- 1Department of Information Technology, College of Computer, Qassim University, Buraidah, Saudi Arabia
- 2Department of Mathematical Sciences, Computer Science Unit, Taraba State University, Jalingo, Nigeria
COVID-19 contact-tracing applications (CTAs) offer enormous potential to mitigate the surge of positive coronavirus cases, thus helping stakeholders to monitor high-risk areas. The Kingdom of Saudi Arabia (KSA) is among the countries that have developed a CTA known as the Tawakkalna application, to manage the spread of COVID-19. Thus, this study aimed to examine and predict the factors affecting the adoption of Tawakkalna CTA. An integrated model which comprises the technology acceptance model (TAM), privacy calculus theory (PCT), and task-technology fit (TTF) model was hypothesized. The model is used to understand better behavioral intention toward using the Tawakkalna mobile CTA. This study performed structural equation modeling (SEM) analysis as well as artificial neural network (ANN) analysis to validate the model, using survey data from 309 users of CTAs in the Kingdom of Saudi Arabia. The findings revealed that perceived ease of use and usefulness has positively and significantly impacted the behavioral intention of Tawakkalna mobile CTA. Similarly, task features and mobility positively and significantly influence task-technology fit, and significantly affect the behavioral intention of the CTA. However, the privacy risk, social concerns, and perceived benefits of social interaction are not significant factors. The findings provide adequate knowledge of the relative impact of key predictors of the behavioral intention of the Tawakkalna contact-tracing app.
1. Introduction
The usage of mobile contact-tracing apps (CTAs) has developed exponentially due to the severe impact of COVID-19. One major reason behind this development is as a result of the effort made to contain the spread of the coronavirus (1–3). Stakeholders are concerned about easing movement control and physical distancing (4) and the weaknesses of manual contact tracing (5). These circumstances have presented a compelling reason for stakeholders to depend on digital monitoring, enabling more effective, engaging, and nearly instant tracing of cases than is the case with traditional manual tracing methods (6, 7). The mobile contact-tracing apps are technology-based solutions used to help increase the conventional contact-tracing process. The apps operate by identifying contacts at risk of COVID-19 automatically (2). Hence, the contact-tracing apps exchange information of personal phones within close range, which informs people of infected individuals. Also, the apps serve as an anchor point to inform citizens and provide suggestions on whether they should go to isolation or not.
Many contact-tracing solutions have been introduced by various countries and technology companies (8, 9). Recent advancements by Google and Apple in these efforts are providing crisis managers with many features, such as personalized messages, depending on the mobile user's current geographic location (9). Additionally, mobile phones, due to their ease of use, are influencing citizens, with actual usage statistics demonstrating a consistent rise in adoption (10), indicating that many countries are shifting to technology-based platforms. As a result, several studies have investigated these applications that monitor infected individuals and their surroundings (10, 11). One of the primary issues is the personal information provided by users (2, 12). The use of mobile devices enables data capture that can be shared with third-party developers, analytics, and decision-makers (13). This may raise concerns about the user's privacy, which are even greater when the authorities or other public entities hold such sensitive information (14–16). Furthermore, the many regulations used to protect users' privacy have increased the importance of this issue (17, 18). Hence, all organizations and stakeholders must contribute to knowledge and enhanced understanding of the rights, perceptions, and behaviors of users of CTAs.
Some studies have reported that privacy is the primary concern for users of COVID-19 tracing apps (2, 10). This concern negatively influences the individual's intention to share or disclose personal information, therefore blocking the adoption of the app. Others have indicated that the potential benefits of these apps may outweigh the risks of being exposed to the coronavirus (19). However, contrary opinions have indicated that this is not simply the case of a pandemic, such as COVID-19, as individual social activities are threatened (4). Hence, essential elements influencing the acceptance of CTAs, especially the risk of losing social involvement, have not been well-examined. Moreover, in practice, the apps' effectiveness is frequently promoted as enabling immediate benefits to be obtained by users through factors such as the apps' usefulness. Still, most people hold some concerns about the apps' appropriateness and ease of use: as emphasized in the literature, although appealing, the apps are fraught with issues (10, 20).
Although, Kaspar (21) highlighted that most users are interested and eager to use these types of app, however, it is still vehemently clear that the factors that encourage users' intention to use and adopt these apps need to be researched. Accordingly, the Kingdom of Saudi Arabia (KSA), one of the many countries that have introduced a CTA (known as Tawakkalna), is using the app to manage the spread of COVID-19. Therefore, this study primary focus is to investigate the factors influencing users' willingness to utilize an app, which has the ability to provide users with information concerning potential association with individual's who may have contracted the virus. To achieve its aim, the study is guided by the following research question:
• RQ: To what extent does existing technology adoption factors influences the behavioral intention of Tawakkalna?
By applying the task-technology fit (TTF) model, the technology acceptance model (TAM), and privacy calculus theory (PCT), this study examines the impact of factors, such as perceived privacy risk, perceived social risk, and social interaction, as well as perceived ease of use (PEoU), perceived usefulness (PU), and TTF on behavioral intention of Tawakkalna. Also, this study investigate the predictive relevance of the key indicators via machine learning (ML) technique that has received little attention in the current literature (22, 23), particularly the application of artificial neural network (ANN). Moreover, this study is among the first to test the predictors of behavioral intention of CTA using an integrated model and a dual-stage SEM–ANN approach. Therefore, the following section summarizes the rationale and existing literature on the TTF model, the TAM, and PCT and presents a conceptual model of Tawakkalna acceptance, along with interrelated hypotheses. The research approach is then discussed. Next, the results section contains information on the hypotheses testing conducted. Finally, additional discussion and implications are offered to substantiate the conclusions, limitations, and recommendations for further research.
2. Literature Review
2.1. Background and Motivation
The speed at which COVID-19 cases are increasing and fear of overburdening health services has made numerous countries implemented measures such as lockdowns to restrict the virus spread (8). As a result, new technology-based strategies for identifying contacts have been suggested, mainly when case detection is aggressive (6, 7). The digital contact-tracing mobile apps have been developed by government and health authorities as a response to track association of individual and automatically provide instructions concerning self-isolation measures to potentially infected people. Also, Apple and Google have introduced a third-party apps on iOS and Android devices to facilitate the development of CTAs by the public health agencies worldwide (8, 9, 20). Although, Apple and Google assert that user privacy and security are central to the design, privacy concerns have been raised (20, 24).
The privacy concerns have caused difficulties with trust around users' consent and participation in downloading and using such apps. This is particularly true in liberal countries that support socially progressive opinions. Moreover, in those countries, the usage of such apps is optional, effectively negating their purpose. According to (25) as cited in (8), the effectiveness of contact-tracing apps depends on the number of people in the population who use them. Approximately 50–70% of the population within a region or a country is highly recommended. The scientific and epidemiological evidence suggests that CTAs can lower pandemic-related suffering and ease lockdowns (26). Interestingly, online surveys outcomes, conducted in advanced countries (France, United Kingdom, Italy, Germany, and the United States) has demonstrated strong support for the CTAs (11). However, as emphasized by (8), this does not imply that people will use the app.
Numerous studies indicate that CTAs can considerably contribute in limiting and halting the spread of COVID-19 by accelerating reporting and contact-tracing practices through enhanced proximity tracing, digital data flow, and geolocation monitoring (8, 27). They could play a critical role, given the widespread usage of internet-connected devices, increasing the speed with which many smartphone users can be monitored in real time to determine infection hotspots. Contact-tracing apps are critical to COVID-19 management measures in several countries (28). Additionally, they can be vital in flagging other illnesses, mainly when physical contact tracing is impossible. Indeed, work by Kucharski et al. (29) emphasized that combining testing and contact tracing significantly reduced the transmission of the coronavirus more than either self-isolation or mass testing.
Notably, the literature has expressed consensus regarding the difficulty of implementing contact tracing without infringing an individual's privacy (8, 9, 24). The threat to personal privacy was significant enough for Google and Apple to work on an exposure notification API, an application programming interface (API). The API enables public health organizations to deploy contact-tracing solutions designed to protect users' privacy and security. The efficiency of the apps in tracking and tracing individuals infected with COVID-19 has however been questioned in advanced countries by industry experts and academics. The apps have been limited by privacy, security, and technical issues, and their influence on the COVID-19 pandemic remains unknown (30, 31). Moreover, many countries worldwide have not made any effort to implement CTAs, and their COVID-19 management has been among the best (e.g., Mauritius, Tanzania, and Iceland) (32). Figure 1 presents countries across the world, showing those with no tracing, limited tracing, and comprehensive tracking.
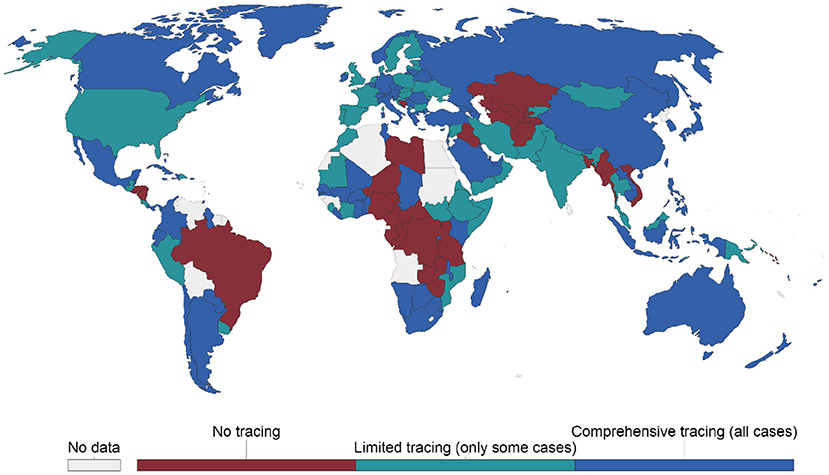
Figure 1. Countries with COVID-19 contact-tracing initiatives as of July 6, 2021. Source: Hale et al. (33).
2.1.1. Adoption of CTA
The development of contact tracing apps and adoption is a never-ending problem for public health systems and policymakers (34). This study offers an evidence-based, situation-specific concern to understand better the theoretical and practical significance of CTA adoption in KSA. The literature review shows that most of the contact tracing apps adoption where conducted in liberal countries (10, 11, 34–41). According to the existing studies, the unified theory of acceptance and use of technology (UTAUT), privacy calculus theory (PCT), and extended technology adoption model (eTAM) dominated the studies of CTA adoption. Similarly, privacy concerns are the major issues studied in CTA adoption, and most of the literature shows that privacy is not a significant predictor of CTA. However, privacy concerns are not investigated in other regions, such as the Middle East and Africa. This calls for more studies to investigate this phenomenon in another geographical context.
Moreover, the current CTA adoption literature have integrated multiple theories in their studies. For example, work by Sharma et al. (36) integrated fairness theory (FT), dual calculus theory (DCT), protection motivation theory (PMT), theory of planned behavior (TPB), and Hofstede's cultural dimension theory (HCDT) to investigate the adoption intention of CTA. The literature review has identified a few studies that employed a two-stage analysis to investigate CTA adoption. Specifically, Duan and Deng (37) employed CB-SEM with an artificial neural network (ANN) approach to investigate the CTA adoption through the lens of the unified theory of acceptance and use of technology and privacy calculus theory in Australia. In contrast, the study by Nguyen et al. (39) investigated the extended technology adoption model through PLS-SEM and fuzzy set/qualitative comparative analysis (fsQCA). The summary of the review findings is presented in Table 1. This review provides a validated list of elements influencing people's CTA adoption concerns and attitudes in various countries. However, similar studies in the context of KSA are lacking to allow policymakers to take systematic steps to address these issues and enhance CTA acceptance.
2.1.2. Tawakkalna
The recent support by information technology has enable several countries implemented CTAs to restrict the spread of the COVID-19 virus. The Kingdom of Saudi Arabia (KSA) is among the countries that have harnessed technology innovation to manage the spread of the coronavirus. Thus, in the KSA's efforts and as part of its commitment to protect the health and safety of its citizens and residents from the risk of COVID-19 transmission, the government introduced an app called “Tawakkalna” to trace the health status of individuals and to permit them to enter public places. The app was developed by the Saudi Data and Artificial Intelligence Authority (SDAIA) to support government efforts to fight the coronavirus spread; this is along with other initiatives such as Tetamman, Tabaud, Sehha, and Mawid (42). In the nine months since Tawakkalna's inception, the app has accumulated over 17 million users (43). This result demonstrates the app's high reliability as one of the most successful and effective digital solutions.
As a result, the Tawakkalna app was built to support the digital issuance of movement approvals for private sector and government employees as well as individuals with emergency concerns. This is in collaboration with the Ministry of Health and other relevant authorities to limit the spread of the pandemic during the movement control order period. Individuals, as well as health, private, and security entities that are not primarily concerned with movement control, can use the app to automate all interactions between relevant parties, significantly reducing the economic, health, and social consequences of policies adopted to eradicate COVID-19 (6). Similarly, Tawakkalna assists managers of the crisis to monitor CTA users' health status. Moreover, the app provides other services, such as allowing individuals to report policy violations or any suspected cases through social responsibility and encouraging users to take precautions before leaving their homes (11). According to a survey conducted by (44), Tawakkalna has higher ratings for users' expectations in its key performance indicators (KPIs) than its counterparts, the Tetamman and Tabaud.
Furthermore, several studies in the literature have been conducted on the KSA's COVID-19 technology-supported management (42, 44, 45). Although, peak number of these studies focused on investigating the role of mobile applications in health or investigating the adoption factors without a link to adoption theory in the information systems literature. Hence, most of the existing work has not addressed the adoption factors based on the theoretical lens of information systems literature concerning technology adoption model, as well as the holistic picture of privacy risk concerns. Hence the focus of this study is to investigate the acceptance of the Tawakkalna app via the TAM, and the TTF and PCT theoretical models.
2.2. Theoretical Foundation
Technology adoption theoretical models are used to examine adoption behavior in the IS literature. The following sections discuss the models covered in this study. To be specific, the explanation of each model is then provided along with the reason why it is suitable for this study.
2.2.1. Technology Acceptance Model (TAM)
The technology acceptance model (TAM), created by (46) and (47), comprises two fundamental factors, perceived ease of use (PEoU) and perceived usefulness (PU), and a third factor called attitude toward use (ATU). PU is defined as a person's belief that utilizing a system will improve his/her performance and PEoU as explaining a person's belief that using a system is effortless. Additionally, Davis et al. (48) emphasized that the actual usage of the system is influenced by the user's behavioral intention which is driven, in part, by the user's attitude toward the system's use and perceived usage (49). The TAM is employed in this study as it has been primarily acknowledged by academic researchers in the technology adoption literature (23, 50–52). Taherdoost (52) and Alwabel and Zeng (23) added that the TAM is arguably the most frequently quoted model in the field of technology acceptance. It has received strong empirical backing during past decades. Moreover, research by Turner et al. (53) suggested that, by using the TAM at the time of the release of a technology, the model should be able to forecast future usage of that technology. Similarly, the suitability of the TAM to predict new technology usage has been widely emphasized (51, 52). Research has suggested the use of acceptance theories, such as the TAM, in future CTA studies (8). Apart from being a widely compared model, the TAM, with some updates, has been utilized to assess the intention to use apps in various industries and contexts (54–56), including health app (57).
Benbasat and Barki (58) argued that many adoption contexts are required to understand diverse behavioral factors. Understanding specific behaviors would provide more significant recommendations for the design and practice of technology than just arguing for higher usefulness. CTA are still in their infancy, and just a few researchers have studied whether PU and PEOU perceptions continue to be sufficient to account for users' behavioral intents to use CTA. Although, CTA are sophisticated technologies that operate under various laws, settings, and features that vary based on social norms of a particular country (8, 36). No study has investigated the PU or PEOU from the Tawakkalna point of view. Therefore, this study employs the two independent variables of the TAM. Additionally, this work aims to close the gap in the literature on TAM and other technology adoption models by applying machine learning (ML) techniques to aid in developing a predictive technology acceptance model that has received little attention in the existing literature (23). To our knowledge, no study has used ML techniques such as artificial neural networks to predict PU and PEOU in CTA adoption. As a result, both PU and PEOU are required and appropriate for examination in this study.
2.2.2. Privacy Calculus Theory (PCT)
According to privacy calculus theory (PCT), consumers make privacy-related decisions by weighing the benefits of any information disclosed against the risks of its exposure. Thus, this study focuses on the specific risks that influence users' acceptance of CTAs. The PCT has been employed to better understand consumers' assessment of the objectivity of disclosing private information (59–61). Furthermore, the PCT claims that consumers make privacy decisions by weighing the potential benefits against the potential risks posed by disclosing their personal information (62). Thus, the concept of privacy has a significant impact on how information is disclosed (63, 64), in the online context, privacy refers to the individual's awareness and control over the gathering and use of his/her personal data (65).
Prior research has established that a user's decision to download a new app is not always reasonable when the risks and rewards of information trading are evaluated. Instead, external pressures such as time restrictions, quick gratification, or optimistic bias impact on the decision, leading to acceptance of the advantages while ignoring the risks (66, 67). As consumers gain experience with mobile apps, they tend to focus on the benefits and downplay the potential hazards. Users may be unaware of the personal health data trade-off with a CTA when downloading the app. This research employs PCT to focus on the rational evaluation of risk-benefit calculations that users may undertake to determine whether to accept or reject this type of interaction. Using findings from earlier studies that employed PCT to explore electronic commerce (e-commerce) and mobile commerce (62, 68–70), and CTA adoption (36, 71), this research examines the critical risk and reward components of contact tracing, a hitherto understudied area. The hypothesis is that both perceived risks and advantages influence users' acceptance of Tawakkalna.
2.2.3. Task-Technology Fit (TTF) Model
Over the past few decades, a significant amount of research has been conducted to understand behavioral intentions, including general use intention, actual usage, and continuous use of information systems (IS) via technology adoption models (46, 72, 73). Among several theoretical frameworks, the TTF model focuses on how newly developed technological solutions (IS products) fit a user's current tasks, hence boosting the user's performance (74–76). The assessment of how well the technologies incorporated in an IS-based product meet users' current tasks is the primary focus of the TTF model. The TTF model has been extensively applied in information system research and recently, it is frequently coupled with other models, such as the information system success model (77), social cognitive theory (SCT) (78), and the unified theory of technology acceptance and use (UTAUT) (79, 80). As with the TTF model, the use context established by an IT product is critical for its acceptability and use (81, 82).
The CTA was developed and introduced as a result of the integration of information technologies into health services. The amount of research conducted under the contact-tracing umbrella in the study of IT usage intentions has been substantial (10, 44, 45). The concept of mobility has been viewed as an explicit technological component within the TTF framework (79, 81, 83, 84). By utilizing a mobile app for a CTA, users' perceptions of the TTF model can be enhanced by the ability to perform certain activities anywhere and at any time (79, 83, 85), which, in turn, changes their behavioral intention toward the task. Mobile health apps used in emergencies can be classified as “general-purpose apps” or “built-for-disaster-purpose apps” (86, 87), with contact tracing falling under the latter category. Most CTAs have capabilities that allow interaction patterns between citizens and crisis management authorities during emergencies (88). The use of technology-mediated mobile apps is already ingrained in our societal structure. Therefore, in addition to understanding the mobile CTA, evaluating its technical characteristics, particularly its mobility, need to be evaluated. In light of the success reported in the literature of the TTF model on behavioral intentions toward mobile apps, this study applies the model via integration with TAM and PCT to better understand usage intentions toward the KSA's CTAs.
3. Model and Hypotheses Development
Research on theory development has highlighted the need for integrating theories and models (34, 36, 37, 80, 89, 90). Based on the TAM, PCT, and the TTF model, this study develops and applies an integrated model to explain behavioral use intention toward the Tawakkalna COVID-19 tracing app, as shown in Figure 2. Behavioral intention (BI) has been described as the extent to which an individual has made deliberate decisions about whether to perform or not to perform a particular behavior or the probability of an individual's intention to engage in a specific behavior (91, 92). This study defines BI as the extent to which an individual will adopt or continue to use Tawakkalna in the future (71). Accordingly, the TTF model is influenced by both mobility and task characteristics. The TAM PEoU also has effects on perceived usefulness (PU). The BI is determined mainly by the TTF, PU, PEoU, perceived privacy risk, perceived social risk, and social interaction. The perceived social risk has both positive and negative effects on behavioral intention. The following section discusses the research model and its hypotheses in detail.
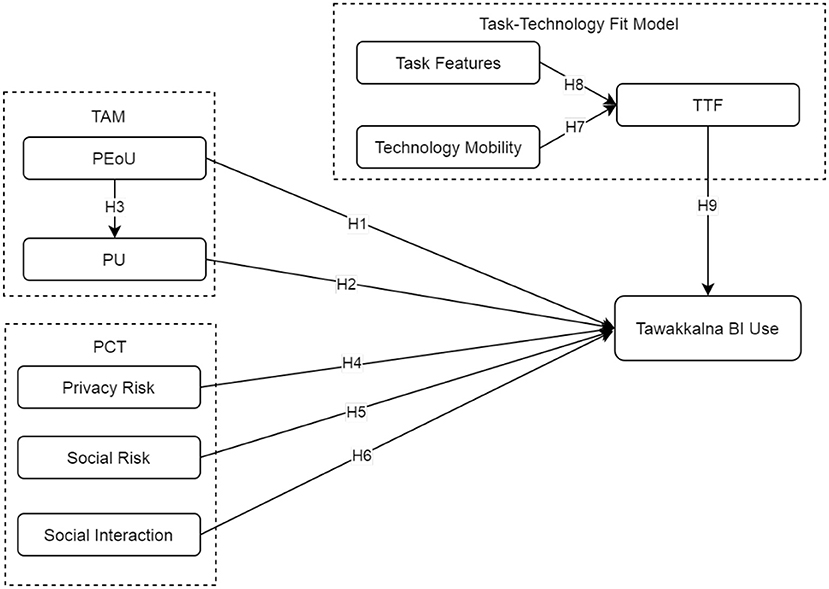
Figure 2. Research model for adoption of Tawakkalna contact-tracing app (CTA). BI, behavioral intention; PCT, privacy calculus theory; PEoU, perceived ease of use; PR, privacy risk; PU, perceived usefulness; SI, social interaction; SR, social risk; TAM, technology acceptance model; TF, task features; TM, technology mobility; TTF, task-technology fit.
3.1. TAM Constructs
Earlier studies that used the TAM to examine public health IS apps discovered that PEoU and PU were the most prevalent and significant technology acceptance factors (93–95). Perceived usefulness is defined as the degree to which an individual believes that utilizing a system will reasonably improve his/her performance (46). Perceived ease of use is described as a person's belief that utilizing technology will involve minimal exertion (96). Similarly, according to the TAM, an individual's attitude toward a technological system is defined by these two variables: PU and PEoU. Moreover, Velicia-Martin et al. (10) recently investigated COVID-19 tracing app acceptance via the TAM's theoretical lens. The results of that study found that PEoU and PU were significant predictors of intention to use the CTAs. Hence, this study anticipates that the KSA population will embrace and use Tawakkalna due to the benefits associated with its use. As a result, the following hypotheses are formulated:
Hypothesis 1 (H1): Perceived ease of use has a positive and significant effect on behavioral intention of Tawakkalna.
Hypothesis 2 (H2): Perceived usefulness has a positive and significant effect on behavioral intention of Tawakkalna.
Hypothesis 3 (H3): Perceived ease of use positively and significantly affects perceived usefulness.
3.2. PCT Constructs
Internet-related perceived privacy risk refers to the extent to which internet users are concerned about how and to what extent an online entity collects and uses their personal information (97). This indicates a perceived disconnection between users' expectations and the reality of how their personal information will be managed (13). Numerous difficulties are arising in the context of COVID-19 crisis management that may impede users' acceptance of contact tracing. These difficulties cover the six main dimensions that shape internet privacy concerns: data collection, improper access, secondary usage, error, awareness, and control (98). The degree to which an individual is concerned about the amount of personal data that the internet app has gathered is characterized as part of data collection. Concerns about secondary data usage occur when an individual is worried that personal information might be used for another reason or shared with third parties without his/her consent. Improper access is when an individual is concerned about personal information being stolen or made available to unauthorized parties. Error focus refers to the accuracy of personal information and the techniques used to correct and maintain error-free personal data. Awareness refers to an individual's understanding of privacy terms and conditions. Control reflects the lack of adequate measures to control data collection and management.
Privacy concerns are critical in the COVID-19 tracing app context, as the app requires the acquisition of not only personal information but also location information, which many individuals regard as very sensitive due to the increased potential of information misuse (99–101). The sharing of personal information, such as health status and location details, is of particular concern to users of certain mobile apps (19). Additionally, several studies in the literature have already raised concerns about the impact of privacy issues in relation to CTAs (8, 10, 36, 71). As a result, it is reasonable to predict that Tawakkalna will be seen negatively by persons with a high level of perceived privacy concerns who are likely to view location tracking and information storage as a danger to their freedom and privacy. Thus, the following hypothesis is proposed:
Hypothesis 4 (H4): Perceived privacy risk has a negative and significant effect on behavioral intention of Tawakkalna.
Moreover, perceived risk has been characterized as the uncertainty, uneasiness, and worry felt by users when they cannot anticipate the repercussions of providing personal information online (102). This level of disclosure is enhanced in the mobile environment, as it enables the detection of a user's location, the time, and the presence of other connected users in the vicinity (62). Although perceived risk has been conceptualized as a multidimensional term encompassing financial, performance, physical, physiological, and social risk (13, 60). This study focuses on social risk as a particularly prominent component in the contact-tracing application.
In PCT, the readiness to disclose information is connected to a negative perception of risk and the perception of a favorable benefit. For example, by revealing location-based information or health status to authorities, individuals may benefit from physical movement (4, 16), which may increase the number of users inclined to use the contact-tracing app. On the other hand, location-based information is viewed as highly sensitive (16) by users who perceive this information as intrusive, who do not want their social activities to be threatened by authorities due to COVID-19 risk exposure, or who are fearful of being forced to self-isolate. Hence, the pandemic has significantly harmed individuals' capacity to have deep interpersonal relationships with other people and has severely impaired the extreme human need for contact, discouraging or containing any physical manifestation of attachment and connection (4, 103). The reason is that authorities are viewing reduced social interaction as the way to save millions of lives during the COVID-19 pandemic (104, 105). Moreover, the pandemic has displaced many people from their jobs and, crucially, from their social networks, forcing them to collaborate and maintain affiliations remotely and distantly (106). Thus, the following hypotheses are stated concerning social risk and social interaction benefits that could be derived from using a CTA.
Hypothesis 5 (H5): Perceived social risk has a negative and significant effect on behavioral intention of Tawakkalna.
Hypothesis 6 (H6): Perceived social interaction has a positive and significant effect on behavioral intention of Tawakkalna.
3.3. TTF Constructs
According to previous literature, the TTF framework has been modified to meet the nature of emergency response settings and, thus, it is integrated into the research model in its entirety. As previously stated, mobility is viewed as a distinguishing technological element of mobile contact tracing in this study. To be more precise, mobility is defined as the degree to which users perceive their ability to access and use mobile contact tracing at any time and from any location (79, 81, 82). This technological aspect is particularly beneficial for crisis and emergency responses, as an individual suffering a time-sensitive occurrence may immediately access the application (87, 107). As a result, the following hypothesis is formulated:
Hypothesis 7 (H7): Technology mobility is positively related to task-technology fit.
Moreover, in the TTF framework, task features/characteristics are typically interpreted as helpful behaviors that satisfy users' needs in using an information system (IS) (79, 82, 108). Typically, the Tawakkalna tracing app has a bundle of specific task characteristics that are reshaped to fit the study setting (84). Individuals can utilize the app to seek/share health information, engage with authorities, access COVID-19 vaccine, and report concerns (43, 88). Users can also execute various emergency response tasks within a single platform via their mobile at any time and from any location (87). As a result of these task features or characteristics, the following hypothesis is formulated:
Hypothesis 8 (H8): Task features or characteristics are positively related to task-technology fit.
Validation of H7 and H8 contributes to knowledge of the fit between mobility and emergency response tasks and, thus, are crucial to the research questions. According to (79), a strong match between task and technology promotes users' behavioral intentions, whereas a weak match has a negative effect on their behavioral intentions. Numerous studies in the literature on other mobile apps have demonstrated how consumers' perceived task-technology fit affects their adoption of a specific app (77, 79, 84, 85). For example, evidence on users' behavioral intentions regarding mobile learning demonstrates the effective implementation of the TTF model (108, 109). Similarly, an individual's perceived task-technology fit concerning emergency responses via mobile social media is a significant predictor of his/her behavioral intention toward mobile social media during emergencies (82). Thus, this study proposes that the TTF could impact on the success of behavioral intention toward Tawakkalna as the app is mobile-based and has learning, health, and social support. Hence, the following hypothesis is formulated:
Hypothesis 9 (H9): Task-technology fit positively and significantly affects behavioral intention of Tawakkalna.
4. Research Methodology
4.1. Data Collection Process
The targeted sample comprised residents of the KSA, including citizens and non-citizens, who were using the Tawakkalna app and were above 18 years old. Purposive sampling, a non-probability sampling strategy, was used in this study. It is defined as an approach in which targeted items meet particular requirements (110). Purposive sampling (judgement sampling) is the purposeful selection of a participant based on the participant's characteristics (111). It is a non-random strategy that does not require any underlying principle or a predetermined quantity of participants. The researcher uses this technique to survey a population that meets certain criteria for being viable for the study (110, 111). The researcher determines what information is required and then seeks out persons who can and will supply it based on their knowledge or experience (111). Purposive sampling improves the study's rigor and the reliability of the data and outcomes by better matching the sample to the research's goals and objectives (112). Moreover, the purposive sampling is an effective strategy for researching the early phases, when target participants have little or no expertise with the technology under inquiry (111, 112). Contact-tracking apps, notably Tawakkanla, are still in their infancy and consequently have a small user base (8). Additionally, this technique has been employed to study the adoption of existing IT (113). Thus, it is appropriate for this research.
In data gathering, purposive sampling can be used with several techniques (110). To recruit respondents, this study used the snowball sampling technique which follows purposive sampling (110, 112). Snowball sampling is the process of identifying participants “through referrals made among people who share or know of others who possess some characteristics that are of research interest” (114). This technique is suitable for this study due to the difficulty of obtaining a list of targeted users of Tawakkalna to ask them to describe their perceptions and experiences (110). A pre-test phase was conducted to validate and refine the questionnaire (115). Five information systems professors were asked to assess the survey questionnaire in order to confirm the questions and items' face validity. Minor revisions were made in response to their feedback. The survey was prepared on google form and the link was then sent by email and posted on two popular and highly used social network tools in the KSA, namely, WhatsApp and Twitter. The initial respondents were invited to forward the link to family members, friends, and coworkers, in order to encourage them to complete the questionnaire and share the URL with others (112). Two survey links were created to reveal the numbers recruited from the selected settings. In total, 1,080 clicks were accessed via the questionnaire link; 78% of responses were recruited from Twitter and WhatsApp, while 22% received an email invitation. Furthermore, responses that were incomplete (549), responses that were completed in less than the average time for survey completion (7 min) (193), and responses that indicated the respondent was under the age of 18 (30) were removed from the study during the data cleaning. Hence, the final sample consists of 309 records which were completed and ready for further evaluation. The data collection procedure was conducted in 2 weeks between March 1 and 15, 2021.
A sufficient sample size should be used to estimate a model's parameters (116). When using confirmatory factor analysis (CFA), (117) recommended that the sample size be greater than 300 in order to objectively and adequately accomplish the study. However, the work by Hair et al. (116) disputed this figure, stating that, for a minimum R2 (coefficient of determination) value of 0.25 with 5% error probability and 80% statistical power, the minimum sample size should be 45 for at least a maximum number of five arrows pointing to the dependent variable. Our sample is larger than those in similar SEM–ANN studies (118). Therefore, based on the criteria listed above and the statistical analysis method used in this study, the total sample size obtained (N = 309) is deemed adequate and sufficient for estimating the parameters of the model. As this study adopted partial least squares (PLS) and conducted CFA, the normality issue did not need to be considered for normally distributed variables (119, 120).
4.2. Item Development
The item creation phase is important to confirm the content validity of measurement items (120). The item measures utilized in this study were designed and tested previously in well-established research, with minimal alterations to match the purpose of this study (121). Hinkin (122) hinted that there is no strict rule governing the number of items that should be included in each construct. Although, it is critical to guarantee that each construct's domain is adequately sampled (123). Also, work by Gefen et al. (120) insisted that three indicators that are completely dependent on a single common factor can statistically detect the construct factor measurement model. Accordingly, most constructs in the current study were measured by at least three items (see Table A1).
Behavior intention (BI) was reflected by three items adopted from (71, 73). Perceived ease of use and usefulness scales were adopted from (47) and (10). Three items were created for task features (TF) (79, 82), while mobility (TM) (79, 81, 82) was measured using four items. Three items were employed for each of TTF (79, 82) and social interaction (SI) (103). Also, this study adapted four reflective indicators to measure each of privacy risk (PR) (13, 98) and social risk (SR) (13). A 7-point Likert scale was used as a multiple-item scale so respondents could rate their degree of agreement to record their responses. The scale ranged from “strongly agree” to “strongly disagree,” with “7” being strong agreement and “1” being strong disagreement (122). The questionnaire was written in English and translated into the Arabic language as responses were sought from all layers of Saudi citizens, providing them with the opportunity to participate.
4.3. Common Method Bias and Non-response Bias
The study findings may be vulnerable to common method bias (CMB), as survey data were self-reported, and behavior was not quantified as it was based on users' self-assessment (124). As a result, some methods were implemented to evaluate and mitigate the potential for CMB, as recommended by (125). To be specific (126), priori procedural remedies were incorporated. This method was utilized during the pre-test phase to refine the scale items and eliminate potential ambiguities, with multiple-choice questions periodically included to break up the pattern of questions rated using Likert scales. Moreover, to check for CMB, Harman's single-factor test was run. The result shows that the total variance was <50%, which is 40.72%, indicating that CMB was not an issue in this study (Table 2). Also, the path coefficients obtained from the structural model assessment had varying degrees of relevance (125).
This study applied the non-response bias test (121). Thus, two subsamples were created based on the order in which respondents responded to the survey questionnaire. The first 75 early responders were divided into two groups, while the second 75 late respondents were divided into two groups. The two groups were compared using a two-tailed t-test with a 5% threshold of significance (127). There were no significant differences in the test outcomes between these two groups of responders. As a result, this study was not concerned with non-response bias.
4.4. Data Analysis Methods
4.4.1. Structural Equation Modeling (SEM)
The study used a PLS-SEM technique to analyze the data. Two primary reasons prompted the researchers to select this technique: firstly, the PLS technique does not make a strict assumption about the normal distribution of data (128). Secondly, the PLS technique is preferable for composite analysis (129). Another reason for using the PLS-SEM technique was a dearth of established hypotheses for predicting behavioral intention toward the COVID-19 CTA, as well as the model's relative complexity, as it contained nine constructs. As such, the study evaluated the measurement model first by determining the constructs' reliability and validity, and then estimated the path coefficients and other structural model parameters through CFA based PLS-SEM approach, in accordance with available recommendations (129, 130). Therefore, SmartPLS 3 software was specifically employed throughout the SEM process.
4.4.2. Artificial Neural Network (ANN)
The study re-examined the research model using an artificial neural network (ANN). The dual analysis was undertaken due to the benefits from both PLS-SEM and ANN strengths (22, 23, 131, 132). Thus, the ANN was used to determine the predictors of Tawakkalna use intention (133). The PLS-SEM technique is regularly used to examine and test causal relationships (116, 134). Moreover, Chan and Chong (134) and Teo et al. (135) reported that the ANN is utilized to identify complex linear and non-linear relationships. Although recent studies suggested that the ANN is not enough to handle a complex nonlinear relationship (136, 137). Nevertheless, the ANN approach give better results than conventional prediction methods (137) and is more precise than the usual regression strategy in terms of prediction (82, 138). As a result, the ANN approach has been utilized to examine the link between dependent and independent variables in IS research (22, 82, 132, 139, 140). Hence, this study employed ANN analysis to determine the factors that significantly influence Tawakkalna use intention.
5. Results
5.1. Descriptive Statistics
Table 3 shows the demographic profile of respondents. The sample mostly comprised male respondents (66.9%). Ages ranged from 18 to 41 (79.9%) and 21% were older than 41 years. The sample level of education shows that 90.4% have a Bachelor's degree or higher. With respect to mobile app usage, 78.3% of the sample used the apps between 1 and 7 h daily. This data is consistent with a previous survey report indicating that the average daily mobile usage time is between 5 and 6 h (141). Furthermore, according to the mean values, the number of responses falls between 4 and 7, which spans from 4.39 to 5.39. In addition, the standard deviation range is narrow, indicating that the values are near the mean. In addition, the data shows minor variations and deviations. Rumsey (142) defined the data concentration toward the mean as the respondents' agreement on the impact of factors on behavioral intention. Table 2 shows the results of the mean and standard deviation of the constructs.
Moreover, to better confirm the normality distribution, the skewness and kurtosis tests were used to evaluate multivariate normality in the data (143). The skewness test verifies that the variable distribution is symmetrical by determining the most likely scenario (116). The ranges from −1.96 to +1.96 are used to determine if the data is normally distributed. However, if the sample size is more than 300, the thresholds for skewness and kurtosis are −2 to +2 and −7 to +7, respectively (144). The skewness and kurtosis results for this study are reported in Table 4. Hence, the data met the appropriate normality assumption based on the regression normality coefficient. As a result, the motivation to use path analysis using PLS is valid (116).
5.2. Reliability and Validity
The reliability and validity of the study's constructs were determined by confirmatory factor analysis. To test the internal consistency reliability, the values for standard loadings, Cronbach's alpha, Rho_A, composite reliability (CR), and average variance extracted (AVE) were obtained for each construct, as summarized in Table 5. Most item loadings had values >0.7 and were statistically significant at p < 0.001 which is considered acceptable (119). In addition, each construct had an AVE value greater than the critical level of 0.5, indicating good convergent validity. Similarly, all constructs had values for CR, Rho_A, and Cronbach's alpha of more than 0.7, indicating good consistency dependability (115, 119, 129).
Furthermore, the discriminant validity of the variables was determined by comparing the square roots of AVE values and the inter-construct correlations for each construct, as recommended by (129), as presented in Table 6. Accordingly, the diagonal elements were significantly larger than the off-diagonal elements, indicating acceptable discriminant validity. Additionally, a heterotrait-monotrait (HTMT) criterion test was used to assess discriminant validity (129). The HTMT ratios were less than 1, correlating with the Fornell-Larcker criterion test results.
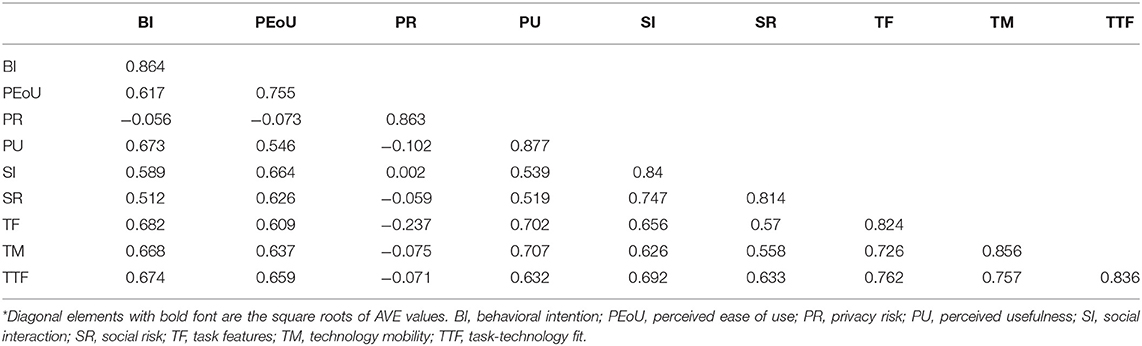
Table 6. Factor correlation coefficients and square roots of average variance extracted (AVE) values*.
5.3. Hypotheses Testing
Various studies have recommended the bias-corrected and accelerated (BCa) bootstrap as the optimal method for discovering relationship effects (145–147). The study therefore ran a minimum of 5,000 bootstrap samples to provide a powerful method for assessing the model's hypothesized relationships (146). Figure 3 and Table 7 summarize and present the findings of the hypotheses' assessments. Except for the influence of variables derived from PCT's behavioral intention, all proposed relationships from the TAM and the TTF model were statistically supported, with 58.8% of the variance in the dependent variables explained by the model. Each of the TAM's variables was highly influential on BI, with the values of all relevant path coefficients exceeding 0.20, and PEoU explaining 29.8% of the variance of PU. As a result, hypotheses H1, H2, and H3 were supported. Regarding risk-related variables, the model produced some intriguing yet sensible conclusions. Both privacy and social risks were not endorsed; however, perceived social interaction had little effect (B = 0.119), but the hypothesis was rejected due to the p-value requirement. The result may show that rational decision-makers make sensible use of Tawakkalna to guide their movements to avoid COVID-19 high-risk areas. Among all variables, PU had the greatest influence on BI (B = 0.546, t − value = 16.713, p = 0.000). Additionally, the results associated with risks and benefits, specifically, social interaction associated with social risk, had little effect on BI (B = 0.119, t − value = 1.333, p = 0.182). As a result, H4, H5, and H6 were not approved. Also, when the indirect effects for the PEoU-PU-BI relationship were observed, the indirect effect of PU on use intention was B = 0.110, t − value = 2.730, p = 0.006, which was also significant.
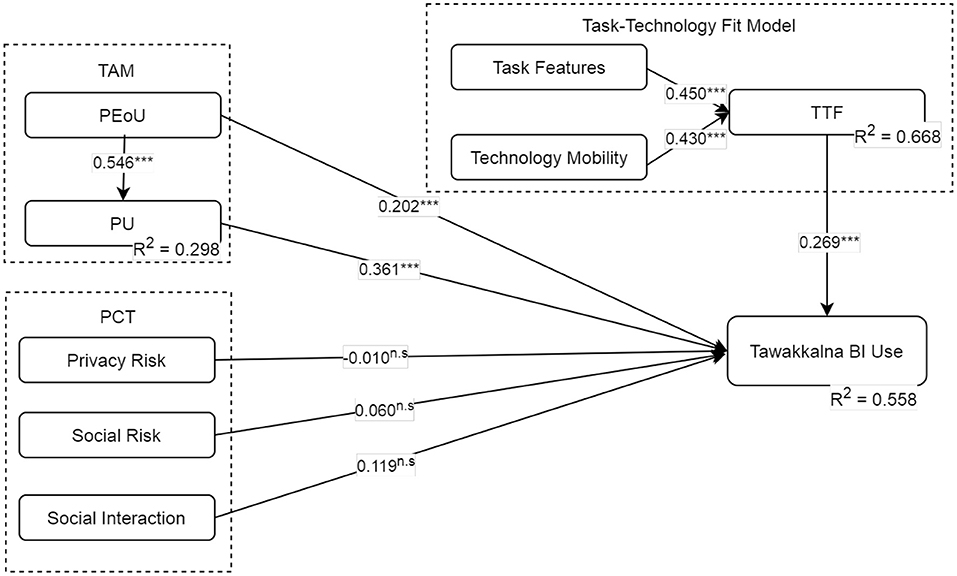
Figure 3. Test results of the structural model. n.s., non-significant; *p < 0.05; **p < 0.01; ***p < 0.001.
Moreover, the assessments of the task-technology fit hypothesis were all supported. Perceived technology mobility (TM) and task features (TF) both influenced perceived TTF at B = 0.430, t − value = 8.372, and p = 0.000; and B = 0.450, t − value = 9.040, and p = 0.000, respectively. As a result, the variables explained 68.8% of the variance in TTF. Therefore, H7 and H8 were also supported. Hence, task features (TF) had a slightly greater positive effect than perceived technology mobility (TM). Also, TTF had a favorable effect on behavioral intention of Tawakkalna (B = 0.269, t − value = 3.083, p = 0.001), supporting H8. Mobility and task characteristics had indirect impacts behavioral intention at B = 0.116, t − value = 3.083, and p = 0.002; and B = 0.121, t − value = 3.219, and p = 0.001, indicating support for indirect relationships.
5.4. Predictive Relevance and Effect Size
Additionally, the suggested model's predictive significance was tested using cross-validated redundancy (Q2), following the existing literature (129, 130, 132). All endogenous factors in Table 8 showed Q2 values >0, indicating that the model was predictive of PU, TTF, and BI. Additionally, (148) effect size (f2) was used to estimate each exogenous variable, as shown in Table 5. According to the magnitude of f2, 0.02, 0.15, and 0.35 correspond to small, medium, and large effects, respectively (130, 132). As indicated in Table 5, PeoU, PU, and TTF had a moderate to significant effect on BI, while the associated risk and benefits variables, namely, PR, SR, and SI, had a negligible impact on behavioral intention (BI). Also, TM and TF had a medium effect on TTF. Similarly, PEoU was a crucial antecedent of PU, having a medium impact. Notably, the associated f2 values that suggested non-significant effects were consistent with the path coefficient findings.
5.5. Artificial Neural Network (ANN) Analysis Results
According to Chong (149), an ANN is a modeling tool capable of imitating human–neural systems and learning. Hence, the learning capabilities of ANNs allow them to be trained to improve their performance (135, 150). In this study, IBM SPSS Statistics (SPSS) v24 software was used to conduct the ANN analysis, following previously published and applied procedures (82, 131, 132, 151). This study identified the relative importance of exogenous elements to an endogenous variable using a multi-layer perceptron (MLP) artificial neural network (ANN) with a feed–forward back-propagation (FFBP) algorithm. Figure 4 depicts the ANN models developed using (152) neural networks drawing guidelines. To avoid overfitting, tenfold cross-validation was performed on the data set (resulting in 10 ANN models), with 70% of the data used for training and 30% for establishing the trained network's projected accuracy (also known as testing). Additionally, the algorithm generated a specified number of hidden neurons, with the hidden layer activated using the hyperbolic tangent activation function. In contrast, the output layers were activated using the sigmoid activation function. The root-mean-square error (RMSE) was determined for each network in the ANN model to determine the model's predictive accuracy, as indicated by numerous studies (82, 131, 135). As shown in Table 9, the ANN model has a mean RMSE of 0.545 for training data and 0.553 for testing data, showing the model's prediction capacity. Apart from implying a level of predictive accuracy, a lower RMSE value indicates a more precise fit and forecast of the data. Additionally, the relevance of external variables was assessed by the number of hidden neurons in an ANN model with non-zero synaptic weights.
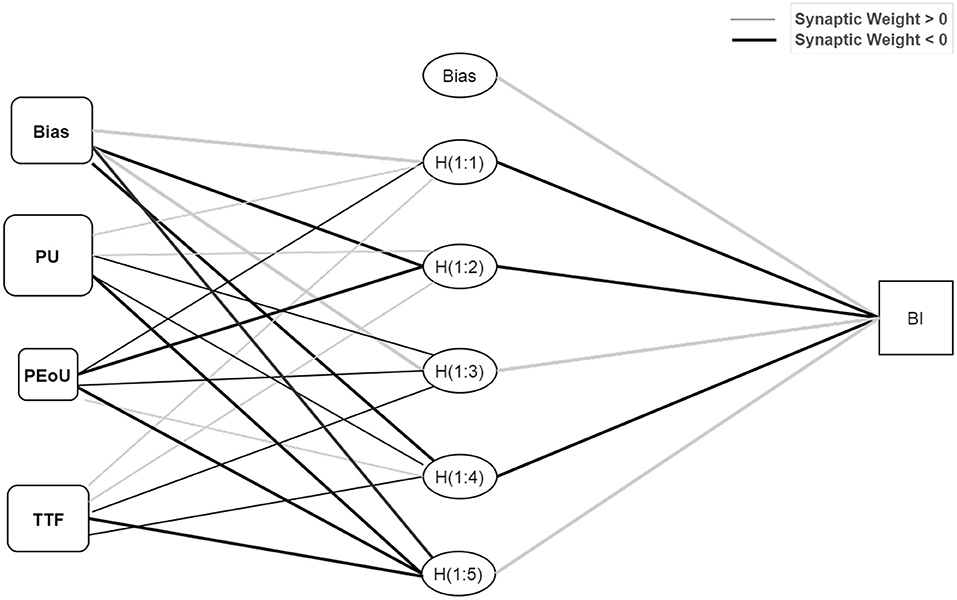
Figure 4. Artificial neural network (ANN) model. Hidden layer activation function: hyperbolic tangent; output layer activation function: sigmoid; input neurons: PU, perceived usefulness; PEoU, perceived ease of use; TTF, task-technology fit.
After establishing the ANN model's anticipated accuracy and predictive relevance, a sensitivity analysis was conducted to statistically evaluate the exogenous variables' predictive capability concerning endogenous components (135, 153). The relative importance of each exogenous variable was determined, and the normalized relative value was computed, as indicated in Table 10. The exogenous variables were then ranked according to their normalized relative relevance and influence strength. Interestingly, when the three variables were examined on the ANN models, perceived usefulness was the strongest predictor of Tawakkalna behavioral intention, with 100% normalized relative importance. Moreover, the result of the relative importance of TTF (66.45%) and perceived ease of use (35.06%) were significant predictors of behavioral intention, in that order. Perceived usefulness was conclusively the strongest predictor of behavioral intention, whereas perceived ease of use was the weakest.
6. Discussion
This study's primary purpose was to determine the key factors influencing users' behavioral intention of a CTA. In this research, an integrated model was developed that combined three perspectives: firstly, behavioral intention is driven by a user's perceived ease of use and usefulness. Secondly, BI is driven by perceived risk and corresponding benefits, classified as privacy risk, social risk, and social interaction. Thirdly, BI is influenced by the fit between technology mobility and task features. Accordingly, the survey data supported six hypotheses in the proposed model and revealed some intriguing findings.
The TAM is considered a valuable model for predicting the behavioral intention of a new app that tracks individuals who have been exposed to a positive COVID-19 case, hence breaking the infection chain (10). Therefore, the hypotheses for the TAM constructs, perceived ease of use and perceived usefulness, were supported by the findings. Interestingly, the variable with the largest significant impact was perceived usefulness. As these hypotheses were derived from the original TAM, the theoretical TAM can be used to examine the adoption of apps to prevent and mitigate the COVID-19 pandemic's effects. These findings corroborate existing studies that employed the extended TAM, testing both perceived ease of use and perceived usefulness as significant factors of use intention (10, 154).
The variables derived from PCT were not found to be significant in the proposed model. Specifically, privacy risk was not significant. Hence, the privacy risk concern was not found to effect the intention to use the tracing app. Thus, H4 was not supported. This suggests that privacy risk concerns about the app, such as the associated risk (from utilizing private information), had no adverse effect on the behavioral intention of the tracing app. This finding was supported by several studies in the literature (10, 155). Our finding also is in the line with previous studies that found insignificant influence between behavior adoption and privacy risks and concerns. These studies include privacy risk on intentions toward IoT health services (156), privacy concerns on Mobile health technologies (157) and CTAs (71).The privacy risk hypothesis was obviously in relation to health concerns. As simply stated by (158), individuals are more concerned about their health, enhancing their readiness to use CTAs developed for this purpose, even at the risk of jeopardizing their personal privacy (19). Li et al. (159) added that the perceptions of the benefits of CTAs are stronger predictors of behavioral intentions than the perceptions regarding security and privacy risk.
Likewise, in relation to the effect on BI, the analysis revealed that social risk and social interaction outcomes were not significant. Thus, findings revealed that the effects of risks, as proposed in H5 and H6, were not significant. The study's findings did not support those in prior research about the social impact of COVID-19 that emphasized the effect of the coronavirus on relationships among the public despite the human need for social contact (4, 106). Nevertheless, the finding could be consistent as the restriction of movement due to pandemic-imposed physical distancing creates a distressing awareness that one's wellbeing depends on others. This is true when comparing the benefits of CTAs to protecting oneself from getting COVID-19 with the combined risk of losing privacy, security, and the social risk (19, 159).
Similarly, privacy and perceived risks were found to have no relationship with behavioral intention. This could be a result of the variable's narrow assessment, which was limited to social and privacy risk perceptions. However, incorporating additional privacy concerns, such as uncertainty avoidance, perceived severity, and vulnerability (36), as well as risk perceptions, such as uncertainty, time, performance, and psychological loss, may provide a more full picture of these risk variables, which may bolster their perceived importance. As a result, researchers are urged to conduct comprehensive studies on risk perceptions associated with the acceptance of CTAs such as Tawakkalna.
Furthermore, in assessing the TTF predictors, the findings indicated that users' perceptions of the mobility of Tawakkalna were ideally aligned with their task characteristics, which influenced their behavioral intention of the tracing app. Mobility and task features accounted for 68.8% of the variance in the TTF in the model which had a more significant effect. The findings supported the earlier IS research regarding the influences of TTF on use intention (77, 82, 84, 160). This study emphasized the critical role of TTF in meeting the users' CTA needs. Also, the findings revealed that perceived usefulness was the most important direct antecedent of BI, followed by TTF. Thus, this research offers significant knowledge about the relative value of TTF as a predictor of a CTA's behavioral intention.
It is worth mentioning that the use intention explained a 58.8% variance in the proposed model. Compared to other variables, TTF had a higher predictive R2 value at 66.8%, with perceived usefulness explaining only 29.8%. Also, the Q2 assessment revealed that the predictive accuracy of TTF was slightly higher than the use intention, at 0.458 and 0.430, respectively. On the other hand, perceived usefulness had the lowest Q2 value (0.151). Thus, the results for the R2 values were aligned with the predictive potential of the model. Moreover, the ANN analysis results were in line with the SEM path coefficient outcomes, which ranked the variables according to their impact on the model. Perceived usefulness was the strongest factor, with TTF the second strongest.
6.1. The Optimality of the Proposed Approach
The information system literature about the technology adoption model primarily predicts behavioral intention, usage intention, or adoption intention as the dependent variable. Most of the studies investigate this variable predictive power through PLS-SEM (10, 34, 39) or CB-SEM (35–37, 41). One of the primary criteria used to examine the model's predictive capacity is the coefficient of determination (R2). Since this study adopted the PLS-SEM approach, the R2 values of existing studies are compared with the current studies. This is to show the novelty and optimality of the proposed approach. Hence, the comparative analysis revealed an exciting result. Firstly, studiess by Velicia-Martin et al. (10) and Hassandoust et al. (34) have higher R2 values than the proposed approach. However, these studies adopted a single theory, while our approach integrated three theories (TAM, PCT, TTF). Secondly, since existing studies based on PLS-SEM have not integrated multiple theories, this study compared the result of the proposed approach with the work by (36), which is based on CB-SEM and incorporated multiple theories (FT, DCT, PMT, TPB, and HCDT). Remarkably, the R2 of the proposed approach is slightly higher than the CB-SEM approach. Thirdly, the proposed approach is the first to apply two-stage SEM–ANN analysis among the existing studies based on the PLS-SEM approach. Moreover, similar studies in the context of middle-east countries, specifically KSA, are lacking. Thus, this study has addressed this gap to allow policymakers to take systematic steps to address these issues and enhance CTA acceptance. Table 11 presents the comparative analysis of the previous studies and the proposed approach.
7. Contributions and Implications
7.1. Theoretical Contributions
Numerous theoretical contributions are made by this study and its findings. The study examines the significant factors in the use of CTAs to determine users' behavioral intention through the lens of the TAM, and the PCT and TTF theoretical models. Despite the advent of health apps, particularly tracking apps, and their use to restrict the transmission of the COVID-19 virus, an information system perspective on the interaction between adoption determinants remains necessary.
Firstly, the TAM was used to understand the predictive potential of behavioral intention (10, 54, 161). The TAM's key constructs were then incorporated into Tawakkalna, a new technology, to reveal the key factors of users' behavioral intention. The key variables of the TAM contributed significantly to the model and improved the investigation's outcomes. The TAM variables thus explain why IS and geolocation technology have been adopted to prevent or reduce coronavirus transmission.
Secondly, through PCT, it was revealed that the public might be persuaded to embrace the app and to sacrifice their privacy and social concerns. Still, complications could arise due to the loss of privacy, higher-level notifications on devices, or the software's incompatibility in other countries (10). Accordingly, one of this study's key focuses is the negative effect of privacy on behavioral intentions. Several studies have focused on users' privacy on tracing apps, the measures to protect people's privacy (162, 163), or individuals' concerns regarding privacy issues (10). This study reveals that when it comes to health issues, individuals are unconcerned with privacy risk. These outcomes support the findings by (10) regarding the insignificant impact of privacy. Additionally, the positively or negatively evaluated social risk and social interaction have not yielded positive results. This does not support assertions by Schleicher (4) and Settersten et al. (106) regarding losing relationships among the public.
Thirdly, while examining the TTF variables, the data reveal that users' perceptions of Tawakkalna's mobility are optimally connected with their task characteristics which influence their behavioral intention. Mobility and task characteristics have indicated a more significant effect on technology fit (77, 82, 84). Hence, this study emphasizes the vital importance of the TTF in addressing the satisfaction of users' needs by mobile CTAs. To our knowledge, this is the first time the TTF model has been used to investigate the adoption of CTA. Therefore, the study offers a theoretical contribution that advances the understanding of the effectiveness of TTF on behavioral usage.
Furthermore, IT adoption studies, using a hybrid measurement analysis, are lacking. Studies have suggested that more research should be undertaken that combines ML tools with structural equation modeling (SEM) (22, 23, 82, 132, 149). Hence, this study establishes the proportional importance of critical characteristics that precede the behavioral intention of Tawakkalna by using the two-stage SEM–ANN analysis to address this gap by ranking these antecedents. The core factor of behavioral intention, namely, PU, helps users to adopt the app, while TTF is ranked second. These factors are shown to be conceptually and practically relevant, and this work contributes to the body of knowledge by directly measuring their relative importance utilizing a two-stage analysis.
This is the first empirical study to examine CTAs from a KSA perspective. Previously, CTA investigations have taken place in various countries, such as the UK (164), Germany (165), USA (159), Ireland (71), and Fiji (36). The differences in culture, infrastructure, legislation, and economies could impact on individuals' decision making (36). Research on theory development has emphasized the importance of measuring theories and models in the contexts of different countries (80). The findings of this study therefore contribute in this regard.
7.2. Practical Implications
COVID-19's spread has resulted in a pandemic, infecting millions of people and killing thousands. The use of notable measures by numerous countries has contained the spread of the coronavirus, enabling healthcare centers to care for the sick. However, this pandemic's constraints have severely affected the world economy. Moreover, stakeholders are demanding that movement control mechanisms should be enforced to control the spread. Health officials must be able to discover positive cases early and follow their prospective contacts. As a result, numerous technology-based tracking methods have been proposed, including mobile apps (1).
Even though the balance between health and the economy must favor the former, the economy has been severely harmed. Thus, tracking technology should be integrated into people's movements in order to protect population safety and facilitate the isolation of new positive cases and their associated contacts. This type of technology has been beneficial in countries such as Malaysia, South Korea, and Japan that were able to rapidly and safely decrease COVID-19's spread, reviving their economies through using geolocation-based apps (166, 167). The study's findings indicate that users' behavioral intentions (BI) are decided by the app's perceived usefulness (PU), perceived ease of use (PEoU), and task-technology fit (TTF). Concern about possible risks such as privacy risk, social risk, and associated benefits were not viewed as equally important by respondents. The choice of health was evident among respondents when they were confronted with choices, such as privacy and social risks.
Correspondingly, the knowledge obtained through this study will be beneficial to app developers who develop geolocation apps and to governments that decide on their use and the associated loss of privacy. Many discussions have been held concerning the loss of privacy rights, especially in liberal countries such as France, the UK, and the USA (8). Governments may find this study incredibly beneficial as they learn that users are particularly concerned about becoming infected or infecting their family members, with this concern significantly impacting on their decision to use the app. The user's intention is also influenced by his/her perception of vulnerability to COVID-19 coronavirus infection. Therefore, both anxiety and a sense of vulnerability, when combined with the perception of a high risk of COVID-19 infection, have a noticeable effect on behavioral intention. These findings corroborate the findings of recent studies (10, 166).
8. Future Work and Limitations
The study acknowledged few limitations. Firstly, respondents are from the KSA, demonstrating that our findings could only be applied to one geographical location. According to Li et al. (159), worries related to public health activate the behavioral immune system. This evolutionary adaptive process is culture-independent and, hence, the study's outcomes should be culture-independent. Therefore, the significant cross-cultural disparities in privacy concerns and potential users who did not use the CTA because of privacy concerns merit additional research. As mentioned earlier, certain cultures, such as those in China, Malaysia, and Japan may be more receptive to the government's acquisition and surveillance of personal data than cultures in more liberal countries.
The pandemic's long-term impact is unknown, although it is guaranteed to last longer than anticipated (4). However, one of the pandemic's most significant and immediate repercussions has been the way it has shattered the relationships among the public. While social participation may influence individuals' ability to adapt during a pandemic, physical separation measures also reveal and alter the character of the relationships among the public. Hence, this study suggests that this social issue is cause for concern. Important theoretical contributions might be made by explaining the mechanisms underlying these user's behaviors, particularly when weighing the risks and advantages of adopting CTAs.
Additionally, age has been demonstrated to be a moderator in numerous research published in the literature (80, 89) and CTA studies (159). The implication is that significant effects could potentially be tempered by age. However, this was not the study's objective, with no comparisons made between age groups. Consequently, future research could examine the acknowledged moderating effect of age. Finally, our findings may apply to other m-health technologies besides CTAs, such as electronic health records and wearables, although we lack the data to conclusively make this claim. As a result, additional research should be conducted to determine whether age acts as a moderator of the significance of the impact and to determine whether prominent disease concerns influence the adoption of other m-health technologies.
Furthermore, certain methodological limitations apply to the study. This includes the point that the data were collected through the snowball sampling approach. Hence, the sample is neither random nor fully representative of the population; this could result in a skewed sample of respondents, raising the issue of socially desired responses. Future research could use other data collection techniques. Moreover, the proposed model does not cover some factors such as government support, facilitating conditions, and social influence. Examining these factors could enhance our understanding of CTA adoption. Therefore, future studies may consider investigating their effect on behavioral intention to adopt Twakkalna and other CTAs. Also, future studies could investigate the impact of trust, as widely reported in the technology adoption literature (22) and as one of the key variables in the extended TAM (TAM2) (168).
9. Conclusion
The search for interventions to quickly and effectively control COVID-19's spread, which has endangered humans despite the increasing efforts committed to managing the pandemic, motivated the researchers to undertake this study. A mobile contact-tracing app is introduced to help KSA citizens to take precautions and to enable health authorities track potential positive cases. The app has various functions, including controlling the spread of the virus without impeding citizens' movement and consequential economic loss. As a result, this study examines the various factors that can affect the app's acceptability by the general population. Based upon the TAM, and PCT and TTF theoretical models, which are commonly used theories regarding behavioral intention, the study investigated the antecedents of Tawakkalna contact tracing as conducted in the Kingdom of Saudi Arabia. Of the nine hypotheses, the six corresponding to TAM and the TTF model were supported. Specifically, PU and PEoU were found to be significant predictors of behavioral intention. Also, the findings demonstrated that three constructs derived from PCT, namely, privacy risk, social risk, and social interaction, were not significant determinants of behavioral intention. Furthermore, users' perceived mobility and task features to use Tawakkalna matched perfectly, explaining 66.8% of the variation of task-technology fit. These conclusions are based on a two-stage SEM–ANN analysis coupled with different testing methodologies. In conclusion, the findings may shed new light on the overall role of contact-tracing apps.
Data Availability Statement
The original contributions presented in the study are included in the article/supplementary material, further inquiries can be directed to the corresponding author/s.
Author Contributions
All authors listed have made a substantial, direct, and intellectual contribution to the work and approved it for publication.
Conflict of Interest
The authors declare that the research was conducted in the absence of any commercial or financial relationships that could be construed as a potential conflict of interest.
Publisher's Note
All claims expressed in this article are solely those of the authors and do not necessarily represent those of their affiliated organizations, or those of the publisher, the editors and the reviewers. Any product that may be evaluated in this article, or claim that may be made by its manufacturer, is not guaranteed or endorsed by the publisher.
Acknowledgments
Researchers would like to thank the Deanship of Scientific Research, Qassim University for funding the publication of this project.
References
1. Li J, Guo X. Global Deployment Mappings and Challenges of Contact-Tracing Apps for COVID-19. (2020). Available online at: https://papers.ssrn.com/sol3/papers.cfm?abstract_id=3609516
2. Azad MA, Arshad J, Akmal SMA, Riaz F, Abdullah S, Imran M, et al. A first look at privacy analysis of COVID-19 contact tracing mobile applications. IEEE Intern Things J. (2020) 8:15796–806. doi: 10.1109/JIOT.2020.3024180
3. Williams SN, Armitage CJ, Tampe T, Dienes K. Public attitudes towards COVID-19 contact tracing apps: a UK-based focus group study. Health Expect. (2021) 24:377–85. doi: 10.1111/hex.13179
4. Schleicher A. The Impact of COVID-19 on Education Insights From Education at a Glance 2020. (2020). Retrieved from: https://www.oecd.org/education/the-impact-of-COVID-19-on-education-insights-education-at-a-glance-2020.pdf
5. Sun K, Viboud C. Impact of contact tracing on SARS-CoV-2 transmission. Lancet Infect Dis. (2020) 20:876–7. doi: 10.1016/S1473-3099(20)30357-1
6. Ferretti L, Wymant C, Kendall M, Zhao L, Nurtay A, Bonsall D, et al. Quantifying dynamics of SARS-CoV-2 transmission suggests that epidemic control and avoidance is feasible through instantaneous digital contact tracing. MedRxiv. (2020) 1–1. doi: 10.1101/2020.03.08.20032946
7. Müller J, Kretzschmar M. Contact tracing-Old models and new challenges. Infect Dis Model. (2021) 6:222–31. doi: 10.1016/j.idm.2020.12.005
8. Akinbi A, Forshaw M, Blinkhorn V. Contact tracing apps for the COVID-19 pandemic: a systematic literature review of challenges and future directions for neo-liberal societies. Health Inform Sci Syst. (2021) 9:1–15. doi: 10.1007/s13755-021-00147-7
9. Rahman M. Here Are the Countries Using Google and Apple's COVID-19 Contact Tracing API. (2021). Available online at: https://www.xda-developers.com/google-apple-COVID-19-contact-tracing-exposure-notifications-api-app-list-countries/ (accessed May 9, 2022).
10. Velicia-Martin F, Cabrera-Sanchez JP, Gil-Cordero E, Palos-Sanchez PR. Researching COVID-19 tracing app acceptance: incorporating theory from the technological acceptance model. PeerJ Comput Sci. (2021) 7:e316. doi: 10.7717/peerj-cs.316
11. Altmann S, Milsom L, Zillessen H, Blasone R, Gerdon F, Bach RL, et al. Acceptability of app-based contact tracing for COVID-19: cross-country survey study. JMIR mHealth uHealth. (2020) 8:e19857. doi: 10.2196/19857
12. Mayor S. COVID-19: researchers launch app to track spread of symptoms in the UK. Br Med J. (2020) 368:m1263. doi: 10.1136/bmj.m1263
13. Gutierrez A, O'Leary S, Rana NP, Dwivedi YK, Calle T. Using privacy calculus theory to explore entrepreneurial directions in mobile location-based advertising: identifying intrusiveness as the critical risk factor. Comput Hum Behav. (2019) 95:295–306. doi: 10.1016/j.chb.2018.09.015
14. Krishen AS, Raschke RL, Close AG, Kachroo P. A power-responsibility equilibrium framework for fairness: understanding consumers' implicit privacy concerns for location-based services. J Bus Res. (2017) 73:20–9. doi: 10.1016/j.jbusres.2016.12.002
15. Limpf N, Voorveld HA. Mobile location-based advertising: how information privacy concerns influence consumers' attitude and acceptance. J Interact Advert. (2015) 15:111–23. doi: 10.1080/15252019.2015.1064795
16. Zhao L, Lu Y, Gupta S. Disclosure intention of location-related information in location-based social network services. Int J Electron Commerce. (2012) 16:53–90. doi: 10.2753/JEC1086-4415160403
17. Miltgen CL, Peyrat-Guillard D. Cultural and generational influences on privacy concerns: a qualitative study in seven European countries. Eur J Inform Syst. (2014) 23:103–25. doi: 10.1057/ejis.2013.17
18. Rowe F. Contact tracing apps and values dilemmas: a privacy paradox in a neo-liberal world. Int J Inform Manage. (2020) 55:102178. doi: 10.1016/j.ijinfomgt.2020.102178
19. Wang T, Duong TD, Chen CC. Intention to disclose personal information via mobile applications: A privacy calculus perspective. Int J Inform Manage. (2016) 36:531–42. doi: 10.1016/j.ijinfomgt.2016.03.003
20. Vaughan A. The problems with contact-tracing apps. New Sci. (2020) 246:9. doi: 10.1016/S0262-4079(20)30787-9
21. Kaspar K. Motivations for social distancing and app use as complementary measures to combat the COVID-19 pandemic: quantitative survey study. J Med Intern Res. (2020) 22:e21613. doi: 10.2196/21613
22. Leong LY, Hew TS, Ooi KB, Chong AYL. Predicting the antecedents of trust in social commerce-A hybrid structural equation modeling with neural network approach. J Bus Res. (2020) 110:24–40. doi: 10.1016/j.jbusres.2019.11.056
23. Alwabel ASA, Zeng XJ. Data-driven modeling of technology acceptance: A machine learning perspective. Expert Syst Appl. (2021) 185:115584. doi: 10.1016/j.eswa.2021.115584
24. Hearn A. Digital Contact Tracing Will Fail Unless Privacy Is Respected, Experts Warn. Guard. Newspaper (2020).
25. Fussell S. The Apple-Google Contact Tracing Plan Won't Stop COVID Alone. Conde Nast. (2020). Available online at: https://www.wired.com/story/apple-google-contact-tracing-wont-stop-covid-alone
26. Parker MJ, Fraser C, Abeler-Dörner L, Bonsall D. Ethics of instantaneous contact tracing using mobile phone apps in the control of the COVID-19 pandemic. J Med Ethics. (2020) 46:427–31. doi: 10.1136/medethics-2020-106314
27. Vokinger KN, Nittas V, Witt CM, Fabrikant SI, Von Wyl V. Digital health and the COVID-19 epidemic: an assessment framework for apps from an epidemiological and legal perspective. Swiss Med Weekly. (2020) 2020:20282. doi: 10.4414/smw.2020.20282
28. Kretzschmar ME, Rozhnova G, Bootsma MC, van Boven M, van de Wijgert JH, Bonten MJ. Impact of delays on effectiveness of contact tracing strategies for COVID-19: a modelling study. Lancet Publ Health. (2020) 5:e452–9. doi: 10.1016/S2468-2667(20)30157-2
29. Kucharski AJ, Klepac P, Conlan AJ, Kissler SM, Tang ML, Fry H, et al. Effectiveness of isolation, testing, contact tracing, and physical distancing on reducing transmission of SARS-CoV-2 in different settings: a mathematical modelling study. Lancet Infect Dis. (2020) 20:1151–60. doi: 10.1016/S1473-3099(20)30457-6
30. Leslie M. COVID-19 fight enlists digital technology: contact tracing apps. Engineering. (2020) 6:1064. doi: 10.1016/j.eng.2020.09.001
31. Xu W, Wu J, Cao L. COVID-19 pandemic in China: Context, experience and lessons. Health Policy Technol. (2020) 9:639–48. doi: 10.1016/j.hlpt.2020.08.006
32. Howell B. The countries who've handled covid-19 the best and worst. MoveHub. (2022). Available online at: https://www.movehub.com/blog/best-and-worst-covid-responses/ (accessed May 9, 2022).
33. Hale T, Angrist N, Goldszmidt R, Kira B, Petherick A, Phillips T, et al. A global panel database of pandemic policies (Oxford COVID-19 Government Response Tracker). Nat Hum Behav. (2021) 5:529–38. doi: 10.1038/s41562-021-01079-8
34. Hassandoust F, Akhlaghpour S, Johnston AC. Individuals' privacy concerns and adoption of contact tracing mobile applications in a pandemic: a situational privacy calculus perspective. J Am Med Inform Assoc. (2021) 28:463–71. doi: 10.1093/jamia/ocaa240
35. Walrave M, Waeterloos C, Ponnet K. Ready or not for contact tracing? Investigating the adoption intention of COVID-19 contact-tracing technology using an extended unified theory of acceptance and use of technology model. Cyberpsychol Behav Soc Netw. (2021) 24:377–83. doi: 10.1089/cyber.2020.0483
36. Sharma S, Singh G, Sharma R, Jones P, Kraus S, Dwivedi YK. Digital health innovation: exploring adoption of COVID-19 digital contact tracing apps. IEEE Trans Eng Manage. (2020) 2020:3019033. doi: 10.1109/TEM.2020.3019033
37. Duan SX, Deng H. Hybrid analysis for understanding contact tracing apps adoption. Indus Manage Data Syst. (2021) 21:1599–616. doi: 10.1108/IMDS-12-2020-0697
38. Blom AG, Wenz A, Cornesse C, Rettig T, Fikel M, Friedel S, et al. Barriers to the large-scale adoption of the COVID-19 contact-tracing app in Germany: survey study. J Med Intern Res. (2021) 23:e23362. doi: 10.2196/23362
39. Nguyen TT, Nguyen TCAH, Tran CD. Exploring individuals' adoption of COVID-19 contact-tracing apps: a mixed-methods approach. Library Hi Tech. (2021) 40:376–93. doi: 10.1108/LHT-06-2021-0180
40. Dowthwaite L, Fischer J, Vallejos EP, Portillo V, Nichele E, Goulden M, et al. Public adoption of and trust in the NHS COVID-19 contact tracing app in the United Kingdom: quantitative online survey study. J Med Intern Res. (2021) 23:e29085. doi: 10.2196/29085
41. Meier Y, Meinert J, Krämer NC. Investigating factors that affect the adoption of COVID-19 contact-tracing apps: A privacy calculus perspective. Technol Mind Behav. (2021) 2:1–10. doi: 10.1037/tmb0000040
42. Alsyouf A. Mobile Health for Covid-19 pandemic surveillance in developing countries: the case of Saudi Arabia. Solid State Technol. (2020) 63:2474–85.
43. tawakkalna. (n.d.). Tawakkalna. Available online at: https://ta.sdaia.gov.sa/en/index (accessed May 8, 2022).
44. Hidayat-ur Rehman I, Ahmad A, Ahmed M, Alam A. Mobile applications to fight against COVID-19 pandemic: the case of Saudi Arabia. J Technol Educ Manage Inform. (2021) 10:69–77. doi: 10.18421/TEM101-09
45. Orfali R, Perveen S, Aati HY, Al-Taweel A. nCOVID-19 outcomes on curfews and lockdown: Precautionary decisions in Saudi Arabia. Health Policy Technol. (2021) 2021:100538. doi: 10.1016/j.hlpt.2021.100538
46. Davis FD. Perceived usefulness, perceived ease of use, and user acceptance of information technology. MIS Quart. (1989) 13:319–40. doi: 10.2307/249008
47. Davis FD, Bagozzi RP, Warshaw PR. User acceptance of computer technology: a comparison of two theoretical models. Manage Sci. (1989) 35:982–1003. doi: 10.1287/mnsc.35.8.982
48. Davis FD, Bagozzi RP, Warshaw PR. Extrinsic and intrinsic motivation to use computers in the workplace 1. J Appl Soc Psychol. (1992) 22:1111–32. doi: 10.1111/j.1559-1816.1992.tb00945.x
49. Liu ZY. An analysis of technology acceptance model-exploring user acceptance and intension of taxi-hailing app in Shanghai (Bachelor's thesis). Gothenburg University, Gothenburg, Sweden (2015). Available online at: https://gupea.ub.gu.se/handle/2077/38592
50. King WR, He J. A meta-analysis of the technology acceptance model. Inform Manage. (2006) 43:740–55. doi: 10.1016/j.im.2006.05.003
51. Alkhalifah A, D'Ambra J. Factors effecting user adoption of I dentity management systems: an empirical study. In: PACIS. Hanoi (2012). p. 182.
52. Taherdoost H. A review of technology acceptance and adoption models and theories. Proc Manuf. (2018) 22:960–7. doi: 10.1016/j.promfg.2018.03.137
53. Turner M, Kitchenham B, Brereton P, Charters S, Budgen D. Does the technology acceptance model predict actual use? A systematic literature review. Inform Softw Technol. (2010) 52:463–79. doi: 10.1016/j.infsof.2009.11.005
54. Munoz-Leiva F, Climent-Climent S, Liébana-Cabanillas F. Determinants of intention to use the mobile banking apps: an extension of the classic TAM model. Spanish J Mark ESIC. (2017) 21:25–38. doi: 10.1016/j.sjme.2016.12.001
55. Chen Cf, de Rubens GZ, Xu X, Li J. Coronavirus comes home? Energy use, home energy management, and the social-psychological factors of COVID-19. Energy Res Soc Sci. (2020) 68:101688. doi: 10.1016/j.erss.2020.101688
56. Yuesti A, Ayu Asri Pramesti IG, Verawati Y. Interests of individual taxpayers using e-filing for tax reporting during the pandemic COVID-19. Talent Dev Excell. (2020) 12:4284–92.
57. Cho H, Chi C, Chiu W. Understanding sustained usage of health and fitness apps: incorporating the technology acceptance model with the investment model. Technol Soc. (2020) 63:101429. doi: 10.1016/j.techsoc.2020.101429
59. Keith MJ, Babb Jr JS, Furner CP, Abdullat A. Privacy assurance and network effects in the adoption of location-based services: An iPhone experiment. In: ICIS 2010 Proceedings (2010). p. 237. Available online at: https://aisel.aisnet.org/icis2010_submissions/237
60. Sun Y, Wang N, Shen XL, Zhang JX. Location information disclosure in location-based social network services: privacy calculus, benefit structure, and gender differences. Comput Hum Behav. (2015) 52:278–92. doi: 10.1016/j.chb.2015.06.006
61. Xu H, Luo XR, Carroll JM, Rosson MB. The personalization privacy paradox: an exploratory study of decision making process for location-aware marketing. Decis Support Syst. (2011) 51:42–52. doi: 10.1016/j.dss.2010.11.017
62. Pentina I, Zhang L, Bata H, Chen Y. Exploring privacy paradox in information-sensitive mobile app adoption: a cross-cultural comparison. Comput Hum Behav. (2016) 65:409–19. doi: 10.1016/j.chb.2016.09.005
63. Lowry PB, Moody G, Vance A, Jensen M, Jenkins J, Wells T. Using an elaboration likelihood approach to better understand the persuasiveness of website privacy assurance cues for online consumers. J Am Soc Inform Sci Technol. (2012) 63:755–76. doi: 10.1002/asi.21705
64. Shah MH, Peikari HR, Yasin NM. The determinants of individuals' perceived e-security: evidence from Malaysia. Int J Inform Manage. (2014) 34:48–57. doi: 10.1016/j.ijinfomgt.2013.10.001
65. Hann IH, Hui KL, Lee SYT, Png IP. Overcoming online information privacy concerns: an information-processing theory approach. J Manage Inform Syst. (2007) 24:13–42. doi: 10.2753/MIS0742-1222240202
66. Barth S, De Jong MD. The privacy paradox-Investigating discrepancies between expressed privacy concerns and actual online behavior-A systematic literature review. Telem Inform. (2017) 34:1038–58. doi: 10.1016/j.tele.2017.04.013
67. Wottrich VM, van Reijmersdal EA, Smit EG. The privacy trade-off for mobile app downloads: the roles of app value, intrusiveness, and privacy concerns. Decis Support Syst. (2018) 106:44–52. doi: 10.1016/j.dss.2017.12.003
68. Dinev T, Xu H, Smith JH, Hart P. Information privacy and correlates: an empirical attempt to bridge and distinguish privacy-related concepts. Eur J Inform Syst. (2013) 22:295–316. doi: 10.1057/ejis.2012.23
69. Kim DJ. Self-perception-based versus transference-based trust determinants in computer-mediated transactions: a cross-cultural comparison study. J Manage Inform Syst. (2008) 24:13–45. doi: 10.2753/MIS0742-1222240401
70. Li H, Sarathy R, Xu H. The role of affect and cognition on online consumers' decision to disclose personal information to unfamiliar online vendors. Decis Support Syst. (2011) 51:434–45. doi: 10.1016/j.dss.2011.01.017
71. Fox G, Clohessy T, van der Werff L, Rosati P, Lynn T. Exploring the competing influences of privacy concerns and positive beliefs on citizen acceptance of contact tracing mobile applications. Comput Hum Behav. (2021) 121:106806. doi: 10.1016/j.chb.2021.106806
72. Bhattacherjee A. Understanding information systems continuance: an expectation-confirmation model. MIS Quart. (2001) 25:351–70. doi: 10.2307/3250921
73. Venkatesh V, Morris MG, Davis GB, Davis FD. User acceptance of information technology: toward a unified view. MIS Quart. (2003) 27:425–78. doi: 10.2307/30036540
74. Gerhart N, Peak DA, Prybutok VR. Searching for New answers: the application of task-technology fit to E-Textbook usage. Decis Sci J Innov Educat. (2015) 13:91–111. doi: 10.1111/dsji.12056
75. Goodhue DL, Thompson RL. Task-technology fit and individual performance. MIS Quart. (1995) 19:213–36. doi: 10.2307/249689
76. Howard MC, Rose JC. Refining and extending task-technology fit theory: creation of two task-technology fit scales and empirical clarification of the construct. Inform Manage. (2019) 56:103134. doi: 10.1016/j.im.2018.12.002
77. Tam C, Oliveira T. Understanding the impact of m-banking on individual performance: DeLone & McLean and TTF perspective. Comput Hum Behav. (2016) 61:233–44. doi: 10.1016/j.chb.2016.03.016
78. Lin TC, Huang CC. Understanding knowledge management system usage antecedents: an integration of social cognitive theory and task technology fit. Inform Manage. (2008) 45:410–7. doi: 10.1016/j.im.2008.06.004
79. Zhou T, Lu Y, Wang B. Integrating TTF and UTAUT to explain mobile banking user adoption. Comput Hum Behav. (2010) 26:760–7. doi: 10.1016/j.chb.2010.01.013
80. Venkatesh V, Thong JY, Xu X. Consumer acceptance and use of information technology: extending the unified theory of acceptance and use of technology. MIS Quart. (2012) 36:157–78. doi: 10.2307/41410412
81. Mallat N, Rossi M, Tuunainen VK, Öörni A. The impact of use context on mobile services acceptance: the case of mobile ticketing. Inform Manage. (2009) 46:190–5. doi: 10.1016/j.im.2008.11.008
82. Li Y, Yang S, Zhang S, Zhang W. Mobile social media use intention in emergencies among Gen Y in China: an integrative framework of gratifications, task-technology fit, and media dependency. Telem Inform. (2019) 42:101244. doi: 10.1016/j.tele.2019.101244
83. Kim MJ, Chung N, Lee CK, Preis MW. Motivations and use context in mobile tourism shopping: applying contingency and task-technology fit theories. Int J Tour Res. (2015) 17:13–24. doi: 10.1002/jtr.1957
84. Tam C, Oliveira T. Performance impact of mobile banking: using the task-technology fit (TTF) approach. Int J Bank Mark. (2016) 34, 434–457. doi: 10.1108/IJBM-11-2014-0169
85. Oliveira T, Faria M, Thomas MA, Popovič A. Extending the understanding of mobile banking adoption: when UTAUT meets TTF and ITM. Int J Inform Manage. (2014) 34:689–703. doi: 10.1016/j.ijinfomgt.2014.06.004
86. Reuter C, Kaufhold MA, Spielhofer T, Hahne AS. Social media in emergencies: a representative study on citizens' perception in Germany. Proc ACM Hum Comput Interact. (2017) 1:1–19. doi: 10.1145/3134725
87. Tan ML, Prasanna R, Stock K, Hudson-Doyle E, Leonard G, Johnston D. Mobile applications in crisis informatics literature: a systematic review. Int J Disast Risk Reduct. (2017) 24:297–311. doi: 10.1016/j.ijdrr.2017.06.009
88. Reuter C, Kaufhold MA. Fifteen years of social media in emergencies: a retrospective review and future directions for crisis informatics. J Conting Crisis Manage. (2018) 26:41–57. doi: 10.1111/1468-5973.12196
89. Venkatesh V. Adoption and use of AI tools: a research agenda grounded in UTAUT. Ann Oper Res. (2021) 308:641–52. doi: 10.1007/s10479-020-03918-9
90. Kamble S, Gunasekaran A, Arha H. Understanding the Blockchain technology adoption in supply chains-Indian context. Int J Prod Res. (2019) 57:2009–33. doi: 10.1080/00207543.2018.1518610
91. Chao CM. Factors determining the behavioral intention to use mobile learning: an application and extension of the UTAUT model. Front Psychol. (2019) 10:1652. doi: 10.3389/fpsyg.2019.01652
92. Phua PL, Wong SL, Abu R. Factors influencing the behavioural intention to use the internet as a teaching-learning tool in home economics. Proc Soc Behav Sci. (2012) 59:180–7. doi: 10.1016/j.sbspro.2012.09.263
93. Cho K, Van Merriënboer B, Gulcehre C, Bahdanau D, Bougares F, Schwenk H, et al. Learning phrase representations using RNN encoder-decoder for statistical machine translation. arXiv[Preprint].arXiv:14061078. (2014). doi: 10.3115/v1/D14-1179
94. Whitten P, Doolittle G, Mackert M. Providers' acceptance of telehospice. J Palliat Med. (2005) 8:730–5. doi: 10.1089/jpm.2005.8.730
95. Olver IN, Selva-Nayagam S. Evaluation of a telemedicine link between Darwin and Adelaide to facilitate cancer management. Telemed J. (2000) 6:213–8. doi: 10.1089/107830200415144
96. Elkaseh AM, Wong KW, Fung CC. Perceived ease of use and perceived usefulness of social media for e-learning in Libyan higher education: a structural equation modeling analysis. Int J Inform Educ Technol. (2016) 6:192. doi: 10.7763/IJIET.2016.V6.683
97. Malhotra NK, Kim SS, Agarwal J. Internet users' information privacy concerns (IUIPC): the construct, the scale, and a causal model. Inform Syst Res. (2004) 15:336–55. doi: 10.1287/isre.1040.0032
98. Hong W, Thong JY. Internet privacy concerns: an integrated conceptualization and four empirical studies. MIS Quart. (2013) 37:275–98. doi: 10.25300/MISQ/2013/37.1.12
99. Junglas IA, Johnson NA, Spitzmüller C. Personality traits and concern for privacy: an empirical study in the context of location-based services. Eur J Inform Syst. (2008) 17:387–402. doi: 10.1057/ejis.2008.29
100. Fodor M, Brem A. Do privacy concerns matter for Millennials? Results from an empirical analysis of location-based services adoption in Germany. Comput Hum Behav. (2015) 53:344–53. doi: 10.1016/j.chb.2015.06.048
101. Zhu H, Ou CX, van den Heuvel WJA, Liu H. Privacy calculus and its utility for personalization services in e-commerce: an analysis of consumer decision-making. Inform Manage. (2017) 54:427–37. doi: 10.1016/j.im.2016.10.001
102. Geetha V, RanGaRajan K. A conceptual framework for perceived risk in consumer online shopping. Glob Manage Rev. (2015) 10:1–19.
103. Lunn PD, Timmons S, Belton CA, Barjaková M, Julienne H, Lavin C. Motivating social distancing during the COVID-19 pandemic: an online experiment. Soc Sci Med. (2020) 265:113478. doi: 10.1016/j.socscimed.2020.113478
104. Greenstone M, Nigam V. Does Social Distancing Matter? University of Chicago, Becker Friedman Institute for Economics Working Paper (2020). doi: 10.2139/ssrn.3561244
105. Ferguson NM, Laydon D, Nedjati-Gilani G, Imai N, Ainslie K, Baguelin M, et al. Impact of non-pharmaceutical interventions (NPIs) to reduce COVID-19 mortality and healthcare demand. Imper Coll COVID-19 Resp Team. (2020) 1–20. doi: 10.25561/77482
106. Settersten RA Jr, Bernardi L, Härkönen J, Antonucci TC, Dykstra PA, Heckhausen J, et al. Understanding the Effects of COVID-19 Through a Life Course Lens. Elsevier (2020) 45:100360. doi: 10.1016/j.alcr.2020.100360
107. Gómez D, Bernardos AM, Portillo JI, Tarrío P, Casar JR. A review on mobile applications for citizen emergency management. In: International Conference on Practical Applications of Agents and Multi-Agent Systems. Berlin; Heidelberg: Springer (2013). p. 190–201. doi: 10.1007/978-3-642-38061-7_19
108. Khan IU, Hameed Z, Yu Y, Islam T, Sheikh Z, Khan SU. Predicting the acceptance of MOOCs in a developing country: application of task-technology fit model, social motivation, and self-determination theory. Telem Inform. (2018) 35:964–78. doi: 10.1016/j.tele.2017.09.009
109. Wu B, Chen X. Continuance intention to use MOOCs: integrating the technology acceptance model (TAM) and task technology fit (TTF) model. Comput Hum Behav. (2017) 67:221–32. doi: 10.1016/j.chb.2016.10.028
110. Tongco MDC. Purposive sampling as a tool for informant selection. Ethnobot Res Appl. (2007) 5:147–58. doi: 10.17348/era.5.0.147-158
111. Cooper DR, Schindler PS, Sun J. Business Research Methods. Vol. 9. New York, NY: McGraw-Hill (2006).
113. Sheng H, Nah FFH, Siau K. An experimental study on ubiquitous commerce adoption: impact of personalization and privacy concerns. J Assoc Inform Syst. (2008) 9:15. doi: 10.17705/1jais.00161
114. Biernacki P, Waldorf D. Snowball sampling: problems and techniques of chain referral sampling. Sociol Methods Res. (1981) 10:141–63. doi: 10.1177/004912418101000205
115. MacKenzie SB, Podsakoff PM, Podsakoff NP. Construct measurement and validation procedures in MIS and behavioral research: Integrating new and existing techniques. MIS Quart. (2011) 35:293–334. doi: 10.2307/23044045
116. Hair Jr JF, Sarstedt M, Ringle CM, Gudergan SP. Advanced Issues in Partial Least Squares Structural Equation Modeling. Los Angeles, CA: Sage Publications (2017). doi: 10.15358/9783800653614
117. Stevens JP. Exploratory and confirmatory factor analysis. In: Applied Multivariate Statistics for the Social Sciences. Routledge (2012). p. 337–406.
118. Liébana-Cabanillas F, Marinkovic V, de Luna IR, Kalinic Z. Predicting the determinants of mobile payment acceptance: a hybrid SEM-neural network approach. Technol Forecast Soc Change. (2018) 129:117–30. doi: 10.1016/j.techfore.2017.12.015
119. Chin WW. Commentary: Issues and opinion on structural equation modeling. MIS Quart. (1998) 22:vii–xvi.
120. Gefen D, Rigdon E, Straub D. An update and extension to SEM guidelines for administrative and social science research. Editorial comment. MIS Quart. (2011) 35:3–12. doi: 10.2307/23044042
121. Churchill Jr GA. A paradigm for developing better measures of marketing constructs. J Mark Res. (1979) 16:64–73. doi: 10.1177/002224377901600110
122. Hinkin TR. A brief tutorial on the development of measures for use in survey questionnaires. Organ Res Methods. (1998) 1:104–21. doi: 10.1177/109442819800100106
123. Straub D, Boudreau MC, Gefen D. Validation guidelines for IS positivist research. Commun Assoc Inform Syst. (2004) 13:24. doi: 10.17705/1CAIS.01324
124. Straub D, Limayem M, Karahanna-Evaristo E. Measuring system usage: implications for IS theory testing. Manage Sci. (1995) 41:1328–42. doi: 10.1287/mnsc.41.8.1328
125. Barlette Y, Jaouen A, Baillette P. Bring Your Own Device (BYOD) as reversed IT adoption: insights into managers' coping strategies. Int J Inform Manage. (2021) 56:102212. doi: 10.1016/j.ijinfomgt.2020.102212
126. Podsakoff PM, MacKenzie SB, Podsakoff NP. Sources of method bias in social science research and recommendations on how to control it. Annu Rev Psychol. (2012) 63:539–69. doi: 10.1146/annurev-psych-120710-100452
127. Field A. Discovering Statistics Using SPSS: Book Plus Code for E Version of Text. London: SAGE Publications Limited (2009). p. 896.
128. Leong LY, Jaafar NI, Ainin S. Understanding Facebook commerce (f-commerce) actual purchase from an artificial neural network perspective. J Electron Commer Res. (2018) 19:75–103. doi: 10.1037/t67487-000
129. Hair Jr JF, Hult GTM, Ringle CM, Sarstedt M. A Primer on Partial Least Squares Structural Equation Modeling (PLS-SEM). Magdeburg: Sage Publications (2021). doi: 10.1007/978-3-030-80519-7
130. Sarstedt M, Ringle CM, Hair JF. Partial least squares structural equation modeling. Handb Mark Res. (2017) 26:1–40. doi: 10.1007/978-3-319-05542-8_15-1
131. Hew JJ, Badaruddin MNBA, Moorthy MK. Crafting a smartphone repurchase decision making process: do brand attachment and gender matter? Telem Inform. (2017) 34:34–56. doi: 10.1016/j.tele.2016.12.009
132. Hew JJ, Leong LY, Tan GWH, Lee VH, Ooi KB. Mobile social tourism shopping: a dual-stage analysis of a multi-mediation model. Tour Manage. (2018) 66:121–39. doi: 10.1016/j.tourman.2017.10.005
133. Scott JE, Walczak S. Cognitive engagement with a multimedia ERP training tool: assessing computer self-efficacy and technology acceptance. Inform Manage. (2009) 46:221–32. doi: 10.1016/j.im.2008.10.003
134. Chan FT, Chong AY. A SEM-neural network approach for understanding determinants of interorganizational system standard adoption and performances. Decis Support Syst. (2012) 54:621–30. doi: 10.1016/j.dss.2012.08.009
135. Teo AC, Tan GWH, Ooi KB, Hew TS, Yew KT. The effects of convenience and speed in m-payment. Indus Manage Data Syst. (2015) 115:311–31. doi: 10.1108/IMDS-08-2014-0231
136. Hussain W, Merigó JM, Raza MR. Predictive intelligence using ANFIS-induced OWAWA for complex stock market prediction. Int J Intell Syst. (2021) 1–1. doi: 10.1002/int.22732
137. Hussain W, Merigó JM, Raza MR, Gao H. A new QoS prediction model using hybrid IOWA-ANFIS with Fuzzy C-means, subtractive clustering and grid partitioning. Inform Sci. (2022) 584:280–300. doi: 10.1016/j.ins.2021.10.054
138. Joshi R, Yadav R. An integrated SEM neural network approach to study effectiveness of brand extension in Indian FMCG industry. Business Perspect Res. (2018) 6:113–28. doi: 10.1177/2278533718764502
139. Ahani A, Rahim NZA, Nilashi M. Forecasting social CRM adoption in SMEs: a combined SEM-neural network method. Comput Hum Behav. (2017) 75:560–78. doi: 10.1016/j.chb.2017.05.032
140. Qasem YA, Asadi S, Abdullah R, Yah Y, Atan R, Al-Sharafi MA, et al. A multi-analytical approach to predict the determinants of cloud computing adoption in higher education institutions. Appl Sci. (2020) 10:4905. doi: 10.3390/app10144905
141. by Statista Research Department P, 7, J. Time Spent on Average on a Smartphone in the U.S. (2021). Available online at: https://www.statista.com/statistics/1224510/time-spent-per-day-on-smartphone-us/ (accessed May 9, 2022).
142. Rumsey DJ. How to Interpret Standard Deviation in a Statistical Data Set.(2018). Retrieved from: https://www.dummies.com/article/academics-the-arts/math/statistics/how-to-interpret-standard-deviation-in-a-statistical-data-set-169772/ (accessed May 9, 2021).
143. Oppong FB, Agbedra SY. Assessing univariate and multivariate normality. A guide for non-statisticians. Math Theory Model. (2016) 6:26–33.
144. Kline RB. Principles and Practice of Structural Equation Modeling. Kuala Lumpur: Guilford Publications (2015).
145. Ramayah T, Cheah J, Chuah F, Ting H, Memon MA. Partial Least Squares Structural Equation Modeling (PLS-SEM) Using smartpls 3.0. Kuala Lumpur: Pearson (2018).
146. Hayes AF, Scharkow M. The relative trustworthiness of inferential tests of the indirect effect in statistical mediation analysis: does method really matter? Psychol Sci. (2013) 24:1918–27. doi: 10.1177/0956797613480187
147. Streukens S, Leroi-Werelds S. Bootstrapping and PLS-SEM: a step-by-step guide to get more out of your bootstrap results. Eur Manage J. (2016) 34:618–32. doi: 10.1016/j.emj.2016.06.003
148. Cohen J. Statistical Power Analysis for the Behavioral Sciences. 2nd ed. Hillsdale, NJ: Lawrence Erlbaum Associates (1988).
149. Chong AYL. Predicting m-commerce adoption determinants: a neural network approach. Expert Syst Appl. (2013) 40:523–30. doi: 10.1016/j.eswa.2012.07.068
150. Chang PC, Lin JJ, Dzan WY. Forecasting of manufacturing cost in mobile phone products by case-based reasoning and artificial neural network models. J Intell Manufact. (2012) 23:517–31. doi: 10.1007/s10845-010-0390-7
151. Tan GWH, Ooi KB, Leong LY, Lin B. Predicting the drivers of behavioral intention to use mobile learning: a hybrid SEM-Neural Networks approach. Comput Hum Behav. (2014) 36:198–213. doi: 10.1016/j.chb.2014.03.052
152. LeNail A. NN-SVG: Publication-ready neural network architecture schematics. J Open Source Softw. (2019) 4:747.
153. Ooi KB, Tan GWH. Mobile technology acceptance model: an investigation using mobile users to explore smartphone credit card. Expert Syst Appl. (2016) 59:33–46. doi: 10.1016/j.eswa.2016.04.015
154. Saura JR, Palos-Sanchez P, Velicia-Martin F. What drives volunteers to accept a digital platform that supports NGO projects? Front Psychol. (2020) 11:429. doi: 10.3389/fpsyg.2020.00429
155. Palos-Sanchez PR, Correia MB, Saura JR. An empirical examination of adoption of mobile applications in Spain and Portugal, based in UTAUT. Int J Mobile Commun. (2019) 17:579–603. doi: 10.1504/IJMC.2019.102085
156. Kim D, Park K, Park Y, Ahn JH. Willingness to provide personal information: perspective of privacy calculus in IoT services. Comput Hum Behav. (2019) 92:273–81. doi: 10.1016/j.chb.2018.11.022
157. Fox G. To protect my health or to protect my health privacy? A mixed-methods investigation of the privacy paradox. J Assoc Inform Sci Technol. (2020) 71:1015–29. doi: 10.1002/asi.24369
158. Chan EY, Saqib NU. Privacy concerns can explain unwillingness to download and use contact tracing apps when COVID-19 concerns are high. Comput Hum Behav. (2021) 119:106718. doi: 10.1016/j.chb.2021.106718
159. Li T, Cobb C, Yang J, Baviskar S, Agarwal Y, Li B, et al. What makes people install a COVID-19 contact-tracing app? Understanding the influence of app design and individual difference on contact-tracing app adoption intention. Pervas Mobile Comput. (2021) 75:101439. doi: 10.1016/j.pmcj.2021.101439
160. Lu HP, Yang YW. Toward an understanding of the behavioral intention to use a social networking site: an extension of task-technology fit to social-technology fit. Comput Hum Behav. (2014) 34:323–32. doi: 10.1016/j.chb.2013.10.020
161. Melas CD, Zampetakis LA, Dimopoulou A, Moustakis V. Modeling the acceptance of clinical information systems among hospital medical staff: an extended TAM model. J Biomed Inform. (2011) 44:553–64. doi: 10.1016/j.jbi.2011.01.009
162. Liu JK, Au MH, Yuen TH, Zuo C, Wang J, Sakzad A, et al. Privacy-preserving COVID-19 contact tracing app: a zero-knowledge proof approach. IACR Cryptol ePrint Arch. (2020) 2020:528.
163. Yasaka TM, Lehrich BM, Sahyouni R. Peer-to-peer contact tracing: development of a privacy-preserving smartphone app. JMIR mHealth uHealth. (2020) 8:e18936. doi: 10.2196/18936
164. Horvath L, Banducci S, James O. Citizens' attitudes to contact tracing apps. J Exp Polit Sci. (2020) 9:118–30. doi: 10.1017/XPS.2020.30
165. Trang S, Trenz M, Weiger WH, Tarafdar M, Cheung CM. One app to trace them all? Examining app specifications for mass acceptance of contact-tracing apps. Eur J Inform Syst. (2020) 29:415–28. doi: 10.1080/0960085X.2020.1784046
166. Abeler J, Altmann S, Milsom L, Toussaert S, Zillessen H. Support in the UK for App-Based Contact Tracing of COVID-19. Oxford (2020).
167. De Carli A, Franco M, Gassmann A, Killer C, Rodrigues B, Scheid E, et al. WeTrace-a privacy-preserving mobile COVID-19 tracing approach and application. arXiv[Preprint].arXiv:200408812. (2020). Available online at: https://arxiv.org/abs/2004.08812v1
168. Venkatesh V, Davis FD. A theoretical extension of the technology acceptance model: four longitudinal field studies. Manage Sci. (2000) 46:186–204. doi: 10.1287/mnsc.46.2.186.11926
Appendix
Keywords: COVID-19, contact-tracing app, health, privacy, behavioral intention (BI), technology adoption
Citation: Alkhalifah A and Bukar UA (2022) Examining the Prediction of COVID-19 Contact-Tracing App Adoption Using an Integrated Model and Hybrid Approach Analysis. Front. Public Health 10:847184. doi: 10.3389/fpubh.2022.847184
Received: 13 January 2022; Accepted: 28 April 2022;
Published: 24 May 2022.
Edited by:
Ruy Freitas Reis, Universidade Federal de Juiz de Fora, BrazilReviewed by:
Carolina Ribeiro Xavier, Universidade Federal de São João del-Rei, BrazilDavid Cutting, Queen's University Belfast, United Kingdom
Copyright © 2022 Alkhalifah and Bukar. This is an open-access article distributed under the terms of the Creative Commons Attribution License (CC BY). The use, distribution or reproduction in other forums is permitted, provided the original author(s) and the copyright owner(s) are credited and that the original publication in this journal is cited, in accordance with accepted academic practice. No use, distribution or reproduction is permitted which does not comply with these terms.
*Correspondence: Ali Alkhalifah, YS5hbGtoYWxpZmFoQHF1LmVkdS5zYQ==