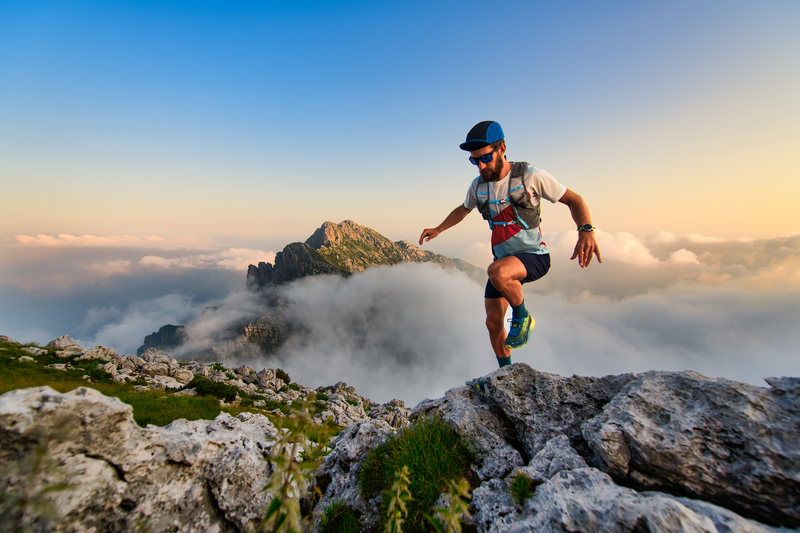
94% of researchers rate our articles as excellent or good
Learn more about the work of our research integrity team to safeguard the quality of each article we publish.
Find out more
ORIGINAL RESEARCH article
Front. Public Health , 31 March 2022
Sec. Environmental Health and Exposome
Volume 10 - 2022 | https://doi.org/10.3389/fpubh.2022.854922
This article is part of the Research Topic Air Quality, Climate and Public Health View all 14 articles
Background: Sulfur dioxide (SO2) has been reported to be related to the mortality of respiratory diseases, but the relationship between SO2 and hospital inpatient visits with respiratory diseases and the potential impact of different seasons on this relationship is still unclear.
Methods: The daily average concentrations of air pollutants, including SO2 and meteorological data in Ganzhou, China, from 2017 to 2019 were collected. The data on daily hospitalization for respiratory diseases from the biggest hospital in the city were extracted. The generalized additive models (GAM) and the distributed lag non-linear model (DLNM) were employed to evaluate the association between ambient SO2 and daily inpatient visits for respiratory diseases. Stratified analyses by gender, age, and season were performed to find their potential effects on this association.
Results: There is a positive exposure-response relationship between SO2 concentration and relative risk of respiratory inpatient visits. Every 10 μg/m3 increase in SO2 was related to a 3.2% (95% CI: 0.6–6.7%) exaltation in daily respiratory inpatient visits at lag3. In addition, SO2 had a stronger association with respiratory inpatient visits in women, older adults (≥65 years), and warmer season (May-Oct) subgroups. The relationship between SO2 and inpatient visits for respiratory diseases was robust after adjusting for other air pollutants, including PM10, NO2, O3, and CO.
Conclusion: This time-series study showed that there is a positive association between short-term SO2 exposure and daily respiratory inpatient visits. These results are important for local administrators to formulate environmental public health policies.
Air pollution is one of the most important public health problems around the world (1). Sulfur dioxide (SO2), mainly comes from various industrial processes, transportation, and vehicles, power plants, and fuel (coal) combustion, is one of the most common and irritant air pollutants in developing countries and industrialized areas (2–5). Several epidemiological studies have revealed that SO2 exposure is related to human respiratory health (6, 7), including stimulating the respiratory tract (8), leading to the decline of pulmonary function (9, 10), and the increased mortality due to respiratory diseases (11, 12). However, there are few studies on the relationship between SO2 and respiratory morbidity in developing countries. Inpatient visit is an important indicator of morbidity and has been widely used to assess the adverse effects associated with atmospheric pollutants (13). Therefore, it will be helpful to understand the impact of SO2 on the respiratory system by evaluating the relationship between ambient air SO2 and the number of hospitalized cases of the respiratory system.
Ganzhou is located in the southern part of Jiangxi Province. The ambient temperature and lifestyle of Ganzhou are typical of southern China. In 2019, the mean concentrations of PM10, SO2, NO2, O3, and CO in Ganzhou were 51, 12, 22, 74, and 1.2 mg/m3, respectively. Except for SO2, the concentrations of other air pollutants in Ganzhou were lower than the mean concentration of 337 Chinese cities (63 μg/m3 for PM10, 11 μg/m3 for SO2, 27 μg/m3 for NO2, 148 μg/m3 for O3, and 1.4 mg/m3 for CO, respectively) (14). Compared with lower levels of other air pollutants, the concentration of SO2 in Ganzhou is higher than that of the national average in China, drawing more attention to the potential respiratory health damage caused by SO2.
In this study, the daily average concentrations of air pollutants, including SO2 from 2017 to 2019, were collected from the local Environmental Protection Bureau. The respiratory inpatient visits were recorded during the same period in the biggest hospital in Ganzhou. We analyzed the association between the level of SO2 exposure and the daily respiratory inpatient visit, investigated populations more sensitive to SO2 exposure, assessed the potential impact of seasonal changes on SO2 lagging effects, and explored the exposure-response relationship between SO2 concentrations and different population subgroups.
Ganzhou (24°29′- 27°09′ N; 113°54′- 116°38′ E) is located in the southern region of China. The terrain of the area is dominated by mountains and hills. Ganzhou has a total population of 9.82 million and an area of 39,379 km2, accounting for 23.6% of the total area of Jiangxi. The area is characterized by a subtropical monsoon climate. The average annual rainfall in Ganzhou in 2020 is 1,706.4 mm; the average temperature is 19.9°C; the average sunshine hours is 1,637.9 h (15).
In this time-series analysis study, we extracted the daily respiratory inpatient visits from January 1, 2017, to December 31, 2019, from the medical database of the largest hospital (the First Affiliated Hospital of Gannan Medical University) in Ganzhou. A total of 9,668 respiratory inpatient visits were recorded during the study period. The respiratory inpatients were identified by the primary code of admission diagnosis (ICD-10: J00–J99). The concentration data of atmospheric pollutants in this study came from the Ganzhou Environmental Protection Bureau. There were five air monitoring stations in the city, and the mean daily concentrations of ambient PM10, SO2, NO2, O3, and CO were collected by these fixed monitoring stations. The daily temperature and humidity data during the study period were collected from the Ganzhou Meteorological Bureau. The daily air pollutant concentrations and meteorological data were not missing.
Daily inpatient visits are generally considered to be rare events and have a Poisson distribution. Therefore, this study used the generalized additive model (GAM) and the distributed lag non-linear model (DLNM) to investigate the relationship between SO2 and respiratory daily hospitalizations. Due to potential non-linear effects, natural spline functions were used to control the confounding factors, such as long-term trends, relative humidity, temperature, day of the week effect, and the effect of holidays. The GAM was selected to evaluate the health effects of SO2 under different lag days (including single-day lag effects and multi-day lag effects). Referring to previous studies (16, 17), the model of the hysteresis effect of SO2 is as follows:
where E(Yt) means the expected number of inpatient visits for the respiratory system at day t; Xt and β represent the concentration of SO2 in the atmosphere on day t and the regression coefficient, respectively; ns is natural spline function; df refers to the degrees of freedom; DOW means the day of the week; Holiday indicates the effect of holidays; α refers to a constant.
The DLNM was used to reflect the exposure-response relationship between SO2 concentrations and the relative risk of respiratory inpatient visits based on the hysteresis effect. The cross-basis function can combine the two dimensions of atmospheric pollutant concentration and lag days. Referring to related research (18–20), the model of the exposure-response relationship is as follows:
where E(Yt) denotes the expected number of respiratory inpatient visits at lag day t; Xt, I and β represent the cross-basis function of SO2 and the regression coefficient, respectively.
Several analyses were adopted to investigate the relation between SO2 and respiratory inpatient visits. Firstly, single-pollutant model, including single-day lag (from lag0, which meant current day estimated effect, to lag7, which meant the previous 7th day estimated effect) and multi-day lag (from lag0–1, which represented the mean of the current day effect and lag1 effect, to lag0–7, which represented the mean of the current day effect and the previous 7 days' effects), was selected to research the lag pattern of SO2. Secondly, the expose-response relationship between the SO2 concentration and the relative risk of respiratory inpatient visits was plotted. Thirdly, the stratified analysis was selected to explore the relationship between the SO2 level and the inpatient visits for respiratory diseases in different gender, ages, and season subgroups. Finally, the multi-pollutant model was used to evaluate the stability of the single pollutant model after adjusting for other atmospheric pollutants, such as PM10, NO2, O3, and CO.
The results of the exposure-response relationship were presented as the relative risk (RR) of respiratory inpatient visits caused by SO2 exposure as a continuous variable. The rest of the results were presented as the percentage changes (PC) and 95% CI in daily respiratory inpatient visits each 10 μg/m3 increment of SO2 levels. In this study, two-sided p < 0.05 was considered statistically significant. All statistical analyses were conducted in R 4.0.2 using the “mgcv” and “dlnm” packages.
Table 1 presents descriptive results for ambient air pollutant concentrations, meteorological parameters, and daily respiratory inpatient visits. During the 1,095 days from January 1, 2017, to December 31, 2019, the average daily concentrations (standard deviation) of atmospheric pollutants were 62.7 (34.6) μg/m3 for PM10, 18.5 (11) μg/m3 for SO2, 24.2 (13) μg/m3 for NO2, 71.7 (34.5) μg/m3 for O3, and 1.3 (0.3) mg/m3 for CO, respectively. Moreover, the daily mean relative humidity and temperature were 74% and 19.6°C, respectively. A total of 9,668 respiratory inpatient visits were recorded from 2017 to 2019; the daily mean count of inpatient visits was 9. Women accounted for ∽34.1% of all the cases, and younger people (<65 years) accounted for ∽48.1% of all the cases.
Table 1. Data for ambient air pollutants, weather conditions, and respiratory inpatient visits in Ganzhou from 2017 to 2019.
The correlations between meteorological parameters and atmospheric pollutants are shown in Table 2. All the correlation coefficients between the meteorological factors and the atmospheric pollutants were statistically significant. The daily concentration of SO2 was positively correlated with other air pollutants (PM10, NO2, O3, and CO) and temperature (correlation coefficient = 0.07–0.71) and negatively correlated with relative humidity (correlation coefficient = −0.41). Moreover, PM10 and NO2 had positive associations with other air pollutants (correlation coefficient = 0.12–0.71), while there was a negative correlation between CO and O3 (correlation coefficient = −0.16). The temperature was also negatively associated with relative humidity (correlation coefficient = −0.31).
Table 3 shows the percent changes in daily respiratory inpatient visits associated with a 10 μg/m3 increase in SO2 on different lag days in different gender and age subgroups. The delayed effects of SO2 were significant at lag3, lag4, lag0–3, lag0–4, lag0–5, lag0–6, and lag0–7, with the maximum effect observed at lag4 (PC: 3.4%; 95% CI: 0.4–6.4%) in single-lag days. The maximum effect of multi-lag days was at lag 0:5 with 6.9% (95% CI: 1.6–12.5%). For men, the association between SO2 exposure and respiratory admission was not statistically significant at any lag days. The SO2 concentration was significantly correlated with women at lag1, lag4, and all the multi-lag days, with the largest effect observed at lag1 (PC: 7.1%; 95% CI: 1.9–12.6%) in the single-lag day model. For younger people (<65 years), there is no statistically significant relationship between SO2 and inpatient visits for respiratory diseases at any lag days. For the elderly (≥65 years), daily respiratory inpatient visits were significantly associated with SO2 concentration at lag1, lag4, and all the multi-lag days except lag0:7; the strongest association was observed at lag1 (PC: 5.5%; 95% CI: 1–10.2%) in single-lag day model.
Table 3. Percentage change (95% CI) of inpatient visits for respiratory diseases per 10 μg/m3 increase in concentrations of SO2 for different lag days in the single-pollutant model.
Figure 1 presents a positive exposure-response relationship between the SO2 concentration and the relative risk of respiratory inpatient visits. The exposure-response curves of the total population, men, women, and the elderly (≥65 years) were raised relatively faster in the range of 0–20 μg/m3, which meant that the risk of respiratory hospitalization increases rapidly under relatively low concentrations of SO2 exposure; the curves in the range of 20–50 μg/m3 were relatively flat, indicating that the risk of hospitalization remains at a relatively high level with the increase of SO2 concentration. The curve for younger people (<65 years) decreased slightly in the range of 0–10 μg/m3 and increased at higher concentration (20–50 μg/m3), which showed the risk of hospitalization for younger people's respiratory system does not change much under relatively low concentration of SO2 exposure.
Figure 1. Relative risk (RR) of respiratory inpatient visits caused by different SO2 concentrations.
As shown in Figure 2, the association between the SO2 concentration and the daily respiratory inpatient visits was stronger in the warm season (May–Oct) rather than in the cold season (Nov–Apr). For the elderly (≥65 years), the positive association between the SO2 concentration and the respiratory inpatient visits was statistically significant only in warm seasons. Table 4 summarizes the percent changes for daily respiratory inpatient visits associated with each 10 μg/m3 increase of SO2 concentration in multiple pollutant models. We used the data from lag3 and lag4 because the single-day lag effect of SO2 was statistically significant in the above lag days. The results from the multi-pollutant model indicated that the relationship between SO2 and respiratory inpatient visits was meaningless at lag3 after PM10 was controlled. When adjusted for all atmospheric pollutants, including PM10, NO2, O3, and CO, the association between SO2 and inpatient visits was still statistically significant at lag4. The effects of SO2 on respiratory inpatient visits were slightly decreased after adjusting for PM10, O3, and CO (2.6–3.3%) at lag4 and the percent change of inpatient visits became 3.8% after adjusting for NO2.
Figure 2. Percentage change (95% CI) of inpatient visits per 10 μg/m3 increase in concentrations of SO2 for different lag days in different seasons. (A) represents total people; (B) represents male; (C) represents female; (D) represents the elderly people (≥65 years); (E) represents younger people (<65 years).
Table 4. Percentage change (95% CI) of inpatient visits associated with 10 μg/m3 increase of SO2 under multiple pollutant models.
Our research is a quantitative evaluation of the relevance between SO2 and daily respiratory inpatient visits in Ganzhou from 2017 to 2019 using the GAM and the DLNM. The results indicated that the elevation in SO2 concentration was significantly associated with an increase in respiratory inpatient visits, especially in the warm season (May–Oct), women, and elderly (≥65 years) subgroups. This association was stable after adjusting for other atmospheric pollutants. The exposure-response curves of the SO2 concentrations and the relative risk of respiratory inpatient visits were nearly non-linear with no obvious thresholds.
This study showed significant cumulative effects of SO2 concentration in a single pollutant model, with the peak at lag0–5. At this point, per 10 μg/m3 elevation of SO2 concentration was associated with a 6.9% (95% CI: 1.6–12.5%) increment in daily respiratory inpatient visits. The results of the previous studies were consistent with ours, indicating that the SO2 concentration was positively related to respiratory diseases (21). Early in vivo studies have observed that SO2 exposure causes bronchoconstriction and induces respiratory diseases (22). A study conducted in Thailand indicated that a 10 μg/m3 increase in the SO2 concentration was associated with a 1.83% (95% CI: 1.2–2.4%) enhancement in total respiratory hospital admissions at lag0–4 (23). Similarly, the positive relationship between respiratory admissions and SO2 was observed at lag 4 [relative risk (RR): 1.12; 95% CI: 1.05–1.21] in Malaysia (24). A systematic review also suggested that every 10 μg/m3 increase of the SO2 levels corresponded to 0.7% (95% CI: 0.1–1.4%) increment respiratory morbidity at lag0 (25). An ecological study reported that in Shenyang, a typical heavily polluted city in China, per 10 μg/m3 increase in SO2 was related to 0.7% (95% CI: 0–1.4%) increase in respiratory admissions at lag0 (26). The earliest observed positive relation between SO2 exposure and respiratory inpatient visits in our study was at lag3 in a single-day lag model. After comparing the average SO2 concentrations in Ganzhou (18.5 μg/m3) and Shenyang (55 μg/m3) during the study period, we speculated that the exposure of higher concentrations of SO2 had intraday effects on the respiratory health, while the exposure of relatively low concentrations of SO2 had a delayed effect.
In this study, the earliest observed association between the SO2 concentration and the daily inpatient visits with respiratory diseases was at lag0–3 in the multi-day lag model. Conversely, some earlier studies indicated that the strongest effects were observed on the current day (25) or lag0–1 (23). A plausible explanation for the longer-than-normal hysteresis effect observed in Ganzhou may be that long-term exposure to SO2 leads to a decrease in the sensitivity of residents to SO2. In addition, the differences in the estimated effects and lagged patterns of SO2 may be related to different outcome indicators of the study, local economic development, and the gender structure of the total population in different regions. A retrospective study from Switzerland suggested that the relationship between the SO2 exposure and the hospital admissions for respiratory diseases was not statistically significant (27). This might reflect the difference in population sensitivity caused by different cultural backgrounds and dietary structures.
The stratified analysis suggested that women and elderly (≥65 years) are more sensitive to SO2 exposure, which is consistent with previous studies (28, 29). An observational study conducted in Lanzhou, China, found that the estimated effect size of SO2 on respiratory hospital admissions was slightly larger in women than in men (30). Physiological factors, such as lower red blood cell count and higher airway response, may be important reasons that cause women to be more sensitive to atmospheric pollutant exposure (31, 32). Moreover, the health differences according to sex may be affected by smoking, drinking, and other unhealthy living habits and occupational environment. Interestingly, in an earlier multi-city time series analysis, gender differences in the impact of SO2 were not observed, and this study pointed out that the insignificant gender differences may be due to factors, such as study design, sample size, and modeling strategy (33).
Consistent results have been reported on the effects of ambient air pollution on specific age groups. Qiu et al. found that each 10 μg/m3 increase in SO2 corresponds to a 3.4% (95% CI: 2.3–4.5%) increase in overall respiratory hospital admissions among the elderly (>65 years) in the Sichuan Basin (33). Similarly, earlier studies observed that SO2 was more associated with hospital admissions for respiratory diseases in older adults (>65) than in other age subgroups (26). The elderly are more susceptible to pathogen exposure due to the weaker immune defenses and respiratory functions (34). This may be an important reason for their increased sensitivity to SO2 exposure.
The health effects of SO2 in different seasons have long been debated. The results showed a positive relation between SO2 and daily respiratory inpatient visits during the warm season. Two study indicated that the relationship between SO2 and respiratory disease morbidity could be observed only during the cold season (heating season) (30, 35). It was worth mentioning that the research site is located in northern China, where temperatures are well below freezing in winter, and fossil fuels need to be burned for heating. The SO2 level in the cold season in northeast China was much higher than that in the warm season. However, there was no statistical difference in the SO2 concentration in Ganzhou during the cold and warm seasons (Supplementary Table 1). A recent study, also from southern China, was consistent with our findings, confirming a statistically significant association between SO2 and respiratory disease mortality during warm seasons (36). People in warm seasons tend to spend longer times outdoors. Even indoors, residents often open windows during warm seasons, and the indoor SO2 level is closer to the ambient SO2 level. Moreover, the different meteorological conditions (especially extreme temperatures) in different regions may be one of the important reasons for the variability (37–39).
Understanding the exposure-response curves of atmospheric pollutants is very important for the making of environmental and public health policies. As shown in Figure 1, we found that even at very low exposure levels, a positive correlation between SO2 and daily respiratory inpatient visits could be observed in the elderly subgroups. Similarly, several studies demonstrated adverse effects of SO2 at relatively low concentrations (40, 41). The health effect of SO2 as a single pollutant or with other atmospheric pollutants has long been controversial. In this study, the association between the SO2 concentration and the respiratory inpatient visits was stable after adjusting for other air pollutants at lag4. A study in Japan by Yorifuji et al. (42) also suggested that the relationship between SO2 exposure and respiratory system mortality can still be observed after adjusting for atmospheric pollutants, such as nitrogen dioxide and particulate matter. Yang et al. (43) proposed that multi-pollutant models increase the standard error, so the effects of multi-pollutant models tend to be slightly lower than those of single-pollutant models, which was consistent with the results of this study. However, a systematic review suggests that after adjusting for PM10 and NO2, no association between respiratory morbidity and SO2 had been observed (25). It is worth noting that there is a strong correlation between atmospheric pollutants, and it is difficult to accurately assess the adverse effects of a single pollutant even if multi-pollutant models are used (44).
In this study, the generalized additive model was adopted to adjust for the influence of confounding factors, such as long-term trends of the time, meteorological conditions, weekend, and holiday effects. Our study revealed a positive correlation between the SO2 concentration and the morbidity of respiratory diseases in China. Nevertheless, the study has several limitations. Firstly, the average concentration at fixed air monitoring stations was used as a proxy for individual exposure, which may underestimate the adverse effects of SO2 (45). Secondly, even if multi-pollutant models were used, the independent effects of SO2 could not be fully explored because there are no data on other particulate pollutants except PM10 in this study. Thirdly, the main model of this study did not include several confounding factors, such as daily activities and socioeconomic status, which may not fully reflect the association between SO2 and respiratory inpatient visits.
The relationship between the elevated SO2 concentrations and the daily respiratory inpatient visits was observed in Ganzhou, a subtropical city in southern China, even though the average daily SO2 concentrations were lower than the minimum allowable exposure concentration set by the WHO (46) and China (47). We call on scholars in different regions to research on the health damage caused by common air pollutants to provide a theoretical basis for local health promotion and environmental health policy formulation. Future investigations should require more rigorous experimental designs (e.g., a combination of animal experiments and population epidemiological investigations) to identify the subgroups susceptible to respiratory damage caused by the SO2 exposure.
Our study indicated that SO2 concentration is positively associated with daily inpatient visits for respiratory disease. The association is closer in women, elderly people (≥65 years), and warmer seasons (May–Oct) subgroups. These results provide further evidence to support the potential health effects of exposure to SO2. We hope that our study can remind researchers and managers to pay more attention to the adverse effects of SO2 in developing countries.
The data analyzed in this study is subject to the following licenses/restrictions: the disclosure and use of inpatient information requires the consent of the relevant departments of the hospital. Requests to access these datasets should be directed to https://www.gyyfy.com/.
XZho carried out literature retrieval, determined the research direction and data analysis according to the existing literature, and wrote the article. YG participated in the data collection of articles and assisted in statistical analysis. DW and WC explained the data results, discussed them in combination with existing articles, and assisted in writing the first draft of the article. XZha was mainly responsible for contacting the hospital to provide the data required for this study and putting forward modification opinions on the first draft of the article. All authors read and approved the final manuscript.
This work was funded by Gannan Medical University (Fund No. QD201901) and Department of Education of Jiangxi Province (Fund No. GJJ190786).
The authors declare that the research was conducted in the absence of any commercial or financial relationships that could be construed as a potential conflict of interest.
All claims expressed in this article are solely those of the authors and do not necessarily represent those of their affiliated organizations, or those of the publisher, the editors and the reviewers. Any product that may be evaluated in this article, or claim that may be made by its manufacturer, is not guaranteed or endorsed by the publisher.
The Supplementary Material for this article can be found online at: https://www.frontiersin.org/articles/10.3389/fpubh.2022.854922/full#supplementary-material
1. Kelly FJ, Fussell JC. Air pollution and public health: emerging hazards and improved understanding of risk. Environ Geochem Health. (2015) 37:631–49. doi: 10.1007/s10653-015-9720-1
2. Craig K. A Review of the Chemistry, Pesticide Use, and Environmental Fate of Sulfur Dioxide, as Used in California. Cham: Springer International Publishing (2019), 33–64.
3. Darynova Z, Amouei TM, Abdrakhmanov T, Sabyrzhan S, Sagynov S, Hopke PK, et al. SO2 and HCHO over the major cities of Kazakhstan from 2005 to 2016: influence of political, economic and industrial changes. Sci Rep. (2020) 10:12635. doi: 10.1038/s41598-020-69344-w
4. Li Z, Wang Y, Xu Z, Cao YE. Characteristics and sources of atmospheric pollutants in typical inland cities in arid regions of central Asia: a case study of Urumqi city. PLoS ONE. (2021) 16:e249563. doi: 10.1371/journal.pone.0249563
5. Zhao C, Sun Y, Zhong Y, Xu S, Liang Y, Liu S, et al. Spatio-temporal analysis of urban air pollutants throughout China during 2014–2019. Air Qual Atmos Health. (2021) 14:1619–32. doi: 10.1007/s11869-021-01043-5
6. Grzywa-Celińska A, Krusiński A, Milanowski J. 'Smoging kills' - Effects of air pollution on human respiratory system. Ann Agric Environ Med. (2020) 27:1–5. doi: 10.26444/aaem/110477
7. Yu Y, Yu Z, Sun P, Lin B, Li L, Wang Z, et al. Effects of ambient air pollution from municipal solid waste landfill on children's non-specific immunity and respiratory health. Environ Pollut. (2018) 236:382–90. doi: 10.1016/j.envpol.2017.12.094
8. Li T, Zhang X, Li C, Bai X, Zhao Z, Norback D. Onset of respiratory symptoms among Chinese students: associations with dampness and redecoration, PM10, NO2, SO2 and inadequate ventilation in the school. J Asthma. (2020) 57:495–504. doi: 10.1080/02770903.2019.1590591
9. Chen X, Liu F, Niu Z, Mao S, Tang H, Li N, et al. The association between short-term exposure to ambient air pollution and fractional exhaled nitric oxide level: a systematic review and meta-analysis of panel studies. Environ Pollut. (2020) 265:114833. doi: 10.1016/j.envpol.2020.114833
10. Gao N, Xu W, Ji J, Yang Y, Wang S, Wang J, et al. Lung function and systemic inflammation associated with short-term air pollution exposure in chronic obstructive pulmonary disease patients in Beijing, China. Environ Health. (2020) 19:12. doi: 10.1186/s12940-020-0568-1
11. Chen J, Shi C, Li Y, Ni H, Zeng J, Lu R, et al. Effects of short-term exposure to ambient airborne pollutants on COPD-related mortality among the elderly residents of Chengdu city in Southwest China. Environ Health Prev Med. (2021) 26:7. doi: 10.1186/s12199-020-00925-x
12. Orellano P, Reynoso J, Quaranta N. Short-term exposure to sulphur dioxide (SO2) and all-cause and respiratory mortality: a systematic review and meta-analysis. Environ Int. (2021) 150:106434. doi: 10.1016/j.envint.2021.106434
13. Tian Y, Liu H, Liang T, Xiang X, Li M, Juan J, et al. Ambient air pollution and daily hospital admissions: a nationwide study in 218 Chinese cities. Environ Pollut. (2018) 242:1042–9. doi: 10.1016/j.envpol.2018.07.116
14. (2019 2020-05-18). Report on the State of the Ecology and Environment in China (2019). Available online at: https://english.mee.gov.cn/Resources/Reports/soe/SOEE2019/202012/P020201215587453898053.pdf (accessed October 01, 2022).
15. (2021 2021-01-12). Ganzhou Municipal People's Government. Available online at: https://www.ganzhou.gov.cn/gzszf/c100146/list_sq.shtml (accessed May 2, 2022).
16. Cheng B, Ma Y, Wang H, Shen J, Zhang Y, Guo L, et al. Particulate matter pollution and emergency room visits for respiratory diseases in a valley Basin city of Northwest China. Environ Geochem Health. (2021) 43:3457–68. doi: 10.1007/s10653-021-00837-x
17. Li Y, Li C, Liu J, Meng C, Xu C, Liu Z, et al. An association between PM2.5 and pediatric respiratory outpatient visits in four Chinese cities. Chemosphere. (2021) 280:130843. doi: 10.1016/j.chemosphere.2021.130843
18. Liang S, Sun C, Liu C, Jiang L, Xie Y, Yan S, et al. The influence of air pollutants and meteorological conditions on the hospitalization for respiratory diseases in Shenzhen City, China. Int J Environ Res Public Health. (2021) 18:5120. doi: 10.3390/ijerph18105120
19. Slama A, Sliwczynski A, Woznica-Pyzikiewicz J, Zdrolik M, Wisnicki B, Kubajek J, et al. The short-term effects of air pollution on respiratory disease hospitalizations in 5 cities in Poland: comparison of time-series and case-crossover analyses. Environ Sci Pollut Res Int. (2020) 27:24582–90. doi: 10.1007/s11356-020-08542-5
20. Chen H, Cheng Z, Li M, Luo P, Duan Y, Fan J, et al. Ambient air pollution and hospitalizations for ischemic stroke: a time series analysis using a distributed lag nonlinear model in Chongqing, China. Front Public Health. (2021) 9:762597. doi: 10.3389/fpubh.2021.762597
21. Goudarzi G, Geravandi S, Idani E, Hosseini SA, Baneshi MM, Yari AR, et al. An evaluation of hospital admission respiratory disease attributed to sulfur dioxide ambient concentration in Ahvaz from 2011 through 2013. Environ Sci Pollut Res Int. (2016) 23:22001–7. doi: 10.1007/s11356-016-7447-x
22. Peden DB. Mechanisms of pollution-induced airway disease: in vivo studies. Allergy. (1997) 52 (38 Suppl.):37–44. doi: 10.1111/j.1398-9995.1997.tb04869.x
23. Phosri A, Ueda K, Phung V, Tawatsupa B, Honda A, Takano H. Effects of ambient air pollution on daily hospital admissions for respiratory and cardiovascular diseases in Bangkok, Thailand. Sci Total Environ. (2019) 651 :1144–53. doi: 10.1016/j.scitotenv.2018.09.183
24. Tajudin M, Khan MF, Mahiyuddin W, Hod R, Latif MT, Hamid AH, et al. Risk of concentrations of major air pollutants on the prevalence of cardiovascular and respiratory diseases in urbanized area of Kuala Lumpur, Malaysia. Ecotoxicol Environ Saf. (2019) 171:290–300. doi: 10.1016/j.ecoenv.2018.12.057
25. Newell K, Kartsonaki C, Lam K, Kurmi O. Cardiorespiratory health effects of gaseous ambient air pollution exposure in low and middle income countries: a systematic review and meta-analysis. Environ Health. (2018) 17:41. doi: 10.1186/s12940-018-0380-3
26. Chang Q, Zhang H, Zhao Y. Ambient air pollution and daily hospital admissions for respiratory system-related diseases in a heavy polluted city in Northeast China. Environ Sci Pollut Res Int. (2020) 27:10055–64. doi: 10.1007/s11356-020-07678-8
27. Filippini M, Masiero G, Steinbach S. The impact of ambient air pollution on hospital admissions. Eur J Health Econ. (2019) 20:919–31. doi: 10.1007/s10198-019-01049-y
28. Lu F, Zhou L, Xu Y, Zheng T, Guo Y, Wellenius GA, et al. Short-term effects of air pollution on daily mortality and years of life lost in Nanjing, China. Sci Total Environ. (2015) 536:123–9. doi: 10.1016/j.scitotenv.2015.07.048
29. Zhu J, Zhang X, Zhang X, Dong M, Wu J, Dong Y, et al. The burden of ambient air pollution on years of life lost in Wuxi, China, 2012-2015: a time-series study using a distributed lag non-linear model. Environ Pollut. (2017) 224:689–97. doi: 10.1016/j.envpol.2017.02.053
30. Dong J, Liu Y, Bao H. Revalue associations of short-term exposure to air pollution with respiratory hospital admissions in Lanzhou, China after the control and treatment of current pollution. Int J Hyg Environ Health. (2021) 231:113658. doi: 10.1016/j.ijheh.2020.113658
31. Clougherty JE. A growing role for gender analysis in air pollution epidemiology. Environ Health Perspect. (2010) 118:167–76. doi: 10.1289/ehp.0900994
32. Sorensen M, Daneshvar B, Hansen M, Dragsted LO, Hertel O, Knudsen L, et al. Personal PM2.5 exposure and markers of oxidative stress in blood. Environ Health Perspect. (2003) 111:161–6. doi: 10.1289/ehp.111-1241344
33. Qiu H, Yu H, Wang L, Zhu X, Chen M, Zhou L, et al. The burden of overall and cause-specific respiratory morbidity due to ambient air pollution in Sichuan Basin, China: a multi-city time-series analysis. Environ Res. (2018) 167:428–36. doi: 10.1016/j.envres.2018.08.011
34. Ding PH, Wang GS, Guo YL, Chang SC, Wan GH. Urban air pollution and meteorological factors affect emergency department visits of elderly patients with chronic obstructive pulmonary disease in Taiwan. Environ Pollut. (2017) 224:751–8. doi: 10.1016/j.envpol.2016.12.035
35. Guo J, Ma M, Xiao C, Zhang C, Chen J, Lin H, et al. Association of air pollution and mortality of acute lower respiratory tract infections in shenyang, China: a time series analysis study. Iran J Public Health. (2018) 47:1261–71.
36. Zhu F, Ding R, Lei R, Cheng H, Liu J, Shen C, et al. The short-term effects of air pollution on respiratory diseases and lung cancer mortality in Hefei: a time-series analysis. Respir Med. (2019) 146:57–65. doi: 10.1016/j.rmed.2018.11.019
37. Ban J, Xu D, He MZ, Sun Q, Chen C, Wang W, et al. The effect of high temperature on cause-specific mortality: a multi-county analysis in China. Environ Int. (2017) 106:19–26. doi: 10.1016/j.envint.2017.05.019
38. Cheng J, Xu Z, Bambrick H, Su H, Tong S, Hu W. The mortality burden of hourly temperature variability in five capital cities, Australia: time-series and meta-regression analysis. Environ Int. (2017) 109:10–9. doi: 10.1016/j.envint.2017.09.012
39. Zhao Q, Zhao Y, Li S, Zhang Y, Wang Q, Zhang H, et al. Impact of ambient temperature on clinical visits for cardio-respiratory diseases in rural villages in northwest China. Sci Total Environ. (2018) 612:379–85. doi: 10.1016/j.scitotenv.2017.08.244
40. Carey IM, Atkinson RW, Kent AJ, van Staa T, Cook DG, Anderson HR. Mortality associations with long-term exposure to outdoor air pollution in a national English cohort. Am J Respir Crit Care Med. (2013) 187:1226–33. doi: 10.1164/rccm.201210-1758OC
41. Wang L, Liu C, Meng X, Niu Y, Lin Z, Liu Y, et al. Associations between short-term exposure to ambient sulfur dioxide and increased cause-specific mortality in 272 Chinese cities. Environ Int. (2018) 117:33–9. doi: 10.1016/j.envint.2018.04.019
42. Yorifuji T, Kashima S, Suryadhi M, Abudureyimu K. Acute exposure to sulfur dioxide and mortality: historical data from Yokkaichi, Japan. Arch Environ Occup Health. (2019) 74:271–8. doi: 10.1080/19338244.2018.1434474
43. Yang Y, Cao Y, Li W, Li R, Wang M, Wu Z, et al. Multi-site time series analysis of acute effects of multiple air pollutants on respiratory mortality: a population-based study in Beijing, China. Sci Total Environ. (2015) 508:178–87. doi: 10.1016/j.scitotenv.2014.11.070
44. Liu M, Xue X, Zhou B, Zhang Y, Sun B, Chen J, et al. Population susceptibility differences and effects of air pollution on cardiovascular mortality: epidemiological evidence from a time-series study. Environ Sci Pollut Res Int. (2019) 26:15943–52. doi: 10.1007/s11356-019-04960-2
45. Goldman GT, Mulholland JA, Russell AG, Strickland MJ, Klein M, Waller LA, et al. Impact of exposure measurement error in air pollution epidemiology: effect of error type in time-series studies. Environ Health. (2011) 10:61. doi: 10.1186/1476-069X-10-61
46. WHO. WHO Air Quality Guidelines for Particulate Matter, Ozone, Nitrogen Dioxide and Sulfur Dioxide. Summary of Risk Assessment. World Health Organization Regional Office for Europe (2006).
47. (2012 2012-02-29). Ambient Air Quality Standard. Available online at: http://www.mee.gov.cn/ywgz/fgbz/bz/bzwb/dqhjbh/dqhjzlbz/201203/W020120410330232398521.pdf
Keywords: sulfur dioxide, air pollution, respiratory disease, inpatient visits, time-series analysis, generalized additive models, distributed lag non-linear models
Citation: Zhou X, Gao Y, Wang D, Chen W and Zhang X (2022) Association Between Sulfur Dioxide and Daily Inpatient Visits With Respiratory Diseases in Ganzhou, China: A Time Series Study Based on Hospital Data. Front. Public Health 10:854922. doi: 10.3389/fpubh.2022.854922
Received: 14 January 2022; Accepted: 01 March 2022;
Published: 31 March 2022.
Edited by:
Zhiwei Xu, The University of Queensland, AustraliaReviewed by:
Mohammad Javad Mohammadi, Ahvaz Jundishapur University of Medical Sciences, IranCopyright © 2022 Zhou, Gao, Wang, Chen and Zhang. This is an open-access article distributed under the terms of the Creative Commons Attribution License (CC BY). The use, distribution or reproduction in other forums is permitted, provided the original author(s) and the copyright owner(s) are credited and that the original publication in this journal is cited, in accordance with accepted academic practice. No use, distribution or reproduction is permitted which does not comply with these terms.
*Correspondence: Xiaokang Zhang, emhhbmd4aWFva2FqdUAxNjMuY29t
Disclaimer: All claims expressed in this article are solely those of the authors and do not necessarily represent those of their affiliated organizations, or those of the publisher, the editors and the reviewers. Any product that may be evaluated in this article or claim that may be made by its manufacturer is not guaranteed or endorsed by the publisher.
Research integrity at Frontiers
Learn more about the work of our research integrity team to safeguard the quality of each article we publish.