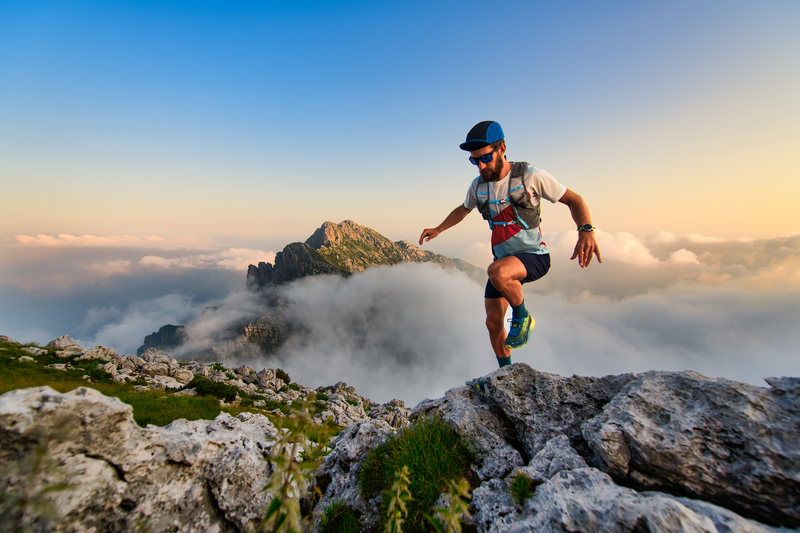
95% of researchers rate our articles as excellent or good
Learn more about the work of our research integrity team to safeguard the quality of each article we publish.
Find out more
ORIGINAL RESEARCH article
Front. Public Health , 03 March 2022
Sec. Digital Public Health
Volume 10 - 2022 | https://doi.org/10.3389/fpubh.2022.849322
This article is part of the Research Topic Voice Technology and Conversational Agents in Health Care Delivery View all 6 articles
Background: About 23% of households in the United States have at least one child who has special healthcare needs. As most care activities occur at home, there is often a disconnect and lack of communication between families, home care nurses, and healthcare providers. Digital health technologies may help bridge this gap.
Objective: We conducted a pre-post study with a voice-enabled medical note taking (diary) app (SpeakHealth) in a real world setting with caregivers (parents, family members) of children with special healthcare needs (CSHCN) to understand feasibility of voice interaction and automatic speech recognition (ASR) for medical note taking at home.
Methods: In total, 41 parents of CSHCN were recruited. Participants completed a pre-study survey collecting demographic details, technology and care management preferences. Out of 41, 24 participants completed the study, using the app for 2 weeks and completing an exit survey. The app facilitated caregiver note-taking using voice interaction and ASR. An exit survey was conducted to collect feedback on technology adoption and changes in technology preferences in care management. We assessed the feasibility of the app by descriptively analyzing survey responses and user data following the key focus areas of acceptability, demand, implementation and integration, adaptation and expansion. In addition, perceived effectiveness of the app was assessed by comparing perceived changes in mobile app preferences among participants. In addition, the voice data, notes, and transcriptions were descriptively analyzed for understanding the feasibility of the app.
Results: The majority of the recruited parents were 35–44 years old (22, 53.7%), part of a two-parent household (30, 73.2%), white (37, 90.2%), had more than one child (31, 75.6%), lived in Ohio (37, 90.2%), used mobile health apps, mobile note taking apps or calendar apps (28, 68.3%) and patient portal apps (22, 53.7%) to track symptoms and health events at home. Caregivers had experience with voice technology as well (32, 78%). Among those completed the post-study survey (in Likert Scale 1–5), ~80% of the caregivers agreed or strongly agreed that using the app would enhance their performance in completing tasks (perceived usefulness; mean = 3.4, SD = 0.8), the app is free of effort (perceived ease of use; mean = 3.2, SD = 0.9), and they would use the app in the future (behavioral intention; mean = 3.1, SD = 0.9). In total, 88 voice interactive patient notes were generated with the majority of the voice recordings being less than 20 s in length (66%). Most noted symptoms and conditions, medications, treatment and therapies, and patient behaviors. More than half of the caregivers reported that voice interaction with the app and using transcribed notes positively changed their preference of technology to use and methods for tracking symptoms and health events at home.
Conclusions: Our findings suggested that voice interaction and ASR use in mobile apps are feasible and effective in keeping track of symptoms and health events at home. Future work is suggested toward using integrated and intelligent systems with voice interactions with broader populations.
The Maternal and Child Health Bureau defines children with special healthcare needs (CSHCN) as children “who have or are at increased risk for a chronic physical, developmental, behavioral, or emotional condition and who also require health and related services of a type or amount beyond that required by children generally” (1). Approximately 23% of households in the United States have at least one CSHCN (2). Since many care activities occur at home, outside of the clinic, caregivers (usually “unpaid caregivers” such as parents and family members) provide daily care, by tracking symptoms, medications, and health events. In addition, caregivers are frequently tasked with communicating with healthcare providers, medical suppliers, insurance companies and schools to coordinate care services. Medical diaries are often used to keep medical notes, to note symptoms and medications given, and to communicate with healthcare providers (HCP) (3). There is room for improvement for care management of CSHCN with digital health technologies.
The COVID-19 pandemic has increased the need for digital health technologies (DHT), especially for health management and tracking (4), remote patient monitoring (5), and telehealth (6). Investments in DHT have raised 14.7 billion USD at the first half of 2021 (7), with top funded fields focused on chronic and non-communicable conditions. In parallel, there is a growing body of literature and funded research related to digital health use in the pediatric domain (8). Studies support that available DHT (e.g., mobile phones, apps, sensors, text messages, websites) play a key role in facilitating care management and care communications (9, 10). Connected and integrated DHT (e.g., patient portals) facilitates timely communication of health activities and medical notes, reducing information barriers and improving continuous care for pediatric patients without being at the clinic (11–13). Literature further demonstrates the evidence on DHT's efficacy, feasibility, and utility in the pediatric domain (10, 14–17). However, the success of DHT in care management and communication is dependent on caregivers and patients capturing and sharing health events that occur outside of the clinic.
To improve the collection of patient notes, convenience is an important factor (18), especially for caregivers of CSCHN, who need to track care activities regularly (e.g., medications, treatment, therapies, etc.). Current digital health tools are crafted toward collecting structured patient health data and use pre-defined mechanisms to capture information (e.g., survey, checklist). Such mechanisms may be limited and miss the narratives surrounding health events (19). In addition, it can be challenging for caregivers of CSHCN to take complete notes of medical events. This is especially true at times when both their hands are devoted to providing care for their child.
To address this gap, we proposed a voice-interactive app, SpeakHealth, which enables caregivers to take medical notes through voice interaction and an automatic speech recognition (ASR) system without depending on typing or focusing on a device or screen. Voice interactive technologies (e.g., voice assistants) and ASR algorithms have been improving over the years. They enable users to command and interact with digital tools using speech and dialogue mechanisms and show promise for a variety of healthcare uses (20–23). In our earlier work (18), we prototyped the SpeakHealth app and collected feedback from parents and healthcare providers which informed the design and features of the app. In this study, we aimed to test the feasibility of the SpeakHealth app in a real world setting through a pre-post study. Participants reported their care and technology preference characteristics (pre-study), used the voice interactive app for 2-weeks to keep their medical notes, and reported their perceived care preference changes and technology adoption informed by the technology acceptance model (post-study) (24). Bowen et al.'s (25) key focus areas were used to synthesize feasibility of the app, and self-reported responses and app data was used to synthesize perceived effectiveness.
Building on top of the previous prototype (18), we improved user interface, features, accessibility to note taking and reviewing. The SpeakHealth app was written in JavaScript using React Native, a cross-platform development library, which accelerated development compared to writing native code for iOS (26). Data is stored, processed, and retrieved from the cloud using Amazon Web Services (AWS) (27). In particular, Cognito facilitates sign in and user creation, AppSync provides an API to perform CRUD operations on the data store, DynamoDB. S3 was used to store audio files, and lambda functions handled the transcription process. The backend was written using the AWS CDK, which describes the infrastructure as code to allow quick deployment and updating. Communication between the frontend and backend is handled using the AWS Amplify library.
Caregivers activated SpeakHealth using the Siri Shortcut “start SpeakHealth”. The app recorded the audio note until manually stopped or after the sixty-second time limit was reached (Figure 1). Then, the audio note was sent to AWS (Amazon Web Services) which performed the transcription using AWS Transcribe (28). Once the job was completed, the app updated to include the audio note's transcription. Figure 1 further outlines the app's functionalities. Caregivers were able to redo or create new audio and text notes, correct transcriptions, and delete existing notes. Caregivers could then group and categorize individual notes into reports. Reports allow caregivers to organize notes for their own benefit or to easily show grouped notes to the child's provider. Reports could also be exported as PDF for easy sharing. The app included a portal to allow caregivers to view patient medications and appointments. However, during the testing period, not all participants were able to sign into the portal due to technical errors.
The study was designed as a pre-post study, where the SpeakHealth app was proposed as a tool for care management. Changes in participant's preferences and care management behavior were observed through pre-post surveys and data collected through the app (voice notes and transcriptions).
Parents were invited to participate in the study through a non-probability sampling method using the network of Nationwide Children's Hospital (Columbus, OH). We sent email invitations via hospital mail-list and to the parents of children receiving care at NCH complex care clinic. The study was announced through digital boards and hospital social media channels. In addition, we worked with a local community partner (OhioF2F) to announce the study. The recruitment was time-bound, and participants were recruited between October- December 2020. Even though we are not able to access total online reach numbers, we were able to send email invites to 644 out of 1,290 patient families of children who received care at the complex care clinic in 2020.
The inclusion criteria: (1) being a parent of children who have been diagnosed with one or multiple complex medical conditions (2) being a user of an iPhone 8 (or above) or iPhone with iOS 13 or above during the study period. Forty-one participants met the inclusion criteria, consented to participate in the study and completed the introduction survey. Out of 41, 12 participants only filled out the introduction survey. Four participants filled out the introduction survey and used the app for 2 weeks. Twenty-four participants completed the full study by filling out the introduction survey, using the app for 2 weeks and submitting responses for the exit survey. Participants were compensated with a gift card up to $30 ($10 for completing the introduction survey, +$10 for participating for 2-weeks of app use, +$10 for completing the exit survey). Institutional review board of Nationwide Children's Hospital approved this study (#00000231).
Interested participants were directed to a RedCap online survey (29), where they completed eligibility screening. Eligible participants received an email providing details about the study and a link to an online consent form. Once consented, participants filled out an introduction survey, which included questions about demographics, the child's medical conditions, mobile app use and voice interactive technologies, care coordination and healthcare management, app needs and expectations (Tables 1–4 provided details on introduction survey content). Following the introduction survey, participants were guided through online tutorials for how to install and use of the app. Participants used the app for at least 2 weeks. During this period, they received periodic email reminders providing quick tips about app features (Appendix 1). Voice notes and transcriptions created through the app were collected and stored at AWS servers.
Table 3. Technology interaction in symptoms tracking symptoms, health events and care activities (n = 41).
At the end of this period, participants received exit surveys, which included questions about care coordination and healthcare management to assess any changes in behavior toward care activities, symptom tracking and health events after using the app. Additionally, participants responded to questions about assessing their adoption of the app. We used technology acceptance model constructs (perceived usefulness, perceived ease of use and behavioral intention) to develop this adoption questionnaire (24). Participants responded using 5-point Likert scales (0: Strongly disagree, 1: Disagree, 2: Neither agree nor disagree, 3: Agree, 4: Strongly agree).
All the data were descriptively analyzed and reported. We used descriptive analysis guided by Creswell and Creswell (30). We reported measures as frequency, mean, standard deviation, score distribution and relative ranking comparisons within the groups. The analysis was conducted separately for pre-study and post-study surveys. We used Microsoft Office tools for analysis and reporting.
The majority of the parents were 35–44 years old (22, 53.7%), part of a two-parent household (30, 73.2%), white (37, 90.2%), had more than one child (31, 75.6%), lived in Ohio (37, 90.2%), had a yearly household income higher than the median for the U.S. [$67.521 (31)] (29, 72.5%) and had received higher level education (undergraduate or graduate degrees) (25, 61%) (Table 1).
The children with special health care needs ranged from 1 to 17 years old with an average of 7.5 years old (SD = 4.2). More than half of CSHCN had developmental delay (32, 78%), learning disability (23, 56.1%), speech problems (23, 56.1%), and vision problems (23, 56.1%). In addition, a substantial amount of CSHCN had intellectual disability, seizures, joint or muscle problems, cerebral palsy, epilepsy, asthma, hearing problems, brain injury, neurologic and neuromuscular disorders, and other genetic disorders. The majority received daily prescribed medication (35, 85.4%), physical and occupational therapy (33, 80.5%), behavioral or speech therapy (27, 65.9%), used a feeding tube (22, 53.7%) or used a wheelchair (22, 53.7%). Most of the caregivers have been tracking symptoms and health activities at home frequently (all the time or often, 29, 70.8%). These included following appointments (37, 90.2%), medications and refills (30, 73.2%), and tracking symptoms (26, 63.4%) (Table 2).
The majority of caregivers had been using mobile health apps, mobile note taking apps or calendar apps (28, 68.3%) and patient portal apps (22, 53.7%) to track symptoms, health events and care activities at home (Table 3). In addition, there was a preference for taking notes on a paper or card (16, 39%) or in a dedicated notebook or calendar (15, 36.6%). Mobile phones and apps had higher ratings and preference as the ideal tool or technology (37, 90.2%), followed by pen and paper or keeping notebooks (16, 39.0%), voice assistants (13, 31.7%), tablet PC or iPad (10, 24.4%), and laptop or personal computers (7, 17.1%). Care management related information seeking activities were primarily completed through nurse or doctor calls (25, 61%) and web or internet searches (24, 58.5%).
The caregivers had experience with voice technology, with the majority using voice assistants over their smartphones (32, 78%) for more than a year. In addition, almost half of them had owned voice interactive devices or smart speakers (23, 56.1%) for more than a year (Table 4).
Feedback on the voice interactive app adoption was collected using technology acceptance model (24). Figure 2 illustrates app adoption questionnaire and response frequency distribution grouped under TAM constructs. Approximately 80% of the caregivers agreed or strongly agreed that using the SpeakHealth app would enhance their performance in completing tasks (perceived usefulness; mean = 3.4, SD = 0.8), the app is free of effort (perceived ease of use; mean = 3.2, SD = 0.9), and they would use the app in the future (behavioral intention; mean = 3.1, SD = 0.9). Given the small sample size of respondents (n = 23, one participant's response was removed due to highly missing data), we were not able to do statistical analysis toward explaining app use behavior. We reported the frequency distribution of responses and mean value to report user perceptions in item and construct level.
Figure 2. Technology adoption responses grouped under technology acceptance model constructs. Number of responses/total responses is given in each bar chart.
In total, 95 patient notes were taken (after removing 19 “test” notes, which are created by users to try out the app). Seven out of 95 notes were taken through text entry. We had one “super-user” participant who kept 29 notes throughout the study period. Excluding that, each remaining caregiver who used the app (n = 23) kept 4 notes in average (SD = 2.6). Out of 88 voice interactive patient notes, most of the notes were taken in <10 s or were between 11 and 20 s in length (Table 5). Only one caregiver created note reports.
The voice interactive notes were usually taken for symptoms and conditions (“Right shoulder pain…”, “Spot on lip is gone. Overall doing well. Has a runny nose it no fever or any other symptoms.”, “Knees hurting today…”), medication (“Gave [patient name] 2 Benadryl at 6:00am…”, “[patient name] does not take his medicine after lunch.”), treatment or therapy (“7 pm trach care completed…”), mood or behavior (“[patient name] was so happy that we understand him…“), seizure (“Starting seizure. Touched his arm no response. Eyes fixed. Lasted only a few seconds. Looked as though [patient] was staring right through me”), appointments (“Appointment this afternoon… mom took [patient name]”), vital signs (“[patient name] oxygen was still hanging out around 80 today”, “...blood sugar is 127.”), personal notes (“it was pointless”, “[patient] is still having a lot of pain, but we haven't had to give the strong meds again yet”, “I'm so proud of my baby”), Sleep (“[Patient name] still not sleeping through the night. [patient] is still waking up and crying”), nutrition (“Only one feed for tomorrow, 200 ml”) explaining process/procedure (“Yesterday we started…gabapentin at a rate of 2.6 ml that will continue for one week, then we will switch rate to 2 ml over the course of another week, then 1.6 ml for another week with final rate at 0.6 ml...”) bowel movements (“BM today, formed small”). A single note or entry may have multiple themes. Some notes contained a summary of the day instead of individual instances created throughout a day. For example, a caregiver may prefer to take a note about symptom, treatment, procedure, medication, and patient's mood all in one note.
Metadata included in the notes stored in AWS showed that 14 of the audio transcriptions were edited after being created, indicating errors in the transcriptions that were corrected by the participants. This was determined by comparing the timestamp of the note created against last updated and whether the note was considered a transcribed audio recording linked to an audio file. The note data was not versioned, meaning we cannot view the original transcription and how the errors were corrected. Also, since AWS Transcribe is constantly changing and improving, it is unlikely we could recreate the original transcriptions from the collected audio recordings.
Caregivers provided their feedback about their preference changes after they completed using the SpeakHealth app for at least 2 weeks (Figure 3). More than half of the caregivers reported that voice interaction with the SpeakHealth app and using transcribed notes changed their preference of technology to use (13/21, 59%) and methods for tracking symptoms and health events at home positively (14/24, 58%). Half of the caregivers changed the frequency of tracking symptoms and health events (11/22, 50%).
We conducted a feasibility study of a voice-enabled medical note taking (diary) app in a real world setting with caregivers (parents, family members) of children with special healthcare needs (CSHCN) to understand the impact and implications of voice interaction and automatic speech recognition for medical note taking at home. The majority of participants were young parents, with a college degree or above education, middle income level, living in a two-parent household with multiple children. Demographically, we were unable to achieve higher diversity in our data, which would have been available from a more heterogeneous group in terms of race, family type, income, and education. However, CSHCN of participants were representative, as they had various and multiple chronic conditions, and a need for frequent care management (medication, treatments, symptom tracking). The reported conditions and care management needs are in line with the national survey of CSHCN (2), the majority of which require specific care services such as medication, treatment, therapy, feeding and mobility support. Similarly, this confirms the need for care management and coordination including the ability to track health events and symptoms at home, as well as tools for easier provider communications (32, 33).
Mobile tools and technologies were the primary preference for tracking health activities and taking notes, and they were also used for health information seeking activities as part of care management. This finding aligns with the overall trend and adoption in mobile technology ownership in the U.S. (34) and caregiver preferences (13). Specifically, online patient portals have been widely used among participants. The primary reason may be that they enable direct communication with HCPs, and their wide availability through hospitals to communicate with caregivers and telehealth visits especially after the pandemic (33). This preference is supported by the common trend of using mobile apps in pediatric care management and care coordination (13, 18). Parental familiarity and use of voice assistants via smartphones and smart speakers are a promising indicator toward future utilization of voice interaction in care management. It will need to be built integrated with current healthcare technologies, moving the needle from voice interaction being primarily used for health seeking activities (21, 35) and health screening (20, 36) to the area of care management.
Using the data collected pre and post study, the feasibility of the app was interpreted by descriptively analyzing survey responses and user data following key focus areas adapted from Bowen et al. (25): acceptability, demand, implementation and integration, adaptation, and expansion. Perceived effectiveness was interpreted through survey responses about the perceived changes in the preferences in mobile apps used for symptom tracking and recording health activities.
In response to the voice interactive app adoption survey (n = 23), the majority of participants agreed that the SpeakHealth app is easy to use, useful for keeping notes and tracking health events, and that they would prefer to use SpeakHealth in the long term. Furthermore, the survey showed that SpeakHealth met parents' preferences toward the use of mobile technologies and voice interactions. Mobile apps showed similar early adoption trends among parents for care management (37). As voice technologies become more aligned with current habits and lifestyles, increased adoption of the technology can be expected.
User data for voice interactive notes showed that caregivers are interested in voice engagement and note taking for shorter notes most of the time (<20 s), and toward noting symptoms and conditions, medications, treatment and therapies, and patient behaviors. Shorter notes principally increase effective note taking, and potentially accuracy in transcriptions since shorter sentences are often less complex. Voice engagement may change note taking behavior over time, especially when integrated with other smart devices (e.g., smart speakers). Multimodal voice interactive technologies should be implemented in the future to assess acceptability using multiple platforms and digital ecosystems.
The overall demand for mobile apps and voice technologies is increasing. As of today, 97% of the U.S. adult population have a mobile device (34) and 35% own smart speakers (38). This suggests potential infrastructure availability, as well as general awareness and demand for these communication tools. In our study, even though we did not have the equal race distribution, we had participants from low income (n = 6) and limited education populations (no degree or high school degree, n = 11), yet overall feedback toward using mobile and voice interactive apps was positive. Similar to the literature, there was a demand on mobile technology to improve health outcomes (39, 40). The responses to the adoption survey and the collected voice interactive notes show the distribution of technology and voice interaction across demographics. However, for further implementations, digital equity, access, and digital literacy should be assessed to improve engagement of voice interactive apps.
The implementation of voice engagement through SpeakHealth was convenient, since caregivers were able to use the app on their own phone and to access the note taking services. SpeakHealth was low cost to develop and nested in the integrated ecosystem of the mobile phone, allowing users to install and use the app without needing any added instruction. Also, the ratio between voice interactive notes taken and notes being edited was low, indicating AWS ASR was able to capture the intended text most of the time. Overall, mobile platforms and ASR technology showed competence in our study. Even so, we do not have the knowledge of what errors led to participants editing transcriptions during the study. Potentially they could be related to complex sentences or medical terms or interference from noisy environments (41). Should that be the case, such occurrences may potentially lead to frustration over time.
In our study, we provided a glimpse of how speech technologies can be utilized in care management for caregivers with CSHCN. Large scale adaptation and expansion of the voice interactive care management tools is possible. As Amazon, Google and Microsoft provide more HIPAA compliant AI-as-a-services (which range from ASR, NLP models, speech-to-text, text-to-speech, and translation mechanisms) modern cloud and mobile application ecosystems will become increasingly easier to integrate into patient-facing apps at scale (with minimal quality of service loss for large user bases and populations). Such services are constantly improving, and the accuracy can be expected to increase over time (e.g., Amazon Medical Scribe for medical terms in transcriptions) (42).
After using SpeakHealth, caregivers shared their feedback on their perceived changes toward technology use, frequency and methods in tracking symptoms and health events. They displayed a preference to use voice interactive apps for note taking activities and increase in perceived effectiveness in care management. Even though it is limited observation, the change is promising that voice interactive apps may increase the frequency of tracking symptoms over time. Effectiveness of voice interaction has already been demonstrated in daily use (43, 44). However, our observation should be further investigated with a larger sample size (and data points) in a longitudinal study.
The convenience of using natural language instead of a screen gives caregivers the ability to take ongoing notes on care management while simultaneously providing home care. It allows for better family engagement and less distraction, especially when a caregivers' attention is primarily directed toward caring for a child. Voice assistant-based apps have already been implemented for care management among the adult and elder populations with chronic conditions (45). In the future, SpeakHealth-like mechanisms could be integrated with smart devices featuring ambient implementation with voice assistants to be a valuable partner in care management (46), building early warning mechanisms for personalized health (19) and public health (20), contributing to personal medical notes as supporting the shared decision making (47), medication adherence and treatment (48) and improving patient-reported outcomes with integration into the electronic medical records (49).
The use of “defaults” in technology (Siri as default voice assistant) and human nature (speech as default communication mechanism) creates a window of opportunity to improve human-computer engagement (22, 50, 51). A voice user interface (VUI) with applications helps to overcome barriers of language, literacy and improve digital equity and telehealth practices. Interoperable and integrated systems with hospital EHR may also improve care coordination, and eventually reduce hospital admissions (52). Health outcomes could be informed by the rich unstructured patient-generated health data (PGHD) (19) and speech biomarkers collected through the audio notes (53). Yet, practitioners should be aware of potential infrastructure, legal and practical barriers implementing voice interactive healthcare practices (20, 21, 54, 55).
The strength of our study was that it provided a new perspective about the cohort of caregivers of CSHCN, by investigating their preferences, perceptions and engagement using one of the emerging technologies in health care. The results can present an understanding of the responses of participants to create outcome measures in the future.
In our study, we had several limitations. The recruitment was online, therefore verifying parents meeting inclusion criteria was limited. We were not able to gather the total number of reaches as social media, therefore the response rate is unknown. We had a high number of dropouts from the pre-survey group, which limited the analysis. We did not conduct sample size calculations, but this study will help to estimate the effective sample size for future larger scale studies. We were not able to investigate association between voice notes, condition, and demographics due to limited sample size and voice notes taken through statistical analysis. Similarly, we were not able to measure health outcomes or comparative effectiveness of the app use (vs. currently used methods) through standardized measures, and therefore, only able to report perceived effectiveness through the post-study survey. In addition, the demographic distribution skewed toward white race, higher education received, middle income, younger and technology user parents. More input from marginalized or underserved groups regarding the use of voice interactive technologies is needed, as the results could be impacted by the performance of ASR (56). The study duration was limited (2-weeks) to achieve long term observation toward behavior change in note taking and assess the impact of the app. Finally, our study did not capture differences between entered text and transcribed notes.
We reported a pre-post study for assessing feasibility of voice interaction and ASR via a mobile app (SpeakHealth). This implementation mimicked the interaction and a real-world use of mainstream voice assistants on smart devices. Our findings suggest that voice interaction and ASR use in mobile apps are feasible and effective in keeping track of symptoms and health events at home. Future work is suggested toward integrated and intelligent systems with broader populations.
The datasets presented in this article are not publicly available due to the inclusion of personal health information (PHI). Requests to access the datasets should be directed to ZW1yZS5zZXpnaW5AbmF0aW9ud2lkZWNoaWxkcmVucy5vcmc=.
The studies involving human participants were reviewed and approved by Institutional review board of Nationwide Children's Hospital. The patients/participants provided their written informed consent to participate in this study.
ES, YH, and GN conceived of the presented idea. ES developed the research design, conducted the study, and drafted the manuscript. GN assisted the recruitment. YH and GN supported and supervised the study. BO developed the app. BA supported the app design. All authors discussed the results and contributed to the final manuscript.
This study was supported by the Health Resources and Services Administration Maternal and Child Health Bureau Grand Challenge for Care Coordination for CSHCN (# 720467022000).
The authors declare that the research was conducted in the absence of any commercial or financial relationships that could be construed as a potential conflict of interest.
All claims expressed in this article are solely those of the authors and do not necessarily represent those of their affiliated organizations, or those of the publisher, the editors and the reviewers. Any product that may be evaluated in this article, or claim that may be made by its manufacturer, is not guaranteed or endorsed by the publisher.
Authors are thankful to SpeakHealth stakeholder and advisory board members, John Luna, Dan Digby, Simon Linwood, Amad Hussain, Carrie Robinson, Justin Jackson and OhioF2F foundation for their support and contribution to our project.
The Supplementary Material for this article can be found online at: https://www.frontiersin.org/articles/10.3389/fpubh.2022.849322/full#supplementary-material
1. McPherson M, Arango P, Fox H, Lauver C, McManus M, Newacheck PW, et al. New definition of children with special health care needs. Pediatrics. (1998) 102:137–40. doi: 10.1542/peds.102.1.137
2. The National Survey of Children With Special Health Care Needs: Chartbook 2009-2010. US Department of Health and Human Services Health Resources and Services Administration (2013). Available online at: https://mchb.hrsa.gov/sites/default/files/mchb/data-research/nscsh-chartbook-06-2013.pdf (accessed January, 2022).
3. Chew C-C, Hss A-S, Chan H-K, Hassali MA. Medication safety at home: a qualitative study on caregivers of chronically ill children in Malaysia. Hosp Pharm. (2020) 55:405–11. doi: 10.1177/0018578719851719
4. Abrams EM, Szefler SJ. Managing asthma during coronavirus disease-2019: an example for other chronic conditions in children and adolescents. J Pediatr. (2020) 222:221–6. doi: 10.1016/j.jpeds.2020.04.049
5. Tabacof L, Kellner C, Breyman E, Dewil S, Braren S, Nasr L, et al. Remote patient monitoring for home management of coronavirus disease 2019 in New York: a cross-sectional observational study. Telemed J E Health. (2021) 27:641–8. doi: 10.1089/tmj.2020.0339
6. McFarland S, Coufopolous A, Lycett D. The effect of telehealth versus usual care for home-care patients with long-term conditions: a systematic review, meta-analysis and qualitative synthesis. J Telemed Telecare. (2021) 27:69–87. doi: 10.1177/1357633X19862956
7. H1 2021 Digital Health Funding: Another Blockbuster Year…in Six Months. Rock Health (2021). Available online at: https://rockhealth.com/insights/h1-2021-digital-health-funding-another-blockbuster-year-in-six-months/ (accessed December 16, 2021).
8. Riley WT, Oh A, Aklin WM, Sherrill JT, Wolff-Hughes DL, Diana A, et al. Commentary: pediatric digital health supported by the National Institutes of Health. J Pediatr Psychol. (2019) 44:263–8. doi: 10.1093/jpepsy/jsy108
9. Richardson PA, Harrison LE, Heathcote LC, Rush G, Shear D, Lalloo C, et al. mHealth for pediatric chronic pain: state of the art and future directions. Expert Rev Neurother. (2020) 20:1177–87. doi: 10.1080/14737175.2020.1819792
10. Ramsey RR, Plevinsky JM, Kollin SR, Gibler RC, Guilbert TW, Hommel KA. Systematic review of digital interventions for pediatric asthma management. J Allergy Clin Immunol Pract. (2020) 8:1284–93. doi: 10.1016/j.jaip.2019.12.013
11. Ramsey RR, Guilbert TW. Exciting era of sensor-based electronic monitoring of adherence in pediatric asthma. Pediatrics. (2021) 147:e2020036749. doi: 10.1542/peds.2020-036749
12. Gentles SJ, Lokker C, McKibbon KA. Health information technology to facilitate communication involving health care providers, caregivers, and pediatric patients: a scoping review. J Med Internet Res. (2010) 12:e22. doi: 10.2196/jmir.1390
13. Baysari MT, Westbrook JI. Mobile applications for patient-centered care coordination: a review of human factors methods applied to their design, development, and evaluation. Yearb Med Inform. (2018) 24:47–54. doi: 10.15265/IY-2015-011
14. Ranade-Kharkar P, Weir C, Norlin C, Collins SA, Scarton LA, Baker GB, et al. Information needs of physicians, care coordinators, and families to support care coordination of children and youth with special health care needs (CYSHCN). J Am Med Inform Assoc. (2017) 24:933–41. doi: 10.1093/jamia/ocx023
15. Sezgin E, Weiler M, Weiler A, Lin S. Proposing an ecosystem of digital health solutions for teens with chronic conditions transitioning to self-management and independence: exploratory qualitative study. J Med Internet Res. (2018) 20:e10285. doi: 10.2196/10285
16. Looman WS, Antolick M, Cady RG, Lunos SA, Garwick AE, Finkelstein SM. Effects of a telehealth care coordination intervention on perceptions of health care by caregivers of children with medical complexity: a randomized controlled trial. J Pediatr Health Care. (2015) 29:352–63. doi: 10.1016/j.pedhc.2015.01.007
17. Desai AD, Wang G, Wignall J, Kinard D, Singh V, Adams S, et al. User-centered design of a longitudinal care plan for children with medical complexity. J Am Med Inform Assoc. (2020) 27:1860–70. doi: 10.1093/jamia/ocaa193
18. Sezgin E, Noritz G, Lin S, Huang Y. Feasibility of a voice-enabled medical diary app (SpeakHealth) for caregivers of children with special health care needs and health care providers: mixed methods study. JMIR Form Res. (2021) 5:e25503. doi: 10.2196/25503
19. Hussain S-A, Sezgin E, Krivchenia K, Luna J, Rust S, Huang Y. A natural language processing pipeline to synthesize patient-generated notes toward improving remote care and chronic disease management: a cystic fibrosis case study. JAMIA Open. (2021) 4:ooab084. doi: 10.1093/jamiaopen/ooab084
20. Sezgin E, Huang Y, Ramtekkar U, Lin S. Readiness for voice assistants to support healthcare delivery during a health crisis and pandemic. NPJ Digit Med. (2020) 3:122. doi: 10.1038/s41746-020-00332-0
21. Yang S, Lee J, Sezgin E, Bridge J, Lin S. Clinical advice by voice assistants on postpartum depression: cross-sectional investigation using Apple Siri, Amazon Alexa, Google Assistant, and Microsoft Cortana. JMIR Mhealth Uhealth. (2021) 9:e24045. doi: 10.2196/24045
22. Sezgin E, Militello LK, Huang Y, Lin S A. scoping review of patient-facing, behavioral health interventions with voice assistant technology targeting self-management and healthy lifestyle behaviors. Transl Behav Med. (2020) 10:606–28. doi: 10.1093/tbm/ibz141
23. Bérubé C, Schachner T, Keller R, Fleisch E, Wangenheim F V, Barata F, et al. Voice-based conversational agents for the prevention and management of chronic and mental health conditions: systematic literature review. J Med Internet Res. (2021) 23:e25933. doi: 10.2196/25933
24. Davis FD. Perceived usefulness, perceived ease of use, and user acceptance of information technology. Miss Q. (1989) 13:319–40. doi: 10.2307/249008
25. Bowen DJ, Kreuter M, Spring B, Cofta-Woerpel L, Linnan L, Weiner D, et al. How we design feasibility studies. Am J Prev Med. (2009) 36:452–7. doi: 10.1016/j.amepre.2009.02.002
26. React Native. (2022). Available online at: https://reactnative.dev/ (accessed January 5, 2022).
27. Amazon Web Services (AWS). (2022). Available online at: https://aws.amazon.com/products/ (accessed January 5, 2022).
28. Amazon Web Services - Transcribe. (2022). Available online at: https://aws.amazon.com/transcribe/ (accessed January 5, 2022).
29. Redcap. (2022). Available online at: https://www.project-redcap.org/ (accessed January 5, 2022).
30. Creswell JW, David Creswell J. Research Design: Qualitative, Quantitative, and Mixed Methods Approaches. SAGE Publications (2017).
31. Shrider EA, Kollar M, Chen F, Semega J. Income poverty in the United States: 2020. Current Population Reports US Census Bureau (2021). Available online at: https://www.census.gov/library/publications/2021/demo/p60-273.html (accessed January, 2022).
32. Golden SL, Nageswaran S. Caregiver voices: coordinating care for children with complex chronic conditions. Clin Pediatr. (2012) 51:723–9. doi: 10.1177/0009922812445920
33. Shah NR, Drane A. How health systems can care for caregivers. NEJM Catalyst. (2021) 2. doi: 10.1056/CAT.21.0213
34. Demographics of Mobile Device Ownership and Adoption in the United States (2021). Available online at: https://www.pewresearch.org/internet/fact-sheet/mobile/ (accessed December 20, 2021).
35. Miner AS, Milstein A, Schueller S, Hegde R, Mangurian C, Linos E. Smartphone-based conversational agents and responses to questions about mental health, interpersonal violence, and physical health. JAMA Intern Med. (2016) 176:619–25. doi: 10.1001/jamainternmed.2016.0400
37. Casilang CG, Stonbraker S, Japa I, Halpern M, Messina L, Steenhoff AP, et al. Perceptions and attitudes toward mobile health in development of an exclusive breastfeeding tool: focus group study with caregivers and health promoters in the Dominican Republic. JMIR Pediatr. Parent. (2020) 3:e20312. doi: 10.2196/20312
38. Kinsella B. U.S. Smart Speaker Growth Flat Lined in 2020 (2021). Available online at: https://voicebot.ai/2021/04/14/u-s-smart-speaker-growth-flat-lined-in-2020/ (accessed December 20, 2021).
39. Leziak K, Birch E, Jackson J, Strohbach A, Niznik C, Yee LM. Identifying mobile health technology experiences and preferences of low-income pregnant women with diabetes. J Diabetes Sci Technol. (2021) 15:1018–26. doi: 10.1177/1932296821993175
40. Tripicchio GL, Kay M, Herring S, Cos T, Bresnahan C, Gartner D, et al. Development and preliminary feasibility of iByte4Health: a mobile health (mHealth) pediatric obesity prevention intervention to engage parents with low-income of children 2–9 years. Nutrients. (2021) 13:4240. doi: 10.3390/nu13124240
41. Palanica A, Thommandram A, Lee A, Li M, Fossat Y. Do you understand the words that are comin outta my mouth? Voice assistant comprehension of medication names. NPJ Digit Med. (2019) 2:55. doi: 10.1038/s41746-019-0133-x
42. Palanica A, Fossat Y. Medication name comprehension of intelligent virtual assistants: a comparison of Amazon Alexa, Google Assistant, and Apple Siri Between 2019 and 2021. Front Digit Health. (2021) 3:669971. doi: 10.3389/fdgth.2021.669971
43. Ruan S, Wobbrock JO, Liou K, Ng A, Landay JA. Comparing speech and keyboard text entry for short messages in two languages on touchscreen phones. Proc ACM Interact Mob Wearable Ubiquitous Technol. (2018) 1:1–23. doi: 10.1145/3161187
44. ThinkwithGoogle. Voice Search Mobile Use Statistics - Think with Google. (2019). Available online at: https://www.thinkwithgoogle.com/marketing-strategies/search/voice-search-mobile-use-statistics/ (accessed January 5, 2022).
45. Cheng A, Raghavaraju V, Kanugo J, Handrianto YP, Shang Y. Development and evaluation of a healthy coping voice interface application using the Google home for elderly patients with type 2 diabetes. In: 2018 15th IEEE Annual Consumer Communications Networking Conference (CCNC) (Las Vegas, NV), p. 1–5.
46. Sezgin E, Noritz G, Elek A, Conkol K, Rust S, Bailey M, et al. Capturing at-home health and care information for children with medical complexity using voice interactive technologies: multi-stakeholder viewpoint. J Med Internet Res. (2020) 22:e14202. doi: 10.2196/14202
47. Mafi JN, Gerard M, Chimowitz H, Anselmo M, Delbanco T, Walker J. Patients contributing to their doctors' notes: insights from expert interviews. Ann Intern Med. (2018) 168:302–5. doi: 10.7326/M17-0583
48. Corbett CF, Combs EM, Chandarana PS, Stringfellow I, Worthy K, Nguyen T, et al. Medication adherence reminder system for virtual home assistants: mixed methods evaluation study. JMIR Form Res. (2021) 5:e27327. doi: 10.2196/27327
49. Desai AD, Jacob-Files EA, Wignall J, Wang G, Pratt W, Mangione-Smith R, et al. Caregiver and health care provider perspectives on cloud-based shared care plans for children with medical complexity. Hosp Pediatr. (2018) 8:394–403. doi: 10.1542/hpeds.2017-0242
51. Sezgin E. Can we use commercial mobile apps instead of research mobile apps in healthcare research? Front Public Health. (2021) 9:685439. doi: 10.3389/fpubh.2021.685439
52. Peter S, Chaney G, Zappia T, Van Veldhuisen C, Pereira S, Santamaria N. Care coordination for children with complex care needs significantly reduces hospital utilization. J Spec Pediatr Nurs. (2011) 16:305–12. doi: 10.1111/j.1744-6155.2011.00303.x
53. Robin J, Harrison JE, Kaufman LD, Rudzicz F, Simpson W, Yancheva M. Evaluation of speech-based digital biomarkers: review and recommendations. Digit Biomark. (2020) 4:99–108. doi: 10.1159/000510820
54. Ermolina A, Tiberius V. Voice-controlled intelligent personal assistants in health care: International Delphi Study. J Med Internet Res. (2021) 23:e25312. doi: 10.2196/25312
55. Bickmore TW, Ólafsson S, O'Leary TK. Mitigating patient and consumer safety risks when using conversational assistants for medical information: exploratory mixed methods experiment. J Med Internet Res. (2021) 23:e30704. doi: 10.2196/30704
Keywords: voice interaction, mobile app, automatic speech recognition, children with special healthcare needs, voice assistant, remote care management, feasibility, patient-generated health data (PGHD)
Citation: Sezgin E, Oiler B, Abbott B, Noritz G and Huang Y (2022) “Hey Siri, Help Me Take Care of My Child”: A Feasibility Study With Caregivers of Children With Special Healthcare Needs Using Voice Interaction and Automatic Speech Recognition in Remote Care Management. Front. Public Health 10:849322. doi: 10.3389/fpubh.2022.849322
Received: 05 January 2022; Accepted: 07 February 2022;
Published: 03 March 2022.
Edited by:
Sudip Bhattacharya, Swami Rama Himalayan University, IndiaReviewed by:
Shailesh Kumar Tripathi, Rajendra Institute of Medical Sciences, IndiaCopyright © 2022 Sezgin, Oiler, Abbott, Noritz and Huang. This is an open-access article distributed under the terms of the Creative Commons Attribution License (CC BY). The use, distribution or reproduction in other forums is permitted, provided the original author(s) and the copyright owner(s) are credited and that the original publication in this journal is cited, in accordance with accepted academic practice. No use, distribution or reproduction is permitted which does not comply with these terms.
*Correspondence: Emre Sezgin, ZXNlemdpbjFAZ21haWwuY29t
Disclaimer: All claims expressed in this article are solely those of the authors and do not necessarily represent those of their affiliated organizations, or those of the publisher, the editors and the reviewers. Any product that may be evaluated in this article or claim that may be made by its manufacturer is not guaranteed or endorsed by the publisher.
Research integrity at Frontiers
Learn more about the work of our research integrity team to safeguard the quality of each article we publish.