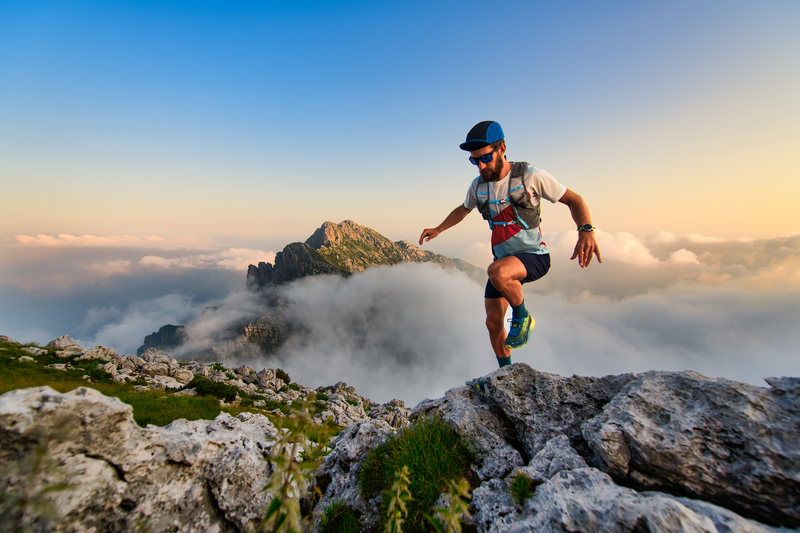
94% of researchers rate our articles as excellent or good
Learn more about the work of our research integrity team to safeguard the quality of each article we publish.
Find out more
SYSTEMATIC REVIEW article
Front. Public Health , 23 June 2022
Sec. Digital Public Health
Volume 10 - 2022 | https://doi.org/10.3389/fpubh.2022.869238
This article is part of the Research Topic Big Data Analytics for Smart Healthcare applications View all 109 articles
Early diagnosis, prioritization, screening, clustering, and tracking of patients with COVID-19, and production of drugs and vaccines are some of the applications that have made it necessary to use a new style of technology to involve, manage, and deal with this epidemic. Strategies backed by artificial intelligence (A.I.) and the Internet of Things (IoT) have been undeniably effective to understand how the virus works and prevent it from spreading. Accordingly, the main aim of this survey is to critically review the ML, IoT, and the integration of IoT and ML-based techniques in the applications related to COVID-19, from the diagnosis of the disease to the prediction of its outbreak. According to the main findings, IoT provided a prompt and efficient approach to tracking the disease spread. On the other hand, most of the studies developed by ML-based techniques aimed at the detection and handling of challenges associated with the COVID-19 pandemic. Among different approaches, Convolutional Neural Network (CNN), Support Vector Machine, Genetic CNN, and pre-trained CNN, followed by ResNet have demonstrated the best performances compared to other methods.
The outbreak of COVID-19 in Wuhan City, Hubei Province, China, began in December 2019 through the seafood wholesale market (1). Later, on January 30, 2020, the World Health Organization (WHO) declared the prevalence of Covid-19 as an emergency pandemic worldwide (2). Many governments have declared it a dangerous pandemic and imposed full quarantine to prevent the spread of COVID-19. Several countries have reduced their growing infection by tightening quarantine and forcing people to maintain social distance (3). Even if through complete quarantine, they failed to control the COVID-19 completely. Some countries have joined in the medical development to treat COVID-19. However, to date, there is no specific drug to treat COVID-19. However, few drugs have been suggested as potential research therapies. The proposed drug has been studied under WHO-led clinical trials (4). According to several studies, since COVID-19 is a communicable disease, the WHO has stated that complete quarantine could be the only way to prevent COVID-19 (5).
The COVID-19 outbreak has created many challenges in human life worldwide (6). The most devastating impact, increasing casualties and deaths (around the world), has made it clear the need for social and business restrictions (7). With the expansion of the COVID-19 pandemic, the world community has faced many other problems in various aspects of life, such as economic and social life, psychological wellness, political interactions, cultural activities, educational limitations, religious restrictions, and even sports events (8, 9). Such examples highlight the need for effective and intelligent systems to deal with such crises in the pandemic situation (9). Early diagnosis, prioritization, screening, clustering and tracking of patients, and production of drugs and vaccines are some of the applications that have made it necessary to use a new style of technology to involve, manage, and deal with this epidemic (10). Machine Learning (ML) and Artificial intelligence (AI) algorithms displayed promising ability in prediction and classification (11–22) including disease prediction (23–34), virus genome analysis (24, 35, 36), and medical imaging and Internet of Things (37–40). Strategies backed by artificial intelligence (A.I.) and the Internet of Things (IoT) have been undeniable to understand how the virus works and try to prevent it from spreading (9, 41). These techniques have evolved with the development of computing resources with cloud computing and recent advances in ML. These advances enable researchers to process large amounts of data and extract information. ML-based methods used in processing and modeling data on COVID-19 disease can increase efficiency and speed up results by improving computations. Several researchers have moved toward using ML-based techniques for different applications in the COVID-19 dataset, such as classification using C.T. Images (42), chest C.T. Images (43), and X-ray images (44).
Given the diversity of data, applications, and even the multiplicity of machine learning methods, it is necessary to develop a comprehensive survey study that can consider all the strengths and weaknesses in a standard and systematic study. Table 1 presents similar survey studies developed in the field for describing their ability to convey their message on the subject reviewed. Table 1 discusses the study's strengths to find the main research gap.
In one of the early studies, Gou et al. presented a survey to evaluate the ML-based techniques for diagnosing COVID-19 using medical data collection, image preprocessing, feature extraction, and image classification. The study evaluates Transfer, ensemble, unsupervised and semi-supervised learnings, convolutional neural networks, graph neural networks, and explainable deep neural networks. Evaluations focused on the advantages and limitations of the diagnosis techniques (45). Abumalloh et al. presented a state-of-the-art ML-based technique for handling medical image processing in the context of the COVID-19 crisis (46). Khan et al. developed a survey of the applications of A.I. for preventing the COVID-19 pandemic (47). El-Rashidy et al. conducted a review study to describe A.I.'s role in preventing the COVID-19 pandemic using the five applications, including COVID-19 diagnosis, estimation of the COVID-19 outbreak, and patient characteristics, as well as vaccine development (48). Later on, Alballa and Turaiki surveyed the recent articles on ML techniques for COVID-19 diagnosis, mortality rate prediction, and violence risk estimation (49). As can be deduced, many survey studies have been developed. But, the existence of a study that can systematically review and discuss two interrelated areas of the ML and the IoT in the form of an article has been lost from the research literature.
The main contribution of this study is to systematically investigate and analyze the role of ML and the Internet of Medical Things (IoMT) to address the challenges associated with diagnosis of the COVID-19 and its outbreak prediction. Here we comprehensively investigate the merits and shortcomings of the ML and IoMT tools proposed for these tasks and present a numerical and statistical analysis.
There is an urgent need to utilize existing technologies to their full potential. Internet of Things (IoT) and ML is regarded as one of the most trending technologies with great potential in fighting against the coronavirus outbreak. The IoT comprises a scarce network in which the IoT devices sense the environment and send valuable data on the internet. In this review, we examine the current status of IoT applications and ML related to COVID-19, identify their deployment and operational challenges, and suggest possible opportunities to contain the pandemic further.
The IoT provides the materials needed to help the world minimize the effects of COVID-19. The Internet of Things works with a wide range of applications to ensure compliance with health authorities' safety instructions and precautions. The Internet of Things has a scalable network with the potential to deal with the vast amount of data received from sensors used by several programs to combat COVID-19. In addition, reliable IoT networks reduce critical data delivery times, which can help provide a timely response during the global COVID-19 epidemic. Due to the prevalence of the COVID-19, the role of the Internet of Things was never as needed as it is now.
Artificial intelligence (A.I.) is one of the most important and promising technologies that help revolutionize many fields by creating a revolution. The introduction of machine learning algorithms and artificial intelligence to the Internet of Things has opened new doors in this field. Machine learning provides the opportunity to learn and extract meaningful patterns from data. Because IoT device data is collected in a database, it can easily be used to predict the prevalence and effects of the coronavirus and how to reduce it. Data of patients with COVID-19 help predict the future behavior of the virus and regional comparison of its effects. In addition, it also helps with the possible adaptation of COVID-19 symptoms to an effective and rapid A.I. treatment.
The patient's medical record and the results obtained help to predict better treatment choices based on artificial intelligence and machine learning (ML) algorithms and lead to rapid recovery and patient monitoring. Artificial intelligence-based emergency traffic control paves the way for ambulances and other emergency service providers. BlueDot was one of the first artificial intelligence companies to predict the outbreak of the Corona virus and identify its global threat. They provided information on the mobility pattern of the virus and its potential for spread. Other A.I. companies also joined hands to work with COVID-19, including Deargen, Insilico Medicine, and S.R.I. Biosciences and Iktos, Benevolent AI, DeepMind, Nanox, Baidu, Alibaba, and EndoAngel Medical Technology Co.
Here we conclude that there is a gap in how to address the strengths and weaknesses of machine learning and IoT methods that need to be addressed. In the meantime, to close this gap, we will need to classify, determine the pros and cons, challenges and limitations, and outline ways to deal effectively with COVID-19. In line with this basic need to have a deeper insight into the applications and effects of machine learning and the Internet of Things on the COVID-19 Pandemic, we presented research to be able to study these methods in different ways and in a practical way.
Accordingly, the main purpose of this review article is to examine the ML, IoT, and the integration of IoT and ML-based techniques in the applications related to COVID-19 from the diagnosis of the disease to the prediction of its outbreak.
The study has three main sections:
- A section for describing the studies developed by IoT and IoT-ML based techniques in COVID-19 applications.
- A section for presenting the role of ML-based techniques in COVID-19 applications.
- A section for presenting the main findings, challenges, and future perspectives.
A systematic review may provide technical and practical literature for a specific topic (50). A systematic review requires a proper collection of papers on the subject. Preparing a dataset is one of the main steps in determining review work quality (50). According to the P.R.I.S.M.A. guidelines, the present study has collected the most relevant studies from W.O.S. and Scopus libraries (51). There are four steps for preparing the database using the P.R.I.S.M.A. guidelines, including (52): (1) identification, (2) screening, (3) eligibility, and (4) inclusion. In the identification step, we employed the frequently used keywords, including COVID-19, pandemic, diagnosis, detection, Prediction, Monitoring, Classification, Identification, IoT, and Machine learning to search within the article title abstract and keywords. In the first step, about 109 articles have been identified and selected from the Thomson Reuters Web-of-Science (WoS) and Elsevier Scopus. In the Screening step, the duplicate articles have been removed. Twenty-five articles (about 23%) have been removed from the dataset. Thirty nine articles (about 36%) have been eliminated due to the lack of details on the methods, datasets, full text, etc. A total of 45 cases (about 41%) have been included in the screening step. In the eligibility step, the authors team surveyed the full text of the papers, and marked the relevant articles during monitoring eligibility. In this phase, 23 cases (about 22%) have been selected for investigating the evaluation criteria and including in the dataset.
IoT is an interconnected set of computing tools from simple to complex that can be used in conjunction with mechanical or digital machines in the presence of humans, animals, or objects. IoT technology can easily transfer data from the source to the destination through the network without the operator's presence. This technology can be considered a special tool in human-human interaction or human-computer interaction (53, 54). An IoT platform includes the minimum equipment required, such as smart devices equipped with the web (55). These systems consist of processors, sensors, and communication hardware to collect, send, control, manage, and convert data into accessible data (55, 56). These systems connect to an IoT port used to send data to the cloud so that data can be analyzed and shared (57). These devices can operate by connecting to other related systems based on their information (58). These tools perform many of their tasks without human intervention.
Today, IoT technology in health and treatment is growing rapidly (59). The main applications of IoT in the process of treatment and intelligent health can include identification, digitization of medical information, patient transfer to the hospital, use of vital signs sensors, use of smartphones in communication, and digitization of medical processes (60, 61). Furthermore, IoT has become more popular and important due to the COVID-19 pandemic (62). Because this virus is highly contagious and has a high risk to human health, and has caused many problems for the medical staff, using non-contact methods to diagnose as soon as possible, control patients, monitor the condition of patients with acute illness, as well as maintain social distance, can be an important factor in breaking off part of the virus infection cycle (63, 64). In non-contact methods, the IoT is a leader and can solve many problems in this field (65). Figure 1 presents the main applications of the IoT in COVID-19 era.
Table 2 presents the highlighted studies for applying IoT-based techniques to tackle COVID-19. This table represents the studies based on the main four columns. First, the objective briefly describes the main objective of each study. Methodology/proposed algorithm presents the main algorithm and procedure employed by each study. Keyword indicates the main points and concentration of the study and finally, the application section presents the field of the application of each method.
Figure 2 presents the contribution of different applications which are performed by IoT techniques to track COVID-19 related fields.
Figure 2 is generated based on Table 2 to present the main applications and their portions by studies for handling IoT in the COVID-19 pandemic. Monitoring, detection, and diagnosis are the main application of IoT-based techniques in tackling the COVID-19 pandemic. Monitoring can be performed in different ways. Accordingly, Roy et al. employed IoT as a real-time solution for monitoring COVID-19 outbreaks (66). Also, Otoom et al. employed IoT to provide monitoring and detection data using a real-time system to feed to the machine learning algorithms for further applications or handling (69). Singh et al. and Vedaei et al. used IoT as a tool for monitoring COVID-19 patients and their health condition in cooperating with an interconnected network (70, 71). Ashraf et al. proposed a smart edge surveillance system to monitor wearable smart gadgets which are operated according to IoT-based technology (72). Karmore et al. developed a Medical Diagnosis Humanoid to provide a complete diagnostic system for COVID-19 using IoT-based technology (74). De Vito et al. presented the outputs of a high-resolution A.Q. monitoring system based on an IoT-based technique (80).
Baskaran et al. used a non-contact infrared sensor to examine the body temperature to detect the patients with COVID-19 (73). Wang et al. exploited the social relationships in the platform of Social IoT to solve controlling issues of the COVID-19 epidemic by sharing the limited protective resources (77). Kumar et al. investigated an IoT based platform to prevent the spreading of COVID-19 (78). Kolhar et al. developed a platform of a decentralized IoT-based biometric based on a face detection platform for handling COVID-19 outbreaks (79). Aman et al. developed an architecture of IoT based framework for medical applications with respect to combat COVID-19 (38). Manalu et al. investigated the information technology to respond COVID-19 pandemic trend in accordance with the IoT technology (81).
Figure 3 presents the main contribution of these papers. According to the reviewed studies, the COVID-19 dataset can be imported from three main sources, including Radiography, statistics of health centers, and Sensors for prediction, monitoring, identification, detection, diagnosis, and classification purposes. The output of the techniques needs to be evaluated to confirm the approach performance and accuracy values. The frequently used parameters for performance analysis include Accuracy, Precision, Recall, R.M.S.E., Correlation coefficient and mean absolute percentage error. This can be considered a brief explanation of the main contribution of the present study. This study successfully presents the advantages and disadvantages of each technique for a specific task in handling the COVID-19 dataset and proposes the future perspectives. Also, this study can detect the main challenges and limitations.
There is a need to categorize the main applications of IoT and the relevance technique following COVID-19. Table 3 presents the study's main contributions to the application of IoT and integrated IoT-ML-based techniques. Based on Table 3, the exact application of each of the methods used can be extracted. It is also possible to find out which methodology is still available for which application can be considered a research opportunity for the future. Also, by carefully examining the different reasons for the tendency of each method to the fields shown in independent research, which can be considered necessary research and planning opportunities for policymakers in this field.
As shown in Table 3, IoT-based technology requires ML-based techniques to complete the task. Figure 4 presents the share of each methodology in the applications by percentage.
As shown in Figure 4, IoT has been used more than other applications to monitor and detect COVID-19 cases. However, it has been less popular in the identification at the same time.
Utilizing the ML platform led to reducing the adverse effects of the disease and accelerating the healing process (62). The combination of A.I. and ML has led to advances in treatment, medication, screening, prognosis, contact tracking, and the drug/vaccine development process and reduced human intervention in medical performance (82). ML is also used as a tool for managing virtual queues to prevent crowds in physical waiting rooms or long queues. In addition, it is used to predict waiting times and implement calls in a privacy manner in conjunction with the cell phone platform (83).
The ML method is widely used in data analysis by intelligently producing an analytical model. This method is a subset of artificial intelligence that analyzes data and produces a model for estimating, categorizing, optimizing, predicting, identifying problems, and decision-making (84, 85).
New computing technologies have made the problems assessed by ML-based techniques today a little different from the way they are analyzed based on past technologies (86). These techniques began to evolve from pattern recognition to a comprehensive theory of the ability of computers to perform specific tasks without the need for special planning (87, 88).
In the field of medicine and treatment, ML is known as one of the most practical tools for analyzing medical data, identifying, predicting, and even treating different situations. With the advancement of medical science in today's world and the production of large volumes of medical data, there is an urgent need to analyze this data (89). Figure 5 presents the main applications of ML-based techniques for medical science to tackle the COVID-19 pandemic. Identifying the prevalence, effective parameters in the eradication of the virus, identifying patients in the early stages, patients' pattern behaviors, and predicting outbreak and mortality rates can be considered practical and effective areas of ML-based techniques (90, 91).
Table 4 presents the highlighted studies for the application of ML-based techniques for handling COVID-19. Similar to Tables 3, 4 discuss them in four columns. The objective column briefly describes the main objective of each study. Methodology/proposed algorithm presents the main algorithm and procedure employed by each study. Keyword indicates the main points and concentration of the study and finally, application section presents the field of the application of each method.
According to Table 4, ML-based techniques are employed for detection, identification, monitoring, diagnosis, prediction, and classification purposes in the presence of the COVID-19 dataset. Figure 6 presents the summary of each application separately. Singh and Kaur employed an ML-based platform using hybrid random forest, Gaussian Naïve Bayes, and Generative adversarial network as a healthcare application to detect COVID-19 cases (93). Vinod et al. developed a pre-trained CNN method as an ML-based technique integrated using C.T. scan and chest x-ray images to identify, detect, and predict the positive infected patients (94). Ardakani et al. developed an ML-based technique (ResNet) for fast diagnosis of COVID-19 cases compared to radiology data (96). Polsinelli et al. developed a study to detect COVID-19 promptly using CNN as a frequently used DL-based architecture (97). Nour et al. proposed an intelligence computer-aided model based on CNN with SVM classifier architecture on chest X-rays to support daily clinical applications (98). Chowdhury et al. investigated the utility of A.I. in the rapid and accurate detection of COVID-19 in the presence of chest X-ray images (102). Sethi et al. employed Logistic Regression (L.R.), Multinomial Naïve Bayes, Decision Tree (D.T.), Random Forest (R.F.), SVM, and XGBoost classifiers to analyze the emotions expressed by people using social media to monitor and detect sentiment behind COVID-19 (110). Ardabili et al. developed ML-based techniques for the prediction of COVID-19 outbreaks (91). In another study, Ardabili et al. also employed a hybrid ML-based technique (Multilayered perceptron integrated by gray wolf optimizer) for the global prediction of COVID-19 cases (90). In addition, Loey et al. employed the DL-based ResNet method in the presence of an SVM-based classifier to detect a masked face (92).
According to Figure 6, detection, diagnosis, and prediction can be considered as the main categories of the application of ML-based methods in COVID-19. In general, one of the main sections of analyzing IoT-based and ML-Based techniques applied for a specific field is their evaluation in terms of accuracy, error, or in other word performance of the model. Table 5 presents the evaluation criteria employed for each model.
According to Table 5, accuracy, followed by the recall and precision parameters has owned the highest portion of the evaluation criteria employed for analyzing COVID-19 based dataset using IoT and ML-based techniques. In the following, Table 6 is generated from Table 4 for indicating the share of each ML-based technique for each application and their main contributions. According to Table 6, ResNet as an architecture of deep learning methods followed by CNN, XGBoost, SVM, D.T., and L.R. has been used more often to tackle work with COVID-19 related data.
Figure 7 presents the share of different ML methods for different tasks to tackle the COVID-19 pandemic. As is clearly indicated in this figure, ResNet, followed by CNN, is the most common application of ML in this field. This can be due to the model's nature for handling different applications like monitoring, detection, identification, classification, and diagnosis. In comparison, other methods can do a limited number of applications.
Models developed using ML and IoT-ML require an evaluation step for recognizing their performance and accuracy values. According to the studies reviewed, the most effective and frequently used evaluation criteria are including Accuracy, Recall, Precision, Root mean square error (R.M.S.E.), Correlation coefficient and Mean absolute percentage error (M.A.P.E.). These criteria compare the models' output and actual values and provide a comparison score (90, 91). In the present study, we employed the criteria values reported by each study for evaluating and comparing the models. Table 7 presents the main criteria for evaluation.
This section presents the main findings of IoT based techniques (Table 8) and ML-based techniques (Table 9). Each table includes two main columns called findings and pros. and cons.
According to Table 8, most of these studies lack numerical analysis for the method's performance. One of the main reasons can be the nature of the IoT technique, which goes through a practical process and shows its performance in practical applications and does not need to provide numerical statistics. In all these applications, IoT could successfully cope with the task. IoT provided a fast and efficient approach to tracking the disease spread (66). On the other hand, it can be employed as a real-time framework to minimize the impact of communicable diseases through the early detection of cases (67). In the study by Singh et al. IoT technology successfully increased patient satisfaction and reduced the readmission rate in the hospital (70). However, there is a need to integrate IoT platforms with ML-based techniques for detection purposes. In the study by Rahman et al. DL applications with IoT platforms provided promising findings to detect A.E. attacks. However, there is a need for further research, attention, and implementation of appropriate defense mechanisms, safeguards, and controls (67). Kolhar et al. employed Multi-task Cascaded Convolutional Network architecture (M.C.C.N.N.), and findings claimed that the efficiently integrated by Raspberry Pi increased the robustness of detection and recognition (79).
According to the findings given in Table 9, the most share of studies developed by ML-based techniques for handling COVID-19 based datasets provided performance criteria. The most share of the performance criteria, according to Figure 8 is related to the accuracy factor. Accuracy factor is a general and normalized factor. Therefore, it can be employed for comparing the ML-based methods with different datasets. Figure 9 presents the accuracy values for each model for comparing their performance in handling the COVID-19 dataset.
Figure 9 indicates CNN with SVM classifier, Genetic CNN, and pre-trained CNN followed by ResNet, provided highest accuracy values. On the other hand, the lowest accuracy was related to single CNN followed by XGboost and K.N.N. techniques.
Nowadays, when the world is struggling with COVID-19 disease, every innovation and technology is used to fight this disease. Like many other areas, healthcare requires the support of new technologies such as IoT, and ML. Exploring the disease-related dataset, data preparation, prevention, and control of infectious diseases has become one of the main purposes of A.I. IoT and ML have a vital personality in better understanding, dealing with the COVID-19 crisis, and discovering the COVID-19 vaccine. ML-based technology allows computers to predict the pattern and speed of disease transmission with their intelligence and by mimicking large amounts of data. A.I. uses information from people with coronary heart disease, and improved and dead people as tracking data.
To combat the spread of the corona virus, IoT-based methods of communicating with patients provide transparency and a better understanding of how the virus is spread and strengthen the treatment and research process. ML is one of the new technologies for tracking the spread of the virus and finding effective parameters in it. The ML method can successfully identify high-risk patients and predict the necessary measures to deal with possible infections to reduce the point of the effect of the disease. In addition, ML-based methods can estimate the risk of patient mortality through previous analysis. This technique improves patients' planning, treatment, and reduction and is a complementary medical tool that works with data and evidence. On the other hand, this technology improves decision-making and reduces the cost of treatment and diagnosis. At the same time, in medical imaging, ML tools help to recognize the patterns in the images and strengthen the ability of radiologists to diagnose the possibility of disease and early diagnosis of the disease.
One of the main limitations of IoT, and ML-based techniques for applications in COVID-19 is the lack of a complete dataset. This can be due to the unique development of models by limited data for a specific application within the same data field. The purpose of using IoT, A.I., or ML-based techniques is to solve a specific problem in the real world with a real application that requires the use of special hardware and equipment. There are limitations in the cost and availability of developing and equipping communication hardware in therapeutic, diagnostic, estimation, and forecasting applications for IoT technology or ML-based techniques. Also, there are limited best practices available for IoT developers. The lack of IoT edge authentication and licensing standards has led to restrictions on the application and enactment of laws, regulations, and policies in the use of this technology, and this has led to the absence of IoT-based incident response activities as the best methods. All of these limitations mean that there is still no focus on identifying ways to gain situational awareness of the security of IoT assets in a medical complex.
According to the reviewed studies, the COVID-19 dataset can be imported from three primary sources: radiography, health centers' statistics, and Sensors for prediction, monitoring, identification, detection, diagnosis, and classification purposes. The output of the techniques needs to be evaluated to confirm the approach performance and accuracy values. The frequently used parameters for performance analysis include Accuracy, Precision, Recall, R.M.S.E., Correlation coefficient, and mean absolute percentage error. This can be considered a brief explanation as the main contribution of the present study. This study successfully presents the advantages and disadvantages of each technique for a specific task in handling the COVID-19 dataset and proposes future perspectives. Also, this study can detect the main challenges and limitations.
It is also possible to find out which methodology is still available for which application can be considered a research opportunity for the future. Also, by carefully examining the different reasons for the tendency of each method to the fields shown in independent research, which can be considered necessary research and planning opportunities for policymakers in this field.
The presence of the ML platform led to reducing the adverse effects of the disease and accelerating the healing process, advances in treatment, medication, screening, prognosis, contact tracking, and the drug/vaccine development process, and reduced human intervention in medical performance as a tool for the management of virtual queues to prevent crowds in physical waiting rooms or long queues. It is used to predict waiting times and implement calls privately with the cell phone platform.
Based on the studies conducted in this study, we achieved the following results:
• IoT has been used more than other applications to monitor and detect COVID-19 cases. In contrast, it has been less popular in the identification.
• ML method is widely used in data analysis by producing an analytical model intelligently for estimating, categorizing, optimizing, predicting, identifying problems, and decision making.
• New computing technologies have made the problems assessed by ML-based techniques, began to evolve from pattern recognition to a comprehensive theory of the ability of computers to perform specific tasks without the need for special planning.
• Identifying the prevalence, effective parameters in eradicating the virus, identifying patients in the early stages, patients' pattern behaviors, and predicting outbreak and mortality rates can be considered practical and compelling areas of ML-based techniques.
• Detection, diagnosis, and prediction can be considered the main categories of the application of ML-based methods in COVID-19. In general, one of the main sections of analyzing IoT-based and ML-Based techniques applied for a specific field is their evaluation in terms of accuracy, error, or performance of the model.
• Accuracy, followed by the recall and precision parameters, has the highest portion of the evaluation criteria employed for analyzing the COVID-19 dataset using IoT and ML-based techniques. ResNet, as an architecture of deep learning methods followed by CNN, XGBoost, SVM, D.T., and L.R., has been used more often to tackle work with COVID-19 related data.
• Resnet follows CNN is The most common use of ML to contribute various methods for different tasks to combat Pandemic COVID-19. This trend can be due to the model's nature for handling different applications like monitoring, detection, identification, classification, and diagnosis. At the same time, other methods can do a limited number of applications.
• Models developed using ML and IoT-ML require an evaluation step for recognizing their performance and accuracy values. According to the studies reviewed, the most effective and frequently used evaluation criteria include Accuracy, Recall, Precision, Root mean square error (R.M.S.E.), Correlation coefficient, and Mean absolute percentage error (M.A.P.E.). These criteria compare the models' output and actual values and provide a comparison score (90, 91). In the present study, we employed the criteria values reported by each study for evaluating and comparing the models.
• Most of these studies lack numerical analysis for the method's performance. One of the main reasons can be the nature of the IoT technique, which goes through a practical process and shows its performance in practical applications and does not need to provide numerical statistics. In all these applications, IoT could successfully cope with the task. IoT provided a fast and efficient approach to tracking the disease spread. On the other hand, it can be employed as a real-time framework to minimize the impact of communicable diseases through the early detection of cases.
• The most share of studies developed by ML-based techniques for handling COVID-19 based dataset provided performance criteria. The most share of the performance criteria is related to the accuracy factor. The accuracy factor is general and normalized. Therefore, it can be employed for comparing the ML-based methods with different datasets.
• CNN, SVM classifier, Genetic CNN, and pre-trained CNN followed by ResNet provided the highest accuracy values. On the other hand, the lowest accuracy was related to single CNN, followed by XGboost and K.N.N. techniques.
The present study categorizes the applications of IoT, IoT-ML, and ML-based techniques to tackle COVID-19-related problems. The main applications are monitoring, detection, identification, classification, and diagnosis. Studying, comparing, and investigating these applications requires a proper judgment about the performance and effectiveness of outputs. According to a deep consideration of the evaluation criteria, it has been investigated that the accuracy, followed by the recall and precision parameters, have owned the highest portion of the evaluation criteria employed for analyzing COVID-19 based dataset using IoT and ML-based techniques.
Most of the studies lack numerical analysis for the method performance. One of the main reasons can be the nature of the IoT technique which goes through a practical process and shows its performance in practical applications. In all the applications, IoT could successfully cope with the tasks. Such that, IoT provided a fast and efficient approach to tracking the disease spread. Most of the studies developed by ML-based techniques for handling COVID-19-based datasets provided performance criteria. According to the results section, the following points can be extracted:
- IoT provided a fast and efficient approach to tracking the disease spread.
- IoT can be employed as a real-time framework to minimize the impact of communicable diseases through early detection of cases.
- The most popular performance criteria are related to the accuracy factor.
- ML-based methods are able to be used with different types of datasets.
- CNN with SVM classifier, Genetic CNN, and pre-trained CNN followed by ResNet, provided the highest accuracy values.
- A.I. is a result-oriented technology employed for proper screening, analysis, forecasting, and tracking of current and potential future patients.
Policy-making in COVID-19 disease to examine the weaknesses and strengths and vulnerabilities of society in terms of the penetration of pathogenic viruses can be considered additional measures and future studies. On the other hand, the study of collective behaviors can also be considered as a perspective to complete studies to prevent similar social harms, reduce costs incurred, and not surprise human life. The future perspective is to employ an advanced analytic ML-based platform that supports huge-data analytics. This trend moves toward smart health interconnected with innovative technologies in the sensor industry. The future is waiting for tremendous promotion in smart health.
The original contributions presented in the study are included in the article/supplementary material, further inquiries can be directed to the corresponding author/s.
SB and SA designed the study. SA, AY, BP, AK, and AM wrote the paper. SB, AB, HA-R, MM, and AK edited the manuscript. SA, AY, and BP carried out all the analyses. SB, SA, AY, BP, and MM generated all figures and all tables. HA-R and AB were not involved in any analyses. All authors have read and approved the final version of the paper.
The authors declare that the research was conducted in the absence of any commercial or financial relationships that could be construed as a potential conflict of interest.
All claims expressed in this article are solely those of the authors and do not necessarily represent those of their affiliated organizations, or those of the publisher, the editors and the reviewers. Any product that may be evaluated in this article, or claim that may be made by its manufacturer, is not guaranteed or endorsed by the publisher.
1. Yuki K, Fujiogi M, Koutsogiannaki S. COVID-19 pathophysiology: a review. Clin Immunol. (2020) 215:108427. doi: 10.1016/j.clim.2020.108427
2. Thanh Le T, Andreadakis Z, Kumar A, Gómez Román R, Tollefsen S, Saville M, et al. The COVID-19 vaccine development landscape. Nat Rev Drug Discov. (2020) 19:305–6. doi: 10.1038/d41573-020-00073-5
3. Haug N, Geyrhofer L, Londei A, Dervic E, Desvars-Larrive A, Loreto V, et al. Ranking the effectiveness of worldwide COVID-19 government interventions. Nat Hum Behav. (2020) 4:1303–12. doi: 10.1038/s41562-020-01009-0
4. Marshall JC, Murthy S, Diaz J, Adhikari N, Angus DC, Arabi YM, et al. A minimal common outcome measure set for COVID-19 clinical research. Lancet Infect Dis. (2020) 20:e192–7. doi: 10.1016/S1473-3099(20)30483-7
5. Cao X. COVID-19: immunopathology and its implications for therapy. Nat Rev Immunol. (2020) 20:269–70 doi: 10.1038/s41577-020-0308-3
6. Ciotti M, Ciccozzi M, Terrinoni A, Jiang WC, Wang CB, Bernardini S. The COVID-19 pandemic. Crit Rev Clin Lab Sci. (2020) 57:365–88. doi: 10.1080/10408363.2020.1783198
7. Mahmoudi MR, Heydari MH, Qasem SN, Mosavi A, Band SS. Principal component analysis to study the relations between the spread rates of COVID-19 in high risks countries. Alexandria Eng J. (2021) 60:457–64. doi: 10.1016/j.aej.2020.09.013
8. Kumar A, Gupta PK, Srivastava A. A review of modern technologies for tackling COVID-19 pandemic. Diabetes Metab Syndr. (2020) 14:569–73. doi: 10.1016/j.dsx.2020.05.008
9. Vaishya R, Javaid M, Khan IH, Haleem A. Artificial Intelligence (AI) applications for COVID-19 pandemic. Diabetes Metab Syndr. (2020) 14:337–9. doi: 10.1016/j.dsx.2020.04.012
10. Mendhe D, Wankhede P, Wanjari M, Alwadkar S. Mucormycotic osteomyelitis of maxilla post-COVID patient: a case report. Pan Afr Med J. (2021) 39:275. doi: 10.11604/pamj.2021.39.275.30480
11. Ahmadinia M, Ahangarikiasari H. Data aggregation in wireless sensor networks based on environmental similarity: a learning automata approach. J Netw. (2014) 9:2567. doi: 10.4304/jnw.9.10.2567-2573
12. Ahmadinia M, Meybodi MR, Esnaashari M. Energy-efficient and multi-stage clustering algorithm in wireless sensor networks using cellular learning automata. IETE J Res. (2013) 59:774–82. doi: 10.4103/0377-2063.126958
13. Alinejad-Rokny H, Pourshaban H, Orimi AG. Network motifs detection strategies and using for bioinformatic networks. J Bionanosci. (2014) 8:353–9. doi: 10.1166/jbns.2014.1245
14. Bahrani P, Minaei-Bidgoli B, Parvin H, Mirzarezaee M, Keshavarz A. User and item profile expansion for dealing with cold start problem. J Intell Fuzzy Syst. (2020) 38:4471–83. doi: 10.3233/JIFS-191225
15. Mahmoudi MR, Akbarzadeh H, Parvin H, Nejatian S, Rezaie V. Consensus function based on cluster-wise two level clustering. Artif Intell Rev. (2021) 54:639–65. doi: 10.1007/s10462-020-09862-1
16. Niu H, Khozouie N, Parvin H, Beheshti A. An ensemble of locally reliable cluster solutions. Appl Sci. (2020) 10:1891. doi: 10.3390/app10051891
17. Niu H, Xu W, Akbarzadeh H, Parvin H, Beheshti A. Deep feature learnt by conventional deep neural network. Comput Elect Eng. (2020) 84:106656. doi: 10.1016/j.compeleceng.2020.106656
18. Parvin H. Behrouz Minaei, Hosein Alizadeh, and Akram Beigi. A novel classifier ensemble method based on class weightening in huge dataset. In: International Symposium on Neural Networks. (2011). p. 144–150
19. Parvin H, Parvin S. A classifier ensemble of binary classifier ensembles. Int J Learn Manage Syst. (2013) 1:37–47. doi: 10.12785/ijlms/010204
20. Parvin H. MirnabiBaboli, M. Proposing a classifier ensemble framework based on classifier selection and decision tree. Eng Appl Artif Intell. (2015) 37:34–42. doi: 10.1016/j.engappai.2014.08.005
21. Alinejad-Rokny H, Pedram MM. Discovered motifs with using parallel Mprefixspan method. Sci Res Essays. (2011) 6:4220–6. doi: 10.5897/SRE11.212
22. Parvin H, Seyedaghaee N. A heuristic scalable classifier ensemble of binary classifier ensembles. J Bioinform Intell Control. (2012) 1:163–70. doi: 10.1166/jbic.2013.1016
23. Alinejad-Rokny H. Proposing on optimized homolographic motif mining strategy based on parallel computing for complex biological networks. J Med Imag Health Inform. (2016) 6:416–24. doi: 10.1166/jmihi.2016.1707
24. Alinejad-Rokny H, Anwar F, Waters SA, Davenport MP. Source of CpG depletion in the HIV-1 genome. Mol Biol Evol. (2016) 33:3205–12. doi: 10.1093/molbev/msw205
25. Alinejad-Rokny H, Sadroddiny E. Machine learning and data mining techniques for medical complex data analysis. Neurocomputing. (2018) 276. doi: 10.1016/j.neucom.2017.09.027
26. Bayati M, Rabiee HR, Mehrbod M, Vafaee F, Ebrahimi D, Forrest AR. CANCERSIGN: a user-friendly and robust tool for identification and classification of mutational signatures and patterns in cancer genomes. Sci Rep. (2020) 10:1–11. doi: 10.1038/s41598-020-58107-2
27. Dashti H, Dehzangi A, Bayati M, Breen J, Lovell N, Ebrahimi D. Integrative analysis of mutated genes and mutational processes reveals seven colorectal cancer subtypes. BMC Bioinform. (2022) 23:1–24. doi: 10.1186/s12859-022-04652-8
28. Ghareyazi A, Mohseni A, Dashti H, Beheshti A, Dehzangi A, Rabiee HR. Whole-genome analysis of de novo somatic point mutations reveals novel mutational biomarkers in pancreatic cancer. Cancers. (2021) 13:4376. doi: 10.3390/cancers13174376
29. Heidari R, Akbariqomi M, Asgari Y, Ebrahimi D. A systematic review of long non-coding RNAs with a potential role in Breast Cancer. Mut Res Rev Mut Res. (2021) 787:108375. doi: 10.1016/j.mrrev.2021.108375
30. Javanmard R, JeddiSaravi K. Proposed a new method for rules extraction using artificial neural network and artificial immune system in cancer diagnosis. J Bionanosci. (2013) 7:665–72. doi: 10.1166/jbns.2013.1160
31. Kalantari AK. Computational intelligence approaches for classification of medical data: State-of-the-art, future challenges and research directions. Neurocomputing. (2018) 276:2–22. doi: 10.1016/j.neucom.2017.01.126
32. Shamshirband S, Fathi M, Dehzangi A, Chronopoulos AT. A review on deep learning approaches in healthcare systems: Taxonomies, challenges, and open issues. J Biomed Inform. (2021) 113:103627. doi: 10.1016/j.jbi.2020.103627
33. Shamshirband S, Rabczuk T, Chau KW. A survey of deep learning techniques: application in wind and solar energy resources. IEEE Access. (2019)7:164650–66. doi: 10.1109/ACCESS.2019.2951750
34. Khakmardan S, Rezvani M, Pouyan AA, Fateh M. MHiC, an integrated user-friendly tool for the identification and visualization of significant interactions in Hi-C data. BMC Genom. (2020) 21:1–10. doi: 10.1186/s12864-020-6636-7
35. Rajaei P, Jahanian KH, Beheshti A, Band SS, Dehzangi A. VIRMOTIF: a user-friendly tool for viral sequence analysis. Genes. (2021) 12:186. doi: 10.3390/genes12020186
36. Gooneratne SL, Alinejad-Rokny H, Ebrahimi D, Bohn PS, Wiseman RW, O'Connor DH, et al. Linking pig-tailed macaque major histocompatibility complex class I haplotypes and cytotoxic T lymphocyte escape mutations in simian immunodeficiency virus infection. J Virol. (2014) 88:14310–25. doi: 10.1128/JVI.02428-14
37. Sharifrazi D, Alizadehsani R, Joloudari JH, Shamshirband S, Hussain S, Sani ZA. CNN-KCL: Automatic myocarditis diagnosis using convolutional neural network combined with k-means clustering. Preprint. (2020). doi: 10.20944/preprints202007.0650.v1
38. Aman AH, Hassan WH, Sameen S, Attarbashi ZS, Alizadeh M, Latiff LA, et al. IoMT amid COVID-19 pandemic: Application, architecture, technology, and security. J Netw Comput Appl. (2020) 174:102886. doi: 10.1016/j.jnca.2020.102886
39. Bekhet S, Hassaballah M, Kenk MA, Hameed MA. An Artificial Intelligence Based Technique for COVID-19 Diagnosis from Chest X-Ray. (2020). p. 191–5.
40. Heidari M, Mirniaharikandehei S, Khuzani AZ, Danala G, Qiu Y, Zheng B. Improving the performance of CNN to predict the likelihood of COVID-19 using chest X-ray images with preprocessing algorithms. Int J Med Inform. (2020) 144:104284. doi: 10.1016/j.ijmedinf.2020.104284
41. Sufian A, Jat DS, Banerjee A, Sufian A, Jat DS, Banerjee A.(2020) Insights of artificial intelligence to stop spread of covid-19. In: Big Data Analytics and Artificial Intelligence Against COVID-19: Innovation Vision and Approach. Cham: Springer. p. 177–90. doi: 10.1007/978-3-030-55258-9_11
42. Barstugan M, Ozkaya U, Ozturk S. Coronavirus (covid-19) classification using ct images by machine learning methods. arXiv preprint. arXiv:2003.09424 (2020)
43. Özkaya U, Öztürk S, Budak S, Melgani F, Polat K. Classification of COVID-19 in chest CT images using convolutional support vector machines. arXiv preprint. arXiv:2011.05746 (2020)
44. Öztürk S, Özkaya U, Barstugan M. Classification of Coronavirus (COVID-19) from X-ray and CT images using shrunken features. Int J Imaging Syst Technol. (2021) 31:5–15. doi: 10.1002/ima.22469
45. Guo X, Zhang YD, Lu S, Lu Z. A survey on machine learning in COVID-19 diagnosis. CMES-Computer Modeling in Engineering and Sciences. (2021). doi: 10.32604/cmes.2022.017679
46. Abumalloh RA, Nilashi M, Yousoof Ismail M, Alhargan A, Alghamdi A, Alzahrani AO, et al. Medical image processing and COVID-19: a literature review and bibliometric analysis. J Infect Public Health. (2022) 15:75–93. doi: 10.1016/j.jiph.2021.11.013
47. Khan M, Mehran MT, Haq ZU, Ullah Z, Naqvi SR, Ihsan M, et al. Applications of artificial intelligence in COVID-19 pandemic: a comprehensive review. Exp Syst Appl. (2021) 185:115695. doi: 10.1016/j.eswa.2021.115695
48. El-Rashidy N, Abdelrazik S, Abuhmed T, Amer E, Ali F, Hu J-W, et al. Comprehensive survey of using machine learning in the COVID-19 pandemic. Diagnostics (Basel). (2021) 11:1155. doi: 10.3390/diagnostics11071155
49. Alballa N, Al-Turaiki I. Machine learning approaches in COVID-19 diagnosis, mortality, and severity risk prediction: a review. Inform Med Unlocked. (2021) 24:100564. doi: 10.1016/j.imu.2021.100564
50. Koutsos TM, Menexes GC, Dordas CA. An efficient framework for conducting systematic literature reviews in agricultural sciences. Sci Total Environ. (2019) 682:106–17. doi: 10.1016/j.scitotenv.2019.04.354
51. Fleming PS, Koletsi D, Pandis N. Blinded by PRISMA: are systematic reviewers focusing on PRISMA and ignoring other guidelines? PLoS ONE. (2014) 9:e96407. doi: 10.1371/journal.pone.0096407
52. Page MJJE, McKenzie PM, Bossuyt I, Boutron TC, Hoffmann CD, Mulrow L, et al. The PRISMA 2020 statement: an updated guideline for reporting systematic reviews. BMJ. (2021) 372.
53. Bandyopadhyay D, Sen J. Internet of things: applications and challenges in technology and standardization. Wire Pers Commun. (2011) 58:49–69. doi: 10.1007/s11277-011-0288-5
54. Zeinab KA, Elmustafa SA. Internet of things applications, challenges and related future technologies. World Sci News. (2017) 67:126–48.
55. Keramidas G, Voros N, Hübner M. Components and Services for IoT Platforms. Cham: Springer. (2016). doi: 10.1007/978-3-319-42304-3
56. Adryan B, Obermaier D, Fremantle P. The Technical Foundations of IoT. Washington, DC: Artech House. (2017).
57. Nagaraj. Introduction to Sensors in IoT and Cloud Computing Applications. Potomac: Bentham Science Publishers (2021).
58. Rahman MA, Asyhari AT. The emergence of Internet of Things (IoT): connecting anything, anywhere. Computers. (2019) 8:40. doi: 10.3390/computers8020040
59. Boumehrez F, Sahour AH, Doghmane N. Telehealth care enhancement using the internet of things technology. Bull Electr Eng Inform. (2021) 10:2652–60. doi: 10.11591/eei.v10i5.2968
60. Bolhasani H, Mohseni M, Rahmani AM. Deep learning applications for IoT in health care: a systematic review. Inform Med Unlocked. (2021) 23:100550. doi: 10.1016/j.imu.2021.100550
61. Marques G, Bhoi AK, Victor Hugo C, Hareesha K. IoT in Healthcare and Ambient Assisted Living. Cham: Springer. (2021). doi: 10.1007/978-981-15-9897-5
62. Chamola V, Hassija V, Gupta V, Guizani M. comprehensive review of the COVID-19 pandemic and the role of IoT, drones, AI, blockchain, and 5G in managing its impact. IEEE Access. (2020) 8:90225–65. doi: 10.1109/ACCESS.2020.2992341
63. Taylor W, Abbasi QH, Dashtipour K, Ansari S, Shah SA, Khalid A, et al. Review of the state of the art in non-contact sensing for COVID-19. Sensors. (2020) 20:5665. doi: 10.3390/s20195665
64. Khan MB, Zhang Z, Li L, Zhao W, Hababi MAMA, Yang X, et al. A systematic review of non-contact sensing for developing a platform to contain COVID-19. Micromachines. (2020) 11:912. doi: 10.3390/mi11100912
65. Mohammed B, Isa SM. The role of internet of things (IoT) in the containment and spread of the novel COVID-19 pandemic. In: Computational Intelligence Methods in COVID-19: Surveillance, Prevention, Prediction and Diagnosis. Cham: Springer (2021). p. 109–19. doi: 10.1007/978-981-15-8534-0_6
66. Roy A, Kumbhar FH, Dhillon HS, Saxena N, Shin SY, Singh S. Efficient monitoring and contact tracing for COVID-19: a smart IoT-based framework. IEEE Internet Things Mag. (2020) 3:17–23. doi: 10.1109/IOTM.0001.2000145
67. Rahman A, Hossain MS, Alrajeh NA, Alsolami F. Adversarial examples—Security threats to COVID-19 deep learning systems in medical IoT devices. IEEE Int Things J. (2020) 8:9603–10. doi: 10.1109/JIOT.2020.3013710
68. Ahmed I, Ahmad A, Jeon G. An IoT-based deep learning framework for early assessment of COVID-19. IEEE Internet Things J. (2020) 8:15855–62 doi: 10.1109/JIOT.2020.3034074
69. Otoom M, Otoum N, Alzubaidi MA, Etoom Y, Banihani R. An IoT-based framework for early identification and monitoring of COVID-19 cases. Biomed Signal Process Control. (2020) 62:102149. doi: 10.1016/j.bspc.2020.102149
70. Singh RP, Javaid M, Haleem A, Suman R. Internet of things (IoT) applications to fight against COVID-19 pandemic. Diabetes Metab Syndr Clin Res Rev. (2020) 14:521–4. doi: 10.1016/j.dsx.2020.04.041
71. Vedaei SS, Fotovvat A, Mohebbian MR, Rahman GM, Wahid KA, Babyn P, et al. an IoT-based system for automated health monitoring and surveillance in post-pandemic life. IEEE Access. (2020) 8:188538. doi: 10.1109/ACCESS.2020.3030194
72. Ashraf MU, Hannan A, Cheema SM, Ali Z, Alofi A. Detection and Tracking Contagion using IoT-Edge Technologies: Confronting COVID-19 Pandemic. (2020). p. 1–6.
73. Baskaran P, Rajaram B, Kumaratharan N. IoT Based COVID Preventive System for Work Environment. (2020). p. 65–71. doi: 10.1109/I-SMAC49090.2020.9243471
74. Karmore S, Bodhe R, Al-Turjman F, Kumar RL, Pillai S. IoT based humanoid software for identification and diagnosis of Covid-19 suspects. IEEE Sens J. (2020) doi: 10.1109/JSEN.2020.3030905
75. Akhund TMNU, Jyoty WB, Siddik MAB, Newaz NT, Al Wahid SA, Sarker MM. IoT Based Low-Cost Robotic Agent Design for Disabled and Covid-19 Virus Affected People. (2020). p. 23–6.
76. Ndiaye M, Oyewobi SS, Abu-Mahfouz AM, Hancke GP, Kurien AM, Djouani K. IoT in the wake of COVID-19: a survey on contributions, challenges and evolution. IEEE Access. (2020) 8:186821–39. doi: 10.1109/ACCESS.2020.3030090
77. Wang B, Sun Y, Duong TQ, Nguyen LD, Hanzo L. Risk-aware identification of highly suspected COVID-19 cases in social IoT: a joint graph theory and reinforcement learning approach. IEEE Access. (2020) 8:115655–61. doi: 10.1109/ACCESS.2020.3003750
78. Kumar K, Kumar N, Shah R. Role of IoT to avoid spreading of COVID-19. Int J Intell Netw. (2020) 1:32–5. doi: 10.1016/j.ijin.2020.05.002
79. Kolhar M, Al-Turjman F, Alameen A, Abualhaj MM. three layered decentralized IoT biometric architecture for city lockdown during COVID-19 outbreak. IEEE Access. (2020) 8:163608–17. doi: 10.1109/ACCESS.2020.3021983
80. De Vito S, Esposito E, D'Elia G, Del Giudice A, Fattoruso G, Ferlito S, et al. High Resolution Air Quality Monitoring with IoT Intelligent Multisensor devices during COVID-19 Pandemic Phase 2 in Italy. (2020). p. 1–6.
81. Manalu EP, Muditomo A, Adriana D, Trisnowati Y. Role of Information Technology for Successful Responses to Covid-19 Pandemic. (2020). p. 415–20.
82. Lalmuanawma S, Hussain J, Chhakchhuak L. Applications of machine learning and artificial intelligence for Covid-19 (SARS-CoV-2) pandemic: a review. Chaos Solitons Fractals. (2020) 139:110059. doi: 10.1016/j.chaos.2020.110059
83. Kyritsis I, Deriaz M. A Queue Management Approach for Social Distancing and Contact Tracing. (2020). p. 66–8. doi: 10.1109/AI4I49448.2020.00022
84. Jordan I, Mitchell TM. Machine learning: Trends, perspectives, and prospects. Science. (2015) 349:255–60. doi: 10.1126/science.aaa8415
86. Kashyap P. Machine Learning for Decision Makers: Cognitive Computing Fundamentals for Better Decision Making. Berlin: Springer. (2017). doi: 10.1007/978-1-4842-2988-0
87. Abdulkareem H, Mohammed MA, Gunasekaran SS, Al-Mhiqani MN, Mutlag AA, Mostafa SA, et al. A review of fog computing and machine learning: concepts, applications, challenges, and open issues. IEEE Access. (2019) 7:153123–40. doi: 10.1109/ACCESS.2019.2947542
89. Kolachalama VB, Garg PS. Machine learning and medical education. NPJ digital medicine. (2018) 1:1–3. doi: 10.1038/s41746-018-0061-1
90. Ardabili S, Mosavi A, Band SS, Varkonyi-Koczy AR. Coronavirus Disease (COVID-19) Global Prediction Using Hybrid Artificial Intelligence Method of ANN Trained with Grey Wolf Optimizer. (2020). p. 000251–4 doi: 10.34055/osf.io/vfcex
91. Ardabili SF, Mosavi A, Ghamisi P, Ferdinand F, Varkonyi-Koczy AR, Reuter U, et al. Covid-19 outbreak prediction with machine learning. Algorithms. (2020) 13:249. doi: 10.3390/a13100249
92. Loey GM. A hybrid deep transfer learning model with machine learning methods for face mask detection in the era of the COVID-19 pandemic. Measurement. (2021) 167:108288. doi: 10.1016/j.measurement.2020.108288
93. Singh P, Kaur R. An integrated fog and Artificial Intelligence smart health framework to predict and prevent COVID-19. Global Trans. (2020) 2:283–92. doi: 10.1016/j.glt.2020.11.002
94. Vinod N, Prabaharan SJC. Solitons, and Fractals. Data science and the role of Artificial Intelligence in achieving the fast diagnosis of Covid-19. Chaos Solitons Fractals. (2020) 140:110182. doi: 10.1016/j.chaos.2020.110182
95. Karthik R, Menaka R, Hariharan M. Learning distinctive filters for COVID-19 detection from chest X-ray using shuffled residual CNN. Appl Soft Comput. (2021) 99:106744. doi: 10.1016/j.asoc.2020.106744
96. Ardakani AA, Kanafi AR, Acharya UR, Khadem N, Mohammadi A. Application of deep learning technique to manage COVID-19 in routine clinical practice using CT images: Results of 10 convolutional neural networks. Comput Biol Med. (2020) 121, 103795. doi: 10.1016/j.compbiomed.2020.103795
97. Polsinelli M, Cinque L, Placidi G. light CNN for detecting COVID-19 from CT scans of the chest. Pattern Recognit Lett. (2020) 140:95–100. doi: 10.1016/j.patrec.2020.10.001
98. Nour M, Cömert Z, Polat K. novel medical diagnosis model for COVID-19 infection detection based on deep features and Bayesian optimization. Appl Soft Comput. (2020) 97:106580. doi: 10.1016/j.asoc.2020.106580
99. Han Z, Wei B, Hong Y, Li T, Cong J, Zhu X, et al. Accurate screening of COVID-19 using attention-based deep 3D multiple instance learning. IEEE Trans Med Imaging. (2020) 39:2584–94. doi: 10.1109/TMI.2020.2996256
100. Marques G, Agarwal D. de la Torre Díez I. Automated medical diagnosis of COVID-19 through EfficientNet convolutional neural network. Appl Soft Comput. (2020) 96:106691. doi: 10.1016/j.asoc.2020.106691
101. Mohammed MA, Abdulkareem KH, Al-Waisy AS, Mostafa SA, Al-Fahdawi S, Dinar AM, et al. Benchmarking methodology for selection of optimal COVID-19 diagnostic model based on entropy and TOPSIS methods. IEEE Access. (2020) 8:99115–31. doi: 10.1109/ACCESS.2020.2995597
102. Chowdhury ME, Rahman T, Khandakar A, Mazhar R, Kadir MA, Mahbub Z B, et al. Can AI help in screening viral and COVID-19 pneumonia? IEEE Access. (2020) 8:132665–76. doi: 10.1109/ACCESS.2020.3010287
103. Dlamini GS, Müller SJ, Meraba RL, Young RA, Mashiyane J, Chiwewe T, et al. Classification of COVID-19 and other pathogenic sequences: a dinucleotide frequency and machine learning approach. IEEE Access. (2020) 8:195263–73. doi: 10.1109/ACCESS.2020.3031387
104. Narzullaev A, Muminov Z, Narzullaev M. Contact Tracing of Infectious Diseases Using Wi-Fi Signals and Machine Learning Classification. (2020). p. 1–5.
105. Hassan A, Shahin I, Alsabek MB. Covid-19 detection system using recurrent neural networks. (2020). p. 1–5.
106. Cihan P. Fuzzy Rule-Based System for Predicting Daily Case in COVID-19 Outbreak. (2020). p. 1–4.
107. Zheng B, Liu Y, Zhu Y, Yu F, Jiang T, Yang D, et al. MSD-Net: multi-scale discriminative network for COVID-19 lung infection segmentation on CT. IEEE Access. (2020) 8:185786–95. doi: 10.1109/ACCESS.2020.3027738
108. Zheng N, Du S, Wang J, Zhang H, Cui W, Kang Z, et al. Predicting COVID-19 in China using hybrid AI model. IEEE Transac Cybern. (2020) 50:2891–904. doi: 10.1109/TCYB.2020.2990162
109. Babukarthik R, Adiga VAK, Sambasivam G, Chandramohan D, Amudhavel JJIA. Prediction of COVID-19 Using Genetic Deep Learning Convolutional Neural Network (GDCNN). IEEE Access. (2020) 8:177647–66. doi: 10.1109/ACCESS.2020.3025164
Keywords: machine learning, COVID-19, Internet of Things (IoT), deep learning, big data, information systems, internet of medical things, coronavirus
Citation: Band SS, Ardabili S, Yarahmadi A, Pahlevanzadeh B, Kiani AK, Beheshti A, Alinejad-Rokny H, Dehzangi I, Chang A, Mosavi A and Moslehpour M (2022) A Survey on Machine Learning and Internet of Medical Things-Based Approaches for Handling COVID-19: Meta-Analysis. Front. Public Health 10:869238. doi: 10.3389/fpubh.2022.869238
Received: 04 February 2022; Accepted: 20 April 2022;
Published: 23 June 2022.
Edited by:
Ali Kashif Bashir, Manchester Metropolitan University, United KingdomReviewed by:
Taki Hasan Rafi, Hanyang University, South KoreaCopyright © 2022 Band, Ardabili, Yarahmadi, Pahlevanzadeh, Kiani, Beheshti, Alinejad-Rokny, Dehzangi, Chang, Mosavi and Moslehpour. This is an open-access article distributed under the terms of the Creative Commons Attribution License (CC BY). The use, distribution or reproduction in other forums is permitted, provided the original author(s) and the copyright owner(s) are credited and that the original publication in this journal is cited, in accordance with accepted academic practice. No use, distribution or reproduction is permitted which does not comply with these terms.
*Correspondence: Shahab S. Band, c2hhbXNoaXJiYW5kc0B5dW50ZWNoLmVkdS50dw==; Arthur Chang, Y2hhbmdhcnRAeXVudGVjaC5lZHUudHc=; Amir Mosavi, YW1pci5tb3NhdmlAa3ZrLnVuaS1vYnVkYS5odQ==; Massoud Moslehpour, d3JpdGV0b2RybUBnbWFpbC5jb20=
Disclaimer: All claims expressed in this article are solely those of the authors and do not necessarily represent those of their affiliated organizations, or those of the publisher, the editors and the reviewers. Any product that may be evaluated in this article or claim that may be made by its manufacturer is not guaranteed or endorsed by the publisher.
Research integrity at Frontiers
Learn more about the work of our research integrity team to safeguard the quality of each article we publish.