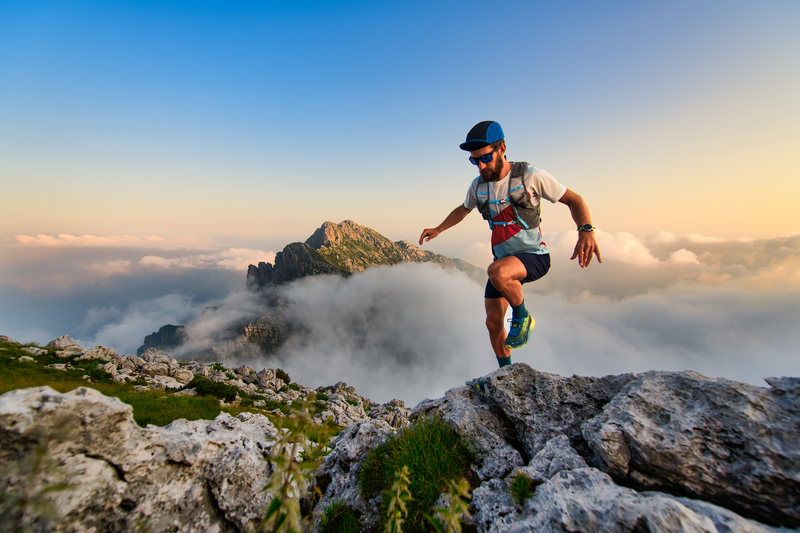
94% of researchers rate our articles as excellent or good
Learn more about the work of our research integrity team to safeguard the quality of each article we publish.
Find out more
ORIGINAL RESEARCH article
Front. Public Health , 07 July 2022
Sec. Aging and Public Health
Volume 10 - 2022 | https://doi.org/10.3389/fpubh.2022.824514
This article is part of the Research Topic Insights in Aging and Public Health: 2022 View all 21 articles
Objectives: Aim to evaluate the effect of social participation on utilization of medical services among middle-aged and elderly residents in China.
Methods: We used data from the 2018 wave of the China Health and Retirement Longitudinal Study. Social participation is classified into three types. Furthermore, to control for confounding factors, our study computed propensity score matching (PSM) to evaluate the effect of social participation on the utilization of medical services.
Result: The result of PSM indicates that social participation significantly positively affects the utilization of outpatient services, the average treatment effect on the treated (ATT = 0.038***) and the utilization of inpatient services (ATT = 0.015**) by middle-aged and elderly residents. Furthermore, the utilization of outpatient health care services was significantly positively associated with leisure activities (ATT = 0.035***), social activities to help others (ATT = 0.031***), and learning activities to gain new knowledge (ATT = 0.034***) among middle-aged and elderly residents. The utilization of inpatient health care was significantly positively associated with leisure activities (ATT = 0.015***) but had no significant association with social deeds that help others and increased new knowledge among middle-aged and elderly residents.
Conclusion: Thus, social participation significantly positively affects healthcare utilization by middle-aged and elderly residents. Hence, the government and society should provide more conveniences and promote social participation among middle-aged and elderly residents.
Population aging is a global problem, and China has the most rapidly aging population in the world (1). With the aging of the people in China, diseases among older adults, such as chronic non-communicable diseases and mental health disorders, have gained increasing attention (2), resulting in an emerging demand for health services by middle-aged and elderly residents (3).
The Andersen Health Services Utilization Initial Model, which includes predisposing, enabling, and need factors, is one of the most widespread research frameworks to indicate the influence of a healthcare utilization factor (4). Among the middle-aged and elderly residents, physical, and mental health had a negative connection with the utilization of medical services (5, 6), and social variables, such as regular involvement in activities of clubs, societies, and religious organizations, increase the odds of health service utilization (7).
Social participation was defined as participation in social activities, including engagement as volunteers and social groups interacting with the community (8). It is common knowledge that middle-aged and elderly residents have a relatively lower social participation rate. In addition, social participation is positively associated with improved overall health, which increases with age (9, 10). Besides providing better health, social participation improves the social bonds of middle-aged and elderly residents, and older people stay active by participating in voluntary work (11).
Although social participation is meaningful, few studies have examined its influence on the utilization of health services. Research reveals that the association between social participation and health services is complex. The results were affected by other factors, including health factors (12, 13). One study that used a nationally representative sample of older adults found that people with high social participation were significantly less likely to utilize health care services (visit their family doctor and district nurse last month). When controlling for demographic factors, physical and mental health, and physical activity, the longitudinal data indicated that social participation was positively associated with contacting home help services (13). Another researcher noted that social participation was significantly connected with low inpatient visits by controlling initial health variables (12).
The association between social participation and healthcare utilization due to confounding factors is unclear. Therefore, this study used the propensity score method (PSM) to control for confounding factors and explore the effect of social participation on the utilization of health care services among middle-aged and elderly residents. PSM is a statistical technique that can effectively reduce selection bias due to confounding variables between two different groups, such as the number of social participants and unsociable participants among middle-aged and elderly residents (14). PSM is widely used in social participation or medical service utilization. Yean Wang divided the middle-aged and elderly into two groups: internet users and non-internet users, and used the PSM method to analyze the connection between Internet use and levels of depression. The results show that older adults who reported internet use have lower depression levels than did those who did not use the internet, with adjustments made for Confounding factors (15). Jong-Yi Wang found that mortality and total medical expenditures per capita were significantly higher in psychiatric patients than in non-psychiatric patients by 1:1 dual propensity score matching (16).
The data from the 2018 wave of the China Health and Retirement Longitudinal Study (CHARLS) was a nationally representative longitudinal study conducted by the China Center for Economic Research (CCER), Peking University (17). The baseline survey of CHARLS was performed in 2011, and samples were surveyed every 2 years. Four national study waves of national study until 2018, analyzing 17,543 Chinese residents over 45 years old. Participants were selected from 450 villages and urban communities of 28 provinces in China, through probability-proportional-to-size sampling, using a multistage stratified sampling technique. They were interviewed through a high-quality questionnaire, which required detailed information about demographic backgrounds, health care and status, cognition, depression, work retirement, and property ownership (18).
The Biomedical Ethics Review Committee approved the CHARLS Study at Peking University in January 2011. All enrollees were notified, and informed consent was obtained before the interviews. We applied to the CHARLS team for data for this study and received anonymous enrollees (19).
To comply with the research purpose, the invalid samples from 2018 comprised 257 participants aged 45 years, and 2018 participants with missing variables were eliminated. The final sample consisted of 17,543 enrollees, including 9,328 enrollees who participated socially and 8,215 participants who did not. The effective sample rate was 88.5% (Figure 1).
Figure 1. Flowchart of the observation's selection and classification; CHARLS, (China Health Retirement Longitudinal Study).
To measure current social participation, we asked participants 10 multiple-choice questions about their involvement in the following activities, in the previous month: (1) interacting with friends; (2) playing Mahjong, playing chess, playing cards, or going to a community club; (3) providing help to family, friends, or neighbors who do not live with you; (4) went to the sport, social, or other kind of the club; (5) took part in a community-related organization; (6) voluntary or charity work; (7) cared for a sick or disabled adult who does not live with you; (8) attended an educational or training course; (9) stock investment; and (10) using the internet. If respondents answered at least one “yes” to the above questions, they were defined as “social participants.” The other respondents were defined as unsociable participants (20).
We classified social activities into three types to explore the associations between various categories of social participation and the utilization of medical services. The labels of training, 1, 2, 4, and 5, were regarded as leisure activities; the classifications of action, which were 3, 6, and 7, were considered as social activities to help others; the categorization of training, consisting of 8, 9, and 10, were regarded as learning activities to acquire new knowledge (21). Among 17,543 middle-aged and elderly residents, 7,797 (44.5%) enrollees engaged in leisure activities, 2,831(16.1%) partook in social activities to help others, and 2,286 (13.0%) joined learning activities for new knowledge.
This research included two types of services for medicine utilization: (1) outpatient visits: we asked participants whether they had visited a hospital, public health center, clinic, or were seen by health workers or doctors for outpatient care, last month; (2) inpatient visits: participants who had received inpatient care in the past year (22). The variables of outpatient and inpatient utilization was the classification variable (1 = yes, 0 = no).
According to the Andersen Health Services Utilization Initial Model framework, we classified the covariates that were comprehensively arranged and screened from the CHARLS questionnaire into three elements with some effect on the utilization of health services (23).
The parts included:(1) Propensity characteristics; age, gender, education level (no formal education, primary school, middle school, high school, and above), marriage (married and currently living with a spouse or others), household register (urban, rural), number of family members: (2) Capacity resources; income (household income in the past year), insurance (had at least one type of insurance or others), receive from child (financial support received from children, last year), provide to children (financial aid provided to children, last year): (3) Health needs; smoke (smoking currently, never smoked, stopped smoking), drink (drink alcohol now, never drank, stooped drinking), health status (perfect, good, poor, very poor), chronic disease (had at least one type of chronic illness or others), the activities of daily living (ADL) (the capacity of daily living activity, had 20 questions and a total of 80 points. The higher the score, the lower the ADL score). Covariables, including “income,” “receive from the child,” and “provide to the child” were natural logarithm transformed, as the original data were skewed to the right.
The t-test and Pearson chi-square test were used for the social participation survey to analyse the differences in demographic characteristics between the social and unsociable participation groups.
The primary statistical analysis method used was the propensity score matching (PSM) model computed through psmatch2 of Stata 15.0. The PSM model was a non-parametric statistical method to reduce the selective bias between treatment and control units (18, 24). The confounding factors were balanced, based on the propensity score by matching two groups, reflecting the selective probability of receiving treatment. The average treatment effect on the treated (ATT) was estimated after the PSM method reduced the influence of confounding variables in matched groups, which was the mean of the individual causal effect in the treated unit (25).
PSM analysis was classified into three steps. The first was calculating the propensity score according to the logistic model, including the abovementioned covariates. Second, the treatment and control groups were matched based on the propensity score, where matching methods varied in PSM, including the k-nearest neighbor matching method, radius matching method, and kernel matching method (15). Third, the ATT was evaluated. If the value of ATT is positive and statistically significant, it represents that social participation has increased the healthcare utilization rate. Suppose the value of ATT is negative and statistically significant. In that case, it means that social participation has decreased the health care utilization rate, such as ATT of social participation was 0.061 to inpatient visits, showing that the social participation had increased the inpatient visit rate within 1 year by approximately six percent points (26). The kernel matching method at a bandwidth of 0.06 was used, as frequently computed in the literature (27, 28). The k-nearest neighbor matching method (one to four), and the radius matching method (Caliper = 0.03) confirmed the robustness of the results.
The PSM method resulted in erroneous standard errors owing to the high correlation between matched samples and the error between the estimated propensity score and the actual value. The current approach is to substitute the standard empirical mistake with the empirical standard error. Our research used the bootstrap method to obtain the empirical standard error (15).
The data used in this study were derived from the CHARLS database, so patients and the public were not involved in the design or conduct of this research.
Samples regarding social activity were selected in two groups and descriptive analyses were conducted for the differences between the groups (t-test was computed for continuous variables, and chi-square test was computed for classification variables). For outcome variables, social participants were more likely to visit outpatients (p < 0.001), and unsociable participants were likelier to visit inpatients (p < 0.001). There were statistically significant social participation differences between the two groups of middle-aged and elderly residents on 11 covariates: middle-aged and older residents who were younger (p < 0.001), had a higher education level (p < 0.001), had a spouse (p < 0.001), provided for children more (p < 0.001), had higher income (p < 0.001), had insurance (p < 0.001), smoked (p < 0.001), drank (p < 0.001), had better health (p < 0.001), had lower ADL (p < 0.001), were urban residents (p < 0.001), had a higher incidence of social participation. This finding suggests that the effect of social participation on the utilization of medical services is complicated to calculate (Table 1).
Table 2 shows results for the overall balance t-test for 15 covariates. After kernel matching the propensity score, the final sample size in this analysis was 9,325 social participation enrollees and 8,199 unsociable participation enrollees. The bias of overall covariates was lower than 5% (mean bias = 1.7%, median bias = 1.4%) and no longer significant between the two groups (p = 0.294 > 0.05); the covariates of the two groups were significantly balanced, and PSM improved the comparability of social participation enrollees and unsociable participation enrollees.
Table 2. Overall balance t-test and the average treatment effect on the treated of social participation.
The ATT of the raw sample and the sample after kernel matching can be estimated. The estimates reveal that middle-aged and elderly residents who participated socially in the last month had significantly higher outpatient visit rates than those who had not attended socially in the previous month in both the unmatched sample and the kernel matched sample (ATT = 0.029***; 0.038***). Furthermore, the inpatient visits showed a higher rate in middle-aged and elderly residents who did not participate socially in the last month than those who had, before kernel matching (ATT = −0.023***). However, after kernel matching, the middle-aged and elderly residents who participated socially in the last month showed a higher inpatient visit rate than those enrollees who had not (ATT = 0.015**) (Table 2).
Table 3 describes the impact of diverse kinds of social participation on the utilization of medical services. The treatment group and control group's overall balance for each type displayed excellent results for covariate balances after kernel matching. The mean and median biases were jointly lower than 5% (mean bias = 1.1%; 1.9%; 1.7%, median bias = 0.7%; 1.5%; 1.1%), and no longer significant between the two groups in the three types of social participation (p = 0.910, 0.979, and 0.979, 0.05).
Table 3. Overall balance t-test and propensity score matched results and effect of each type of social participation.
Among middle-aged and elderly residents, social participation (leisure activities, social activities helping others, and learning activities) significantly improved the outpatient visit rate in both raw samples and matched samples after kernel matching (ATT = 0.035***; 0.031***; 0.034***). For inpatient visits, all types of social participation had a significantly negative role before matching (ATT = −0.012**; −0.033***; −0.054***), and after kernel matching, the results changed. The inpatient visit rates were positively and significantly different between the treatment unit and control unit for leisure activities (ATT = 0.015***), and there was no significant difference between the two groups in the two other types of social participation (ATT = 0.002, 0.003). Table 4 showed the combined effect of different types of social participation on utilization of medical services. Compared with those who did not participate in any social participation, participants who attended one type of social participation (any one of leisure activities, social activities helping others, or learning activities) had a significantly higher outpatient visit rate and inpatient visit rate after kernel matching (ATT = 0.029***, ATT = 0.014**). The effect was even more significant in the participants who attended two or three types of social participation (ATT = 0.057***, ATT = 0.017**).
Table 4. Overall balance t-test and propensity score matched results and combined effect of each type of social participation.
Our research compared the results of the kernel matching method with radius and k-nearest neighbor matching methods (one to four) and Logistic regression to explore the robustness of the kernel matching method in propensity score matching. Table 5 indicates that the significance of the average treatment effect for the matching methods was the same. The propensity score-matching results were robust.
Table 5. The average treatment effect of propensity score matching among three matching methods and logistic regression.
To the best of our knowledge, this is the first study that used PSM to assess the association between social participation and healthcare utilization. Our research explored the association between social participation and healthcare utilization by PSM among residents aged 45 years and above based on the 2018 CHARLS statistics. By adjustment for 15 confounding variates through PSM, this study demonstrated a significant positive effect of social participation on healthcare service utilization by middle-aged and elderly residents. All the treatment groups; leisure activities, social helping activities, and learning activities, had a higher rate of outpatient visits than their corresponding control groups. Moreover, leisure activities enrollees had a healthy level of inpatient visits in contrast with the team that had not.
In China, there were about 249.49 million residents aged 60 and over at the end of 2018 (29). Severe population aging is a burden on health care utilization; health is a significant relevant intermediary element; and unmet health care needs is deleterious for older adults (30, 31). The association between health care utilization and unmet health care needs was remarkably negative, and the promoted utilization of medical services met health care needs (32).
We found that older men had less social participation than younger men, and the rate of residents over 45 years who participated socially was 53.17%. The incident odds of social participation in prior studies paralleled those in our study. The number of residents participating socially was not high (33, 34). In contrast, the elderly in developed countries usually had high social participation rates (29).
Moreover, regardless of outpatient and inpatient services, residents with greater social participation, utilized health care services more. There are many possible reasons for this finding. First, social activities improved relationships with relatives and friends, which confirmed the optimism associated with the utilization of medical services and preventive health care (35, 36). Improving relationships with friends and relatives may reduce the psychological pressure and financial support of middle-aged and older adults facing medical treatment. Second, previous research shows that socially isolated older adults used health care services less, and there was a significant association between loneliness and lower odds of physician visits, social isolation and loneliness will lead to the weakening of mobility and the obstruction of social communication in middle-aged and older adults (37, 38). Social participation reduces social isolation and helps the elderly to integrate socially. Furthermore, our findings revealed that social participation had a negative connection to the incidence of inpatient visits before matching, similar to previous research that indicates that higher social participation meant lower odds of inpatient visits (12). However, the result changed after matching the factors, possibly because of the covariates for health needs, which most significantly affected the utilization of medical services in the Andersen model.
In Previous researches, the relationship between social participation and medical service utilization was unclear. It was often mixed with other factors, such as health factors. Since health factors were a relatively crucial confounding factor, the opposite result may be obtained after the balance of health factors (12, 13). The same pattern was present in our study, social participants had higher health status, and those with solid health had lower healthcare utilization, utilization of medical services will increase with the balance of health-related variables (23, 39). If the elderly healthy balance to the same level to participate in social activities of the high degree of the elderly, the possibility of medical service utilization will increase. The reasons for the changes in the results before and after the propensity score matching was balancing confounding factors and reducing the influence of confounding factors. The PSM method eliminated the effect of covariates, including health need factors and evaluated the natural result of the connection between social participation and health care utilization.
Our study showed a significantly positive association between diverse types of social participation and outpatient service utilization, and that social participation for leisure, affects inpatient service utilization. Various kinds of social participation revealed diverse characteristics and functions of health care utilization. Leisure activities, such as interacting with friends and playing Mahjong, could enhance relatives' and friends' relationships and relax older adults (40, 41). Social activities to help others, such as voluntary or charity work, generates social integration and fulfillment among older people (21, 42). Learning activities, such as internet use, improved communication and social connections across geographic distances at comparably low consumption (43), and could provide new information about medical services for the elderly. Enhancing the social participation of older adults was a critical factor in successful aging that many older adults value. The government and society should increase the convenience of and promote social participation by providing public spaces to encourage increased social participation in middle-aged and elderly residents.
There are some limitations to our study. First, the 11 covariates in this study may not cover all essential confounding factors for medical service utilization and social participation among middle-aged and elderly residents. Unobservable covariates increase selection bias, influencing PSM results between the treatment and control groups. Second, our study has one indicator that determines social participation in the last month; instead the frequency of current social participation could be examined. The study may ignore the effects of different degrees of social participation on health service utilization. These limitations deserve further scrutiny.
Our research indicated that residents in middle-age and at a later life stage in China who participated socially, had a significantly higher incidence of utilizing health services than those who did not participate socially. Diverse social activities positively affected outpatient service utilization, and leisure activities were positively associated with inpatient service utilization. Considering our conclusion, the government and society should increase the convenience of and promote social participation by providing public spaces to encourage increased social participation in middle-aged and elderly residents.
The datasets presented in this study can be found in online repositories. The names of the repository/repositories and accession number(s) can be found below: (http://charls.pku.edu.cn) China Health and Retirement Longitudinal Study.
The studies involving human participants were reviewed and approved by the Biomedical Ethics Review Committee. The patients/participants provided their written informed consent to participate in this study.
T-YL, TC, and D-CQ contributed to the development of the research ideas and obtained the dataset. T-YL cleaned the dataset. T-YL and TC performed data analyses and drafted the manuscript. All authors commented on the manuscript. All authors contributed to the article and approved the submitted version.
This study was supported by the National Natural Science Foundation of China (Grant Number: 71503090).
The authors declare that the research was conducted in the absence of any commercial or financial relationships that could be construed as a potential conflict of interest.
All claims expressed in this article are solely those of the authors and do not necessarily represent those of their affiliated organizations, or those of the publisher, the editors and the reviewers. Any product that may be evaluated in this article, or claim that may be made by its manufacturer, is not guaranteed or endorsed by the publisher.
The authors thank the staff and participants of the China Health and Retirement Longitudinal Study (CHARLS) team for providing the data.
1. Fang EF, Scheibye-Knudsen M, Jahn HJ, Li J, Ling L, Guo H, et al. A research agenda for aging in China in the 21st century. Ageing Res Rev. (2015) 24:197–205. doi: 10.1016/j.arr.2015.08.003
2. Gong CH, Kendig H, He X. Factors predicting health services use among older people in China: an analysis of the China health and retirement longitudinal study 2013. BMC Health Serv Res. (2016) 16:63. doi: 10.1186/s12913-016-1307-8
3. Ye L, Shia BC, Fang Y, Lee TS. Heterogeneous health profiles and healthcare utilization of the middle-aged and elderly with multiple health insurance schemes in China. Public Health. (2019) 170:61–9. doi: 10.1016/j.puhe.2019.01.011
4. Evashwick C, Rowe G, Diehr P, Branch L. Factors explaining the use of health care services by the elderly. Health Serv Res. (1984) 19:357–82.
5. Liu YB, Hou P, Xue HP, Mao XE, Li YN. Social support, health literacy, and health care utilization among older adults. Asia Pac J Public Health. (2019) 31:359–66. doi: 10.1177/1010539519851674
6. Gerst-Emerson K, Jayawardhana J. Loneliness as a public health issue: the impact of loneliness on health care utilization among older adults. Am J Public Health. (2015) 105:1013–9. doi: 10.2105/AJPH.2014.302427
7. Willie-Tyndale D, McKoy Davis J, Holder-Nevins D, Mitchell-Fearon K, James K, Waldron NK, et al. Predictors of health service utilization among older men in Jamaica. J Gerontol B Psychol Sci Soc Sci. (2019) 74:999–1006. doi: 10.1093/geronb/gbx168
8. Sun J, Lyu S. Social participation and urban-rural disparity in mental health among older adults in China. J Affect Disord. (2020) 274:399–404. doi: 10.1016/j.jad.2020.05.091
9. Ang S. Social participation and health over the adult life course: does the association strengthen with age? Soc Sci Med. (2018) 206:51–9. doi: 10.1016/j.socscimed.2018.03.042
10. van Hees SGM, van den Borne BHP, Menting J, Sattoe JNT. Patterns of social participation among older adults with disabilities and the relationship with well-being: a latent class analysis. Arch Gerontol Geriatr. (2020) 86:103933. doi: 10.1016/j.archger.2019.103933
11. Lin W. A study on the factors influencing the community participation of older adults in China: based on the CHARLS2011 data set. Health Soc Care Commun. (2017) 25:1160–8. doi: 10.1111/hsc.12415
12. Newall N, McArthur J, Menec VH. A longitudinal examination of social participation, loneliness, and use of physician and hospital services. J Aging Health. (2015) 27:500–18. doi: 10.1177/0898264314552420
13. Bath PA, Gardiner A. Social engagement and health and social care use and medication use among older people. Eur J Ageing. (2005) 2:56–63. doi: 10.1007/s10433-005-0022-9
14. Guo S, Fraser MW. Propensity Score Analysis: Statistical Methods and Applications. Thousand Oaks, CA: SAGE publications (2014).
15. Wang Y, Zhang H, Feng T, Wang H. Does internet use affect levels of depression among older adults in China? A propensity score matching approach. BMC Public Health. (2019) 19:1474. doi: 10.1186/s12889-019-7832-8
16. Wang JY, Chang CC, Lee MC, Li YJ. Identification of psychiatric patients with high mortality and low medical utilization: a population-based propensity score-matched analysis. BMC Health Serv Res. (2020) 20:230. doi: 10.1186/s12913-020-05089-6
17. Zhao Y, Atun R, Oldenburg B, McPake B, Tang S, Mercer SW, et al. Physical multimorbidity, health service use, and catastrophic health expenditure by socioeconomic groups in China: an analysis of population-based panel data. Lancet Glob Health. (2020) 8:e840–9. doi: 10.1016/S2214-109X(20)30127-3
18. Li C, Zhou R, Yao N, Cornwell T, Wang S. Health care utilization and unmet needs in Chinese older adults with multimorbidity and functional impairment. J Am Med Dir Assoc. (2020) 21:806–10. doi: 10.1016/j.jamda.2020.02.010
19. Qiu D, Chen T, Liu T, Song F. Smoking cessation and related factors in middle-aged and older Chinese adults: evidence from a longitudinal study. PLoS ONE. (2020) 15:e0240806. doi: 10.1371/journal.pone.0240806
20. Glei DA, Landau DA, Goldman N, Chuang YL, Rodríguez G, Weinstein M. Participating in social activities helps preserve cognitive function: an analysis of a longitudinal, population-based study of the elderly. Int J Epidemiol. (2005) 34:864–71. doi: 10.1093/ije/dyi049
21. Zhou S, Song S, Jin Y, Zheng ZJ. Prospective association between social engagement and cognitive impairment among middle-aged and older adults: evidence from the China health and retirement longitudinal study. BMJ Open. (2020) 10:e040936. doi: 10.1136/bmjopen-2020-040936
22. Han J, Zhang X, Meng Y. Out-patient service and in-patient service: the impact of health insurance on the healthcare utilization of mid-aged and older residents in urban China. Risk Manag Healthc Policy. (2020) 13:2199–212. doi: 10.2147/RMHP.S273098
23. Andersen RM. Revisiting the behavioral model and access to medical care: does it matter? J Health Soc Behav. (1995) 36:1–10. doi: 10.2307/2137284
24. van der Wielen N, Channon AA, Falkingham J. Does insurance enrolment increase healthcare utilisation among rural-dwelling older adults? Evidence from the national health insurance scheme in Ghana. BMJ Glob Health. (2018) 3:e000590. doi: 10.1136/bmjgh-2017-000590
25. Abdia Y, Kulasekera KB, Datta S, Boakye M, Kong M. Propensity scores based methods for estimating average treatment effect and average treatment effect among treated: a comparative study. Biom J. (2017) 59:967–85. doi: 10.1002/bimj.201600094
26. Xian W, Xu X, Li J, Sun J, Fu H, Wu S, et al. Health care inequality under different medical insurance schemes in a socioeconomically underdeveloped region of China: a propensity score matching analysis. BMC Public Health. (2019) 19:1373. doi: 10.1186/s12889-019-7761-6
27. Sun J, Lyu S. Does health insurance lead to improvement of health status among Chinese rural adults? Evidence from the China family panel studies. Int J Health Serv. (2020) 50:350–9. doi: 10.1177/0020731420914824
28. Jonk Y, Lawson K, O'Connor H, Riise KS, Eisenberg D, Dowd B, et al. How effective is health coaching in reducing health services expenditures? Med Care. (2015) 53:133–40. doi: 10.1097/MLR.0000000000000287
29. Feng Z, Cramm JM, Nieboer AP. Social participation is an important health behaviour for health and quality of life among chronically ill older Chinese people. BMC Geriatr. (2020) 20:299. doi: 10.1186/s12877-020-01713-6
30. Li J, Chen X, Han X, Zhang G. Spatiotemporal matching between medical resources and population ageing in China from 2008 to 2017. BMC Public Health. (2020) 20:845. doi: 10.1186/s12889-020-08976-z
31. Zhu H. Unmet needs in long-term care and their associated factors among the oldest old in China. BMC Geriatr. (2015) 15:46. doi: 10.1186/s12877-015-0045-9
32. Bataineh H, Devlin RA, Barham V. Unmet health care and health care utilization. Health Econ. (2019) 28:529–42. doi: 10.1002/hec.3862
33. Zhou Y, Chen Z, Shaw I, Wu X, Liao S, Qi L, et al. Association between social participation and cognitive function among middle- and old-aged Chinese: a fixed-effects analysis. J Glob Health. (2020) 10:020801. doi: 10.7189/jogh.10.020801
34. Shang Q. Social support, rural/urban residence, and depressive symptoms among Chinese adults. J Community Psychol. (2020) 48:849–61. doi: 10.1002/jcop.22302
35. Bremer D, Inhestern L, von dem Knesebeck O. Social relationships and physician utilization among older adults-A systematic review. PLoS ONE. (2017) 12:e0185672. doi: 10.1371/journal.pone.0185672
36. Stafford M, von Wagner C, Perman S, Taylor J, Kuh D, Sheringham J. Social connectedness and engagement in preventive health services: an analysis of data from a prospective cohort study. Lancet Public Health. (2018) 3:e438–46. doi: 10.1016/S2468-2667(18)30141-5
37. Mitsutake S, Koike T, Ishizaki T, Sakurai R, Yasunaga M, Nishi M, et al. Influence of co-existing social isolation and homebound status on medical care utilization and expenditure among older adults in Japan. Arch Gerontol Geriatr. (2020) 93:104286. doi: 10.1016/j.archger.2020.104286
38. Lim KK, Chan A. Association of loneliness and healthcare utilization among older adults in Singapore. Geriatr Gerontol Int. (2017) 17:1789–98. doi: 10.1111/ggi.12962
39. Denkinger MD, Lukas A, Herbolsheimer F, Peter R, Nikolaus T. Physical activity and other health-related factors predict health care utilisation in older adults: the ActiFE Ulm study. Z Gerontol Geriatr. (2012) 45:290–7. doi: 10.1007/s00391-012-0335-1
40. Lee Y, Chi I, Palinkas LA. Retirement, leisure activity engagement, and cognition among older adults in the United States. J Aging Health. (2019) 31:1212–34. doi: 10.1177/0898264318767030
41. Ihle A, Grotz C, Adam S, Oris M, Fagot D, Gabriel R, et al. The association of timing of retirement with cognitive performance in old age: the role of leisure activities after retirement. Int Psychogeriatr. (2016) 28:1659–69. doi: 10.1017/S1041610216000958
42. Kim ES, Konrath SH. Volunteering is prospectively associated with health care use among older adults. Soc Sci Med. (2016) 149:122–9. doi: 10.1016/j.socscimed.2015.11.043
Keywords: social participation, utilization of medical services, propensity score matching, elderly residents, middle-aged residents
Citation: Liu T-Y, Qiu D-C and Chen T (2022) Effects of Social Participation by Middle-Aged and Elderly Residents on the Utilization of Medical Services: Evidence From China. Front. Public Health 10:824514. doi: 10.3389/fpubh.2022.824514
Received: 29 November 2021; Accepted: 20 June 2022;
Published: 07 July 2022.
Edited by:
Chih-Da Wu, National Cheng Kung University, TaiwanReviewed by:
Yanbing Zeng, Capital Medical University, ChinaCopyright © 2022 Liu, Qiu and Chen. This is an open-access article distributed under the terms of the Creative Commons Attribution License (CC BY). The use, distribution or reproduction in other forums is permitted, provided the original author(s) and the copyright owner(s) are credited and that the original publication in this journal is cited, in accordance with accepted academic practice. No use, distribution or reproduction is permitted which does not comply with these terms.
*Correspondence: Ting Chen, Y2hlbnQ0MUB3dXN0LmVkdS5jbg==
Disclaimer: All claims expressed in this article are solely those of the authors and do not necessarily represent those of their affiliated organizations, or those of the publisher, the editors and the reviewers. Any product that may be evaluated in this article or claim that may be made by its manufacturer is not guaranteed or endorsed by the publisher.
Research integrity at Frontiers
Learn more about the work of our research integrity team to safeguard the quality of each article we publish.