- Department of Communication, University of Münster, Münster, Germany
The current study examined antecedents and possible consequences of smartphone use disorder (SmUD). In particular, we aimed to increase the understanding of the interplay of SmUD, mental health, and physical symptoms. Studies found that SmUD is associated with diverse psychological and physical health impairments, ranging from depression and anxiety to headaches and sleep disturbances. Based on existing works, we assumed that mental problems mediate the relationship between SmUD and bodily problems. We conducted a cross-sectional random-quota online survey among 938 German smartphone owners aged 14 to 64 years. An instrument based on the 5th edition of the Diagnostic and Statistical Manual of Mental Disorders (DSM-5) was used to measure SmUD severity. The data was analyzed using structural equation modeling. We identified a rate of 4.0% potentially disordered users. Males and younger participants showed more signs of SmUD. As expected, SmUD severity was found to be associated with physical (more frequent headaches, sleep disturbances, gastrointestinal problems) as well as psychological (higher levels of loneliness, stress, depression/anxiety) health impairments. Investigating the interplay of these variables showed that depression and anxiety, and stress partially mediated the relationship between SmUD severity and physical symptoms. Taken together, our results confirm that increased SmUD severity is associated with mental problems as well as with somatic symptoms. We assume complex (and presumably circular) relationships, which future studies should examine in more detail. SmUD prevention and intervention programs should follow a broad approach that considers decreases in physical and mental health, possibly causing or resulting from SmUD.
Introduction
Smartphone use has rapidly increased in recent years. Currently, about 85% of the US population are smartphone owners (1). In European countries like Germany, rates even reached almost 90% (2). Empirical studies indicate that the increasing diffusion and intensified use of smartphones may be connected to several risks, such as decreases in academic performance [e.g., (3–5)] or a higher danger of being involved in accidents due to distraction [e.g., (6, 7)]. Furthermore, several studies have identified connections between excessive smartphone use and mental problems, such as depression, anxiety, or stress symptoms [e.g., (8–12)]. There is also evidence that excessive smartphone use is associated with physical health impairments, such as sleep disturbances (10–13).
More and more researchers have raised the question of whether smartphone use can take on addictive forms that require professional treatment. Using different terms like smartphone addiction [e.g., (14, 15)], problematic smartphone use [e.g., (8, 16)], or smartphone dependence [e.g., (17, 18)], scholars investigate the extent to which users show signs of a problematic overuse–such as a loss of control, tolerance, or withdrawal symptoms. In the context of computer and videogames, the World Health Organization (WHO) uses the term Gaming Disorder to describe such problematic forms of use. Consequently, scientists have started to use the terms disorder or disordered use also in relation to other forms of addictive media technology use, like, for example, social media (use) disorder [e.g., (19, 20)]. Following Sha et al. (21) and others [e.g., (22, 23)] we will use the term Smartphone Use Disorder (SmUD) as a synonym for uncontrolled, addictive smartphone use in the following. As suggested by Montag et al. (24), we will abbreviate the term with SmUD because SUD could easily be misunderstood as an acronym for Substance Use Disorder.
Many existing studies on SmUD suffer from some methodological limitations. For example, it can be criticized that studies are often not comparable because of a lack of consistency in the diagnostic criteria that were applied. To date, no consensus about how to reliably measure disordered smartphone use has been achieved among scholars, resulting in a very high number of different scales [e.g., (15, 25, 26); for overviews, see (27, 28)]. Some of these instruments consisted of ad-hoc items, some adopted the substance abuse criteria from the 4th edition of the Diagnostic and Statistical Manual of Mental Disorders (DSM-4) by the American Psychiatric Association (APA), and some adopted criteria from screening tools that were originally designed to measure pathological gambling, Internet addiction, or addictive shopping (27). Further, a majority of studies on SmUD is based on self-recruited and non-representative samples (27).
The current study draws data from a random-quota sample of German smartphone owners aged 14 to 64 years. To date, computer and video gaming disorder is the only form of addictive media technology use that has been defined in relevant manuals like the 11th revision of the International Classification of Diseases (ICD-11) by the WHO (“Gaming Disorder”), or the DSM-5 by the APA (“Internet Gaming Disorder”; IGD). However, there are good reasons to assume that SmUD and IGD are overlapping concepts (24). For example, Leung et al. (29) found that SmUD scores and IGD scores significantly correlated, which can be explained by the fact that many smartphone users play mobile games (24). Accordingly, several studies showed that game use positively predicted SmUD [e.g., (30, 31)]. Similarly, social media use disorder scores were found to correlate with SmUD scores (29), and social networking site use was identified a predictor of SmUD [e.g., (30, 32)]. Consequently, it has been argued that IGD and other types of Internet technology use disorders (like social media use disorder), “should […] be defined by the same set of diagnostic criteria” [(33), p. 479]. In line with this argumentation, some recent studies have adopted the DSM-5 IGD criteria to measure disordered (addictive) smartphone use [e.g., (16, 34, 35)]. We follow this approach and use a DSM-5 based scale to measure SmUD.
The central aim of the current study was to deepen the understanding of the antecedents and possible consequences of SmUD. Based on theoretical considerations and previous findings, we constructed and tested a structural model that is presented in Figure 1. The different paths and hypotheses will be explained in detail in the following section.
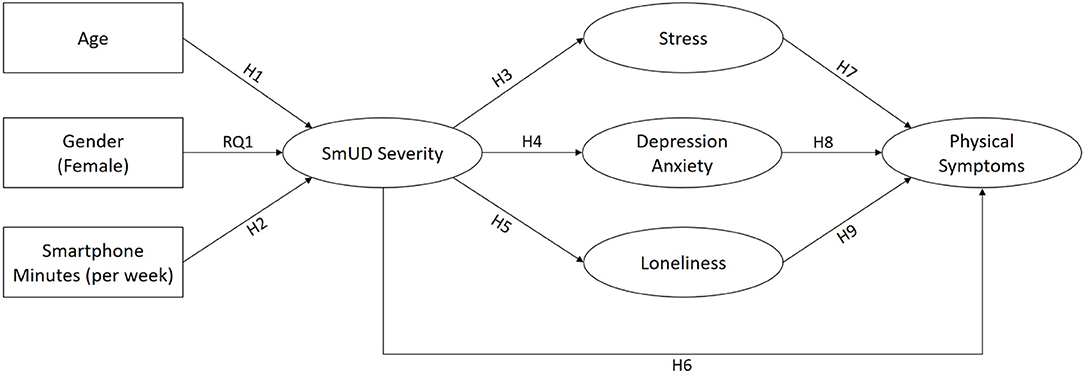
Figure 1. Predicted structural model labeled with hypotheses and research questions. SmUD, smartphone use disorder.
Antecedents and Consequences of Smartphone Use Disorder
Existing studies have shown that age and gender play a role in the development of SmUD (11). Concerning age, research findings indicate that younger users constitute a particular at-risk group for SmUD [e.g., (14, 16, 36–38)]. Accordingly, we hypothesize that age is negatively related to SmUD severity (hypothesis H1). The relationship between gender and SmUD remained somewhat unclear with mixed results concerning the question of whether males or females are more at risk for the development of SmUD (14, 39, 40). Consequently, we ask how gender is related to SmUD (research question RQ1). Several studies have shown that higher smartphone usage times are associated with increased SmUD severity [e.g., (16, 36, 41, 42)]. We therefore hypothesize that smartphone minutes per week are positively related to SmUD severity (hypothesis H2).
A growing body of research focuses on examining psychosocial correlates of SmUD. Some studies considered variables such as stress, depression, anxiety, or loneliness as predictors of SmUD, while others argued that SmUD may lead to psychosocial problems (11). Both of these assumptions are plausible form a theoretical perspective and can be explained by the Interaction of Person-Affect-Cognition-Execution model [I-PACE; (43, 44)], one of the most established theoretical models to explain the development and maintenance of addictive behaviors. I-PACE considers psychopathology (e.g., depression and anxiety) as well as particular social cognitions (e.g., loneliness) as general predisposing variables that can increase the risk to develop addictive media use (43, 44). However, in the later stages of the “addiction process”, excessive, disordered forms of use may also cause or further reinforce daily life problems, leading to social isolation and negative mental conditions [(43), p. 252]. In line with these assumptions, several cross-sectional studies have found loneliness, stress, anxiety, and depression were positively associated with SmUD [e.g., (8, 39, 45–49)]. Recent longitudinal studies have indicated that SmUD can lead to increases in loneliness and mental problems over time (50, 51). Against this background, we hypothesize that SmUD severity is positively related to higher levels of stress (hypothesis H3), depression and anxiety (hypothesis H4), and loneliness (hypothesis H5).
Physical health impairments possibly resulting from SmUD have much less often been examined than associations between SmUD and mental problems (11). However, there is some empirical evidence that SmUD is also related to somatic symptoms. For example, studies have reported a positive relationship between SmUD and sleep deprivation [e.g., (39, 46, 52, 53)]. Fewer studies have investigated other bodily symptoms potentially resulting from SmUD, such as headaches or neck pain [e.g., (54, 55)]. In the current study, we consider three physical symptoms (sleep disturbances, headaches, and gastrointestinal problems) and predict positive associations with SmUD severity (hypothesis H6).
Research has shown that physical symptoms (like gastrointestinal problems, insomnia, and migraines) and mental problems (like depression and anxiety) often co-occur and can reinforce each other [e.g., (56–58)]. For example, a longitudinal study among UK residents showed that anxiety and depression at baseline increased the risk of suffering from insomnia 12 months later and vice versa (59). Further, there is empirical evidence that experiencing stress can trigger headaches (60), impair sleep (61), and may cause gastrointestinal problems (62). Also, loneliness was often considered a risk factor for health problems and has been shown to be positively related to sleep disturbances (63) and other somatic symptoms, such as headaches and nausea (64). Accordingly, we assume that higher levels of stress (hypothesis H7), depression and anxiety (hypothesis H8), and loneliness (hypothesis H9) are positively related to physical symptoms.
Notably, most existing studies investigated direct relationships between measurements of SmUD and selected different aspects of physical wellbeing [e.g., (46, 52, 54, 55)]. Yet, little is known about the interplay of mental problems and physical symptoms associated with SmUD. Demirci et al. (39) have shown that depression and anxiety acted as mediators in the relationship between SmUD and sleep disturbances. They argued that SmUD may lead to depression and anxiety, which, in turn, may cause sleep problems. Similarly, Liu et al. (65) found that rumination mediated the relationship between SmUD and sleep quality. In light of these results and against the background of the theoretical considerations and empirical findings discussed above, we argue that SmUD may increase mental problems and loneliness, which, in turn, could increase the risk to experience sleep disturbances, headaches and gastrointestinal problems. In other words, we hypothesize indirect (mediated) relationships between SmUD severity and physical symptoms via stress (hypothesis H10), depression and anxiety (hypothesis H11), and loneliness (hypothesis H12).
Materials and Methods
Participants and Procedures
The current study was part of a larger online survey on media usage habits of German Internet users aged 14–64 years that was conducted in cooperation with a professional German survey research institute, adhering to the internationally recognized ICC/ESOMAR ethics code for social research and data analytics. The participants were informed about the general purpose of the study, consented in their participation, and had the right to opt out at any time. The participants were recruited via an online access panel and a random-quota procedure was applied to increase the representativeness of the data in terms of age, gender, education, and living region.
In total, 1,053 participants filled out the questionnaire. We screened the dataset for irregularities (i.e., straight-lining answers, very high numbers of missing values, obvious errors in answers to media use questions) and excluded 34 cases. Further, 81 non-smartphone owners were excluded, resulting in a final sample of N = 938 German smartphone owners aged 14–64 years. The mean age was 40.44 years (SD = 13.73). Gender was almost equally distributed with 455 female participants (48.5%) and 483 male participants (51.5%).
Measurements
Means, standard deviations, and Cronbach's alpha values for all measurements are reported in Table 1. Smartphone minutes per week, age, and gender were measured as self-reports.
Smartphone Use Disorder
Aiming to increase the validity and reliability of measuring SmUD, Hussain et al. (16) introduced the Problematic Smartphone Use Scale, which is an adaptation of the established IGDS9-SF by Pontes and Griffiths (66). The IGDS9-SF has been evaluated in numerous international studies [e.g., (67–70)] and is currently one of the most-often used instruments to measure IGD (71). It consists of nine items that were created based on the nine IGD criteria as defined by the APA in the DSM-5. Following the approach by Hussain et al. (16), we adapted a German version of the IGDS9-SF (72) to measure smartphone use instead of game use (e.g., “Do you use your smartphone in order to temporarily escape or relieve a negative mood (e.g., helplessness, guilt, anxiety)?”). The items were introduced by asking the participants about their smartphone usage over the past 12 months and each of the items had to be rated on a 5-point scale ranging from 1 = “never” to 5 = “very often”.
According to Pontes and Griffiths (66), higher scores on the scale can be interpreted as a higher tendency toward disordered use. For research purposes, participants with scores of 36 to 45 points (i.e., all questions, on average, answered with “often” or “very often”) can be considered potentially disordered game users (66). Hussain et al. (16) used the same criterion to assess the prevalence of SmUD.
Mental Problems
Depression and anxiety were measured with the German version (73) of the Patient Health Questionnaire (PHQ-4) by Kroenke et al. (74). The participants were asked to rate four items (e.g., “Feeling down, depressed, or hopeless.”) to measure their mental condition (“Over the last two weeks, how often have you been bothered by the following problems?”; 0 = “not at all” to 3 = “nearly every day”). According to the developers of the instrument, the PHQ-4 can either be used to calculate separate depression and anxiety scores, or to calculate a composite depression and anxiety index based on all 4 items (74). In the current study, we opted for the single factor solution since depression and anxiety are closely related constructs (74) and we wanted to avoid multicollinearity in the structural model.
Loneliness was measured using the 3-item short version [(75); e.g., “How often do you feel that you lack companionship?”; 1 = “never” to 4 = “often”; German items: (76)] of the revised UCLA Loneliness Scale (77).
The participant's level of stress was measured with the 4-item short version of the Perceived Stress Scale (PSS-4) by Cohen et al. (78). For the current study, we used the translated German items by Stächele and Volz (79) that were rated on a 5-point scale ranging from 1 = “never” to 5 = “very often”. Two positively worded items (e.g., “In the last month, how often have you felt confident about your ability to handle your personal problems?”) were recoded before inspecting Cronbach's alpha. A value of α = 0.557 indicated that the scale lacked reliability. Therefore, we decided to exclude the two recoded positive items, which increased Cronbach's alpha substantially to a satisfying level (see Table 1).
Physical Symptoms
Based on the Physical Health Questionnaire by Schat et al. (80), we created three items to measure how often the participants had experienced three widespread somatic symptoms. The participants were asked about their physical health conditions over the last month and had to indicate how often (1 = “never” to 5 = “very often”) they suffered from (a) sleep disturbances, (b) headaches, and (c) gastrointestinal problems (e.g., nausea, abdominal pain, or diarrhea).
Statistical Analysis
Descriptive statistics were calculated using IBM SPSS Statistics. The predicted structural model (Figure 1) was computed using R and the lavaan package (81). We inspected skewness and kurtosis for each variable and conducted Mardia's test of multivariate skewness und kurtosis (82) using the psych package (83). Because the data was not normally distributed, the hypothesized model was calculated using the robust MLR estimator, which features maximum likelihood estimation with scaled test statistics and Huber-White corrected standard errors. FIML-imputation was used to handle missing data (66 missing patterns). For the indirect effects, 95% confidence intervals based on the Monte Carlo approach (84) were calculated using the semTools package (85). Model fit was evaluated based on the recommendations of Hu and Bentler (86): A Comparative Fit Index (CFI) and a Tucker-Lewis Index (TLI) close to 0.95, a Standardized Root Mean Square Residual (SRMR) below 0.08, and a Root Mean Square Error of Approximation (RMSEA) below 0.06.
Results
To get an impression of the spread of SmUD in our sample, we calculated the prevalence of SmUD based on the cut-off point suggested by Pontes and Griffiths (66) and Hussain et al. (16). Thirty-five of the 874 participants that had completed all the questions of the screening tool reached SmUD scores of 36 points and above. This equals a rate of 4.0% potentially disordered smartphone users. Notably, we found that more males (n = 28, 6.2%) than females (n = 7, 1.7%) reached SmUD scores of 36 or above. This difference was significant, χ2(1) = 11.68, p < 0.001, Cramer's V = 0.116.
Structural Model
The estimated structural model is presented in Figure 2. SmUD severity, stress, depression/anxiety, loneliness, and physical symptoms were modeled as latent constructs based on manifest indicators (item scores), while age, gender (male = 0, female = 1) and smartphone minutes (per week) were added as observed variables. To control for the effects of age, gender, and smartphone minutes, additional direct paths from these variables to mental problems and physical symptoms were calculated. For a clearer illustration of the hypothesized relationships, the coefficients of these additional paths are not included in Figure 2, but can be found in Table 2. The calculated fit indices indicated a good model fit: χ2(227) = 707.48, p < 0.001, CFI = 0.951, TLI = 0.941, RMSEA = 0.053, SRMR = 0.037.
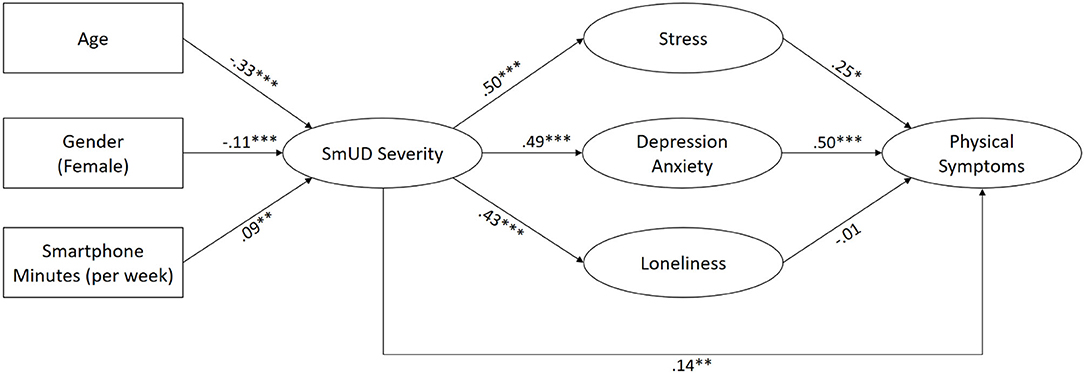
Figure 2. Estimated structural model labeled with standardized regression coefficients. ***p < 0.001, **p < 0.01, *p < 0.05. Direct paths from age, gender, and smartphone minutes to indicators of mental problems, and physical symptoms were estimated but are not shown for a clearer illustration (see Table 2 for a full matrix of all regression coefficients). SmUD, smartphone use disorder.
Hypotheses H1 and H2 were confirmed: Age (β = −0.33, p < 0.001) and smartphone minutes per week (β = 0.09, p < 0.01) were both significantly related to SmUD severity in the expected directions. Concerning gender (RQ1), we found a small significant association indicating that males experienced higher levels of SmUD than females (β = −0.11, p < 0.001).
Affirming hypotheses H3, H4, and H5, we identified significant positive relationships between SmUD severity and stress (β = 0.50, p < 0.001), depression and anxiety (β = 0.49, p < 0.001), and loneliness (β = 0.43, p < 0.001). Further, SmUD severity was positively related to experiencing physical symptoms (β = 0.14, p < 0.01), thus supporting hypothesis H6.
In line with hypotheses H7 and H8, we found that stress (β = 0.25, p < 0.05) as well as depression and anxiety (β = 0.50, p < 0.001) were positively related to physical symptoms. In contrast, hypothesis H9 was not supported since loneliness was not significantly related to physical symptoms (β = −0.01, p = 0.784).
Finally, we tested the indirect (mediated) effects postulated in hypotheses H10 to H12. H10 and H11 were both confirmed: SmUD showed a significant indirect relationship with physical symptoms via stress (B = 0.09 [LLCI = 0.02, ULCI = 0.17], β = 0.12, p < 0.05) and depression and anxiety (B = 0.19 [LLCI = 0.12, ULCI = 0.26], β = 0.25, p < 0.001). Hypothesis H12 had to be rejected since loneliness did not mediate the relationship between SmUD and physical symptoms (B = −0.00 [LLCI = −0.04, ULCI = 0.03], β = −0.01, p = 0.784). The total effect (including direct and indirect paths) of SmUD on physical symptoms was β = 0.50 (B = 0.39 [LLCI = 0.31, ULCI = 0.46], p < 0.001).
Discussion
While some previous studies have indicated prevalence rates of up to 30 to 40% of disordered (or at-risk) users in different populations [e.g., (7, 14, 32, 87)], our results indicate a relatively moderate rate of 4.0% potentially disordered users among German smartphone owners aged 14–64 years. This finding confirms the results of Hussain et al. (16), who used the same screening tool and also reported a relatively low rate of 2.7% disordered users in a (non-representative) sample of 13- to 69-year-old smartphone owners.
Using an adaptation of the IGDS9-SF [as one of the currently most-often used IGD scales; (71)] also makes it easier to compare our results with studies on other forms of media technology use disorders. For example, Reer et al. (72) used the same instrument and identified similar rates of disordered users among German gamers (2.4%) and social media users (2.7%). This raises the question of why IGD is defined in the manuals of the WHO and the APA, while SmUD or social media use disorder are not (even though they seem to be similarly prevalent). This is not an argument for or against the inclusion of these disorders in the relevant manuals, but it is certainly an observation of the unequal treatment of different forms of addictive media technology use.
The central aim of the current study was to examine antecedents (age, gender, smartphone minutes per week) and potential outcomes (mental problems, loneliness, physical symptoms) of SmUD severity.
Several studies have found that SmUD [e.g., (16, 36, 38)] and other forms of addictive media technology use [e.g., (88, 89)] are more prevalent among younger participants. Accordingly, we hypothesized a negative relationship between SmUD severity and age (H1), which was supported.
Concerning gender, studies have painted a heterogeneous picture, with some finding no significant differences [e.g., (40, 90)], some finding males [e.g., (14)], and some finding females [e.g., (39, 91)] to be more at risk of developing disordered forms of smartphone use. We asked the research question how SmUD is related to gender (RQ1) and identified a small significant negative relationship between female gender and SmUD, indicating that males scored higher on the screening tool. Furthermore, we identified a significantly higher proportion of male participants (6.2%) than female participants (1.7%) that reached scores above the cut-off point suggested by Pontes and Griffiths (66) and Hussain et al. (16).
We also examined how smartphone minutes per week were related to SmUD severity and hypothesized a positive association (H2). In line with previous studies [e.g., (16, 36, 41, 42)] and in support of our hypothesis, we found that those who use their smartphones more intensively showed more signs of SmUD. Notably, the strength of the association was smaller than one might have expected. This may be interpreted as a hint that intensified use of smartphones does not necessarily lead to SmUD. However, one should keep in mind that smartphone minutes per week were measured as self-reports. Thus, the small coefficient may also result from problems in objectively assessing screen time, an issue intensively discussed by researchers [e.g., (41, 42)].
Confirming previous findings [e.g., (8, 42, 45–48)] and our hypotheses (H3-H5), we found SmUD severity to be positively related to higher levels of stress, depression and anxiety, and loneliness. In our hypothesized model, we considered loneliness and mental problems consequences of SmUD. This assumption was based on recent longitudinal studies that indicated that SmUD can increase loneliness and mental problems over time (50, 51). However, we are aware that, because of the cross-sectional nature of our work, we cannot claim causality, that is, loneliness and mental problems could in general be causes or consequences of a higher SmUD tendency. Against the background of the I-PACE model (43, 44), both directions of effects are plausible. It can be argued that pre-existing psychosocial problems are predisposing factors for an unhealthy, escapist use of smartphones. However, in later phases of the addiction process, using smartphones in an excessive, disordered manner may also cause or further reinforce daily life problems, including emotional discomfort and loneliness (43). More longitudinal studies are necessary to further clarify the direction (or reciprocity) of these relationships.
The relationship between SmUD and physical health remained somewhat understudied so far (11). However, there is some empirical evidence that SmUD is associated with physical symptoms, such as sleep disturbances [e.g., (39, 46, 52, 53)]. Earlier studies have explained this relationship by possible direct effects of smartphone use on biological functioning [e.g., (92)]: Being permanently present, especially in the bedroom, smartphones may prevent users from sleeping, may disturb their biorhythms through screen light, and may induce a state of mental, emotional, and physiological arousal. Also, the effects of smartphone use on serum melatonin levels (which are responsible for sleep quality) have been discussed (93). In the current study, we considered three widespread physical symptoms (headaches, sleep disturbances, and gastrointestinal problems) and hypothesized a positive direct relationship with SmUD severity (H6), which was supported. This finding further underlines the assumption that SmUD may not only have a negative impact on psychosocial health, but could also lead to physical health impairments.
Based on studies that showed that loneliness, mental problems and physical symptoms often co-occur and can mutually affect each other [e.g., (56–64)], we hypothesized that stress (H7), depression and anxiety (H8), and loneliness (H9) were positively related to physical symptoms. Supporting hypotheses H7 and H8, we found that stress and depression and anxiety positively predicted physical symptoms. These findings can be explained by previous works that argued that conditions such as depression, anxiety, and stress make sleep lighter and more discontinuous [e.g., (58, 61)]. Furthermore, psychological distress can increase the level of muscle tension, which in turn can affect physical symptoms, such as headaches (50). Also, gastrointestinal problems like nausea and diarrhea have previously been reported to be positively related to mental problems, such as stress, depression, and anxiety [e.g., (57, 62)]. However, hypothesis H9 had to be rejected since loneliness showed no significant relationship with physical symptoms. This may (in parts) be explained by the results of a recent meta-analysis, showing that accounting for depression in multivariate analyses weakens the relationship between loneliness and sleep problems (63).
Further, we assumed that SmUD tendencies, physical symptoms, and psychosocial problems are not only directly related, but are also more complexly interwoven with each other. Research by Demirci et al. (39) and Liu et al. (65) indicated that SmUD can cause mental problems, which in turn can lead to sleep disturbances. Accordingly, we hypothesized that mental problems and loneliness mediate the relationship between SmUD tendencies and physical symptoms (H10-H12), which was confirmed for stress and depression/anxiety (but not for loneliness).
Taken together, the significant indirect associations we identified emphasize the necessity to not only consider direct relationships, but to also examine more complex models to further improve the understanding of SmUD and its potential outcomes. Notably, relationships between mental problems (e.g., depression and anxiety) and specific physical health impairments (e.g., migraine and sleep disturbances) were shown to be bidirectional [e.g., (52, 59, 94, 95)]. Thus, it may also be possible that physical symptoms resulting from SmUD contribute to the development or maintenance of negative mental states. An interesting task for future research could be to examine the interplay of SmUD, loneliness, mental problems, and physical symptoms based on a longitudinal study with several measurement points, allowing to also identify possible circular relationships.
Limitations
Our study is subject to some limitations. First, our results are based on cross-sectional data. The structural model was created against the background of existing studies and theoretical considerations. However, longitudinal studies are necessary to confirm our findings. Second, self-reported survey data (as used in the current study) always carries a certain risk of misjudgment of the own situation and behavior, and may be subject to social desirability. In general, we would like to emphasize the need to further improve the quality and validity of SmUD screening tools [also see (28)]. We think that Hussain et al.'s (16) approach to adapt an established scale that is based on the APA's IGD criteria is useful to improve the comparability between studies. However, it should be kept in mind that the IGD criteria were originally not designed to measure SmUD and that further evidence is needed to confirm their accuracy in measuring SmUD.
Conclusion
Our results confirm that increased SmUD severity is associated with several mental and physical health impairments. Further, the significant indirect paths we identified indicate that mental problems could play a crucial role in explaining the relationship between SmUD severity and physical symptoms (like sleep disturbances, gastrointestinal problems, and h eadaches). Against the background of the existing literature, we assume complex (and presumably circular) relationships between SmUD, mental health, and physical symptoms that should be further examined in future research. Unifying SmUD measurement criteria, improving the quality of screening tools, and conducting clinical studies, as well as more representative and longitudinal studies in different countries, are further important research tasks. From a practical perspective, our results underline the importance to follow a broad approach in prevention and intervention campaigns. To break the vicious cycle, such programs should not only focus on strategies to reduce screen time, but should also consider mental problems and physical symptoms that may have led to or may have resulted from SmUD.
Data Availability Statement
The datasets presented in this article are not readily available because they are part of a larger representative survey study that covers several different topics and is subject to further analysis in other contexts. However, the raw data supporting the conclusions of this article are available to qualified researchers, upon reasonable request. Requests to access the datasets should be directed to FR, ZmVsaXgucmVlciYjeDAwMDQwO3VuaS1tdWVuc3Rlci5kZQ==.
Ethics Statement
Ethical review and approval was not required for the study on human participants in accordance with the local legislation and institutional requirements. Written informed consent from the participants' legal guardian/next of kin was not required to participate in this study in accordance with the national legislation and the institutional requirements.
Author Contributions
FR and TQ conceptualized the study. FR administrated the project, conducted the statistical analyses, and wrote the manuscript. L-OW and RJ contributed to the statistical analyses and the writing of the manuscript. TQ obtained the funding, provided feedback on the manuscript, and supervised the project. All authors contributed to the article and approved the submitted version.
Funding
Parts of the research leading to these results have received funding from the Daimler and Benz Foundation via the project Internet und seelische Gesundheit (Internet and Mental Health). The sponsor had no role in the study design, the collection, analysis, interpretation of data, the writing of the report, or the decision to submit the article for publication.
Conflict of Interest
The authors declare that the research was conducted in the absence of any commercial or financial relationships that could be construed as a potential conflict of interest.
Publisher's Note
All claims expressed in this article are solely those of the authors and do not necessarily represent those of their affiliated organizations, or those of the publisher, the editors and the reviewers. Any product that may be evaluated in this article, or claim that may be made by its manufacturer, is not guaranteed or endorsed by the publisher.
Acknowledgments
The authors acknowledge support from the Open Access Publication Fund of the University of Münster, Germany.
References
1. Pew Research Center. Mobile fact sheet. Pew Research (2021). Available online at: https://www.pewresearch.org/internet/fact-sheet/mobile/ (accessed December 13, 2021).
2. Deloitte. Smartphone-Konsum am Limit? [Smartphone use at its limit?]. Deloitte (2020). Available online at: https://www2.deloitte.com/de/de/pages/technology-media-and-telecommunications/articles/smartphone-nutzung-2020.html (accessed December 13, 2021).
3. Felisoni DD, Godoi AS. Cell phone usage and academic performance: an experiment. Comput Educ. (2018) 117:175–87. doi: 10.1016/j.compedu.2017.10.006
4. Lepp A, Barkley JE, Karpinski AC. The relationship between cell phone use, academic performance, anxiety, and satisfaction with life in college students. Comput Hum Behav. (2014) 31:343–50. doi: 10.1016/j.chb.2013.10.049
5. Lepp A, Barkley JE, Karpinski AC. The relationship between cell phone use and academic performance in a sample of US college students. Sage Open. (2015) 5:1–9. doi: 10.1177/2158244015573169
6. Haga S, Sano A, Sekine Y, Sato H, Yamaguchi S, Masuda K. (2015). Effects of using a smart phone on pedestrians' attention and walking. Procedia Manufact. 3:2574–80. doi: 10.1016/j.promfg.2015.07.564
7. Kim HJ, Min JY, Kim HJ, Min KB. Accident risk associated with smartphone addiction: A study on university students in Korea. J Behav Addict. (2017) 6:699–707. doi: 10.1556/2006.6.2017.070
8. Elhai JD, Dvorak RD, Levine JC, Hall BJ. Problematic smartphone use: a conceptual overview and systematic review of relations with anxiety and depression psychopathology. J Affect Disord. (2017) 207:251–9. doi: 10.1016/j.jad.2016.08.030
9. Vahedi Z, Saiphoo A. The association between smartphone use, stress, and anxiety: a meta-analytic review. Stress Health. (2018) 34:347–58. doi: 10.1002/smi.2805
10. Yang J, Fu X, Liao X, Li Y. Association of problematic smartphone use with poor sleep quality, depression, and anxiety: a systematic review and meta-analysis. Psychiatry Res. (2020) 284:112686. doi: 10.1016/j.psychres.2019.112686
11. Busch PA, McCarthy S. Antecedents and consequences of problematic smartphone use: a systematic literature review of an emerging research area. Comput Hum Behav. (2021) 114:106414. doi: 10.1016/j.chb.2020.106414
12. Sohn SY, Rees P, Wildridge B, Kalk NJ, Carter B. Prevalence of problematic smartphone usage and associated mental health outcomes amongst children and young people: a systematic review, meta-analysis and GRADE of the evidence. BMC Psychiatry. (2019) 19:356. doi: 10.1186/s12888-019-2350-x
13. Mac Cárthaigh S, Griffin C, Perry J. The relationship between sleep and problematic smartphone use among adolescents: a systematic review. Dev Rev. (2020) 55:100897. doi: 10.1016/j.dr.2020.100897
14. Aljomaa SS, Al Qudah MF, Albursan IS, Bakhiet SF, Abduljabbar AS. Smartphone addiction among university students in the light of some variables. Comput Hum Behav. (2016) 61:155–64. doi: 10.1016/j.chb.2016.03.041
15. Kwon M, Lee J-Y, Won W-Y, Park J-W, Min J-A, Hahn C, et al. Development and validation of a Smartphone Addiction Scale (SAS). PLoS One. (2013) 8:e56936. doi: 10.1371/journal.pone.0056936
16. Hussain Z, Griffiths MD, Sheffield D. An investigation into problematic smartphone use: the role of narcissism, anxiety, and personality factors. J Behav Addict. (2017) 6:378–86. doi: 10.1556/2006.6.2017.052
17. Bae SM. The relationship between the type of smartphone use and smartphone dependence of Korean adolescents: national survey study. Child Youth Serv Rev. (2017) 81:207–11. doi: 10.1016/j.childyouth.2017.08.012
18. Toda M, Nishio N, Takeshita T. Predictive factors for smartphone dependence: relationship to demographic characteristics, chronotype, and depressive state of university students. Open J Prev Med. (2015) 5:456–62. doi: 10.4236/ojpm.2015.512051
19. Paschke K, Austermann MI, Thomasius R. ICD-11-based assessment of social media use disorder in adolescents: development and validation of the Social Media Use Disorder Scale for Adolescents (SOMEDIS-A). Front Psychiatry. (2021) 12:661483. doi: 10.3389/fpsyt.2021.661483
20. Tang WY, Reer F, Quandt T. The interplay of the Dark Triad and social media use motives to social media disorder. Pers Individ Dif. (2022) 187:111402. doi: 10.1016/j.paid.2021.111402
21. Sha P, Sariyska R, Riedl R, Lachmann B, Montag C. Linking internet communication and smartphone use disorder by taking a closer look at the Facebook and WhatsApp applications. Addict Behav Rep. (2019) 9:100148. doi: 10.1016/j.abrep.2018.100148
22. Zhang Y, Lv S, Li C, Xiong Y, Zhou C, Li X, Ye M. Smartphone use disorder and future time perspective of college students: The mediating role of depression and moderating role of mindfulness. Child Adolesc Psychiatry Ment Health. (2020) 14:3. doi: 10.1186/s13034-020-0309-9
23. Peng S, Zhou B, Wang X, Zhang H, Hu X. Does high teacher autonomy support reduce smartphone use disorder in Chinese adolescents? A moderated mediation model. Addict Behav. (2020) 105:106319. doi: 10.1016/j.addbeh.2020.106319
24. Montag C, Wegmann E, Sariyska R, Demetrovics Z, Brand M. How to overcome taxonomical problems in the study of Internet use disorders and what to do with “smartphone addiction”? J Behav Addict. (2020) 9:908–14. doi: 10.1556/2006.8.2019.59
25. Lin YH, Chang LR, Lee YH, Tseng HW, Kuo TB, Chen SH. Development and validation of the Smartphone Addiction Inventory (SPAI). PLoS One. (2014) 9:e98312. doi: 10.1371/journal.pone.0098312
26. Tossell C, Kortum P, Shepard C, Rahmati A, Zhong L. Exploring smartphone addiction: insights from long-term telemetric behavioral measures. Int J Interact Mobile Technol. (2015) 9:37–43. doi: 10.3991/ijim.v9i2.4300
27. De-Sola Gutiérrez J, de Fonseca FR, Rubio G. Cell-phone addiction: a review. Front Psychiatry. (2016) 7:175. doi: 10.3389/fpsyt.2016.00175
28. Harris B, Regan T, Schueler J, Fields SA. Problematic mobile phone and smartphone use scales: a systematic review. Front Psychology. (2020) 11:672. doi: 10.3389/fpsyg.2020.00672
29. Leung H, Pakpour AH, Strong C, Lin YC, Tsai MC, Griffiths MD, et al. Measurement invariance across young adults from Hong Kong and Taiwan among three internet-related addiction scales: Bergen social media addiction scale (BSMAS), smartphone application-based addiction scale (SABAS), and internet gaming disorder scale-short form (IGDS-SF9)(study Part A). Addict Behav. (2020) 101:105969. doi: 10.1016/j.addbeh.2019.04.027
30. Jeong SH, Kim H, Yum JY, Hwang Y. What type of content are smartphone users addicted to?: SNS vs. games. Comput Hum Behav. (2016) 54:10–7. doi: 10.1016/j.chb.2015.07.035
31. Liu CH, Lin SH, Pan YC, Lin YH. Smartphone gaming and frequent use pattern associated with smartphone addiction. Medicine. (2016) 95:e4068. doi: 10.1097/MD.0000000000004068
32. Cha S-S, Seo B-K. Smartphone use and smartphone addiction in middle school students in Korea: Prevalence, social networking service, and game use. Health Psychol Open. (2018) 5:1–15. doi: 10.1177/2055102918755046
33. van den Eijnden RJ, Lemmens JS, Valkenburg PM. The social media disorder scale. Comput Hum Behav. (2016) 61:478–87. doi: 10.1016/j.chb.2016.03.038
34. Mitchell L, Hussain Z. Predictors of problematic smartphone use: an examination of the integrative pathways model and the role of age, gender, impulsiveness, excessive reassurance seeking, extraversion, and depression. Behav Sci. (2018) 8:74. doi: 10.3390/bs8080074
35. Richardson M, Hussain Z, Griffiths MD. Problematic smartphone use, nature connectedness, and anxiety. J Behav Addict. (2018) 7:109–16. doi: 10.1556/2006.7.2018.10
36. Haug S, Castro RP, Kwon M, Filler A, Kowatsch T, Schaub MP. Smartphone use and smartphone addiction among young people in Switzerland. Journal of Behavioral Addictions. (2015) 4:299–307. doi: 10.1556/2006.4.2015.037
37. Nahas M, Hlais S, Saberian C, Antoun J. Problematic smartphone use among Lebanese adults aged 18–65 years using MPPUS-10. Comput Hum Behav. (2018) 87:348–53. doi: 10.1016/j.chb.2018.06.009
38. van Deursen AJ, Bolle CL, Hegner SM, Kommers PA. Modeling habitual and addictive smartphone behavior: the role of smartphone usage types, emotional intelligence, social stress, self-regulation, age, and gender. Comput Hum Behav. (2015) 45:411–20. doi: 10.1016/j.chb.2014.12.039
39. Demirci K, Akgönül M, Akpinar A. Relationship of smartphone use severity with sleep quality, depression, and anxiety in university students. J Behav Addict. (2015) 4:85–92. doi: 10.1556/2006.4.2015.010
40. Hawi NS, Samaha M. To excel or not to excel: strong evidence on the adverse effect of smartphone addiction on academic performance. Comput Educ. (2016) 98:81–9. doi: 10.1016/j.compedu.2016.03.007
41. Elhai JD, Tiamiyu MF, Weeks JW, Levine JC, Picard KJ, Hall BJ. Depression and emotion regulation predict objective smartphone use measured over one week. Pers Individ Dif. (2018) 133:21–8. doi: 10.1016/j.paid.2017.04.051
42. Rozgonjuk D, Levine JC, Hall BJ, Elhai JD. The association between problematic smartphone use, depression and anxiety symptom severity, and objectively measured smartphone use over one week. Comput Hum Behav. (2018) 87:10–7. doi: 10.1016/j.chb.2018.05.019
43. Brand M, Young KS, Laier C, Wölfling K, Potenza MN. Integrating psychological and neurobiological considerations regarding the development and maintenance of specific Internet-use disorders: an Interaction of Person-Affect-Cognition-Execution (I-PACE) model. Neurosci Biobehav Rev. (2016) 71:252–66. doi: 10.1016/j.neubiorev.2016.08.033
44. Brand M, Wegmann E, Stark R, Müller A, Wölfling K, Robbins TW, et al. The Interaction of Person-Affect-Cognition-Execution (I-PACE) model for addictive behaviors: update, generalization to addictive behaviors beyond internet-use disorders, and specification of the process character of addictive behaviors. Neurosci Biobehav Rev. (2019) 104:1–10. doi: 10.1016/j.neubiorev.2019.06.032
45. Bian M, Leung L. Linking loneliness, shyness, smartphone addiction symptoms, and patterns of smartphone use to social capital. Soc Sci Comput Rev. (2015) 33:61–79. doi: 10.1177/0894439314528779
46. Chen B, Liu F, Ding S, Ying X, Wang L, Wen Y. Gender differences in factors associated with smartphone addiction: a cross-sectional study among medical college students. BMC Psychiatry. (2017) 17:1–9. doi: 10.1186/s12888-017-1503-z
47. Chiu SI. The relationship between life stress and smartphone addiction on Taiwanese university student: a mediation model of learning self-efficacy and social self-efficacy. Comput Hum Behav. (2014) 34:49–57. doi: 10.1016/j.chb.2014.01.024
48. Enez Darcin A, Kose S, Noyan CO, Nurmedov S, Yilmaz O, Dilbaz N. Smartphone addiction and its relationship with social anxiety and loneliness. Behav Inf Technol. (2016) 35:520–5. doi: 10.1080/0144929X.2016.1158319
49. Kim S-G, Park J, Kim H-T, Pan Z, Lee Y, McIntyre RS. The relationship between smartphone addiction and symptoms of depression, anxiety, and attention-deficit/hyperactivity in South Korean adolescents. Ann Gen Psychiatry. (2019) 18:1–8. doi: 10.1186/s12991-019-0224-8
50. Chen IH, Pakpour AH, Leung H, Potenza MN, Su JA, Lin CY, et al. Comparing generalized and specific problematic smartphone/internet use: Longitudinal relationships between smartphone application-based addiction and social media addiction and psychological distress. J Behav Addict. (2020) 9:410–9. doi: 10.1556/2006.2020.00023
51. Lapierre MA, Zhao P, Custer BE. Short-term longitudinal relationships between smartphone use/dependency and psychological well-being among late adolescents. J Adolesc Health. (2019) 65:607–12. doi: 10.1016/j.jadohealth.2019.06.001
52. Chung JE, Choi SA, Kim KT, Yee J, Kim JH, Seong JW, et al. Smartphone addiction risk and daytime sleepiness in Korean adolescents. J Paediatr Child Health. (2018) 54:800–6. doi: 10.1111/jpc.13901
53. Zhang MX, Wu AM. Effects of smartphone addiction on sleep quality among Chinese university students: the mediating role of self-regulation and bedtime procrastination. Addict Behav. (2020) 111:106552. doi: 10.1016/j.addbeh.2020.106552
54. AlAbdulwahab SS, Kachanathu SJ, AlMotairi MS. Smartphone use addiction can cause neck disability. Musculoskelet Care. (2017) 15:10–2. doi: 10.1002/msc.1170
55. Demir Y, Sümer M. Effects of smartphone overuse on headache, sleep and quality of life in migraine patients. Neurosciences. (2019) 24:115–21. doi: 10.17712/nsj.2019.2.20180037
56. Breslau N, Schultz LR, Stewart WF, Lipton RB, Lucia VC, Welch KMA. Headache and major depression: is the association specific to migraine? Neurology. (2000) 54:308. doi: 10.1212/WNL.54.2.308
57. Haug TT, Mykletun A, Dahl AA. Are anxiety and depression related to gastrointestinal symptoms in the general population? Scand J Gastroenterol. (2002) 37:294–8. doi: 10.1080/003655202317284192
58. Jansson-Fröjmark M, Lindblom K. A bidirectional relationship between anxiety and depression, and insomnia? A prospective study in the general population. J Psychosom Res. (2008) 64:443–9. doi: 10.1016/j.jpsychores.2007.10.016
59. Morphy H, Dunn KM, Lewis M, Boardman HF, Croft PR. Epidemiology of insomnia: a longitudinal study in a UK population. Sleep. (2007) 30:274−80. doi: 10.1093/sleep/30.3.274
60. Martin PR. Stress and primary headache: review of the research and clinical management. Curr Pain Headache Rep. (2016) 20:45. doi: 10.1007/s11916-016-0576-6
61. Kalmbach DA, Anderson JR, Drake CL. The impact of stress on sleep: pathogenic sleep reactivity as a vulnerability to insomnia and circadian disorders. J Sleep Res. (2018) 27:e12710. doi: 10.1111/jsr.12710
62. Lee EY, Mun MS, Lee SH, Cho HSM. Perceived stress and gastrointestinal symptoms in nursing students in Korea: a cross-sectional survey. BMC Nurs. (2011) 10:22. doi: 10.1186/1472-6955-10-22
63. Griffin SC, Williams AB, Ravyts SG, Mladen SN, Rybarczyk BD. Loneliness and sleep: a systematic review and meta-analysis. Health Psychol Open. (2020) 7:2055102920913235. doi: 10.1177/2055102920913235
64. Stickley A, Koyanagi A, Koposov R, Blatný M, Hrdlička M, Schwab-Stone M, et al. Loneliness and its association with psychological and somatic health problems among Czech, Russian and US adolescents. BMC Psychiatry. (2016) 16:128. doi: 10.1186/s12888-016-0829-2
65. Liu Q-Q, Zhou Z-K, Yang X-J, Kong F-C, Niu G-F, Fan C-Y. Mobile phone addiction and sleep quality among Chinese adolescents: a moderated mediation model. Comput Hum Behav. (2017) 72:108–14. doi: 10.1016/j.chb.2017.02.042
66. Pontes HM, Griffiths MD. Measuring DSM-5 Internet gaming disorder: development and validation of a short psychometric scale. Comput Hum Behav. (2015) 45:137–43. doi: 10.1016/j.chb.2014.12.006
67. Evren C, Dalbudak E, Topcu M, Kutlu N, Evren B, Pontes HM. Psychometric validation of the Turkish nine-item Internet Gaming Disorder Scale–Short-Form (IGDS9-SF). Psychiatry Res. (2018) 265:349–54. doi: 10.1016/j.psychres.2018.05.002
68. Monacis L, de Palo V, Griffiths MD, Sinatra M. Validation of the Internet Gaming Disorder Scale–Short-Form (IGDS9-SF) in an Italian-speaking sample. J Behav Addict. (2016) 5:683–90. doi: 10.1556/2006.5.2016.083
69. Pontes HM, Griffiths MD. Portuguese validation of the internet gaming disorder scale–short-form. Cyberpsychol Behav Soc Network. (2016) 19:288–93. doi: 10.1089/cyber.2015.0605
70. Wu TY, Lin CY, Årestedt K, Griffiths MD, Broström A, Pakpour AH. Psychometric validation of the Persian nine-item internet gaming disorder scale–short-form: does gender and hours spent online gaming affect the interpretations of item descriptions? J Behav Addict. (2017) 6:256–63. doi: 10.1556/2006.6.2017.025
71. King DL, Chamberlain SR, Carragher N, Billieux J, Stein D, Mueller K, et al. Screening and assessment tools for gaming disorder: a comprehensive systematic review. Clin Psychol Rev. (2020) 77:101831. doi: 10.1016/j.cpr.2020.101831
72. Reer F, Festl R, Quandt T. Investigating problematic social media and game use in a nationally representative sample of adolescents and younger adults. Behav Inf Technol. (2021) 40:776–89. doi: 10.1080/0144929X.2020.1724333
73. Löwe B, Wahl I, Rose M, Spitzer C, Glaesmer H, Wingenfeld K, et al. A 4-item measure of depression and anxiety: validation and standardization of the Patient Health Questionnaire-4 (PHQ-4) in the general population. J Affect Disord. (2010) 122:86–95. doi: 10.1016/j.jad.2009.06.019
74. Kroenke K, Spitzer RL, Williams JB, Löwe B. An ultra-brief screening scale for anxiety and depression: the PHQ-4. Psychosomatics. (2009) 50:613–21. doi: 10.1016/S0033-3182(09)70864-3
75. Hughes ME, Waite LJ, Hawkley LC, Cacioppo JT. A short scale for measuring loneliness in large surveys: results from two population-based studies. Res Aging. (2004) 26:655–72. doi: 10.1177/0164027504268574
76. Reer F, Tang WY, Quandt T. Psychosocial well-being and social media engagement: the mediating roles of social comparison orientation and fear of missing out. New Media Soc. (2019) 21:1486–505. doi: 10.1177/1461444818823719
77. Russell D, Peplau LA, Cutrona CE. The revised UCLA loneliness scale: concurrent and discriminant validity evidence. J Pers Soc Psychol. (1980) 39:472–80. doi: 10.1037/0022-3514.39.3.472
78. Cohen S, Kamarck T, Mermelstein R. A global measure of perceived stress. J Health Soc Behav. (1983) 24:385–96. doi: 10.2307/2136404
80. Schat AC, Kelloway EK, Desmarais S. The Physical Health Questionnaire (PHQ): construct validation of a self-report scale of somatic symptoms. J Occup Health Psychol. (2005) 10:363–81. doi: 10.1037/1076-8998.10.4.363
81. Rosseel Y. lavaan: an R package for structural equation modeling and more. J Stat Softw. (2012) 48:1–36. doi: 10.18637/jss.v048.i02
82. Mardia KV. Measures of multivariate skewness and kurtosis with applications. Biometrika. (1970) 57:519–30. doi: 10.1093/biomet/57.3.519
83. Revelle W. Psych: Procedures for Psychological, Psychometric, Personality Research (2021). Available online at: https://cran.r-project.org/web/packages/psych/psych.pdf (accessed December 31, 2021).
84. MacKinnon DP, Lockwood CM, Williams J. Confidence limits for the indirect effect: distribution of the product and resampling methods. Multivariate Behav Res. (2004) 39:99–128. doi: 10.1207/s15327906mbr3901_4
85. Jorgensen TD, Pornprasertmanit S, Schoemann AM, Rosseel Y. semTools: Useful Tools for Structural Equation Modeling (2021). Availbale online at: https://cran.r-project.org/web/packages/semTools/semTools.pdf (accessed December 31, 2021).
86. Hu LT, Bentler PM. Cutoff criteria for fit indexes in covariance structure analysis: conventional criteria versus new alternatives. Struct Equation Model. (1999) 6:1–55. doi: 10.1080/10705519909540118
87. Samaha M, Hawi NS. Relationships among smartphone addiction, stress, academic performance, and satisfaction with life. Comput Hum Behav. (2016) 57:321–5. doi: 10.1016/j.chb.2015.12.045
88. Andreassen CS, Pallesen S, Griffiths MD. The relationship between addictive use of social media, narcissism, and self-esteem: findings from a large national survey. Addict Behav. (2017) 64:287–93. doi: 10.1016/j.addbeh.2016.03.006
89. Reer F, Quandt T. Games addiction: a comprehensive overview. In: J. Nussbaum (Editor). Oxford Research Encyclopedia of Communication. Oxford University Press (2021). doi: 10.1093/acrefore/9780190228613.013.1154
90. Duke É, Montag C. Smartphone addiction, daily interruptions and self-reported productivity. Addict Behav Rep. (2017) 6:90–5. doi: 10.1016/j.abrep.2017.07.002
91. Randler C, Wolfgang L, Matt K, Demirhan E, Horzum MB, Beşoluk S. Smartphone addiction proneness in relation to sleep and morningness–eveningness in German adolescents. J Behav Addict. (2016) 5:465–73. doi: 10.1556/2006.5.2016.056
92. Cain N, Gradisar M. Electronic media use and sleep in school-aged children and adolescents: a review. Sleep Med. (2010) 11: 735–42. doi: 10.1016/j.sleep.2010.02.006
93. Shrivastava A, Saxena Y. Effect of mobile usage on serum melatonin levels among medical students. Indian J Physiol Pharmacol. (2014) 58:395–9. Available online at: https://ijpp.com/IJPP%20archives/2014_58_4/395-399.pdf (accessed December 13, 2021).
94. Pompili M, di Cosimo D, Innamorati M, Lester D, Tatarelli R, Martelletti P. Psychiatric comorbidity in patients with chronic daily headache and migraine: a selective overview including personality traits and suicide risk. J Headache Pain. (2009) 10:283–90. doi: 10.1007/s10194-009-0134-2
Keywords: smartphone use, disorder, addiction, physical symptoms, depression, sleep disturbances, headaches, mental health
Citation: Reer F, Wehden L-O, Janzik R and Quandt T (2022) Examining the Interplay of Smartphone Use Disorder, Mental Health, and Physical Symptoms. Front. Public Health 10:834835. doi: 10.3389/fpubh.2022.834835
Received: 13 December 2021; Accepted: 28 February 2022;
Published: 14 April 2022.
Edited by:
Uichin Lee, KAIST, South KoreaReviewed by:
Rocco Servidio, University of Calabria, ItalyHanyi Lee, Hanyang University, South Korea
Copyright © 2022 Reer, Wehden, Janzik and Quandt. This is an open-access article distributed under the terms of the Creative Commons Attribution License (CC BY). The use, distribution or reproduction in other forums is permitted, provided the original author(s) and the copyright owner(s) are credited and that the original publication in this journal is cited, in accordance with accepted academic practice. No use, distribution or reproduction is permitted which does not comply with these terms.
*Correspondence: Felix Reer, ZmVsaXgucmVlciYjeDAwMDQwO3VuaS1tdWVuc3Rlci5kZQ==