- Department of Radiation Oncology, Huadong Hospital, Fudan University, Shanghai, China
Purpose: The purpose of this study was to evaluate the accuracy of a lung stereotactic body radiotherapy (SBRT) treatment plan with the target of a newly predicted internal target volume (ITVpredict) and the feasibility of its clinical application. ITVpredict was automatically generated by our in-house deep learning model according to the cone-beam CT (CBCT) image database.
Method: A retrospective study of 45 patients who underwent SBRT was involved, and Mask R-CNN based algorithm model helped to predict the internal target volume (ITV) using the CBCT image database. The geometric accuracy of ITVpredict was verified by the Dice Similarity Coefficient (DSC), 3D Motion Range (R3D), Relative Volume Index (RVI), and Hausdorff Distance (HD). The PTVpredict was generated by ITVpredict, which was registered and then projected on free-breath CT (FBCT) images. The PTVFBCT was margined from the GTV on FBCT images gross tumor volume on free-breath CT (GTVFBCT). Treatment plans with the target of Predict planning target volume on CBCT images (PTVpredict) and planning target volume on free-breath CT (PTVFBCT) were respectively re-established, and the dosimetric parameters included the ratio of the volume of patients receiving at least the prescribed dose to the volume of PTV (R100%), the ratio of the volume of patients receiving at least 50% of the prescribed dose to the volume of PTV in the Radiation Therapy Oncology Group (RTOG) 0813 Trial (R50%), Gradient Index (GI), and the maximum dose 2 cm from the PTV (D2cm), which were evaluated via Plan4DCT, plan which based on PTVpredict (Planpredict), and plan which based on PTVFBCT (PlanFBCT).
Result: The geometric results showed that there existed a good correlation between ITVpredict and ITV on the 4-dimensional CT [ITV4DCT; DSC= 0.83 ±0.18]. However, the average volume of ITVpredict was 10% less than that of ITV4DCT (p = 0.333). No significant difference in dose coverage was found in V100% for the ITV with 99.98 ± 0.04% in the ITV4DCT vs. 97.56 ± 4.71% in the ITVpredict (p = 0.162). Dosimetry parameters of PTV, including R100%, R50%, GI and D2cm showed no statistically significant difference between each plan (p > 0.05).
Conclusion: Dosimetric parameters of Planpredict are clinically comparable to those of the original Plan4DCT. This study confirmed that the treatment plan based on ITVpredict produced by our model could automatically meet clinical requirements. Thus, for patients undergoing lung SBRT, the model has great potential for using CBCT images for ITV contouring which can be used in treatment planning.
Introduction
For patients with early-stage lung cancer, stereotactic body radiotherapy (SBRT) has become one of the primary treatment options. It has been proven to significantly improve the tumor control and overall survival rate of patients with early-stage lung cancer (1–4).
Currently, the most popular treatment method is to use four-dimensional CT (4DCT) imaging to generate the internal target volume (ITV) contour, which expresses the volume of a tumor moving throughout a patient's breathing. This ITV contour from a four-dimensional averaged (4DAVG) image is used to generate a radiation treatment plan (5).
With the 4DCT technique, image acquisition is associated with the patient's breathing curve. The limitations of 4DCT are as follows: (1) Required high patient compliance as an irregular breathing curve can reduce the image quality and affect the accuracy of tumor contouring (6); (2) Complicated and professional operation as it requires a longer time to acquire 4DCT images, which could increase the instability and randomness of the simulation (7); (3) Low popularity as it is estimated that less than half of radiotherapy centers are equipped with four-dimensional scanners (8). Overall, these limits may potentially reduce the SBRT accuracy in treatment.
Conversely, CBCT has high popularity and is conventionally equipped in a linear accelerator (9). In addition, it is mainly used to compare the anatomical landmarks from treatment planning CT images in clinical practice, which are used to determine intra/inter-fraction motion (10). CBCT rotates 360° around the patient's body and then finishes the CT image scanning within a period of time (~1 min) which includes 10–12 respiration time phases and the motion trajectory of the tumor. The limitations of CBCT are as follows. First, poor image quality is the main factor affecting radiation oncologist determination of lung tumor volume (11). Second, due to a prominent amount of and artifacts, the dose calculation based on CBCT images may be inaccurate for treatment planning (12).
In recent years, “deep learning” has been extensively used in medical image processing. Among them, convolutional neural networks (CNNs) are the primary methods of target detection and segmentation (13–16). Mask R-CNN is a simple, flexible, commonly used framework for object instance segmentation, and is popular in medical image processing (17). Bouget et al. used the detection of mediastinal lymph nodes in CT images for lung cancer staging while enabling good instance detection (18). Zhang et al. successfully used Mask R-CNN to detect lung tumors on PET images, which has more effectively and precisely while suitably avoiding incorrect detection of tumors (19). Some previous studies used Mask R-CNN on segmentation, such as detection and classification the breast tumors on sonograms (20) and brain tumor segmentation for dynamic susceptibility contrast-enhanced perfusion imaging (21). These studies also show the great potential of Mask R-CNN in object detection and segmentation, presenting a possibility of it being used in clinical applications of medical images in the future.
Our preliminary research confirmed that the upgraded Mask R-CNN model could predict the ITV with CBCT image accuracy (22). Meanwhile, SBRT delivers high radiation doses to the tumor target in a hypo-fractionated area with a minimum dose to the tissue around the target area (19). Therefore, dosmetric research for lung SBRT is important. This study aimed to evaluate the dose accuracy of a lung SBRT treatment plan with ITVpredict and the feasibility of its clinical application.
Materials and Methods
Patient Data
Forty-five lung cancer patients (average age was 68 years, range: 55–86 years) who underwent SBRT at Huadong Hospital from January 2020 to July 2021 were randomly selected for this study.
Image Acquisition
Each patient's free-breath CT (FBCT) was scanned by a Siemens Somatom Definition AS® CT scanner (Siemens Somatom Sensation, Munich, Germany) with a pitch of 1.5 and slice thickness of 1 mm. 4DCT images were acquired with the additional assistance of Varian real-time position management (RPM) (Varian Medical Systems, Palo Alto, USA) using the same scanning parameters. The first treatment fraction of CBCT images were acquired in our CBCT system (100 kVp and 100 mAs, rotated at 360° with a speed of 6° per second), equipped with a VarianVitalBeamTM linear accelerator (Varian Medical Systems, Palo Alto, USA).
ITV Acquisition
The gross tumor volume (GTV) on FBCT images (GTVFBCT) and the ITV on 4DAVG (ITV4DCT) images were contoured by two radiation oncologists with expertise in lung tumors. Our model, using the Mask R-CNN algorithm with a convolutional block attention module (CBAM) module embedded, was used to automatically establish a newly predicted ITV (ITVpredict) using the CBCT image database (18). Then, we registered the CBCT image and FBCT image and projected the ITVpredict on the FBCT image to calculate the dose. The model workflow is shown in Figure 1.
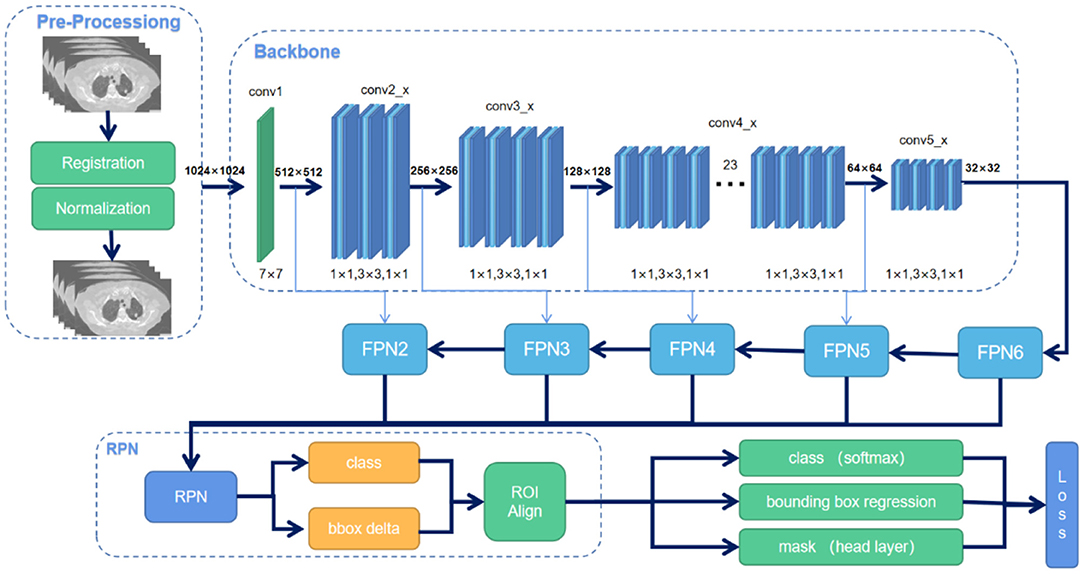
Figure 1. Overview of the Mask R-CNN workflow22. There are two steps. First, it generates proposals for lung tumor regions based on the input cone-beam CT (CBCT) image. Second, it generates a tumor pixel-level mask based on the proposal created in the first step.
Treatment Plan
PTV4DCT and PTVpredict were defined as ITV margins of 5 mm on 4DAVG images and FBCT images, respectively. PTVFBCT was defined as GTVFBCT with a margin of 10 mm in the craniocaudal (CC) direction and 5 mm in the left-right (LR) and anterior-posterior (AP) directions in the FBCT image.
All patient plans were replanned in the Varian Eclipse® system (version 15.5), which was generated by a full arc and was used depending on the location and anatomic relationships of the tumors and normal tissues (Figure 2), by our experienced medical physicists. We used a 6 MV-FFF (DR: 1400 MU/min) energy and the anisotropic analytical dose calculation algorithm (AAA) with a 2.5 mm3 calculating grid in all plans. All patients received prescription of 60 grays (Gy) in 10 fractions (6 Gy per fraction) for over 2 weeks. The planning objectives aimed to cover the PTV with 95% of the prescribed dose in all plans.
Geometry Evaluation Parameters for the Prediction Model
The Dice Similarity Coefficient (DSC), 3D Motion Range (R3D), Relative Volume Index (RVI), and Hausdorff Distance (HD) were calculated to assess the agreement between ITVpredict and ITV4DCT. All statistical tests were performed using SciPy (23) in Python.
PTV Evaluation Parameters
The volume of PTV (VPTV, cm3), mean dose (Dmean), the maximum dose received by 2% (D2%), and the minimum dose received by 98% of the evaluated PTV volume (D98%) were determined. The percent of the PTV receiving 100% of the prescription dose (V100%) and the dose covering 95% of PTV (D95%) were also calculated.
A steep dose gradient at the margin of the target volume is an important part of the SBRT plan to protect the normal organization. Some parameters for quantification have been reported in the literature, including R100%, R50%, the Gradient Index (GI), and the maximum dose 2 cm from the PTV (D2cm).
R100%
R100% is the ratio of the volume of patients receiving at least the prescribed dose to the volume of PTV (9). When the value of R100% is closer to 1, it means that the dose distribution has more conformity for PTV.
R50%
R50% was defined as the ratio of the volume of patients receiving at least 50% of the prescribed dose to the volume of PTV in the Radiation Therapy Oncology Group (RTOG) 0813 Trial (9). The R50% is an evaluation index for damage to irradiated normal tissues (24).
Gradient Index (GI)
The Gradient Index (GI) is defined as the ratio of the volume of the patient receiving at least 50% of the prescription dose to the volume of the patient receiving at least 100% of the prescription dose (25). It was used to measure dose fall-off outside of the PTV. The dose falling off outside the target volume is very important in SBRT, especially as a predictor of complications (26).
OARs Evaluation Parameters and Treatment Efficiency Parameters
The dosimetric parameter acceptance of normal tissues (27), which refers to the RTOG 0813 Trial, is listed in Table 1. The ITV acquisition time was manually recorded for delineation efficiency and automatically recorded for model, and machine monitor units (Mus) were recorded for treatment efficiency.
Statistical Analysis
The statistical significance of the difference between the groups was assessed using 1-way analysis of variance (ANOVA) by SPSS software release 20.0, and the statistical significance was p < 0.05.
Results
Clinical Characteristics
Patient and tumor characteristics of the 45 patients are described in Table 2.
Geometry Evaluation
The DSC value between ITV4DCT and ITVpredict was 0.83 ± 0.18. The DSC value indicates that ITV4DCT and ITVpredict have a good correlation (Table 3). In addition, Figure 3 shows a visual evaluation from different perspectives. ITVpredict can outline the patient's tumor contour and is similar to the radiation oncologist contouring (ITV4DCT). The visual assessment shows that the results are reasonable.
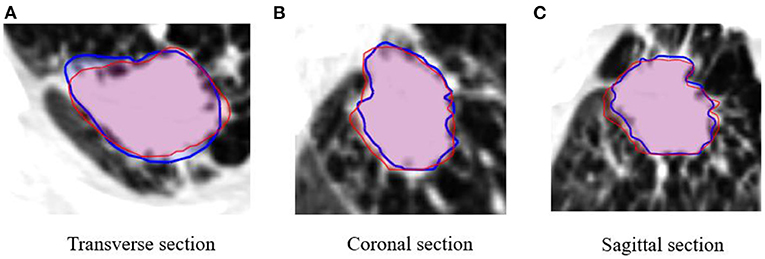
Figure 3. Comparison of contours between ITV on the 4-dimensional CT (ITV4DCT, red) and ITV prediction (ITVpredict, blue) in different views of patient images.
ITV Evaluation
The average volumes of the ITV4DCT and ITVpredict were 21.41 ± 9.38 cm3 and 19.31 ± 8.83 cm3, respectively. Compared to ITV4DCT, ITVpredict reduced ITV volume by 10% on average (p = 0.333). No significant difference was found in ITV volume. However, no significant difference was found in V100% for the ITV with 99.98 ± 0.04% in ITV4DCT vs. 97.56 ± 4.71% in ITVpredict (p = 0.162) (Table 4).
PTV Evaluation
The PTV evaluation parameters are shown in Table 5. The GI value of Planpredict (GI = 3.98 ± 0.42) was slightly lower than that of PlanFBCT (GI = 4.74 ± 1.01), indicating that the descending gradient of PTV was better than that of PlanFBCT and second to that of Plan4DCT (GI = 3.31 ± 0.89). The R100% value for Plan4DCT (R100% =1.05 ± 0.11) was always lower than that for Planpredict (R100% = 1.08 ± 0.05) and PlanFBCT (R100% = 1.12 ± 0.06), and the results show that Plan4DCT has the best performance and high conformability. However, there was no statistically significant difference between the plans (F = 0.141).
The contouring in different views is also similar for the PTV of each plan (Figure 4). The visual assessment shows that the results are reasonable. Figures 5, 6 show the dose color wash and the dose volume histogram (DVH) of the patients, which were calculated for each plan.
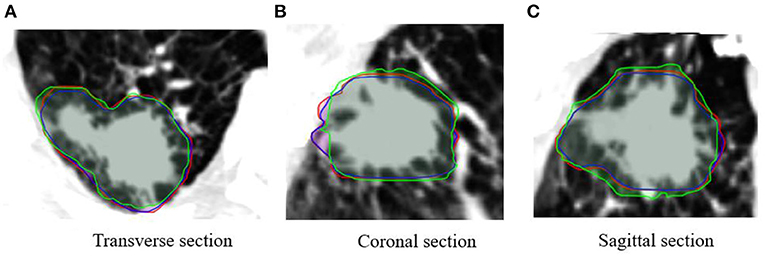
Figure 4. Comparison of contours between planning target volume on four-dimensional CT (PTV4DCT) (red), PTVpredict (blue), and PTVFBCT (green) in different views of patient images (projected PTVpredict and PTVFBCT on 4DCT images).
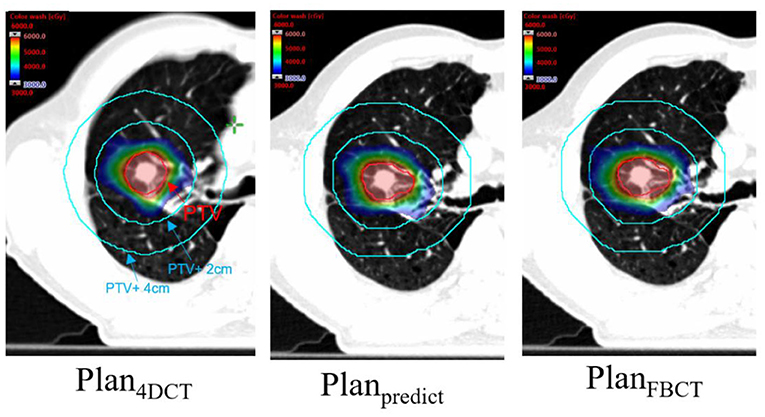
Figure 5. Dose color wash map of each plan for PTV (the area of the red line is PTV, and the red and blue color wash maps represent the 100 and 50% prescription doses, respectively).
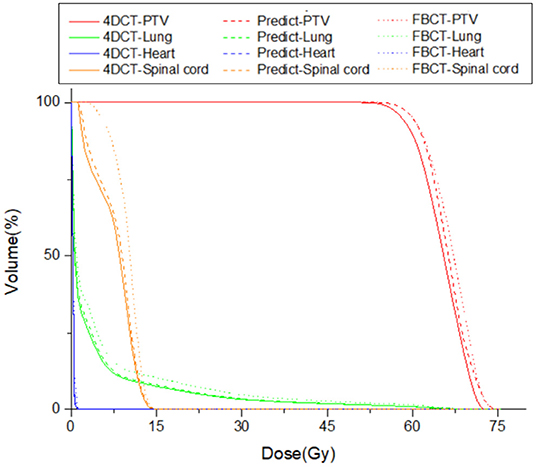
Figure 6. Comparison of the dose-volume histogram (DVH) of one case under the 3 treatment plans: Plan4DCT (solid line), Planpredict (dash line), and PlanFBCT (dot line).
OARs and Treatment Efficiency Evaluation
The estimated dosimetric parameters for all plans met the criteria specified in the RTOG 0813 protocol. The results show that the V20 of Planpredict was better than that of Plan4DCT (with a reduction of 15.4%) and PlanFBCT (with a reduction of 7.8%), which obviously protected the lung (Figure 7), but no difference existed in the dosimetric parameters. Additionally, there was no statistically significant difference in each plan with the maximum dose (Dmax) for the heart, esophagus, and spinal cord (Table 4).
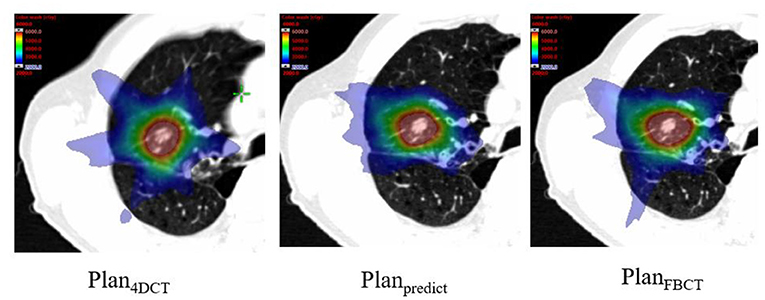
Figure 7. Comparison of color-wash dose distributions of the plans (blue color wash map represents a 20-Gy dose).
The average times of generating ITVs were 1.45 ± 0.31 and 30.28 ± 3.74 min for automatic (by model) and manual ITVs, respectively. The model helped reduce it by 95% of the time on average (p = 0.000).
Discussion
Geometrical Accuracy of the Prediction Model
We used geometric parameters to evaluate the similarity between ITV4DCT and ITVpredict. The DSC value was 0.83 ± 0.18, showing good agreement between the ITVpredict and ITV4DCT contour. DSC > 0.7 is considered to be in good agreement with the gold standard (28, 29). This result can become the basis for follow-up research as it confirmed the feasibility of CBCT images to predict ITV. The accuracy of the ITV contours will directly affect the optimization and calculation of the DVH plan (30). The results show that the ITVpredict volume is 10% smaller than the ITV4DCT volume. Dou et al. found that 4DCT images should be used with caution for patients with highly irregular breathing. The simulation indicates that low-pitch helical 4DCT processes potentially yield large tumor motion measurement errors and overestimate tumor motion (31). However, there was no significant difference between ITV4DCT and ITVpredict. This result of ITVpredict volume reduction was acceptable.
4DCT Limitation and CBCT Potential Application
Four dimensional CT (4DCT) was acquired during the patient positioning stage and could not reflect the deviation of the tumor's respiratory movement during treatment (32, 33). Rabinowitz et al. showed that during the patient positioning stage and the treatment stage, the tumor deviation caused by respiratory motion was an average of 5.1 mm. For thoracic tumors, the tumor deviation caused by respiratory motion could reach 5.8 mm (34). Yang et al. showed that 4DCT could only collect signals of a limited number of respiratory phases, but the patient's breathing may change at any time and cannot accurately reflect the patient's tumor movement during treatment (35). The study showed that CBCT and maximum intensity projection (MIP) images are equivalent in determining the location of ITV (36). Li et al. showed that 4DCT and CBCT images can indicate variations and inter-fractional setup displacement (37). In our study, we used the first CBCT image at the time of treatment to show the respiratory motion range of a tumor during treatment.
PTV Evaluation
Compared with conventional treatment, SBRT has higher requirements for the PTV dose gradient and limited dose limitation of organ at risks (OARs). The RTOG0813 protocol (26) provides guidance on the acceptable values of R100%, R50%, and D2cm based on the PTV volume. The values of R100% and R50% in Plan4DCT and Planpredict were 1.05 ± 0.11, 1.08 ± 0.05, 3.48 ± 0.82, and 5.25 ± 2.01 respectively, which were comparable but slightly different. The result of R100% indicated that Planpredict and Plan4DCT have a similar dose coverage for PTV. The R50% value of Planpredict increased by nearly 30% compared with that of Plan4DCT, which shows that Plan4DCT has a stronger ability to constrain PTV. All plans meet the RTOG0813 protocol and can be used in clinical practice.
In our study, we also researched the dosimetry of GTV on FBCT images to generate PlanFBCT. The results show that after the RTOG0813 guide on margin from GTV, it can also meet the treatment standards and hence can be used for treatment planning. Tian et al. (38) compared the treatment planning and dose calculation of average intensity projection (AIP) and FBCT for SBRT and concluded that the dosimetric of the two datasets were similar.
OARs Evaluation
In addition, the RTOG 0813 agreement contains restrictions on each OAR, such as the lung and spinal cord. For SBRT patients, high-energy rays inevitably pass through a part of normal lung tissue, which affects lung function. Jin et al. (39) found that when V20 was >25%, the incidence of radiation pneumonia significantly increased. Our results show that the mean values of V20 in Plan4DCT and Planpredict were 3.05 ± 0.92% and 2.58 ± 0.71%, respectively, indicating that Planpredict reduces the volume of radiation received by normal lung tissue, thereby reducing the incidence of radiation pneumonitis. The thoracic spinal cord is more likely to be injured in patients with lung SBRT. Radiation myelitis may cause serious consequences, such as paraplegia and respiratory paralysis. The values of spinal cord Dmax in the Plan4DCT and the Planpredict are 9.57 ± 2.61 Gy and 8.89 ± 0.01 Gy. This shows that Planpredict reduces the maximum dose received in the spinal cord, which can thus reduce the incidence of radiation myelitis.
Treatment Efficiency Evaluation
Currently, the model predicts the ITV volume of large tumors more accurately. For patients with lung SBRT, this model can generate ITV on CBCT images in, on average, 1.45 ± 0.31 min. In our research, the generated ITV time of Planpredict was significantly reduced by nearly 95% compared with that of Plan4DCT. Hence, Using the model to input CBCT images can greatly shorten the time to collect patient images and significantly increase the efficiency of tumor delineation for physicians.
This study has some limitations. Firstly, the number of patient samples included was small. Therefore, our patient data did not represent the whole spectrum. Tumors of different sizes and different locations should be included in the future. This could increase patient data for a more uniform tumor volume distribution to ensure accuracy of results in the future.
Conclusion
Geometric results show that self-generated ITVpredict has a good correlation with ITV4DCT, although the ITVpredict volume is 10% smaller than the ITV4DCT volume. This work confirmed the feasibility of the clinical application of ITVpredict to make a treatment plan. There were no significant dosimetry differences between Plan4DCT and Planpredict. Thus, our model has potential application in institutions with or without 4DCT scanning technology or when patient breathing is irregular.
Data Availability Statement
The raw data supporting the conclusions of this article will be made available by the authors, without undue reservation.
Ethics Statement
Written informed consent was obtained from the individual(s) for the publication of any potentially identifiable images or data included in this article.
Author Contributions
SZ and BL: conception and design. XZ and YL: acquisition of data. WG, LZ, and FM: analysis of data. SZ, BL, and JQ: writing, review and/or revision of the manuscript. All authors reviewed, read, and approved the final manuscript and authors contributed to the article, and approved the submitted version.
Funding
This work was partially supported by the National Natural Science Foundation of China (Grant No.11505029 and No.81472794), Shanghai Municipal Commission of Health (Grant Nos. 2018BR23 and 20184Y0099), and Shanghai Municipal Science and Technology Commission (Grant No. 18441904400).
Conflict of Interest
The authors declare that the research was conducted in the absence of any commercial or financial relationships that could be construed as a potential conflict of interest.
Publisher's Note
All claims expressed in this article are solely those of the authors and do not necessarily represent those of their affiliated organizations, or those of the publisher, the editors and the reviewers. Any product that may be evaluated in this article, or claim that may be made by its manufacturer, is not guaranteed or endorsed by the publisher.
References
1. Fakiris AJ, McGarry RC, Yiannoutsos CT, Papiez L, Williams M, Henderson MA, et al. Stereotactic body radiation therapy for early-stage non-small-cell lung carcinoma: four-year results of a prospective phase II study. Int J Radiat Oncol Biol Phys. (2009) 75:677–82. doi: 10.1016/j.ijrobp.2008.11.042
2. Onishi H, Shirato H, Nagata Y, Hiraoka M, Fujino M, Gomi K, et al. Hypofractionated stereotactic radiotherapy (HypoFXSRT) for stage I non-small cell lung cancer: updated results of 257 patients in a Japanese multi-institutional study. J Thorac Oncol. (2007) 2(7 Suppl 3):S94–100. doi: 10.1097/JTO.0b013e318074de34
3. Uematsu M, Shioda A, Suda A, Fukui T, Ozeki Y, Hama Y, et al. Computed tomography-guided frameless stereotactic radiotherapy for stage I non-small cell lung cancer: a 5-year experience. Int J Radiat Oncol Biol Phys. (2001) 51:666–70. doi: 10.1016/S0360-3016(01)01703-5
4. Qiu JJ, Ge W, Zhang L, Yao Y, Zheng X. The feasibility and efficiency of volumetric modulated arc therapy-based breath control stereotactic body radiotherapy for liver tumors. Technol Cancer Res Treat. (2016) 15:674–82. doi: 10.1177/1533034615596273
5. Clements N, Kron T, Franich R, Dunn L, Roxby P, Aarons Y, et al. The effect of irregular breathing patterns on internal target volumes in four-dimensional CT and cone-beam CT images in the context of stereotactic lung radiotherapy. Med Phys. (2013) 40:021904. doi: 10.1118/1.4773310
6. Rietzel E, Pan T, Chen GT. Four-dimensional computed tomography: image formation and clinical protocol. Med Phys. (2005) 32:874–89. doi: 10.1118/1.1869852
7. Watkins WT, Li R, Lewis J, Park JC, Sandhu A, Jiang SB, et al. Patient-specific motion artifacts in 4DCT. Med Phys. (2010) 37:2855–61. doi: 10.1118/1.3432615
8. de Oliveira Duarte S, Rancoule C, He MY, Baury M, Sotton S, Vallard A, et al. Use of 4D-CT for radiotherapy planning and reality in France: data from a national survey. Cancer Radiother. (2019) 23:395–400. doi: 10.1016/j.canrad.2019.02.006
9. Feuvret L, Noël G, Mazeron JJ, Bey P. Conformity index: a review. Int J Radiat Oncol Biol Phys. (2006) 64:333–42. doi: 10.1016/j.ijrobp.2005.09.028
10. Barney BM, Lee RJ, Handrahan D, Welsh KT, Cook JT, Sause WT. Image-guided radiotherapy (IGRT) for prostate cancer comparing kV imaging of fiducial markers with cone beam computed tomography (CBCT). Int J Radiat Oncol Biol Phys. (2011) 80:301–5. doi: 10.1016/j.ijrobp.2010.06.007
11. Fave X, Mackin D, Yang J, Zhang J, Fried D, Balter P, et al. Can radiomics features be reproducibly measured from CBCT images for patients with non-small cell lung cancer? Med Phys. (2015) 42:6784–97. doi: 10.1118/1.4934826
12. Zhang H, Ouyang L, Ma J, Huang J, Chen W, Wang J. Noise correlation in CBCT projection data and its application for noise reduction in low-dose CBCT. Med Phys. (2014) 41:031906. doi: 10.1118/1.4865782
13. Wang C, Hunt M, Zhang L, Rimner A, Yorke E, Lovelock M, et al. Technical Note: 3D localization of lung tumors on cone beam CT projections via a convolutional recurrent neural network. Med Phys. (2020) 47:1161–6. doi: 10.1002/mp.14007
14. Cao H, Liu H, Song E, Ma G, Xu X, Jin R, et al. A two-stage convolutional neural networks for lung nodule detection. IEEE J Biomed Health Inform. (2020) 24:2006–15. doi: 10.1109/JBHI.2019.2963720
15. Gu Y, Lu X, Yang L, Zhang B, Yu D, Zhao Y, et al. Automatic lung nodule detection using a 3D deep convolutional neural network combined with a multi-scale prediction strategy in chest CTs. Comput Biol Med. (2018) 103:220–31. doi: 10.1016/j.compbiomed.2018.10.011
16. Liu C, Hu SC, Wang C, Lafata K, Yin FF. Automatic detection of pulmonary nodules on CT images with YOLOv3: development and evaluation using simulated and patient data. Quant Imaging Med Surg. (2020) 10:1917–29. doi: 10.21037/qims-19-883
17. He K, Gkioxari G, Dollar P, Girshick R. Mask R-CNN. IEEE Trans Pattern Anal Mach Intell. (2020) 42:386–97. doi: 10.1109/TPAMI.2018.2844175
18. Bouget D, Jørgensen A, Kiss G, Leira HO, Langø T. Semantic segmentation and detection of mediastinal lymph nodes and anatomical structures in CT data for lung cancer staging. Int J Comput Assist Radiol Surg. (2019) 14:977–86. doi: 10.1007/s11548-019-01948-8
19. Zhang J, Jiang D, Su H, Dai Z, Dai J, Liu H, et al. Dosimetric comparison of different algorithms in stereotactic body radiation therapy (SBRT) plan for non-small cell lung cancer (NSCLC). Onco Targets Ther. (2019) 12:6385–91. doi: 10.2147/OTT.S201473
20. Chiao JY, Chen KY, Liao KY, Hsieh PH, Zhang G, Huang TC. Detection and classification the breast tumors using mask R-CNN on sonograms. Medicine. (2019) 98:e15200. doi: 10.1097/MD.0000000000015200
21. Jeong J, Lei Y, Kahn S, Liu T, Curran WJ, Shu HK, et al. Brain tumor segmentation using 3D Mask R-CNN for dynamic susceptibility contrast enhanced perfusion imaging. Phys Med Biol. (2020) 65:185009. doi: 10.1088/1361-6560/aba6d4
22. Zhen Li, Shujun Zhang, Libo Zhang, Ya Li, Xiangpeng Zheng, Jie Fu, et al. Deep learning-based Internal Target Volume (ITV) prediction using cone-beam CT Images in lung stereotactic body radiotherapy. Technol Cancer Res Treat. (2022) 21:1–10. doi: 10.1177/15330338211073380
24. Desai DD, Cordrey IL, Johnson EL. A physically meaningful relationship between R50% and PTV surface area in lung SBRT. J Appl Clin Med Phys. (2020) 21:47–56. doi: 10.1002/acm2.12964
25. Paddick I, Lippitz B. A simple dose gradient measurement tool to complement the conformity index. J Neurosurg. (2006) 105(Suppl):194–201. doi: 10.3171/sup.2006.105.7.194
26. Menon SV, Paramu R, Bhasi S, Nair RK. Evaluation of plan quality metrics in stereotactic radiosurgery/radiotherapy in the treatment plans of arteriovenous malformations. J Med Phys. (2018) 43:214–20. doi: 10.4103/jmp.JMP_25_18
27. Bezjak A, Paulus R, Gaspar LE, Timmerman RD, Straube WL, Ryan WF, et al. Safety and efficacy of a five-fraction stereotactic body radiotherapy schedule for centrally located non-small-cell lung cancer: NRG oncology/RTOG 0813 trial. J Clin Oncol. (2019) 37:1316–25. doi: 10.1200/JCO.18.00622
28. Gaede S, Olsthoorn J, Louie AV, Palma D, Yu E, Yaremko B, et al. An evaluation of an automated 4D-CT contour propagation tool to define an internal gross tumour volume for lung cancer radiotherapy. Radiother Oncol. (2011) 101:322–8. doi: 10.1016/j.radonc.2011.08.036
29. Eldesoky AR, Yates ES, Nyeng TB, Thomsen MS, Nielsen HM, Poortmans P, et al. Internal and external validation of an ESTRO delineation guideline - dependent automated segmentation tool for loco-regional radiation therapy of early breast cancer. Radiother Oncol. (2016) 121:424–30. doi: 10.1016/j.radonc.2016.09.005
30. Cao M, Stiehl B, Yu VY, Sheng K, Kishan AU, Chin RK, et al. Analysis of geometric performance and dosimetric impact of using automatic contour segmentation for radiotherapy planning. Front Oncol. (2020) 10:1762. doi: 10.3389/fonc.2020.01762
31. Dou TH, Thomas DH, O'Connell D, Bradley JD, Lamb JM, Low DA. Technical note: simulation of 4DCT tumor motion measurement errors. Med Phys. (2015) 42:6084–9. doi: 10.1118/1.4931416
32. Shah AP, Kupelian PA, Waghorn BJ, Willoughby TR, Rineer JM, Mañon RR, et al. Real-time tumor tracking in the lung using an electromagnetic tracking system. Int J Radiat Oncol Biol Phys. (2013) 86:477–83. doi: 10.1016/j.ijrobp.2012.12.030
33. Purdie TG, Moseley DJ, Bissonnette JP, Sharpe MB, Franks K, Bezjak A, et al. Respiration correlated cone-beam computed tomography and 4DCT for evaluating target motion in stereotactic lung radiation therapy. Acta Oncol. (2006) 45:915–22. doi: 10.1080/02841860600907345
34. Rabinowitz I, Broomberg J, Goitein M, McCarthy K, Leong J. Accuracy of radiation field alignment in clinical practice. Int J Radiat Oncol Biol Phys. (1985) 11:1857–67. doi: 10.1016/0360-3016(85)90046-X
35. Yang M, Timmerman R. Stereotactic ablative radiotherapy uncertainties: delineation, setup and motion. Semin Radiat Oncol. (2018) 28:207–17. doi: 10.1016/j.semradonc.2018.02.006
36. Wang L, Chen X, Lin MH, Xue J, Lin T, Fan J, et al. Evaluation of the cone beam CT for internal target volume localization in lung stereotactic radiotherapy in comparison with 4D MIP images. Med Phys. (2013) 40:111709. doi: 10.1118/1.4823785
37. Li Y, Ma JL, Chen X, Tang FW, Zhang XZ. 4DCT and CBCT based PTV margin in Stereotactic Body Radiotherapy(SBRT) of non-small cell lung tumor adhered to chest wall or diaphragm. Radiat Oncol. (2016) 11:152. doi: 10.1186/s13014-016-0724-5
38. Tian Y, Wang Z, Ge H, Zhang T, Cai J, Kelsey C, et al. Dosimetric comparison of treatment plans based on free breathing, maximum, and average intensity projection CTs for lung cancer SBRT. Med Phys. (2012) 39:2754–60. doi: 10.1118/1.4705353
Keywords: 4DCT, CBCT (cone beam computed tomography), SBRT (stereotactic body radiation therapy), deep learning, Mask R-CNN
Citation: Zhang S, Lv B, Zheng X, Li Y, Ge W, Zhang L, Mo F and Qiu J (2022) Dosimetric Study of Deep Learning-Guided ITV Prediction in Cone-beam CT for Lung Stereotactic Body Radiotherapy. Front. Public Health 10:860135. doi: 10.3389/fpubh.2022.860135
Received: 22 January 2022; Accepted: 21 February 2022;
Published: 22 March 2022.
Edited by:
Ming-Chin Lin, Taipei Medical University, TaiwanReviewed by:
Yueh-hsun Lu, Taipei Medical University, TaiwanRahul Pratap Kotian, Gulf Medical University, United Arab Emirates
Copyright © 2022 Zhang, Lv, Zheng, Li, Ge, Zhang, Mo and Qiu. This is an open-access article distributed under the terms of the Creative Commons Attribution License (CC BY). The use, distribution or reproduction in other forums is permitted, provided the original author(s) and the copyright owner(s) are credited and that the original publication in this journal is cited, in accordance with accepted academic practice. No use, distribution or reproduction is permitted which does not comply with these terms.
*Correspondence: Jianjian Qiu, qiujianjian@fudan.edu.cn
†These authors have contributed equally to this work