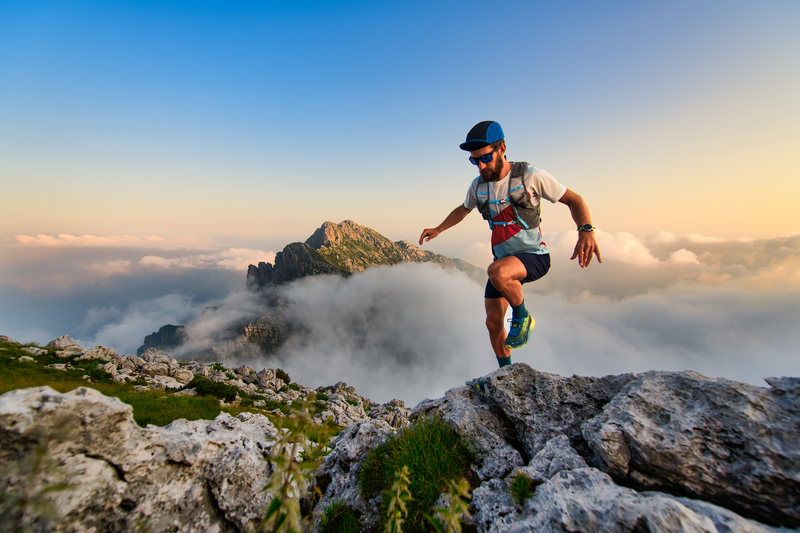
94% of researchers rate our articles as excellent or good
Learn more about the work of our research integrity team to safeguard the quality of each article we publish.
Find out more
ORIGINAL RESEARCH article
Front. Public Health , 08 September 2022
Sec. Occupational Health and Safety
Volume 10 - 2022 | https://doi.org/10.3389/fpubh.2022.907788
At present, the phenomenon of excessive pesticide residues in vegetables is prominent, causing widespread concern among all sectors of society. Excavate the influencing factors in the farmers themselves, government, market and society that affect vegetable farmers' green pesticide application behavior, clarify the influence mechanism of influencing factors on vegetable farmers' green pesticide application behavior. The study includes two parts: First, Grounded theory is used to construct a conceptual model that illustrates vegetable farmers' green pesticide application behavior. The second part applies the structural equation modeling to verify the research hypotheses, and reveals various factors in vegetable farmers' green pesticide application behavior (GB). The Results: Behavioral attitude (BA) and behavioral feedback perception (BP) are precursor variables that determine vegetable farmers' green pesticide application motivation (GM), and thus affect vegetable farmers' GB. Government supervision and regulation (GR), and market adjustment guidance (MG) are external factors that regulate the strength of the relationship between GM and GB. It is necessary to further strengthen the reference and normative role of society in vegetable farmers' GB, and provide a driving strategy for vegetable farmers' GB. Thus, it can better improve the quality of pesticide application and ensure vegetable safety.
At the present stage, China's economic and social development is in a complex environment of multi-center interdependence (1). The continuous increase in agricultural product demand has formed an inertial dependence on the use of pesticides and other chemicals (2). China has 7% of the world's arable land, however China's use of chemical fertilizers and pesticides amounts to 35% of the global total. The direct and indirect pollution caused by excessive pesticide residues of agricultural workers forms a vicious circle, attracting great attention and arousing concern from all sectors of society (3–5). Agricultural production is dominated by large quantities and small scales, and the characteristics of “farmer dispersion and discrete markets” are prominent. Thus, the government cannot fully monitor and control vegetable farmers' pesticide application behavior (6, 7). Excessive pesticide residues affect environmental pollution, vegetable safety and human health (8). In China, the types of pesticides application are complex and diverse. Pesticide types include procymidon, carbendazim, metalaxyl, difenoconazole, alachlor, propamocarb, dimethomorph, Pyraclostrobin, propiconazole and pyridaben, etc. Green pesticide application behavior refers to the application of these pesticides on time and at the proper dose, which can not only produce high targeted insecticidal and herbicidal effects, but also reduce their toxic side effects. Retail vegetable farmers constitute the main group using pesticides. Vegetable farmers often ignore the quality and safety of agricultural products in the production process, because they are driven by profit. They also tend to use highly toxic and violently toxic pesticides to seek economic income that is stable and as high as possible. This behavior has a decisive influence on the formation of pesticide residues, and the standard is even exceeded (9). We have investigated the pesticide residues in several vegetables, and the results are shown in Table 1. From Table 1, we can find that more than half of the pesticide residues in vegetables are not up to standard, and the problem of pesticide residues in vegetables is serious, which needs to be solved. At present, in China's agricultural development, the quality orientation of agricultural products is the most important issue, and reducing pesticide application is a key way to alleviate the serious vegetable safety problems and ecological environment deterioration risks facing agricultural development. To ensure the quality and safety of agricultural products, strictly controlling farmers' pesticide application has become the top priority (1, 4).
In this research, vegetable farmers' green pesticide application behavior needs to the goal of “low residue, low pollution, ensuring quality, and ensuring safety.” This goal includes taking the initiative to select approved pesticides, reducing pesticide application volume and frequency, applying pesticides at safe intervals, avoiding vegetable pesticide residues that exceed the standard, and ensuring the quality and safety of vegetable production activities. Accordingly, this study explores the internal and external factors in vegetable farmers' GB from a micro level, and it explores the influencing factors of vegetable farmers' GB through in-depth interviews. Based on the systematic collection of interview records, grounded theory is used to refine the factors of vegetable farmers' GB. Additionally, the structural equation modeling (SEM) is used for empirical verification to provide a scientific management and decision-making basis for constructing a driving strategy for green pesticide application for vegetable farmers.
In Silent Spring, Carson described the enormous damage to the human body and ecological environment caused by highly toxic pesticides. Since then, the harm caused by unreasonable pesticide application has gradually become a concern of scholars in China and in other countries (10), including scholars in economics, management, marketing, psychology, praxeology and other disciplines (11). Research on the government supervision system for agricultural worker has been conducted at macro disagreement (12), while research on effective methods of encouraging agricultural worker to standardize the application of pesticides and fertilizers has been conducted at the micro level. From the micro level, the research on agricultural workers' pesticide application behavior is mainly based on the school of organizational production represented by Chayanov, the rational small farming school represented by Schultz, and the classic farmer behavior theory of the historical school represented by Huang Zongzhi, The first school believes that the behavior of agricultural worker is not to pursue the maximization of market profits, but to pursue the balance between consumption satisfaction and labor hardship; The second school believes that behavior of agricultural worker is completely rational and makes behavioral decisions with the goal of pursuing maximum profit; The third school puts forward a relatively Commodity Smallholder Theory, and believes that agricultural worker are both profit-seeking and livelihood producers, their decision behavior is affected by both economic and non-economic factors (13). In addition, based on behavior motivation theory, the theory of planned behavior theory, producer behavior theory, game theory, and information asymmetry theory, scholars have focused on the purpose, difference, reality and economics of vegetable farmers' pesticide application behavior, but they have not reached a unified conclusion (14). A consensus has been reached with regard to the notion that the pesticide application behavior of vegetable farmers is a key factor in vegetable quality and safety. Therefore, controlling and regulating vegetable farmers' pesticide application behavior are an important condition of vegetable quality and safety (15).
This study explores the factors in vegetable farmers' pesticide application behavior. The influencing factors of farmers' pesticide application decisions include economic factors, social factors and the internal factors (12). The characteristics of farmers, such as their gender, age, farming experience, family characteristics and educational level, affect their safe pesticide application behavior (16). Risk perception, safe pesticide application awareness and safe pesticide application motivation have important effects on farmers' pesticide application behavior (17). The influencing factors of farmers' pesticide application behavior involve information sharing, information symmetry, agricultural insurance, pesticide residue detection and other factors (18). Farmers' pesticide application behavior is closely related to governmental attention (19). By considering the farmers application behavior, the government could have a more positive impact by strengthening legislation on and establish institutions for the quality and safety of agricultural products, and strictly supervising the behavior of agricultural producers (20, 21). The government can reduce the costs of supervision, increase agricultural subsidies, realize complete information sharing, increase farmers' additional income, reduce the costs of production inputs and take other measures to raise farmers' green pesticide application enthusiasm (22). Having a sound sales channel for high-quality agricultural products, supporting quality control standards and agricultural product quality traceability systems, and increasing consumer' purchases and willingness to pay are favorable conditions that will drive farmers to standardize their pesticide application (23). Under a situation of information asymmetry, it is difficult for consumers to distinguish the quality of agricultural products. Establishing market access systems and agricultural product quality identification systems can encourage farmers to produce high-quality agricultural products (24, 25).
Grounded theory, which is used to qualitatively analyze the information collected by researchers, was proposed by sociologist Barney Glaser et al. This method is characterized by strong theoretical tension and a strong ability to deduce exploratory theory (26). Grounded theory is a way of qualitative research. Its main purpose is to establish a theory based on empirical data. Researchers generally do not have theoretical assumptions before the start of research. They start with actual observation, summarize experience from the original data, and then rise to the systematic theory. It constructs a theoretical system through three links: open coding, spindle coding, and selective coding. In the process of data analysis, continuous comparative analysis is adopted until a new substantial theoretical framework is constructed (27). The specific process of Grounded theory is shown in Figure 1. In this study, the data collection process mainly involved three methods: face-to-face interviews, voice interviews and focus group interviews. The face-to-face interviews mainly consisted of meeting with farmers, effectively adjusting the content of the interviews in real time, and observing non-verbal behaviors. Each interview lasted approximately 30 min. The voice interviews involved using WeChat voice technology, and the interviewees were more willing to express their inner thoughts. Each interview lasted approximately 30 min. The focus group interviews were conducted in two groups of 5 people under the guidance, and each group met for 1 h. The face-to-face interviews, voice interviews and focus group interviews were adopted to comprehensively and systematically collect interview records, and to establish a theoretical model.
In the second part of this study, SEM was used to quantitatively research the conceptual model derived from the grounded theory. Structural equation model (SEM) is a statistical analysis method to identify, estimate and verify the complex theoretical hypothesis model. Structural equation methods is based on questionnaire survey, combined with regression analysis, factor analysis and path analysis to verify the complex theoretical hypothesis model, and can verify the influence relationship and influence effect between variables based on a large number of data. Based on the grounded theory in the previous step, prepare a questionnaire to obtain interview records and draw research hypotheses. Since the questionnaire items need to be related to the categories of the conceptual framework, the key issues of each category were highlighted and designed through reverse transcription. The questionnaire analysis consisted of two parts: the first part consisted of basic data statistics, the second part consisted of hypothesis testing. In the survey, the interviewees were asked to use a 5-point Likert scale anchored by 1 “strongly disagree” and 5 “strongly agree” to rate their level of agreement with particular items. A neutral item “uncertain” was included to prevent the respondents from providing biased results due to exact choices. Before starting the SEM analysis, IBM SPSS 22 was used to analyze the data. The SEM and IBM SPSS 22 were used to verify the causal relationships and pathways between the variables in the conceptual model.
In the qualitative phase of this research, the first part consisted of selecting interview samples. We selected Shandong, Hebei, Henan and Heilongjiang Province as research samples. Shandong, Hebei and Henan Province are the main vegetable production areas in China. Although the vegetable production of Heilongjiang Province ranks in the middle among Chinese provinces, the vegetable industry is one of the leading industries in the agriculture of Heilongjiang Province and plays a decisive role in increasing farmers' income. During the survey process, all the vegetable farmers interviewed had experience with pesticides application. The survey adopted a combination of stratified random sampling and random sampling to select samples, and the prefecture-level municipal administrative regions under the jurisdiction of each province were selected as sample cities (districts). China has a large area and a large number of farmers, so it is impossible to investigate all the farmers. Meanwhile, China's administrative regions are divided into five levels: province, city (districts), county, town and village. Stratified sampling is used to sample from each administrative region, and then random sampling is carried out. Stratified random sampling and random sampling are conform to the requirements of wide, system and rationality of the research sample. The administrative regions of prefecture-level cities under the jurisdiction of each province are selected as the sample cities (districts). Two townships were randomly selected from each city (district), one administrative village was randomly selected from each town, and several eligible farmers were randomly selected from each village. A total of 125 vegetable farmers were selected.
In the quantitative phase of this research, the second part consisted of the questionnaire item selection. The questionnaire was designed based on the research hypotheses drawn in the first part, and also adopted a combination of stratified random sampling and random sampling to conduct large-scale questionnaire surveys with vegetable farmers in Shandong, Hebei, Henan and Heilongjiang provinces. Data collection used the 5-point Likert scale, and took field research and collected the questionnaire on site. A total of 700 questionnaires were distributed, and 698 were recovered. Due to the limitation of some farmers knowledge level and to fill in the questionnaire of emotional factors, follow the screening criteria of invalid questionnaire to eliminate information incomplete and inconsistent questionnaire, delete the data missing and incomplete questionnaire answered clearly is not consistent with actual situation of extreme questionnaire, 38 questionnaires finally selected is invalid, leaving 660 questionnaires for analysis, resulting in an effective response rate of 94.3%. The information of the sample is shown in Table 2.
The first phase of analysis consisted of open coding, which is the process of conceptualizing and categorizing original interview material. A total of 1,031 initial concepts were abstracted, and they were further categorized through cluster analysis to form 28 categories. To save space, three original statements and their initial concepts were selected for each category.
In the second phase, on the basis of open coding, spindle coding discriminates the correlations and relations among the independent categories, and excavates and establishes the potential logical relationships among the categories. Through analysis, it is found that each of the different categories obtained in open coding has an internal connection at the conceptual level. These internal connections were further aggregated and classified, resulting in 5 main categories. The meaning of each category and its corresponding open coding category are shown in Table 3.
In the third phase, selective coding, which is based on spindle coding, explores the relationships between categories and excavates the core categories from the main categories. We analyzed the relationships between the main categories and core categories, and described the whole logical relationship and context in the form of storylines. A storyline is the construction of a new substantive theoretical framework. The logical context in this research storyline reflects the influencing factors and pathways of vegetable farmers' green pesticide application behavior (GB), as shown in Table 4.
In the fourth stage, we used the remaining 1/4 of the interview records for test the theoretical saturation. The results showed that no new concepts were found, and no new logical relationships appeared in the existing main categories, indicating that the attribution model of vegetable farmers' green pesticide application behavior has good theoretical saturation.
Seven categories were generated at the end of the data analysis, behavioral attitude (BA), behavioral feedback perception (BP), government supervision and regulation (GR), market adjustment guidance (MG), social reference specification (SS), green pesticide application motivation (GM), and green pesticide application behavior (GB). The categories were arranged in graphical order based on the associations between them. Through the typical logical relationships in the storylines, the core category of the “attribution model construction and driving strategy of vegetable farmers” green application behaviors can be determined, and the psychological attribution and driving path of vegetable farmers' green application behaviors can be formed, which can be further summarized as the “(environment, needs) -motivation-behavior” chain. The conceptual model is shown in Figure 2. Based on the results of the grounded theory, the following hypotheses are proposed:
H1: Behavioral attitude has positive effect on green pesticide application behavior.
H2: Behavioral attitude has positive effect on green pesticide application motivation.
H3: Behavioral feedback perception has negative effect on green pesticide application behavior.
H4: Behavioral feedback perception has negative effect on green pesticide application motivation.
H5: Green pesticide application motivation has positive effect on green pesticide application behavior.
H6: Green pesticide application motivation plays an intermediary role between behavioral attitude and green pesticide application behavior.
H7: Green pesticide application motivation plays an intermediary role between behavioral feedback perception and green pesticide application behavior.
H8: Government supervision and regulation plays a regulatory role between green pesticide application motivation and green pesticide application behavior.
H9: Market adjustment guidance plays a regulatory role between green pesticide application motivation and green pesticide application behavior.
H10: Social reference specification plays a regulatory role between green pesticide application motivation and green pesticide application behavior.
SPSS24.0 software was used to test the reliability and validity of the questionnaire, and the specific data are shown in Tables 5, 6. Reliability testing uses Cronbach's alpha as an indicator, and the Cronbach's alpha values of all variables are >0.8, indicating that the scale has high internal consistency and good reliability. The values of the factor loading meet the requirements of being between 0.5 and 0.95, and there is a significant difference at the level of p < 0.01 (28). The average variance extract (AVE) is used to measure convergent validity. Construct reliability (CR) is used to measure structural validity. As shown in Table 5 the AVE values of all variables are >0.6, the square root value of AVE is greater than the correlation coefficient of the ranks, and the CR values are >0.9, indicating that the measurement model has good validity (29).
In this study, IBM SPSS AMOS 24 was used to analyze the direct and mediating effects (30). The structural equation modeling is shown in Figure 3. It can be seen from Table 7 that the measurement model is consistent with the survey data. Thus, the model shows a good fit to the data.
The test results of the direct effect are shown in Table 8. Among the variables, five path coefficients are all significant (p < 0.01). Thus, H1, H2, H3, H4, and H5 are all established.
The mediating effects were tested based on the bootstrapping test method proposed by Ji et al. (8), and the test results are shown in Table 9. ① In the interaction relationship between BA and GB, with GM as an intermediary variable, the bias-corrected and percentile 95% confidence interval (CI) did not contain zero, p < 0.01, This result shows that the mediating effect of BA is significant, because BA has a significant direct effect on GB, and GM has a partial mediating effect in the interaction between BA and GB. ②In the interaction relationship between BP and GB, with GM as an intermediary variable, the bias-corrected and percentile 95% confidence interval (CI) did not contain zero, p < 0.01, This result shows that the mediating effect of BP is significant, because BP has a significant direct effect on GB, GM has a partial mediating effect in the interaction between BP and GB. Therefore, H6 and H7 are both established.
The Process plug-in of SPSS software was used, and model type 1 was selected. The non-parametric percentile method of bias correction was used for analysis. Bootstrapping consisted of 5,000 iterations, and the bias-corrected effect test results are shown in Tables 10–12.
Table 10 shows that the interaction terms between GR and GM is significant (β = 0.156, p < 0.001), indicating that GR plays an intermediary role between GM and GB. Table 11 shows that the interaction term between MG and GM is significant (β = 0.154, p < 0.001), indicating that MG plays an intermediary role between GM and GB. Table 12 shows that the interaction term between SS and GM is not significant (β = 0.019, p > 0.1), indicating that SS does not play a regulatory role between GM and GB.
The purpose of drawing the mediating effect diagram is to intuitively show the intermediary role of GR and MG (see Figures 4, 5). Both GR and MG positively mediate the relationship between GM and GB. These conclusions are consistent with the data analysis results in Tables 10, 11. Therefore, H8 and H9 are valid, while H10 is not.
In-depth interviews were conducted to explore the influencing factors of vegetable farmers' green pesticide application behavior (GB), and the grounded theory was used to construct a conceptual model of the influencing factors.
Internal factors in behavior: behavioral attitude (BA), behavioral feedback perception (BP), and green pesticide application motivation (GM) are the internal factors of green pesticide application behavior (GB).
From the research results, vegetable farmers' pesticide residue awareness (PRA), pest and disease awareness (PDA), environmental pollution awareness (EPA), health hazard awareness (HHA), social responsibility awareness (SRA), and knowledge ability level (KAL) have a positive impact on their GM, and these factors further promote their GB. When vegetable farmers have sufficient pesticide residue awareness (PRA), vegetable farmers have a relatively strong GM. Although vegetable farmers have a certain understanding of pests and diseases, as well as the yield and appearance of vegetables, compared with the destruction of the natural ecological balance, they pay more attention to the former. This phenomenon is the result of the combination of vegetable farmers' rationality and irrationality. Vegetable farmers have better environmental pollution awareness (EPA), they believe that pesticide residues cause river, well and soil pollution, and that the improper disposal of pesticide wastes seriously threaten the environment. They have insufficient knowledge of excessive pesticide residues. As a result, vegetable farmers' GM is not strong enough. Vegetable farmers lack the social responsibility awareness (SRA) and the knowledge ability level (KAL) is limited, weakening the subjective initiative of vegetable farmers to apply green pesticides to a certain extent, and inhibiting their GB.
BP has a negative effect on vegetable farmers' GM, and thus inhibits their GB. The BP conforms to human information processing, it is vegetable farmers' perception of their previous personal experience and practical effect feedback, as well as their practice prediction, determining the generation of GM. According to the interview results, most vegetable farmers expressed their willingness to adopt GB, on the premise that their inner needs must be met. According to the commodity smallholder theory proposed by Huang Zongzhi, smallholders' decision-making behavior is affected by both economic and non-economic factors. The GB of vegetable farmers must consider the influence of their own economic interests; if green pesticide application behavior can reduce costs, increase income and prevent danger, then vegetable farmers will adopt GB. If the cost of GB is too high, the profits are too low or risks are incurred, rational vegetable farmers will not adopt GB. Only when vegetable farmers' GB feedback results meet their inner needs, will they actively choose GB, otherwise, their green pesticide application motivation will be greatly reduced or even be zero. At the same time, over the course of many years of farming, the application behavior of vegetable farmers has become habitual, meaning that it is difficult to change.
External factors in behavior: government supervision and regulation (GR), market adjustment guidance (MG) and social reference norms (SS) are the external factors of green pesticide application behavior (GB).
GR is the external factor in vegetable farmers' GB, and it positively mediates the relationship between GM and GB. In the interviews, most vegetable farmers expressed that they did not know the laws and regulations related to the quality and safety of agricultural products; therefore, they had little or no impact on them. However, most vegetable farmers said that they would adopt green pesticide application if China's legal and regulatory enforcement (LRE) was strict and established a quality traceability system (QTS) to hold them accountable for their non-green pesticide application behavior. If the output of green vegetables is lower than ordinary vegetables, government subsidies can make a difference by ensuring that vegetable farmers do not suffer losses and by even providing additional rewards, so that vegetable farmers will be willing to adopt green pesticides application. In the incentive subsidy support (ISS), it is necessary to combat corruption to ensure the effective implementation of support policies. In addition, due to the lack of pesticide operation standardization (POS) and the chaos in pesticide market orders, fake pesticides and highly toxic pesticides still exist. Additionally, vegetable farmers are limited by their ability, and as a result, they randomly purchase pesticides.
MG is an external factor in vegetable farmers' GB, and it positively mediates the relationship between GM and GB. Realizing a quality control entry market (QCEM), quality mark identification (QMI), price based on quality (PBQ) and key risk control (KRC) can effectively improve vegetable farmers' green pesticide application motivation (GM), so that they actively choose GB. Market supervision departments mainly collect stall fees and undertake the task of clearing stores in the fragmented market, and the quality and safety of vegetables are not inspected and supervised. Notably, farmers use advanced methods to obtain good sales channels, and some vegetable farmers use WeChat groups and circles of friends to sell vegetables. While attracting many customers, they can also sell at a good price, which stimulates vegetable farmers' enthusiasm for green pesticide application. The information asymmetry between vegetable farmers and consumers, and consumers' lack of knowledge about vegetable quality and safety, suggest that consumers like to buy vegetables that have a beautiful appearance or that taste good. However, they do not know that the beautiful appearance of vegetables may be due to enlargers, sweeteners, ripening agents and so on. Vegetables that do not use pesticides randomly are not beautiful and have an average taste. In addition, the price of high-quality and safe vegetables is relatively high, and as a result, consumers have insufficient green vegetable recognition, acceptance and purchasing power.
Although some of the hypotheses are not empirically verified, it is undeniable that social factors can enhance the relationship between GM and GB. At present, the interviews found that there are various media publicity methods for agricultural product quality and safety, but the effect is not good. Public supervision and reports have a deterrent effect on vegetable farmers, who are afraid of being punished. If media exposure is used, the concept of smallholders will cause ambivalence among vegetable farmers in regard to using pesticides: on the one hand, they will be afraid of being laughed at by neighbors because of their poor harvest; on the other hand, in most rural areas, people are almost always familiar with each other, exposure will make them become the object of comment. In rural society, the group convergence effect brought by conformity behavior and shortcut behaviors are suppression and promotion factors in vegetable farmers' GB. In particular, neighbors and large vegetable farmers play an indispensable role in the transformation and spread of vegetable application technology. Small-scale vegetable farmers often tend to envy and venerate large vegetable farmers. The successful planting experience of large farmers plays a leading role in demonstration, allowing small-scale vegetable farmers to quickly see the benefits. Social moral constraints (SMC) can restrict vegetable farmers' GB to a certain extent, and it is morally acceptable to spray pesticides on vegetables.
Conducting open coding, spindle coding and theoretical coding of the records of in-depth interviews with vegetable farmers', we explore vegetable farmers' GB and construct a green pesticide application factor model. The qualitative phase of our research shows that the factors in vegetable farmers' GB can be summarized as internal and external factors. Among them, the internal factors include behavioral attitude (BA), behavioral feedback perception (BP), and green pesticide application motivation (GM); external factors include government supervision and regulation (GR), market adjustment guidance (MG), and social reference specification (SS). The quantitative phase of our research shows that vegetable farmers' BA, BP and GM are internal factors in vegetable farmers' GB. Vegetable farmers' BA and BP directly determine the generation and strength of their GM, and indirectly affect GB. As external factors, GR and MG play an intermediary role in the process of generating and enhancing GM and then selecting GB. Strict control by the government, and quality control by the market have an important impact on the vegetable farmers' green pesticide application motivation, thus affecting their green pesticide application behavior. However, social factors cannot be ignored, and it is necessary to improve the existing social environment to encourage vegetable farmers to use green pesticides.
To improve the quality and safety of agricultural products, not only are the complementary advantages of the government, the market and society necessary, but it is also necessary to fully mobilize the enthusiasm of consumers to jointly drive the evolution of vegetable farmers from non-green pesticide application behavior to GB. Starting from multiple perspectives and levels, we construct a multi-agent driving strategy for vegetable farmers to evolve from non-green pesticide application behavior to GB. On the basis of clarifying the influence mechanism of relevant factors on farmers' green pesticide application behavior, the multi-center governance theory, coordination theory and mechanism design theory are used for reference to build a multi governance mechanism for farmers' green pesticide application behavior. The main strategic implications are as follows:
First, government should improve the laws and regulations, strengthen the enforcement of laws and regulations, establish a reward and punishment mechanism, strengthen publicity and education, promote pesticide application standards, pesticide operation standardization, implement pesticide subsidies, establish vegetable quality and safety traceability system, popularize pest prevention technology, promote advanced pesticide, severely combat corruption, and other angles, use administrative measures to supervise and guide vegetable farmers' pesticide application behavior.
Second, give full use to market regulation and control. Focus on regulating the organized physical market, combating strictly the non-contracted non-physical market, improving the organization of the non-organized physical market, strictly inspecting the vegetables entering the market to control the vegetable quality before entering the market. Especially off-season vegetables planted in greenhouses are the key prevention and control targets, and reject unqualified vegetables out of the market. At the same time, introduce and improve the corresponding quality mark identification to distinguish vegetables into different grades. Promote high quality and high price, and encourage consumers to purchase qualified vegetables to reduce the worries of vegetable farmers.
Third, strive to strengthen the social reference effect and let it play its role. Media communication should adopt various modes such as emotional prayer, fearful prayer, relevance prayer, practical experiential activity appeal, moral prayer and others, so that make vegetable farmers empathize and form social moral constraints. Improve the reporting system, and make full use of modern information technology to facilitate the masses to report.
Fourth, avoid information asymmetry among multiple subjects and realize information sharing among multiple subjects. Government departments can establish a complete set of authoritative information publishing and sharing platforms, specific methods can take government departments jointly authorize an information service management agency, collect agricultural product quality and safety market information timely, summarize, identify and analyze timely. Establish and improve agricultural product quality and safety market information warning system and information network platform. Based on this, establishing a multi-agent driving strategy is the only way to promote the evolution of vegetable farmers from non-green to green pesticide application behavior.
The original contributions presented in the study are included in the article/supplementary material, further inquiries can be directed to the corresponding author.
Conceptualization: YT. Methodology and writing—original draft preparation: YT and XC. Investigation: YT, XC, and YJ. Writing—review and editing: YJ, ZY, and XG. Supervision and project administration: YJ, ZY, and XG. Funding acquisition: ZY and YT. All authors have read and agreed to the published version of the manuscript.
This work was supported by Humanities and Social Sciences Foundation of Ministry of Education of China (Grant 18YJC630162), Heilongjiang Province Postdoctoral Science Foundation (Grant LBH-Z17018), Key Laboratory Project of Modern Agricultural Equipment Technology in Northern Cold Region (Grant KF18-01), Young Talents of Northeast Agricultural University (Grant 20XG07), and Heilongjiang Philosophy and Social Sciences Research Planning Project (Grant 21JYD273).
The authors declare that the research was conducted in the absence of any commercial or financial relationships that could be construed as a potential conflict of interest.
All claims expressed in this article are solely those of the authors and do not necessarily represent those of their affiliated organizations, or those of the publisher, the editors and the reviewers. Any product that may be evaluated in this article, or claim that may be made by its manufacturer, is not guaranteed or endorsed by the publisher.
1. Jin J, Wang W, He R, Gong H. Pesticide use and risk perceptions among small-scale farmers in Anqiu county, China. Int J Environ Res Public Health. (2017) 14:29 doi: 10.3390/ijerph14010029
2. Zhao L, Wang C, Gu H, Yue C. Market incentive, government regulation and the behavior of pesticide application of vegetable farmers in China. Food Control. (2018) 85:308–17. doi: 10.1016/j.foodcont.2017.09.016
3. Ngow AVF, Mbise TJ, Ijani ASM, London L, Ajayi OC. Smallholder vegetable farmers in northern tanzania: pesticides use practices, perceptions, cost and health effects. Crop Prot. (2017) 26:1617–24. doi: 10.1016/j.cropro.2007.01.008
4. Kotsanopoulos KV, Arvanitoyannis IS. The role of auditing, food safety, and food quality standards in the food industry: a review. Compr Rev Food Sci Food Saf. (2017) 16:760–75. doi: 10.1111/1541-4337.12293
5. Jallow MFA, Awadh DG, Albaho MS, Devi VY, Thomas BM. Pesticide risk behaviors and factors influencing pesticide use among farmers in Kuwait. Sci Total Environ. (2017) 574:490–8. doi: 10.1016/j.scitotenv.2016.09.085
6. Liu K, Lan Y, Li W, Cao E. Behavior-based pricing of organic and conventional agricultural products based on green subsidies. Sustainability. (2019) 11:1151. doi: 10.3390/su11041151
7. Li L, Guo H. The impact of business relationships on safe production behavior by farmers:evidence from China. Agribusiness. (2019) 35:84–96. doi: 10.1002/agr.21584
8. Ji C, Jin S, Wang H, Ye C. Estimating effects of cooperative membership on farmers' safe production behaviors: evidence from pig sector in China. Food Policy. (2019) 83:231–45. doi: 10.1016/j.foodpol.2019.01.007
9. Liu T, Bruins RJF, Heberling MT. Factors influencing farmers' adoption of best management practices: a review and synthesis. Sustainability. (2019) 10:432. doi: 10.3390/su10020432
10. Khalid SMN. Food safety and quality management regulatory systems in Afghanistan: Policy gaps, governance and barriers to success. Food Control. (2016) 68:192–9. doi: 10.1016/j.foodcont.2016.03.022
11. Hafezi M, Zolfagharinia H. Green product development and environmental performance: investigating the role of government regulations. Int J Product Econ. (2018) 204:395–410. doi: 10.1016/j.ijpe.2018.08.012
12. Hoi PV, Mol PJ, Oosterveer P, van den Brink PJ, Huong TM. Pesticide use in Vietnamese vegetable production: a 10-year study. Int J Agric Sustain. (2016) 14:325–38. doi: 10.1080/14735903.2015.1134395
13. Baur I, Dobricki M, Lips M. The basic motivational drivers of northern and central European farmer. J Rural Stud. (2016) 46:93–101. doi: 10.1016/j.jrurstud.2016.06.001
14. Wsa B, Ma Z, Liu M. Cytochrome P450 family: genome-wide identification provides insights into the rutin synthesis pathway in Tartary buckwheat and the improvement of agricultural product quality. Int J Biol Macromol. (2020) 164:4032–45. doi: 10.1016/j.ijbiomac.2020.09.008
15. Takayuki A, Mariko K. Determination of diniconazole in agricultural products, livestock and marine products by LC-MS/MS. J Food Hygien Soc Japan. (2017) 3:195–200. doi: 10.3358/shokueishi.58.195
16. Sok J, Hogeveen H, Elbers ARW, Oude Lansink AGJM. Using farmers' attitude and social pressures to design voluntary Bluetongue vaccination strategies. Prev Vet Med. (2016) 133:114–9. doi: 10.1016/j.prevetmed.2016.09.016
17. Boatemaa S, Barney MK, Drimie S, Harper J, Korsten L, Pereira L, et al. Awakening from the listeriosis crisis: food safety challenges,practices and governance in the food retail sector in South Africa. Food Control. (2019) 104:333–42. doi: 10.1016/j.foodcont.2019.05.009
18. Imami D, Valentinov V, Skreli E. Food safety and value chain coordination in the context of a transition economy: the role of agricultural cooperatives. Int J Common. (2021) 15:21–34. doi: 10.5334/ijc.1039
19. Liu Y. Research on measures to promote rural community construction pilot work. Contemp Soc Sci. (2017) 1:120–34.
20. Long H. Rural Restructuring in Typical Villages of China. Land Use Transitions and Rural Restructuring in China. Singapore: Springer (2020). p. 415–58. doi: 10.1007/978-981-15-4924-3_8
21. Zhang S, Xiang LEE, Wei LEE. Relationship of Multiple Cooperative Governance Mechanism and Traditional Social Capital Reconstitution in South Chinese Village—Based on the Case of Baini. DEStech Transactions on Social Science, Education and Human Science (ermis) (2019).
22. Johansson J. Collaborative governance for sustainable forestry in the emerging bio-based economy in Europe. Curr Opin Environ Sustain. (2018) 32:9–16. doi: 10.1016/j.cosust.2018.01.009
23. Ali AR, Mevlüt GÜL. Determination of satisfaction level of farmers from agricultural credit cooperative activities: a cases of Çeltikçi district of Burdur. Int J Agric Forest Life Sci. (2019) 3:15–21.
24. Bagheri A, Emami N, Damalas CA, Allahyari MS. Farmers' knowledge, attitudes, and perceptions of pesticide use in apple farms of northern Iran: impact on safety behavior. Environ Sci Pollut Res. (2019) 26:9343–51. doi: 10.1007/s11356-019-04330-y
25. Chen XF. Research on participation willingness of farmers' professional cooperatives members. Open J Soc Sci. (2019) 7:334–45. doi: 10.4236/jss.2019.78024
26. Gava O, Ardakani Z, Delalić A, Azzi N, Bartolini F. Agricultural cooperatives contributing to the alleviation of rural poverty. the case of Konjic (Bosnia and Herzegovina). J Rural Stud. (2021) 82:328–39. doi: 10.1016/j.jrurstud.2021.01.034
27. Giagnocavo C, Bienvenido F, Li M, Zhao YR, Sanchez-Molina JA, Yang XT. Agricultural cooperatives and the role of organisational models in new intelligent traceability systems and big data analysis. Int J Agric Biol Eng. (2017) 10:115–25. doi: 10.25165/j.ijabe.20171005.3089
28. Grashuis J, Su Y. A review of the empirical literature on farmer cooperatives: Performance, ownership and governance, finance, and member attitude. Ann Public Cooperat Econ. (2019) 90:77–102. doi: 10.1111/apce.12205
29. Huang ZH, Liang Q. Agricultural organizations and the role of farmer cooperatives in China since 1978: Past and future. China Agric Econ Rev. (2018) 10:48–64. doi: 10.1108/CAER-10-2017-0189
Keywords: vegetable safety, agricultural product quality and safety, farmer behavior, driving strategy, structural equation
Citation: Teng Y, Chen X, Jin Y, Yu Z and Guo X (2022) Influencing factors of and driving strategies for vegetable farmers' green pesticide application behavior. Front. Public Health 10:907788. doi: 10.3389/fpubh.2022.907788
Received: 30 March 2022; Accepted: 13 July 2022;
Published: 08 September 2022.
Edited by:
Rajnarayan R. Tiwari, ICMR-National Institute for Research in Environmental Health, IndiaReviewed by:
Mohamed Larbi Bouguerra, Faculté des Sciences Mathématiques, Physiques et Naturelles de Tunis, TunisiaCopyright © 2022 Teng, Chen, Jin, Yu and Guo. This is an open-access article distributed under the terms of the Creative Commons Attribution License (CC BY). The use, distribution or reproduction in other forums is permitted, provided the original author(s) and the copyright owner(s) are credited and that the original publication in this journal is cited, in accordance with accepted academic practice. No use, distribution or reproduction is permitted which does not comply with these terms.
*Correspondence: Zhigang Yu, YTE3MzkwNjA1MTg4QDE2My5jb20=; Xiangyu Guo, YTEzNjgzM2FhQDE2My5jb20=
†These authors share first authorship
Disclaimer: All claims expressed in this article are solely those of the authors and do not necessarily represent those of their affiliated organizations, or those of the publisher, the editors and the reviewers. Any product that may be evaluated in this article or claim that may be made by its manufacturer is not guaranteed or endorsed by the publisher.
Research integrity at Frontiers
Learn more about the work of our research integrity team to safeguard the quality of each article we publish.