- 1Department of Public Health Medicine, University of KwaZulu Natal, Durban, KwaZulu-Natal, South Africa
- 2Bloomberg School of Public Health, Johns Hopkins University, Baltimore, MA, United States
- 3Faculty of Health Sciences, University of the Free State, Bloemfontein, South Africa
Background: Subsequent to the demonstrated potential of community health workers (CHWs) in strengthening health systems to improve health outcomes, recent literature has defined context and guidelines for integrating CHW programs into mainstream health systems. However, quantitative measures for assessing the extent of CHW program integration into national health systems need to be developed. The purpose of this study was to validate a newly developed scale, Community Health Worker Program Integration Scorecard Metrics (CHWP-ISM), for assessing the degree of integration of CHW programs into national health systems in Sub-Saharan Africa (SSA).
Methods: Data obtained through a pilot study involving a purposively selected sample of 41 participants selected from populations involved in CHW programs work in selected countries of SSA formed the basis of a 31-item bifactor model. Data were collected between June and December 2019. By applying a latent variable approach implemented with structural equation modeling, data analysis was mainly done using the R statistical environment, applying factor analysis procedures.
Results: Dimensionality, construct validity, and the CHWP-ISM scale's internal consistency were assessed. Confirmatory factor analysis of the CHW-ISM bifactor model supported a co-occurring CHW integration general factor and six unique domain-specific factors. Both the comparative fit index (CFI) and Tucker–Lewis Index (TLI) fit indices were above 0.9, while the root mean square of the residuals (RMSR) was 0.02. Cronbach's alpha (α), Guttman 6 (Lambda 6), and Omega total (ωt) were above 0.8, indicating good scale reliability.
Conclusion: Statistical significance of the bifactor model suggests that CHW integration has to be examined using factors that reflect a single common underlying integration construct, as well as factors that reflect unique variances for the identified six subject-specific domains. The validated CHWP-ISM could be useful to inform policy advisers, health systems, donors, non-governmental organizations, and other CHW program stakeholders with guidance on how to quantitatively assess the integration status of different components of CHW programs into respective critical functions of the health system. Improved integration could increase CHW program functionality, which could in turn strengthen the healthcare systems to improve health outcomes in the region.
Introduction
Research evidence demonstrating the potential of community health workers (CHWs) in strengthening health systems to improve health outcomes has been growing (1–7), particularly their efficacy in improving the reach, impact, and efficiency of health services (8). In addition to improving health outcomes in general, evidence has also demonstrated their prospect in improving child health outcomes, particularly reducing childhood morbidity and mortality. (1, 9–12). This has been attributed to many reasons, including their placement as a bridge between health facilities and communities (13–16), which accord CHWs with an immense and unique advantage of proximity and availability in communities, to mobilize community members to identify and address their own health needs (13, 17).
However, this evident efficacy of CHWs in delivering community-based preventive and curative services is being truncated by varying, subjective, or at worst lack of CHW program integration into national health systems (11, 18–20). In an attempt to review practical strategies to reduce the Under 5 Mortality Rate (U5MR), the 2015 Renewed Promise to Child Survival underscored the need to strengthen health systems to deliver high-quality high-impact interventions (HIIs) (21) for child health. Scott et al. highlighted that the integration of CHWs with health systems necessitates their inclusion into public policies that direct national service delivery, human resources for health, health financing, medical products and technologies, health information and leadership, and governance critical functions of the health system (22). The call for this health workforce cadre to be integrated into national health systems has been stressed (8, 22–29) and in particular, in Sub-Saharan Africa (SSA) region (4, 8).
Recent literature has defined the context, mechanisms, and guidelines for integrating CHW programs into mainstream health systems (1, 19, 25–27, 30–36). However, much of what is currently in use for assessing CHW program integration is based on qualitative measures. Although qualitative metrics add valuable information, given that some integration determinants cannot be captured by quantitative measures, such metrics can be subjective. Qualitative metrics may include human experience or judgment as a factor in measurement and information that can often be difficult to measure due to ambiguity. On the other hand, there are advantages to measuring CHW integration quantitatively because the extent can often be clearly expressed as a ratio, percentage, or even average that can be compared across two or more program settings. Basing CHW integration metrics on quantitatively validated models increases transparency and consistency. To date, little research has been done to assess CHW programs' integration quantitatively or the use of quantitative integration metrics to assess CHW program integration into national health systems, at both policy and implementation levels.
This study validated newly developed scorecard metrics for measuring the extent of CHW programs' integration into national health systems (CHWP-ISM) in Sub-Saharan Africa (38) at the policy level. The paper tests the proposed CHW integration metrics scale's construct validity, dimensionality, and reliability. The items, generated and reported in Mupara et al. (38), included some suggestions proposed by Boateng et al. (37) as best practices for developing and validating scales for health.
Methodology
Theoretical considerations, item selection, and study conceptual framework
The present study was based on the earlier reported study (38), which is part of a wider study undertaken to generate items suitable for assessing the extent of integration of CHW programs into national HS. The study (Mupara et al. (38) study on integration indicators) was based on WHO's building blocks for strengthening health systems (HSS) (Figure 1). In this study, CHW program integration at the policy level has been defined as the policy-level guidelines and directives that specify how CHW programs should be mainstreamed into national HS. These policy guidelines were viewed in line with the WHO Health systems (HS) building blocks, namely, service delivery, human resources for health, health information, medical products and technologies, health financing, and leadership, and governance (39). Therefore, for the purpose of this study, six matching integration domains whose indicators could be measured to ascertain the extent of CHW program integration, specifically at the policy level, were identified. These were CHW Recruitment, Education, and Certification (REC) (23, 25, 26); CHW Role and Responsibilities (R&R); CHW Remuneration; CHW Supervision; CHW Information Management; and CHW Equipment and Supplies (25, 26, 32, 33, 40). Whereas, the earlier study had identified a hundred (100) items for measuring the extent of CHW integration into national health systems, this study took a subset of 31 items, with CHW REC and CHW R&R having six indicators each. The rest of the parameters had five indicators each except for CHW Remuneration which had four indicators. Item selection and distribution for each integration variable are indicated in Table 1 and Figure 1.
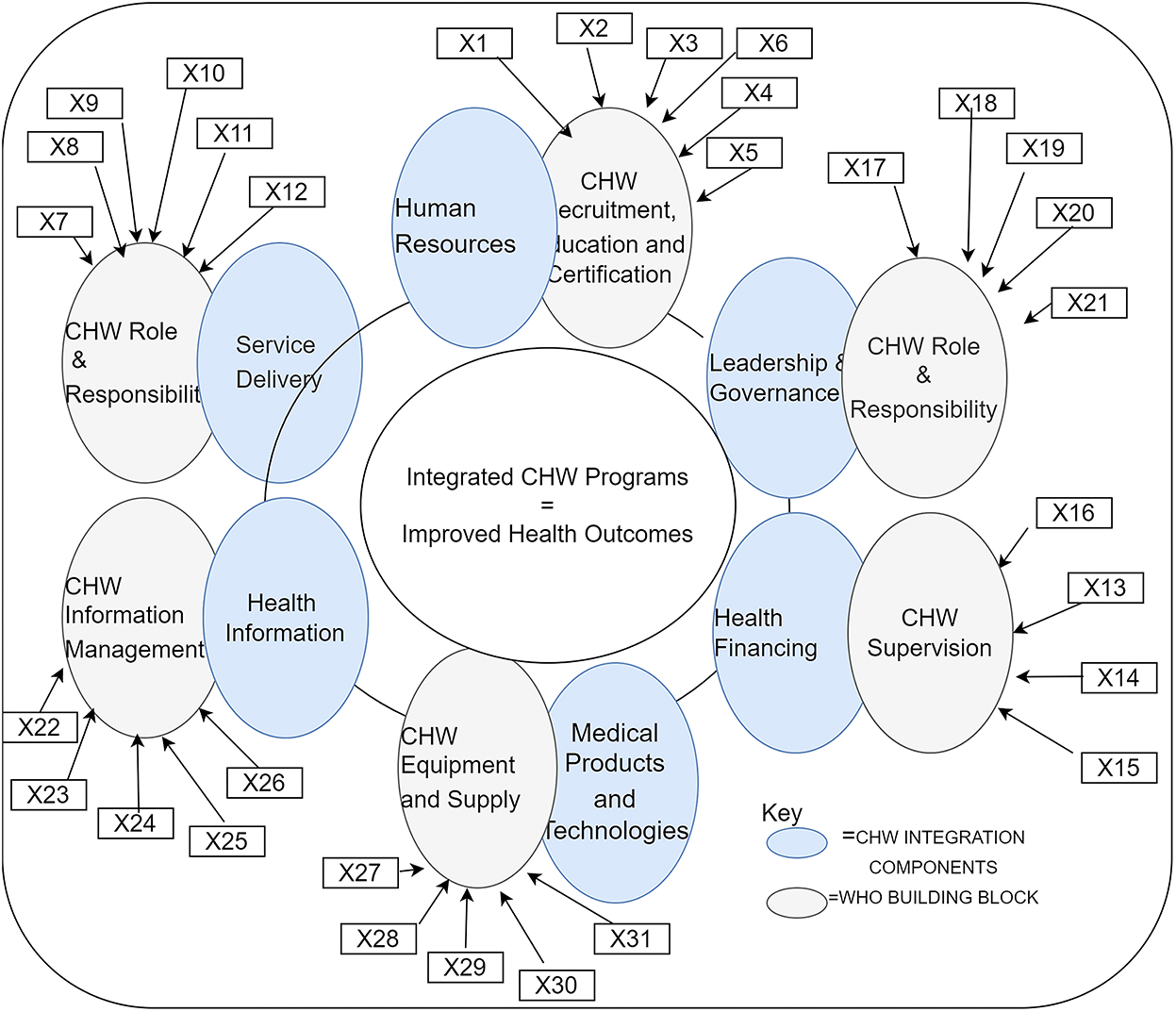
Figure 1. Study conceptual framework (X1–X31 as indicated in Table 1). Source: Adapted from the study by Mupara and Mogaka (38).
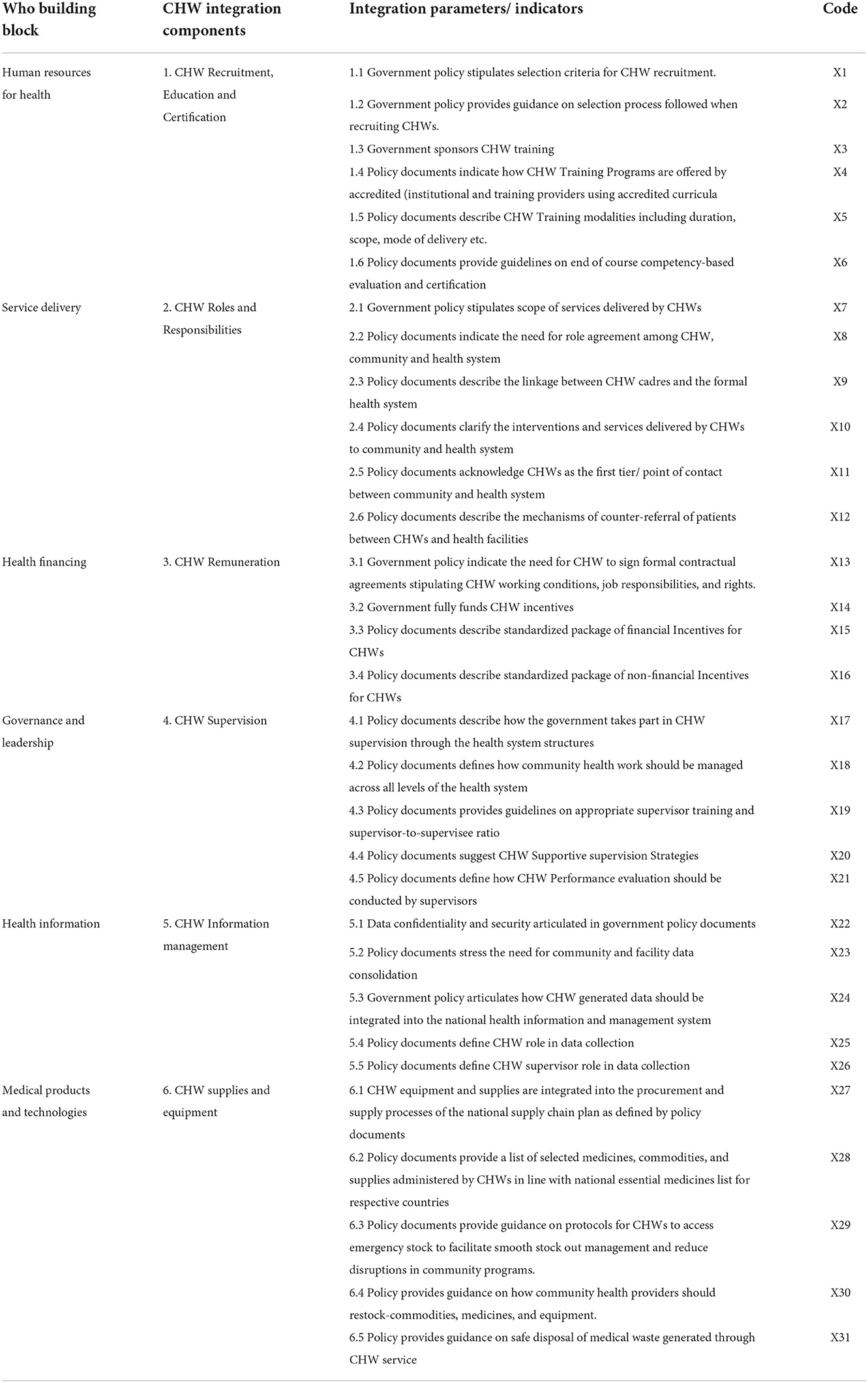
Table 1. The six WHO critical functions and community health worker (CHW) integration program components, integration parameters, and integration indicators (measures).
Study participants
Purposive sampling was employed to identify and invite a sample of 45 healthcare providers who were involved with CHW program work at different levels of service delivery in SSA. Out of the invited 45 healthcare providers, 41 agreed to participate in this study. Figure 2 gives a graphical presentation of the composition of the study participants, comprising of CHWs, senior CHWs, CHW trainers/lecturers, health education technicians and officers, nurses, medical doctors, and public health researchers. Their educational qualifications ranged from certificate, diploma, degree, master's, and Ph.D. holders. In selecting the sample, the study particularly focused on the diversity of participants in terms of educational levels and different healthcare cadres involved in community health work at different levels and settings to ensure data source triangulation.
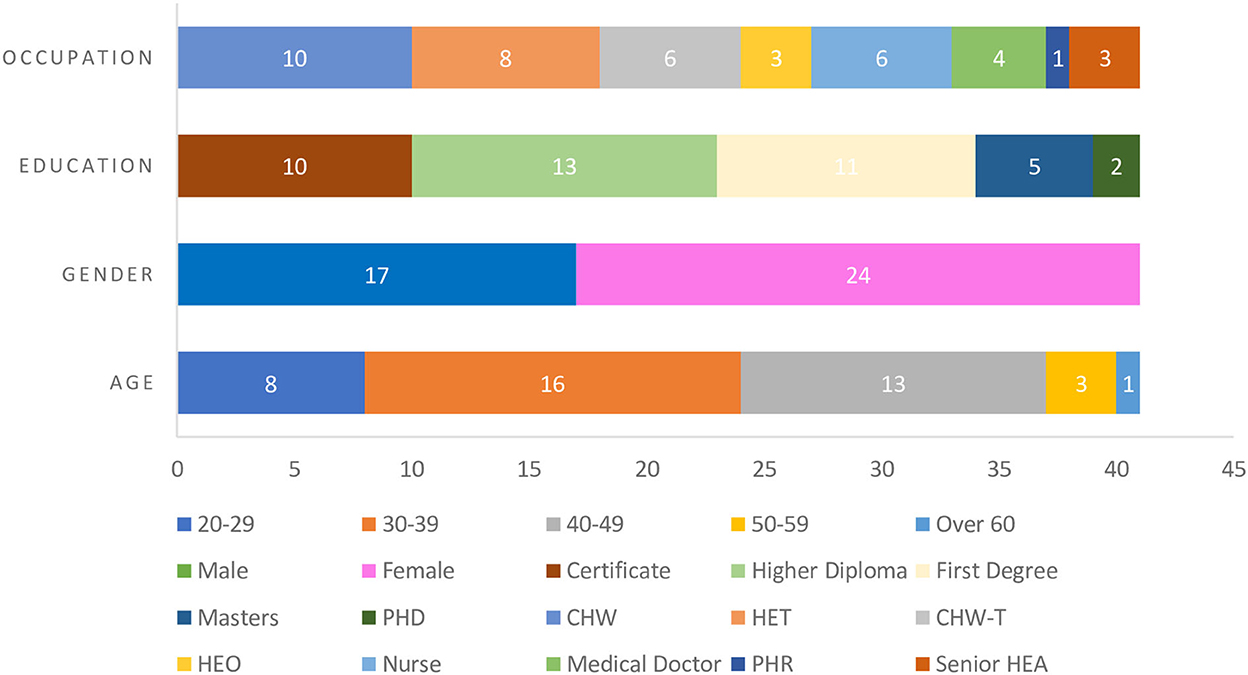
Figure 2. Demographic characteristics of study participants. CHW, community health worker; HET, Health Education Technician; CHW-T, CHW trainer; HEO, Health Education Officer; PHR, Public Health Researcher; HEA, Health Education Assistant.
Procedure
This study was guided by the general principles of the Nuremberg Code, the Declaration of Helsinki, and the Institutional Review Board. A study package was delivered to selected eligible participants by hand, courier services or email depending on the geographical location (distance) of potential participants between January and April 2019. The questionnaires were self-administered. The package included an invitation letter with a study description, a consent form, and a questionnaire. The first section of the questionnaire was designed to elicit demographic information about participants, including their age, gender, educational level, and occupation. The participants were asked to rate their considered opinion on a 5-point (“strongly agree” to “strongly disagree”) Likert-type scale in order to express how certain items could be used to measure the extent of integration of CHW programs into national health systems. The items that participants responded to are listed in Table 1. Forty-one participants successfully completed the survey. Completed questionnaires were retrieved back from participants through hand delivery and couriered services. Data were captured in Excel, cleaned, and processed for analysis using R. There were no missing data.
Statistical considerations
The CHWP-ISM bifactor model was assessed using a latent variable approach implemented with structural equation modeling. The study constructed latent variables (constructs) that were hypothesized to have varying influences on CHW integration. Their relationship with corresponding integration (observable) indicators was then statistically tested using the bifactor modeling method (41, 42) through exploratory and confirmatory factor analyses (EFA/CFA) (43).
The 31 items were grouped to reflect specific domains, which are as seen in the study by Tellegen and Waller (44). The specific domains for this study were CHW REC; CHW R&R; CHW Remuneration; CHW Supervision; CHW Information Management, and CHW Supplies and Equipment as shown in Table 1. A bifactor latent structure analysis, as explained in the study by Reise et al. (45), was done to assess the scale's unidimensional-multidimensional nature. Bifactor models allow for the assessment of hierarchical models of constructs, examining whether indicators contribute to specific (unique) factors over and above their contribution to a general factor (42). This modeling approach enabled us to derive (via EFA) and test (via CFA) the most optimal way to present the CHWP-ISM scale: either as a conceptually broad “CHW integration” unidimensional factor, or as a multidimensional scale made of domain-specific factors; or as a blend of the two, reflecting both general and sub-domain factors. The single factor scale reflected the variance common among all observed measures in the checklist, while the multidimensional checklist reflected additional common variance among item clusters, corresponding to content-specific subdomain constructs. First, a factor analysis model was specified using the “omega” (46) function in “psych” (47) version 1.8.12 R package, i.e., an exploratory factor analysis (EFA). The “omega” function incorporates Leiman transformations (41) in estimating bifactor structures. Next, a CFA model was specified using “lavaan” version 0.6–3 (48, 49) in R version 3.6.1 (50), which provided the fitted item to use with the “omegaFromsem” function for CFA.
Criteria for item retention for the final model were based on factor loading >0.20 on either the general factor or any of the specific factors. The goodness of fit was tested using global and local fit indices. Given that this was a new scale development, cutoff points were according to the following fit criteria: SRMR≤ 0.1, TLI and CFI ≥0.80 for acceptable fit, root mean square error of approximation (RMSEA)≤ 0.06, and SRMR≤ 0.08 (51). Besides, both modification indices and item content theory were used in model modification. Coefficient alpha (α) and 3 omega coefficients (ωT, ωh, and ωhs) from the ‘omegaSem' output were used to examine the internal consistency and reliability of the CHWP-ISM scale.
Results
Demographic characteristics of participants
There were 41 participants out of the initial 45 invited (88% response rate). There were more female participants than male participants (51%). Those with a diploma or above as the highest academic qualifications were the majority (75%), while 24 % of the participants who hold certificate qualifications are CHWs. All participants who took part in the study had a tertiary education qualification and were all proficient in the English language. The rest of the participants' demographic profiles varied greatly and are summarized in Figure 2.
Correlations and descriptive statistics
Descriptive statistics of the data were obtained, including an analysis of outliers, which was defined as any value that is greater than three standard deviations above or below the mean. Skew statistics were assessed using the “mardia” function in “psych” version 1.8.12 (52) R package that applies Mardia's tests for multivariate skewness and kurtosis. Skew values ranged from (–) 1.87 to (–) 0.85 and kurtosis (k) statistics for 30 items ranged from −0.49 to 1.91, with the 31st item indicated at k = 3.61. However, this outlier did not alter results and so it was included in the dataset. The data have a general normal distribution. This justified the use of the maximum likelihood estimation method (ML) in carrying out further analysis using the data.
Variable correlation
We used the R package “corrplot” version 0.84 (53) to visualize the data correlation matrix. The correlogram in Figure 3 displays variables in the correlation matrix and how they relate with each other. In the upper triangle, positive correlations are displayed in blue and negative correlations in red color. Color intensity and the size of the circle are proportional to the correlation coefficients, helping to identify “groups” of variables that share a strong relationship with each other (hierarchical clustering). The lower triangular correlation matrix displays the actual correlation values.
Construct validity
We estimated the CHW-ISM bifactor model by finding the number of latent factors underlying the 31 integration items (observed variables) with an initial EFA. Figure 4 shows the item correlations between observed indicators and the latent variables. Table 2 shows the EFA solution that indicates seven eigenvalues, with five that are >1.0 and two closer to 1, implying seven extractable factors (one general factor and six content-specific factors). This is consistent with our prior hypothesis on CHW-ISM structure: a general (unidimensional) factor and six content-specific unique factors. A further examination of the EFA factor structure in Table 2 shows that, for the most part, the loading pattern of the bifactor solution has items more or less perfectly settling into respective domain-specific parcels that speak to the six domains as hypothesized (Table 1). More generally, however, the items have more loading on the general factor (CHW program integration). This implies a factor structure in which convergent and discriminant validity are evident by the high loadings to the specific factors, with most factors loading above 0.70. Loading on the general factor, however, greatly varies: some items' loadings are < 0.2 (not shown because we set cutoff level of ≥ 0.2 loadings), while others are as high as 0.9. This is strong evidence that the main CHW integration construct can be sub-scaled into its separate, distinct but correlated elements/subscales.
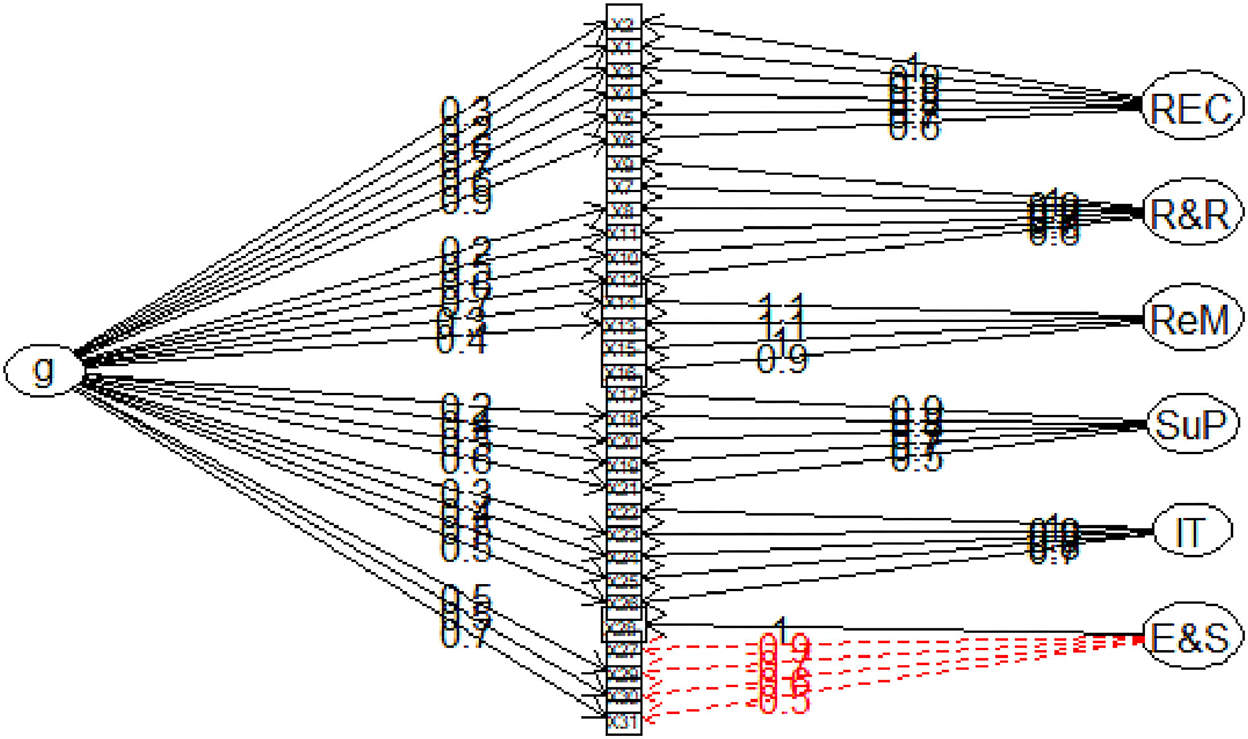
Figure 4. Exploratory bifactor model for CHW-ISM scale. g, the conceptualized general factor indicative of communalities of all items; REC, “CHW Recruitment, Education and Certification” construct; R&R, “CHW Roles and Responsibilities” construct. ReM, “CHW Remuneration”; SuP, “CHW Supervision”; IT, “CHW Information management”; E&S, “CHW supplies and equipment” Items labels (x1–x31) correspond to items as listed in Table 1.
Column “h2” in Table 2 presents values for item-explained common variance (i.e., percent communality) due to the general factor. These values suggest most items from all constructs equally form good candidates for inclusion in a unidimensional (one common factor) item set, i.e., they form the core of a unidimensional CHW-ISM scale. This is evidenced by their large communality values (h2).
To examine the extent the CHW-ISM bifactor model fits the data, we examined a number of absolute and relative fit indices (51). The root mean square of residual (RMSR), the square root of the difference between the residuals of the sample covariance matrix and the hypothesized covariance model, was indicated as 0.02 (acceptable fit is below 0.08 (51)). The comparative fit index (CFI) and Tucker Lewis Index (TLI) above.95 are currently considered an indicator of excellent fit (51, 54), and CHW-ISM Scale's CFI and TLI were above this threshold, indicating excellent fit. Average Variance Extracted (AVE) was indicated as 0.7, which is above the recommended > 0.5 for establishing convergent validity. The above factor loading and model fit statistics present evidence for the construct validity of the CHWP-ISM scale.
CHWP-ISM scale reliability
To test the bifactor model's dimensionality, we examined whether item factor loading of the scale could be explained by one general factor, plus several specific factors corresponding to each of the scale's facets (dimensions).
As seen in Table 2, the standardized coefficient alpha was given as 0.98, implying that 98% of the observed score variance can be attributed to the “true score” variance. However, coefficient alpha has been said to be limited in explaining variance when the data are multidimensional, as in the case of a bifactor model (55). The factor structure in Table 2 suggests an essentially congeneric model and not a tau-equivalent model that the alpha coefficient is particularly suited for (56). Therefore, we also used omega coefficients (41, 45, 46) to indicate the construct reliability of the PMI measurement scale. It has been noted that omega coefficients provide a more accurate approximation of a scale's reliability (57).
Omega total (ωt) accounts for the variance due to the general factor, as well as the group factors. From Table 2, it is indicated that ωt = 0.98. Even though ωt is appropriate for varying factor loadings as seen in the bifactor model, its value is influenced by all modeled sources of common variance and includes item-specific variance as an error (58). To further clarify sources of variance, we used coefficient omega hierarchical (ωh) and coefficient omega hierarchical subscale/group (ωhS). Coefficient ωh estimates the proportion of variance in total scores that can be attributed to a single general factor. From Table 2, when ωt and ωh are compared, we noted that 42% of all reliable variance in the total scores (0.42/0.98) can be attributed to the general factor, assumed to reflect individual differences in the trait of CHW integration. Fifty-seven percent (0.95–0.36) of the reliable variance in total scores is attributable to the multidimensionality caused by the four group factors. This implies that the CHW-ISM scale is principally (~40–60) unidimensional-multidimensional, reflecting a co-occurring CHW integration general factor and six unique content-specific factors. This is also supported by the explained common variance (ECV) due to the general factor, which is 0.22, implying that the general factor explains 22% of the common variance extracted, with 78% of the common variance spread across the six unique group factors.
On the other hand, the unique variance associated with each of the six subscales once the variance associated with the general (unidimensional) factor is partitioned out is indicated by coefficient ωhs. This index reflects the reliability of a subscale score after controlling for the variance due to the general factor (59). In this case, ωhs was reported as REC = 0.89; R&R = 0.73; ReM = 1.21; Sup = 0.68; IT = 0.77; and E&S = 0.38. These values imply that the reliability of subscales is adequate and justifies the use of the CHW-ISM subscales for any future quantitative investigations of CHW integration into health systems.
Discussion
With the growing importance of CHW programs on the global health agenda, comes the responsibility to create a scientific foundation for CHW integration metrics and evaluation. However, CHW integration into national health systems is a broad concept. This implies that the 'CHW integration' construct has a more diverse content, making it more reasonable to question whether it can be adequately measured as a single unidimensional construct or as a multidimensional construct composed of many sub-constructs. In this study, a bifactor model was applied to explore the unidimensional-multidimensional structure of the newly defined CHW integration measurement tool. Study findings provide evidence to the question as to whether the data set had a strong enough common factor (unidimensional), or had a more complex multidimensional (content-specific subcategory) structure. Furthermore, the tool's validity and reliability as a quantitative measure of CHW integration into healthcare systems were investigated and documented. This presented a strong basis for an objective means of measuring CHW integration into national health systems across healthcare jurisdictions.
Although factor loading was adequate, the bifactor CFA model did not suggest a perfect unidimensional CHW integration construct, as shown in Figure 4. Furthermore, strong content-specific correlations did not point to the main common CHW integration construct. Therefore, the combined CHW-ISM bifactor scale presents a better and more plausible model which can explain scale reliability at subscale (multidimensional) as well as at full-scale (unidimensional) levels. This is because the bifactor model shows how all items simultaneously measure both the common CHW integration trait and at the same time account for the variance of each item as influenced by domain-specific (subscale) groupings.
Conclusion
This study aimed at validating the CHW Integration Metrics Scorecard (CHWP-ISM) for assessing the degree of integration of CHW programs into the national health system in SSA. We proposed that this validated CHWP-ISM can be used to evaluate the extent of integration of health interventions aimed at strengthening health systems through the WHO HS building blocks. The metrics scorecard can be used to pair component health interventions (integration variables) with corresponding WHO HS building blocks that they feed into, and then the extent of integration can then be determined considering the integration variables.
The process of integration stage is used to determine if there are country policies that speak to the inclusion of CHW program integration variables into respective health system building blocks. Evidence of integration zooms into the specific guidelines developed from the policies detailing the day-to-day running of the CHW programs in a way that exhibits that they are part and parcel of the health system. Having established that the above is in place, the presence or absence of integration indicators will be used to score all the aspects that make up the specific integration parameter. Thereafter, the aggregation of the integration indicators can be judged against the scale to determine the extent of integration.
In general, the CHWP-ISM can be used to review CHW programs' extent of integration at all levels of the health system be they local, district, provincial, or national. Particularly, to reconnoiter the interaction between the respective components of the health intervention under study and its corresponding WHO building block of the health system. It is hoped that the use of this metrics scorecard to assess the extent of integration of CHWs could better strengthen health systems to improve health outcomes in Sub-Saharan Africa.
One weakness of the study is the assumption that all participants understand CHW policy issues at the same level. Further studies should be conducted with participants with the same level of understanding of CHW policy.
Data availability statement
The raw data supporting the conclusions of this article will be made available by the authors, without undue reservation.
Ethics statement
The studies involving human participants were reviewed and approved by Biomedical Research Ethics Committee, University of KwaZulu-Natal. The patients/participants provided their written informed consent to participate in this study.
Author contributions
All authors listed have made a substantial, direct, and intellectual contribution to the work and approved it for publication.
Conflict of interest
The authors declare that the research was conducted in the absence of any commercial or financial relationships that could be construed as a potential conflict of interest.
Publisher's note
All claims expressed in this article are solely those of the authors and do not necessarily represent those of their affiliated organizations, or those of the publisher, the editors and the reviewers. Any product that may be evaluated in this article, or claim that may be made by its manufacturer, is not guaranteed or endorsed by the publisher.
References
1. Bhutta ZA. Global experience of community health workers for delivery of health related millennium development goals: a systematic review, country case studies, and recommendations for integration into national health systems. Glob Health Workforce Alliance. (2010) 1:61.
2. Javanparast S, Baum F, Labonte R, Sanders D, Rajabi Z, Heidari G. The experience of community health workers training in Iran: a qualitative study. BMC Health Serv Res. (2012) 12:1–8. doi: 10.1186/1472-6963-12-291
3. Schneider H, Lehmann U. Lay health workers and HIV programmes: implications for health systems. AIDS care. (2010) 22:60–7. doi: 10.1080/09540120903483042
4. Singh P, Sullivan S. One Million Community Health Workers: Technical Task Force Report. New York: Earth Institute at Columbia University (2011) p. 304-310.
5. Lewin S, Munabi-Babigumira S, Glenton C, Daniels K, Bosch-Capblanch X, Van Wyk BE, et al. Lay health workers in primary and community health care for maternal and child health and the management of infectious diseases. Cochrane Database of Sys Rev. (2010) 3:14–9. doi: 10.1002/14651858.CD004015.pub3
6. Perry H. Evidence Synthesis Paper: Community. Prepared for the U.S. Government Evidence Summit on Community and Formal health System Support for Enhanced Community Health Worker Performance. (2012) Washington, DC: USAID.
7. Perry HB, Zulliger R, Rogers MM. Community health workers in low-, middle-, and high-income countries: an overview of their history, recent evolution, and current effectiveness. Ann Rev Public Health. (2014) 35:399–421. doi: 10.1146/annurev-publhealth-032013-182354
8. UNAIDS. 2 million African Community Health Workers: Harnessing the Demographic Dividend, Ending AIDS and Ensuring Sustainable Health for all in Africa. Geneva: Joint United Nations Programme on HIV. AIDS (2017).
9. Jones G. How many child deaths can we prevent this year? The lancet. (2003). 362:65–71. doi: 10.1016/S0140-6736(03)13811-1
10. Lassi ZS. Strategies for improving health care seeking for maternal and newborn illnesses in low-and middle-income countries: a systematic review and meta-analysis. Glob Health Action. (2016) 9:31408. doi: 10.3402/gha.v9.31408
11. Perry HB. Comprehensive review of the evidence regarding the effectiveness of community–based primary health care in improving maternal, neonatal, and child health: 1. Rat Meth Database Desc. (2017). doi: 10.7189/jogh.07.010901
12. Kanté AM. The impact of paid community health worker deployment on child survival: the connect randomized cluster trial in rural Tanzania. BMC Health Serv Res. (2019) 19:492. doi: 10.1186/s12913-019-4203-1
13. Usaid. Community Health Worker Incentives: Lessons Learned And Best Practices From Malawi, African Strategies for Health (ASH). Lilongwe: United States Agency for International Development. (2015).
14. Kok MC, Broerse JE, Theobald S, Ormel H, Dieleman M, Taegtmeyer M. Performance of community health workers: situating their intermediary position within complex adaptive health systems. Human Res Health. (2017) 16:1–7. doi: 10.1186/s12960-017-0234-z
15. Naimoli JF, Perry HB, Townsend JW, Frymus DE, McCaffery JA. Strategic partnering to improve community health worker programming and performance: features of a community-health system integrated approach. Hum Resour Health. (2015) 13:1. doi: 10.1186/s12960-015-0041-3
16. Theobald S, MacPherson E, McCollum R, Tolhurst R. Close to community health providers post 2015: Realising their role in responsive health systems and addressing gendered social determinants of health. in BMC proceedings. BioMed Central. (2015). doi: 10.1186/1753-6561-9-S10-S8
17. USAID. Strengthening Primary Health Care through Community Health Workers: Closing the $2 Billion Gap. World Health Organization. (2017).
18. Frymus D. Community health workers and universal health coverage: knowledge gaps and a need based global research agenda by 2015. Geneva: Global Health Workforce Alliance/World Health Organization. (2013).
19. Global Health Workforce Alliance G. Synthesis Paper: Outcomes of Four Consultations on Community Health Workers and Other Frontline Health Workers May/June 2012. Available online at: https://chwcentral.org/resources/synthesis-paper-developed-out-of-the-outcomes-of-four-consultations-on-community-health-workers-and-other-frontline-health-workers-held-in-may-june-2012/ (accessed December 01, 2022).
20. Schneider H, Hlophe D, van Rensburg. Community health workers and the response to HIV/AIDS in South Africa: tensions and prospects. Health Policy Plann. (2008) 23:179–87. doi: 10.1093/heapol/czn006
21. Unicef. Committing to Child Survival: A Promise Renewed. eSocialSciences. (2015). Available online at: https://www.unicef.org/reports/committing-child-survival-promise-renewed (accessed December 01, 2022).
22. Scott K, Beckham SW, Gross M, Pariyo G, Rao KD, Cometto G, et al. What do we know about community-based health worker programs? A systematic review of existing reviews on community health workers. Human Res Health. (2018) 16:39. doi: 10.1186/s12960-018-0304-x
23. Cometto G, Ford N, Pfaffman-Zambruni J, Akl EA, Lehmann U, McPake B, et al. Health policy and system support to optimize community health worker programmes: an abridged WHO guideline. Lancet Glob Health. (2018) 6:1397–404. doi: 10.1016/S2214-109X(18)30482-0
24. Urassa D, Chaya P. Integration of community health workers into a formal health system of tanzania-measuring satisfaction on the training program using kirk patrick model. Glob J Hum Soc Sci Res. (2018) 18:e1987.
25. WHO. WHO Guideline on Health Policy and System Support to Optimize Community Health Worker Programmes. World Health Organization (2018).
26. WHO. WHO Guideline On Health Policy And System Support To Optimize Community Health Worker Programmes Selected Highlights. 2018 Available online at: http://apps.who.int/iris/bitstream/handle/10665/275501/WHO-HIS-HWF-CHW-2018.1-eng.pdf?ua=1 (accessed January 5, 2019).
27. World Health Organization Regional Office for Africa. Community Health Worker Programmes In The Who African Region: Evidence And Options Policy Brief. (2017) Available online at: https://www.afro.who.int/sites/default/files/2017-07/Community%20Health%20Worker%20Policy%20Brief%20-%20English_0.pdf (accessed January 1, 2019).
28. Zambruni JP. Community health systems: allowing community health workers to emerge from the shadows. The Lancet Global Health. (2017) 5:e866–7. doi: 10.1016/S2214-109X(17)30268-1
29. Ibe O, Morrow, M,. Landscape Analysis of National Community Health Worker Programs In 22 of 25 USAID Priority Maternal Child Health Countries. (2019). Available online at: https://www.mcsprogram.org/resource/landscape-analysis-of-national-community-health-worker-programs/ (accessed December 01, 2022).
30. Ballard M, Schwarz R, Johnson A, Church S, Palazuelos D, Mccormick L, et al. Practitioner Expertise To Optimize Community Health Systems: Harnessing Operational Insight. Research Gate (2017). doi: 10.13140/RG.2.2.35507.94247
32. Crigler L, Hill K, Furth R, Bjerregaard D. Community Health Worker Assessment and Improvement Matrix (CHW AIM): A Toolkit for Improving Community Health Worker Programs and Services. (2013). Bethesda, MD: University Research Co., LLC (URC).
33. Perry HL, Crigler S, Hodgins. Developing and Strengthening Community Health Worker Programs at Scale A Reference Guide and Case Studies for Program Managers and Policymakers. Dhaka: University Press Ltd (2014).
34. Perry H, Crigler L, Lewin S, Glenton C, LeBan K, Hodgins S. A new resource for developing and strengthening large-scale community health worker programs. Human Res Health. (2017) 15:1–3. doi: 10.1186/s12960-016-0178-8
35. WHO. Global Strategy on Human Resources for Health: Workforce 2030. (2016). Available online at: https://apps.who.int/iris/bitstream/handle/10665/250368/9789241511131-eng.pdf (accessed December 01, 2022).
36. Walker PR, Downey S, Crigler L, LeBan K. CHW “Principles of Practice” Guiding Principles for NonGovernmental Organisations and Their Partners for Coordinated National Scale-Up of Community Health Worker Programmes. Washington, DC: CORE Group, World Vision International. (2013).
37. Boateng GO. Best practices for developing and validating scales for health, social, and behavioral research. A Primer. (2018) 3:149. doi: 10.3389/fpubh.2018.00149
38. Mupara LM. Scorecard metrics for assessing the extent of integration of community health worker programmes into national health systems. Af J Primary Health Care Family Med. (2021) 13:2691. doi: 10.4102/phcfm.v13i1.2691
39. World Health Organization. (2007). Everybody's business – strengthening health systems to improve health outcomes : WHO's framework for action. World Health Organization. Available online at: https://apps.who.int/iris/handle/10665/43918 (accessed December 01, 2022).
40. Ballard M, Bonds M, Burey J, Dini HS, Foth J, Furth R, et al. CHW AIM: Updated Program Functionality Matrix For Optimizing Community Health Programs. Washington (DC): USAID (2018). doi: 10.13140/Rg.2.2.27361.76644
41. Reise SP, Moore TM, Haviland MG. Bifactor models and rotations: exploring the extent to which multidimensional data yield univocal scale scores. J Pers Assess. (2010) 92:544–59. doi: 10.1080/00223891.2010.496477
42. Chen FF. Modeling general and specific variance in multifaceted constructs: a comparison of the bifactor model to other approaches. J Pers. (2012) 80:219–51. doi: 10.1111/j.1467-6494.2011.00739.x
43. Jöreskog KG, A. general approach to confirmatory maximum likelihood factor analysis. Psychometrika. (1969) 34:183–202. doi: 10.1007/BF02289343
44. Tellegen A, Waller NG. Exploring personality through test construction: development of the multidimensional personality questionnaire. SAGE Handbook Personal Theory Assess. (2008) 2:261–92. doi: 10.4135/9781849200479.n13
45. Reise S, Moore T, Maydeu-Olivares A. Target rotations and assessing the impact of model violations on the parameters of unidimensional item response theory models. Educ Psychol Meas. (2011) 71:684–711. doi: 10.1177/0013164410378690
46. Revelle W,. Using R The Psych Package to Find ω. (2013). Available online at: http://personality-project.org/r/psych/HowTo/R_for_omega.pdf (accessed December 01, 2022).
48. Rosseel Y,. Package ‘lavaan'. (2015) 1:2017. Available online at: https://cran.r-project.org/web/packages/lavaan/lavaan.pdf (accessed December 01, 2022).
49. Rosseel, Y. (2012). lavaan: An R Package for Structural Equation Modeling. J Stat. Software 48, 1–36.
50. Team RCR. A Language and Environment for Statistical Computing. Vienna: R Foundation for Statistical Computing (2016).
51. Hu LT, Bentler PM. Cutoff criteria for fit indexes in covariance structure analysis: conventional criteria versus new alternatives. Struct Eq Model Multidiscip J. (1999) 6:1–55. doi: 10.1080/10705519909540118
52. Revelle W. Procedures for psychological, psychometric, and personality research. Acesso em. (2012) 9:16–9.
54. Kenny DA. Measuring model fit. (2012). Available online at: http://davidakenny.net/cm/fit.htm (accessed December 01, 2022).
55. Graham JM. Congeneric and (essentially) tau-equivalent estimates of score reliability: What they are and how to use them. Educ Psychol Meas. (2006) 66:930–44. doi: 10.1177/0013164406288165
56. Peters GJ. The alpha and the omega of scale reliability and validity: why and how to abandon Cronbach's alpha and the route towards more comprehensive assessment of scale quality. Eu Health Psychol. (2014) 16:56–69.
57. Revelle W, Zinbarg RE. Coefficients alpha, beta, omega, and the glb: comments on Sijtsma. Psychometrika. (2009) 74:145. doi: 10.1007/s11336-008-9102-z
58. Bentler PM. Alpha, dimension-free, and model-based internal consistency reliability. Psychometrika. (2009) 74:137. doi: 10.1007/s11336-008-9100-1
Keywords: community health work, organization & administration, delivery of health care, integrated, standards (integration metrics), structural equation modeling, quality of health care (improved health outcomes)
Citation: Mupara LM, Mogaka JJO, Brieger WR and Tsoka-Gwegweni JM (2022) Health systems strengthening through policy-level integration of community health worker programs into national health systems for improved health outcomes - scorecard metrics validation: A bifactor structural equation model approach. Front. Public Health 10:907451. doi: 10.3389/fpubh.2022.907451
Received: 29 March 2022; Accepted: 14 November 2022;
Published: 14 December 2022.
Edited by:
Faris Lami, University of Baghdad, IraqReviewed by:
Aanuoluwapo Afolabi, University of Ibadan, NigeriaMatthew Bonds, Harvard University, United States
Copyright © 2022 Mupara, Mogaka, Brieger and Tsoka-Gwegweni. This is an open-access article distributed under the terms of the Creative Commons Attribution License (CC BY). The use, distribution or reproduction in other forums is permitted, provided the original author(s) and the copyright owner(s) are credited and that the original publication in this journal is cited, in accordance with accepted academic practice. No use, distribution or reproduction is permitted which does not comply with these terms.
*Correspondence: Lucia Mungapeyi Mupara, bHVtdXBhcmEmI3gwMDA0MDtnbWFpbC5jb20=