD to guide local policies and interventions. The WHO STEP-wise survey approach has a limitation when it comes to children as it targets adults aged 25–64 years (145). Development of tailored methodological approaches that take into consideration childhood obesity-related NCD is therefore required.
First of all, we included children aged 2 and 18 years based on the WHO definition of childhood obesity (3). However, some countries adopt a higher cutoff age for the definition of childhood obesity (46). As a result, we had to exclude some articles because they did not meet our inclusion criteria for the cutoff age. Second, each of the 54 selected studies used different methodological approaches in selecting study population, study setting, and survey methods (national survey, local community schools). Thus, it was not possible to perform a meta-analysis; instead, we provided median prevalence for predefined subgroups. Nevertheless, it was possible to draw several useful trends from the complex datasets of heterogeneous studies. Third, the majority of studies on LMICs seemed to have assessed hypertension more frequently than other NCDs, perhaps due to resource limitations. Consequently, this may have biased the true picture of the distribution of childhood obesity-related NCD in LMICs. Research using advanced screening in LMICs is therefore needed to provide a comprehensive picture of childhood obesity comorbidities burden.
Childhood obesity, with its associated NCD comorbidities, is a major global public health problem. Globally, there is a disparity in the prevalence of childhood obesity-related hypertension, metabolic syndrome, dyslipidemia, and NAFLD comorbidities, between HICs and LMICs, different global regions, and genders. Socioeconomic factors seem to be the main determinant for disparity between LMICs and HICs, besides biological, environmental, cultural, and modifiable lifestyle differences. Implementing targeted lifestyle interventions that are context specific to the socioeconomic realities of the population and informed by local evidence is required. Furthermore, strengthening research and surveillance methods for childhood obesity-related NCD to improve local policies and appropriate interventions is needed.
The original contributions presented in the study are included in the article/Supplementary Material, further inquiries can be directed to the corresponding author.
GO and AA contributed to conception, design of the study, performed article screening, and quality assessment. GO organized literature search and wrote the first draft of the manuscript. AA critically revised and edited the manuscript. Both authors reviewed and approved the finally submitted version.
The authors declare that the research was conducted in the absence of any commercial or financial relationships that could be construed as a potential conflict of interest.
All claims expressed in this article are solely those of the authors and do not necessarily represent those of their affiliated organizations, or those of the publisher, the editors and the reviewers. Any product that may be evaluated in this article, or claim that may be made by its manufacturer, is not guaranteed or endorsed by the publisher.
The research team would like to thank Julie Hogg, Assistant Academic Librarian at Teesside University, for her significant contribution to the development of the search strategy.
The Supplementary Material for this article can be found online at: https://www.frontiersin.org/articles/10.3389/fpubh.2022.923744/full#supplementary-material
1. Proimos J, Klein JD. Noncommunicable diseases in children and adolescents. Pediatrics. (2012) 130:379–81. doi: 10.1542/peds.2012-1475
2. Guariguata L, Jeyaseelan S. Children Non-Communicable Diseases: Global Burden Report. NCD Child (2019). Available online at: http://www.ncdchild.org/media/1603/ncdchild_global_burden-report-2019.pdf (accessed December 15, 2021).
3. WHO. Obesity and Overweight. World Health Organization (2018). Available online at: https://www.who.int/news-room/fact-sheets/detail/obesity-and-overweight (accessed December 20, 2021).
4. Swinburn BA, Caterson I, Seidell JC, James WP. Diet, nutrition and the prevention of excess weight gain and obesity. Public Health Nutr. (2004) 7:123–46. doi: 10.1079/PHN2003585
5. WHO. World Health Organization New Air Pollution Data. World Health Organization (2018). Available online at: http://www.ccacoalition.org/en/news/world-health-organization-releases-new-global-air-pollution-data (accessed December 28, 2021).
6. Seidell JC. Societal and personal costs of obesity. Exp Clin Endocrinol Diabetes. (1998) 106:7–10. doi: 10.1055/s-0029-1212029
7. Galuska DA, Dietz WH. Obesity and Overweight (ICD-10 E66). Chronic Disease Epidemiology and Control 3rd Ed. (2010). p. 269–90.
9. Bray G, Kim K, Wilding J, Federation WO. Obesity: a chronic relapsing progressive disease process. a position statement of the world obesity federation. Obes Rev. (2017) 18:715–23. doi: 10.1111/obr.12551
10. Yancik R, Ershler W, Satariano W, Hazzard W, Cohen HJ, Ferrucci L. Report of the national institute on aging task force on comorbidity. J Gerontol A Biol Sci Med Sci. (2007) 62:275–80. doi: 10.1093/gerona/62.3.275
11. Mercer SW, Smith SM, Wyke S, O'Dowd T, Watt GC. Multimorbidity in primary care: developing the research agenda. Fam Pract. (2009) 26:79–80. doi: 10.1093/fampra/cmp020
12. Uijen AA, van de Lisdonk EH. Multimorbidity in primary care: prevalence and trend over the last 20 years. Eur J Gen Pract. (2008) 14:28–32. doi: 10.1080/13814780802436093
13. Fortin M, Hudon C, Haggerty J, Akker M, Almirall J. Prevalence estimates of multimorbidity: a comparative study of two sources. BMC Health Serv Res. (2010) 10:111. doi: 10.1186/1472-6963-10-111
14. Alkhatib A, Nnyanzi LA, Mujuni B, Amanya G, Ibingira C. Preventing Multimorbidity with Lifestyle Interventions in Sub-Saharan Africa: a new challenge for public health in low and middle-income countries. Int J Environ Res Public Health. (2021) 18:12449. doi: 10.3390/ijerph182312449
15. Fried LP, Ferrucci L, Darer J, Williamson JD, Anderson G. Untangling the concepts of disability, frailty, and comorbidity: implications for improved targeting and care. J Gerontol A Biol Sci Med Sci. (2004) 59:255–63. doi: 10.1093/gerona/59.3.M255
16. Güngör NK. Overweight and obesity in children and adolescents. J Clin Res Pediatr Endocrinol. (2014) 6:129–43. doi: 10.4274/jcrpe.1471
17. Gungor N, Bacha F, Saad R, Janosky J, Arslanian S. Youth type 2 diabetes: insulin resistance, beta-cell failure, or both? Diabetes Care. (2005) 28:638–44. doi: 10.2337/diacare.28.3.638
18. Kahn SE. Clinical review 135: the importance of beta-cell failure in the development and progression of type 2 diabetes. J Clin Endocrinol Metab. (2001) 86:4047–58. doi: 10.1210/jc.86.9.4047
19. Gungor N, Libman I, Arslanian S. Type 2 diabetes mellitus in children and adolescents. In: Pescovitz and Eugster, editors. Pediatric Endocrinology: Mechanisms, Manifestations and Management. Lippincott, Williams and Wilkins (2004).
20. Lee L, Sanders RA. Metabolic syndrome. Pediatr Review. (2012) 33:459. doi: 10.1542/pir.33.10.459
21. Schwimmer JB, Deutsch R, Rauch JB, Behling C, Newbury R, Lavine JE. Obesity, insulin resistance, and other clinicopathological correlates of pediatric nonalcoholic fatty liver disease. J Pediatr. (2003) 143:500–5. doi: 10.1067/S0022-3476(03)00325-1
22. Khaodhiar L, McCowen KC, Blackburn GL. Obesity and its comorbid conditions. Clin Cornerstone. (1999) 2:17–31. doi: 10.1016/S1098-3597(99)90002-9
23. Sharma V, Coleman S, Nixon J, Sharples L, Hamilton-Shield J, Rutter H, et al. A systematic review and meta-analysis estimating the population prevalence of comorbidities in children and adolescents aged 5 to 18 years. Obes Rev. (2019) 20:1341–9. doi: 10.1111/obr.12904
24. Pantalone KM, Hobbs TM, Chagin KM, Kong SX, Wells BJ, Kattan MW, et al. Prevalence and recognition of obesity and its associated comorbidities: cross-sectional analysis of electronic health record data from a large US integrated health system. BMJ Open. (2017) 7:e017583. doi: 10.1136/bmjopen-2017-017583
25. Srinivasan SR, Myers L, Berenson GS. Predictability of childhood adiposity and insulin for developing insulin resistance syndrome (syndrome X) in young adulthood: the Bogalusa heart study. Diabetes. (2002) 51:204–9. doi: 10.2337/diabetes.51.1.204
26. Field AE, Byers T, Hunter DJ, Laird NM, Manson JE, Williamson DF, et al. Weight cycling, weight gain, and risk of hypertension in women. Am J Epidemiol. (1999) 150:573–9. doi: 10.1093/oxfordjournals.aje.a010055
27. Dyer AR, Elliott P. The INTERSALT study: relations of body mass index to blood pressure. INTERSALT Co-operative research group. J Hum Hypertens. (1989) 3:299–308.
28. Li S, Chen W, Srinivasan SR, Xu J, Berenson GS. Relation of childhood obesity/cardiometabolic phenotypes to adult cardiometabolic profile: the Bogalusa heart study. Am J Epidemiol. (2012) 176:S142–9. doi: 10.1093/aje/kws236
29. Srinivasan SR, Bao W, Wattigney WA, Berenson GS. Adolescent overweight is associated with adult overweight and related multiple cardiovascular risk factors: the Bogalusa heart study. Metabolism. (1996) 45:235–40. doi: 10.1016/S0026-0495(96)90060-8
30. Zilanawala A, Davis-Kean P, Nazroo J, Sacker A, Simonton S, Kelly Y. Race/ethnic disparities in early childhood BMI, obesity and overweight in the United Kingdom and United States. Int J Obes. (2015) 39:520–9. doi: 10.1038/ijo.2014.171
31. Falconer CL, Park MH, Croker H, Kessel AS, Saxena S, Viner RM, et al. Can the relationship between ethnicity and obesity-related behaviours among school-aged children be explained by deprivation? a cross-sectional study. BMJ Open. (2014) 4:e003949. doi: 10.1136/bmjopen-2013-003949
32. Mayor S. Socioeconomic disadvantage is linked to obesity across generations, UK study finds. BMJ. (2017) 356:j163. doi: 10.1136/bmj.j163
33. Bann D, Johnson W, Li L, Kuh D, Hardy R. Socioeconomic inequalities in body mass index across adulthood: coordinated analyses of individual participant data from three British birth cohort studies initiated in 1946, 1958 and 1970. PLoS Med. (2017) 14:e1002214. doi: 10.1371/journal.pmed.1002214
34. Weaver RG, Brazendale K, Hunt E, Sarzynski MA, Beets MW, White K. Disparities in childhood overweight and obesity by income in the United States: an epidemiological examination using three nationally representative datasets. Int J Obes (Lond). (2019) 43:1210–22. doi: 10.1038/s41366-019-0331-2
35. Chandalia M, Lin P, Seenivasan T, Livingston EH, Snell PG, Grundy SM, et al. Insulin resistance and body fat distribution in South Asian men compared to Caucasian men. PLoS ONE. (2007) 2:e812-e. doi: 10.1371/journal.pone.0000812
36. Zhang Y-x, Wang S-r. Blood pressure level profiles among children and adolescents with various types of obesity: study in a large population in Shandong, China. Clin Res Cardiol. (2014) 103:553–9. doi: 10.1007/s00392-014-0683-5
37. Zhou X, Hou D-q, Duan J-l, Sun Y, Cheng H, Zhao X-y, et al. [Prevalence of nonalcoholic fatty liver disease and metabolic abnormalities in 387 obese children and adolescents in Beijing, China]. Zhonghua liu xing bing xue za zhi. (2013) 34:446–50.
38. Andes LJ, Cheng YJ, Rolka DB, Gregg EW, Imperatore G. Prevalence of prediabetes among adolescents and young adults in the united States, 2005-2016. JAMA Pediatr. (2020) 174:e194498. doi: 10.1001/jamapediatrics.2019.4498
39. Cheung EL, Bell CS, Samuel JP, Poffenbarger T, Redwine KM, Samuels JA. Race and obesity in adolescent hypertension. Pediatrics. (2017) 139:e20161433. doi: 10.1542/peds.2016-1433
40. Ioakeim-Skoufa I, Poblador-Plou B, Carmona-Pírez J, Díez-Manglano J, Navickas R, Gimeno-Feliu LA, et al. Multimorbidity patterns in the general population: results from the epichron cohort study. Int J Environ Res Public Health. (2020) 17:4242. doi: 10.3390/ijerph17124242
41. Smith SM, Wallace E, Clyne B, Boland F, Fortin M. Interventions for improving outcomes in patients with multimorbidity in primary care and community setting: a systematic review. Syst Rev. (2021) 10:1–23. doi: 10.1186/s13643-021-01817-z
42. Garin N, Koyanagi A, Chatterji S, Tyrovolas S, Olaya B, Leonardi M, et al. Global multimorbidity patterns: a cross-sectional, population-based, multi-country study. J Gerontol A Biomed Sci Med Sci. (2016) 71:205–14. doi: 10.1093/gerona/glv128
43. WHO. Childhood Overweight and Obesity. World Health Organization (2018). Available from: https://www.who.int/dietphysicalactivity/childhood/en/ (accessed January 15, 2022).
44. Bauman A, Craig CL. The place of physical activity in the WHO global strategy on diet and physical activity. Int J Behav Nutr Phys Act. (2005) 2:1–6. doi: 10.1186/1479-5868-2-10
45. Liberati A, Altman DG, Tetzlaff J, Mulrow C, Gøtzsche PC, Ioannidis JPA, et al. The PRISMA statement for reporting systematic reviews and meta-analyses of studies that evaluate health care interventions: explanation and elaboration. Ital J Public Health. (2009) 6:354–91. doi: 10.1371/journal.pmed.1000100
46. Rolland-Cachera MF, Group FTECO. Childhood obesity: current definitions and recommendations for their use. Int J Pediatr Obes. (2011) 6:325–31. doi: 10.3109/17477166.2011.607458
47. Moher D, Liberati A, Tetzlaff J, Altman DG, The PG. Preferred reporting items for systematic reviews and meta-analyses: the PRISMA statement. PLoS Med. (2009) 6:e1000097. doi: 10.1371/journal.pmed.1000097
48. Lizarondo L, Stern C, Carrier J, Godfrey C, Rieger K, Salmond S, et al. Chapter 8: Mixed Methods Systematic Reviews. The Joanna Briggs Institute (2017). Available online at: https://reviewersmanual.joannabriggs.org/ (accessed January 20, 2022).
49. Munn Z, Moola S, Riitano D, Lisy K. The development of a critical appraisal tool for use in systematic reviews addressing questions of prevalence. Int J Health Policy Manag. (2014) 3:123–8. doi: 10.15171/ijhpm.2014.71
50. UN. Standard Country or Area Codes for Statistical Use. United Nations, Statistics Division (1999). Available online at: https://unstats.un.org/unsd/methodology/m49/ (accessed January 20, 2022).
51. Fantom NJ, Serajuddin U. The World Bank's Classification of Countries by Income. World Bank Policy Research Working Paper. (2016). doi: 10.1596/1813-9450-7528
52. Manikandan S. Measures of central tendency: Median and mode. J Pharmacol Pharmacother. (2011) 2:214–5. doi: 10.4103/0976-500X.83300
53. Önsüz FM, Demir F. Prevalence of hypertension and its association with obesity among school children aged 6-15 living in Sakarya Province in Turkey. Turk J Med Sci. (2015) 45:907–12. doi: 10.3906/sag-1407-120
54. Xu H, Li Y, Liu A, Zhang Q, Hu X, Fang H, et al. Prevalence of the metabolic syndrome among children from six cities of China. BMC Public Health. (2012) 12:13. doi: 10.1186/1471-2458-12-13
55. CMA. [Prevalence of metabolic syndrome of children and adolescent students in Chinese six cities]. Zhonghua er ke za zhi. (2013) 51:409–13.
56. Badeli H, Hassankhani A, Naeemi Z, Hosseinzadeh S, Mehrabi S, Pourkarimi M, et al. Prevalence of hypertension and obesity-related hypertension in urban school-aged children in Rasht. Iran J Kidney Dis. (2016) 10:364–8.
57. Basiratnia M, Derakhshan D, Ajdari S, Saki F. Prevalence of childhood obesity and hypertension in south of Iran. Iran J Kidney Dis. (2013) 7:282–9.
58. Cheng H, Yan Y-k, Duan J-l, Er Y-l, Mi J. [Association between obesity in childhood and hypertension incidence: a prospective cohort study] Zhonghua yu fang yi xue za zhi. (2011) 45:696–701.
59. Cheng X, Wang H, Yuan B, Guan P, Wang L. [Prevalence of metabolic syndrome and its family factors for children and adolescents in Chongqing City in 2014]. Wei Sheng Yan Jiu. (2017) 46:557–62.
60. Dong J, Guo X-L, Lu Z-L, Cai X-N, Wang H-C, Zhang J-Y, et al. Prevalence of overweight and obesity and their associations with blood pressure among children and adolescents in Shandong, China. BMC Public Health. (2014) 14:1080. doi: 10.1186/1471-2458-14-1080
61. Esposito M, Gallai B, Roccella M, Marotta R, Lavano F, Lavano SM, et al. Anxiety and depression levels in prepubertal obese children: a case-control study. Neuropsychiatr Dis Treat. (2014) 10:1897–902. doi: 10.2147/NDT.S69795
62. Genovesi S, Antolini L, Giussani M, Brambilla P, Barbieri V, Galbiati S, et al. Hypertension, prehypertension, and transient elevated blood pressure in children: association with weight excess and waist circumference. Am J Hypertens. (2010) 23:756–61. doi: 10.1038/ajh.2010.50
63. Jmal L, Jmal A, Abdennebi M, Feki M, Boukthir S. Prevalence of metabolic syndrome in Tunisian overweight and obese children. Tunis Med. (2019) 97:133–9.
64. Koebnick C, Black MH, Wu J, Martinez MP, Smith N, Kuizon B, et al. High blood pressure in overweight and obese youth: implications for screening. J Clin Hypertens. (2013) 15:793–805. doi: 10.1111/jch.12199
65. Luo J-S, Chen S-K, Fan X, Tang Q, Feng Y. [Prevalence of hypertension and relationship between hypertension and obesity in children and adolescents in Nanning of Guangxi Province]. Zhongguo Dang Dai Er Ke Za Zhi. (2014) 16:1040–4.
66. Manios Y, Androutsos O, Lambrinou C-P, Cardon G, Lindstrom J, Annemans L, et al. A school- and community-based intervention to promote healthy lifestyle and prevent type 2 diabetes in vulnerable families across Europe: design and implementation of the Feel4Diabetes-study. Public Health Nutr. (2018) 21:3281–90. doi: 10.1017/S1368980018002136
67. Nkeh-Chungag BN, Sekokotla AM, Sewani-Rusike C, Namugowa A, Iputo JE. Prevalence of hypertension and pre-hypertension in 13-17 year old adolescents living in Mthatha - South Africa: a cross-sectional study. Cent Eur J Public Health. (2015) 23:59–64. doi: 10.21101/cejph.a3922
68. Pećin I, Samovojska R, Heinrich B, Zeljković-Vrkić T, Laganović M, Jelaković B. Hypertension, overweight and obesity in adolescents: the CRO-KOP study. Coll Antropol. (2013) 37:761–4.
69. Pontiles de Sánchez M, Morón de Salim A, Rodríguez de Perdomo H, Perdomo Oramas G. [Prevalence of no alcohol fatty liver disease (NAFLD) in a population of obese children in Valencia, Venezuela]. Arch Latinoam Nutr. (2014) 64:73–82.
70. Rakočević L, Rakočević V. Incidence of cardiovascular risk factors in obese children. Acta Clin Croat. (2016) 55:407–13. doi: 10.20471/acc.2016.55.03.09
71. Sangun Ö, Dündar B, Köşker M, Pirgon Ö, Dündar N. Prevalence of metabolic syndrome in obese children and adolescents using three different criteria and evaluation of risk factors. J Clin Res Pediatr Endocrinol. (2011) 3:70–6. doi: 10.4274/jcrpe.v3i2.15
72. Saury-Paredes LA. [Prevalence of high blood pressure and their association with body mass index in children between 5 and 11 years of Nahbalam, Yucatan]. Gac Med Mex. (2016) 152:640–4.
73. !!! INVALID CITATION !!!{Badeli 2016 #6206; Genovesi 2010 #1720; Shirasawa 2010 #9819; Zhang 2014 #7876}.
74. Steinthorsdottir SD, Eliasdottir SB, Indridason OS, Agustsdottir IM, Palsson R, Edvardsson VO. Prevalence of hypertension in 9- to 10-year-old Icelandic school children. J Clin Hypertens. (2011) 13:774–9. doi: 10.1111/j.1751-7176.2011.00496.x
75. Suarez-Ortegón MF, Aguilar-de Plata C. Prevalence of metabolic syndrome in children aged 5-9 years from southwest colombia: a cross-sectional study. World J Pediatr. (2016) 12:477–83. doi: 10.1007/s12519-016-0008-z
76. Suazo J, Hodgson MI, Obregón AM, Valladares M, Weisstaub G, Amador P, et al. Prevalence of metabolic syndrome in obese Chilean children and association with gene variants of the leptin-melanocortin system. J Pediatr Endocrinol Metab. (2013) 26:1131–9. doi: 10.1515/jpem-2013-0084
77. Zhang Y-x, Zhao J-s, Chu Z-h, Zhou J-y. Prevalence of elevated blood pressure is associated with the increasing prevalence of obesity among children and adolescents in Shandong, China. Int J Cardiol. (2015) 201:150–1. doi: 10.1016/j.ijcard.2015.07.105
78. Zhang Y-X, Wang S-R. Monitoring of blood pressure in overweight and obese children in Shandong, China. Ann Hum Biol. (2011) 38:603–7. doi: 10.3109/03014460.2011.582884
79. Zhang Y-X, Wang S-R. Comparison of blood pressure levels among children and adolescents with different body mass index and waist circumference: study in a large sample in Shandong, China. Eur J Nutr. (2014) 53:627–34. doi: 10.1007/s00394-013-0571-1
80. Rerksuppaphol S, Rerksuppaphol L. Association of obesity with the prevalence of hypertension in school children from central Thailand. J Res Health Sci. (2015) 15:17–21.
81. Minghelli B, Oliveira R, Nunes C. Association of obesity with chronic disease and musculoskeletal factors. Rev Assoc Med Bras (1992). (2015) 61:347–54. doi: 10.1590/1806-9282.61.04.347
82. Elmaogullari S, Tepe D, Uçaktürk SA, Karaca Kara F, Demirel F. Prevalence of dyslipidemia and associated factors in obese children and adolescents. J Clin Res Pediatr Endocrinol. (2015) 7:228–34. doi: 10.4274/jcrpe.1867
83. Sukhonthachit P, Aekplakorn W, Hudthagosol C, Sirikulchayanonta C. The association between obesity and blood pressure in Thai public school children. BMC Public Health. (2014) 14:729. doi: 10.1186/1471-2458-14-729
84. Lim H, Xue H, Wang Y. Association between obesity and metabolic co-morbidities among children and adolescents in South Korea based on national data. BMC Public Health. (2014) 14:279. doi: 10.1186/1471-2458-14-279
85. Elizondo-Montemayor L, Ugalde-Casas PA, Lam-Franco L, Bustamante-Careaga H, Serrano-González M, Gutiérrez NG, et al. Association of ALT and the metabolic syndrome among Mexican children. Obes Res Clin Pract. (2014) 8:e79–87. doi: 10.1016/j.orcp.2012.08.191
86. Dyson PA, Anthony D, Fenton B, Matthews DR, Stevens DE. High rates of child hypertension associated with obesity: a community survey in China, India and Mexico. Paediatr Int Child Health. (2014) 34:43–9. doi: 10.1179/2046905513Y.0000000079
87. Wang Q, Yin J, Xu L, Cheng H, Zhao X, Xiang H, et al. Prevalence of metabolic syndrome in a cohort of Chinese schoolchildren: comparison of two definitions and assessment of adipokines as components by factor analysis. BMC Public Health. (2013) 13:249. doi: 10.1186/1471-2458-13-249
88. Mehairi AE, Khouri AA, Naqbi MM, Muhairi SJ, Maskari FA, Nagelkerke N, et al. Metabolic syndrome among Emirati adolescents: a school-based study. PLoS ONE. (2013) 8:e56159. doi: 10.1371/journal.pone.0056159
89. Gong CD, Wu QL, Chen Z, Zhang D, Zhao ZY, Peng YM. Glycolipid metabolic status of overweight/obese adolescents aged 9- to 15-year-old and the BMI-SDS/BMI cut-off value of predicting dyslipidemiain boys, Shanghai, China: a cross-sectional study. Lipids Health Dis. (2013) 12:129. doi: 10.1186/1476-511X-12-129
90. Zakeri M, Sedaghat M, Motlagh ME, Tayari Ashtiani R, Ardalan G. BMI correlation with psychiatric problems among 10-18 years Iranian students. Acta Med Iran. (2012) 50:177–84.
91. Papoutsakis C, Yannakoulia M, Ntalla I, Dedoussis GV. Metabolic syndrome in a Mediterranean pediatric cohort: prevalence using International Diabetes Federation-derived criteria and associations with adiponectin and leptin. Metabolism. (2012) 61:140–5. doi: 10.1016/j.metabol.2011.06.006
92. Chen F, Wang Y, Shan X, Cheng H, Hou D, Zhao X, et al. Association between childhood obesity and metabolic syndrome: evidence from a large sample of Chinese children and adolescents. PLoS ONE. (2012) 7:e47380. doi: 10.1371/journal.pone.0047380
93. Wiegand S, Keller KM, Röbl M, L'Allemand D, Reinehr T, Widhalm K, et al. Obese boys at increased risk for nonalcoholic liver disease: evaluation of 16,390 overweight or obese children and adolescents. Int J Obes. (2010) 34:1468–74. doi: 10.1038/ijo.2010.106
94. Rafraf M, Gargari BP, Safaiyan A. Prevalence of prehypertension and hypertension among adolescent high school girls in Tabriz, Iran. Food Nutr Bull. (2010) 31:461–5. doi: 10.1177/156482651003100308
95. Noonan CW, Brown BD, Bentley B, Conway K, Corcoran M, FourStar K, et al. Variability in childhood asthma and body mass index across Northern Plains American Indian communities. J Asthma. (2010) 47:496–500. doi: 10.3109/02770901003759436
96. Kloppenborg JT, Fonvig CE, Nielsen TRH, Mollerup PM, Bøjsøe C, Pedersen O, et al. Impaired fasting glucose and the metabolic profile in Danish children and adolescents with normal weight, overweight, or obesity. Pediatr Diabetes. (2018) 19:356–65. doi: 10.1111/pedi.12604
97. Di Bonito P, Miraglia Del Giudice E, Chiesa C, Licenziati MR, Manco M, Franco F, et al. Preclinical signs of liver and cardiac damage in youth with metabolically healthy obese phenotype. Nutr Metab Cardiovasc Dis. (2018) 28:1230–6. doi: 10.1016/j.numecd.2018.08.007
98. Sadeghi-Demneh E, Azadinia F, Jafarian F, Shamsi F, Melvin JM, Jafarpishe M, et al. Flatfoot and obesity in school-age children: a cross-sectional study. Clin Obes. (2016) 6:42–50. doi: 10.1111/cob.12125
99. Schwandt P, Scholze JE, Bertsch T, Liepold E, Haas GM. Blood pressure percentiles in 22,051 German children and adolescents: the PEP family heart study. Am J Hypertens. (2015) 28:672–9. doi: 10.1093/ajh/hpu208
100. Kajbaf TZ, Asar S, Alipoor MR. Relationship between obesity and asthma symptoms among children in Ahvaz, Iran: a cross sectional study. Ital J Pediatr. (2011) 37:1. doi: 10.1186/1824-7288-37-1
101. Bell LM, Curran JA, Byrne S, Roby H, Suriano K, Jones TW, et al. High incidence of obesity co-morbidities in young children: a cross-sectional study. J Paediatr Child Health. (2011) 47:911–7. doi: 10.1111/j.1440-1754.2011.02102.x
102. Mameli C, Krakauer NY, Krakauer JC, Bosetti A, Ferrari CM, Moiana N, et al. The association between a body shape index and cardiovascular risk in overweight and obese children and adolescents. PLoS ONE. (2018) 13:e0190426. doi: 10.1371/journal.pone.0190426
103. Leon G, de Klerk E, Ho J, Jackman M, Reimer RA, Connors KE, et al. Prevalence of comorbid conditions pre-existing and diagnosed at a tertiary care pediatric weight management clinic. J Pediatr Endocrinol Metab. (2018) 31:385–90. doi: 10.1515/jpem-2016-0245
104. Guijarro de, Armas MAG, Monereo Megías S, Merino Viveros M, Iglesias Bolaños P, Vega Piñero B. [Prevalence of metabolic syndrome in a population of obese children and adolescents] Endocrinol Nutr. (2012) 59:155–9. doi: 10.1016/j.endonu.2012.01.003
105. Çam HH, Ustuner Top F. Prevalence of hypertension and its association with body mass index and waist circumference among adolescents in Turkey: a cross-sectional study. J Pediatr Nurs. (2021) 57:e29–33. doi: 10.1016/j.pedn.2020.09.017
106. Lu X, Shi P, Luo C-Y, Zhou Y-F, Yu H-T, Guo C-Y, et al. Prevalence of hypertension in overweight and obese children from a large school-based population in Shanghai, China. BMC Public Health. (2013) 13:24. doi: 10.1186/1471-2458-13-24
107. Duzova A, Yalçinkaya F, Baskin E, Bakkaloglu A, Soylemezoglu O. Prevalence of hypertension and decreased glomerular filtration rate in obese children: results of a population-based field study. Nephrol Dial Transplant. (2013) 28:iv166–71. doi: 10.1093/ndt/gft317
108. Sapunar J, Aguilar-Farías N, Navarro J, Araneda G, Chandía-Poblete D, Manríquez V, et al. [High prevalence of dyslipidemia and high atherogenic index of plasma in children and adolescents]. Rev Med Chil. (2018) 146:1112–22. doi: 10.4067/S0034-98872018001001112
109. Zhang Y-x, Wang S-r, Zhao J-s, Chu Z-h. Blood pressure level profiles among obese children and adolescents with different stature. Int J Cardiol. (2015) 182:6–7. doi: 10.1016/j.ijcard.2014.12.107
110. Erdal I, Yalçin SS, Aksan A, Gençal D, Kanbur N. How useful are anthropometric measurements as predictive markers for elevated blood pressure in adolescents in different gender? J Pediatric Endocrinol Metab. (2020) 33:1203–11. doi: 10.1515/jpem-2020-0175
111. Shirasawa T, Ochiai H, Ohtsu T, Nishimura R, Morimoto A, Hoshino H, et al. LDL-cholesterol and body mass index among Japanese schoolchildren: a population-based cross-sectional study. Lipids Health Dis. (2013) 12:77. doi: 10.1186/1476-511X-12-77
112. Hosseinpoor AR, Bergen N, Kunst A, Harper S, Guthold R, Rekve D, et al. Socioeconomic inequalities in risk factors for non communicable diseases in low-income and middle-income countries: results from the world health survey. BMC Public Health. (2012) 12:912. doi: 10.1186/1471-2458-12-912
113. Sommer I, Griebler U, Mahlknecht P, Thaler K, Bouskill K, Gartlehner G, et al. Socioeconomic inequalities in non-communicable diseases and their risk factors: an overview of systematic reviews. BMC Public Health. (2015) 15:914. doi: 10.1186/s12889-015-2227-y
114. Sposato LA, Saposnik G. Gross domestic product and health expenditure associated with incidence, 30-day fatality, and age at stroke onset. Stroke. (2012) 43:170–7. doi: 10.1161/STROKEAHA.111.632158
115. Ramachandran A, Snehalatha C, Kapur A, Vijay V, Mohan V, Das AK, et al. High prevalence of diabetes and impaired glucose tolerance in India: national urban diabetes survey. Diabetologia. (2001) 44:1094–101. doi: 10.1007/s001250100627
116. Curtis LJ. An economic perspective on the causal explanations for the socioeconomic inequalities in health. Rev Panam Salud Publica. (2018) 42:e53-e. doi: 10.26633/RPSP.2018.53
117. Hidayat K, Du X, Shi B-M, Qin L-Q. Foetal and childhood exposure to famine and the risks of cardiometabolic conditions in adulthood: a systematic review and meta-analysis of observational studies. Obes Rev. (2020) 21:e12981. doi: 10.1111/obr.12981
118. Blakely T, Hales S, Kieft C, Wilso.n N, Woodward A. Distribution of risk factors by poverty. Comparative quantification of health risks: Global and regional burden of disease attributable to selected major risk factors. Geneva: World Health Organization (2004). p. 1941–2127.
119. Marins VM, Almeida RM, Pereira RA, Sichieri R. The association between socioeconomic indicators and cardiovascular disease risk factors in Rio de Janeiro, Brazil. J Biosoc Sci. (2007) 39:221–9. doi: 10.1017/S0021932006001246
120. Tunstall-Pedoe H. Preventing Chronic Diseases. A Vital Investment: WHO Global Report. Geneva: World Health Organization, Oxford University Press 2006. (2005). p. 200. CHF 30.00. ISBN 92 4 1563001. Available online at: http://www.who.int/chp/chronic_disease_report/en
121. Seabrook JA, Avison WR. Socioeconomic status and cumulative disadvantage processes across the life course: implications for health outcomes. Can Rev Sociol. (2012) 49:50–68. doi: 10.1111/j.1755-618X.2011.01280.x
122. Alkhatib A. Personalising exercise and nutrition behaviours in diabetes lifestyle prevention. Eur Med J. (2020) 5:67–77. doi: 10.33590/emj/19-00139
123. Alkhatib A, Tsang C, Tiss A, Bahorun T, Arefanian H, Barake R, et al. Functional foods and lifestyle approaches for diabetes prevention and management. Nutrients. (2017) 9:1310. doi: 10.3390/nu9121310
124. Islam SMS, Purnat TD, Phuong NTA, Mwingira U, Schacht K, Fröschl G. Non-communicable diseases (NCDs) in developing countries: a symposium report. Global Health. (2014) 10:81. doi: 10.1186/s12992-014-0081-9
125. Goryakin Y, Rocco L, Suhrcke M. The contribution of urbanization to non-communicable diseases: Evidence from 173 countries from 1980 to 2008. Econ Hum Biol. (2017) 26:151–63. doi: 10.1016/j.ehb.2017.03.004
126. Allender S, Wickramasinghe K, Goldacre M, Matthews D, Katulanda P. Quantifying urbanization as a risk factor for noncommunicable disease. J Urban Health. (2011) 88:906. doi: 10.1007/s11524-011-9586-1
127. Yarahmadi S, Etemad K, Hazaveh AM, Azhang N. Urbanization and non-communicable risk factors in the capital city of 6 big provinces of Iran. Iran J Public Health. (2013) 42:113.
128. Juma K, Juma PA, Mohamed SF, Owuor J, Wanyoike A, Mulabi D, et al. First Africa non-communicable disease research conference 2017: sharing evidence and identifying research priorities. J Glob Health. (2019) 8:020301. doi: 10.7189/jogh.09.010201
129. Khorrami Z, Etemad K, Yarahmadi S, Khodakarim S, Kameli M, Hezaveh AM, et al. Urbanization and noncommunicable disease (NCD) risk factors: WHO STEPwise Iranian NCD risk factors surveillance in 2011. East Mediterr Health J. (2017) 23:469. doi: 10.26719/2017.23.7.469
130. Bickler SW, Wang A, Amin S, Halbach J, Lizardo R, Cauvi DM, et al. Urbanization in sub-Saharan Africa: declining rates of chronic and recurrent infection and their possible role in the origins of non-communicable diseases. World J Surg. (2018) 42:1617–28. doi: 10.1007/s00268-017-4389-5
131. Misra A, Khurana L, Isharwal S, Bhardwaj S. South Asian diets and insulin resistance. Br J Nutr. (2009) 101:465–73. doi: 10.1017/S0007114508073649
132. Global, regional, and national comparative risk assessment of 84 behavioural, environmental and occupational, and metabolic risks or clusters of risks for 195 countries and territories, 1990-2017: 1990-2017: a systematic analysis for the global burden of disease study 2017. Lancet. (2018) 392:1923–94. doi: 10.1016/S0140-6736(18)32225-6
133. Pullar J, Wickramasinghe K, Demaio AR, Roberts N, Perez-Blanco K-M, Noonan K, et al. The impact of maternal nutrition on offspring's risk of non-communicable diseases in adulthood: a systematic review. J Global Health. (2019) 9:020405. doi: 10.7189/jogh.09.020405
134. Fay M. The Urban Poor in Latin America. World Bank Publications (2005). doi: 10.1596/0-8213-6069-8
135. Mercado S, Havemann K, Sami M, Ueda H. Urban poverty: an urgent public health issue. J Urban Health. (2007) 84:7–15. doi: 10.1007/s11524-007-9191-5
136. Dhawan J, Bray CL, Warburton R, Ghambhir DS, Morris J. Insulin resistance, high prevalence of diabetes, and cardiovascular risk in immigrant Asians. genetic or environmental effect? Heart. (1994) 72:413–21. doi: 10.1136/hrt.72.5.413
137. Kain K, Catto A, Grant P. Associations between insulin resistance and thrombotic risk factors in high-risk South Asian subjects. Diabet Med. (2003) 20:651–5. doi: 10.1046/j.1464-5491.2003.00958.x
138. Misra A, Khurana L. Obesity-related non-communicable diseases: South Asians vs White Caucasians. Int J Obes. (2011) 35:167–87. doi: 10.1038/ijo.2010.135
139. Caprio S, Daniels SR, Drewnowski A, Kaufman FR, Palinkas LA, Rosenbloom AL, et al. Influence of race, ethnicity, and culture on childhood obesity: implications for prevention and treatment: a consensus statement of Shaping America's health and the obesity society. Diabetes Care. (2008) 31:2211–21. doi: 10.2337/dc08-9024
140. Fall CHD. Fetal programming and the risk of noncommunicable disease. Indian J Pediatr. (2013) 80:S13–20. doi: 10.1007/s12098-012-0834-5
141. Hutchinson RG, Watson RL, Davis CE, Barnes R, Brown S, Romm F, et al. Racial differences in risk factors for atherosclerosis: the ARIC study. Angiology. (1997) 48:279–90. doi: 10.1177/000331979704800401
142. Zhao D, Li Y, Zheng L, Yu K. Brief communication: body mass index, body adiposity index, and percent body fat in Asians. Am J Phys Anthropol. (2013) 152:294–9. doi: 10.1002/ajpa.22341
143. Wang J, Thornton JC, Russell M, Burastero S, Heymsfield S, Pierson Jr RN. Asians have lower body mass index (BMI) but higher percent body fat than do whites: comparisons of anthropometric measurements. Am J Clin Nutr. (1994) 60:23–8. doi: 10.1093/ajcn/60.1.23
144. Misra A. Revisions of cutoffs of body mass index to define overweight and obesity are needed for the Asian-ethnic groups. Int J Obes. (2003) 27:1294–6. doi: 10.1038/sj.ijo.0802412
145. Janssen I, Katzmarzyk P, Boyce W, King M, Pickett W. Overweight and obesity in Canadian adolescents and their association with dietary habits and physical activity pattern. J Adolesc Health. (2004) 35:360–7. doi: 10.1016/S1054-139X(04)00058-8
146. Zhang J, Zhai Y, Feng XQ, Li WR, Lyu YB, Astell-Burt T, et al. Gender differences in the prevalence of overweight and obesity, associated behaviors, and weight-related perceptions in a national survey of primary school children in China. Biomed Environ Sci. (2018) 31:1–11. doi: 10.3967/bes2018.001
147. Baker C. Briefing Paper: Obesity Staistics. UK parliament (2019). Available online at: https://commonslibrary.parliament.uk/topic/social-policy/health/ (accessed February 20, 2022).
148. Shah B, Tombeau Cost K, Fuller A, Birken CS, Anderson LN. Sex and gender differences in childhood obesity: contributing to the research agenda. BMJ Nutr Prev Health. (2020) 3:387–90. doi: 10.1136/bmjnph-2020-000074
Keywords: childhood obesity, comorbidity, disparity, prevalence, non-communicable disease
Citation: Obita G and Alkhatib A (2022) Disparities in the Prevalence of Childhood Obesity-Related Comorbidities: A Systematic Review. Front. Public Health 10:923744. doi: 10.3389/fpubh.2022.923744
Received: 19 April 2022; Accepted: 01 June 2022;
Published: 06 July 2022.
Edited by:
KM Saif-Ur-Rahman, National University of Ireland Galway, IrelandReviewed by:
Shiny Talukder, Rangamati Medical College, BangladeshCopyright © 2022 Obita and Alkhatib. This is an open-access article distributed under the terms of the Creative Commons Attribution License (CC BY). The use, distribution or reproduction in other forums is permitted, provided the original author(s) and the copyright owner(s) are credited and that the original publication in this journal is cited, in accordance with accepted academic practice. No use, distribution or reproduction is permitted which does not comply with these terms.
*Correspondence: Ahmad Alkhatib, ZHJhaG1hZGFsa2hhdGliQGdtYWlsLmNvbQ==
Disclaimer: All claims expressed in this article are solely those of the authors and do not necessarily represent those of their affiliated organizations, or those of the publisher, the editors and the reviewers. Any product that may be evaluated in this article or claim that may be made by its manufacturer is not guaranteed or endorsed by the publisher.
Research integrity at Frontiers
Learn more about the work of our research integrity team to safeguard the quality of each article we publish.