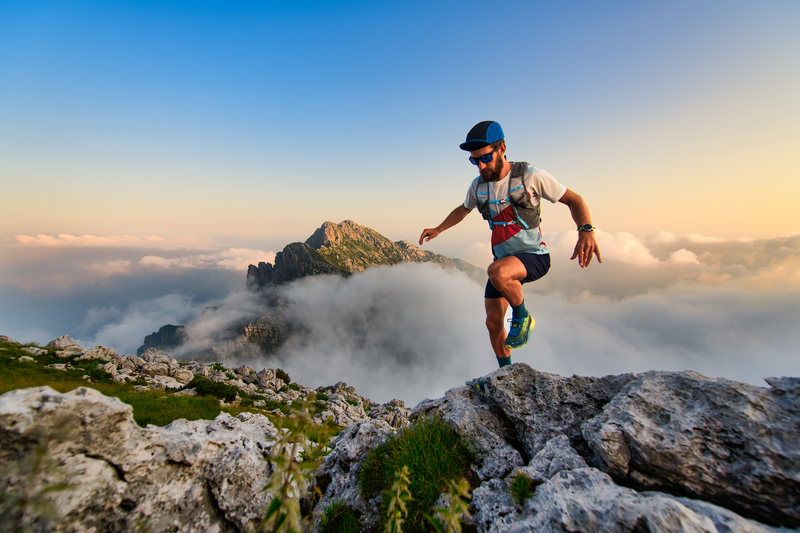
95% of researchers rate our articles as excellent or good
Learn more about the work of our research integrity team to safeguard the quality of each article we publish.
Find out more
ORIGINAL RESEARCH article
Front. Public Health , 24 February 2022
Sec. Digital Public Health
Volume 10 - 2022 | https://doi.org/10.3389/fpubh.2022.822808
This article is part of the Research Topic Machine Learning in Disease Screening, Diagnosis, and Surveillance View all 20 articles
Background: Renal cell carcinoma (RCC) is one of the most common cancers in middle-aged patients. We aimed to establish a new nomogram for predicting cancer-specific survival (CSS) in middle-aged patients with non-metastatic renal cell carcinoma (nmRCC).
Methods: The clinicopathological information of all patients from 2010 to 2018 was downloaded from the SEER database. These patients were randomly assigned to the training set (70%) and validation set (30%). Univariate and multivariate COX regression analyses were used to identify independent risk factors for CSS in middle-aged patients with nmRCC in the training set. Based on these independent risk factors, a new nomogram was constructed to predict 1-, 3-, and 5-year CSS in middle-aged patients with nmRCC. Then, we used the consistency index (C-index), calibration curve, and area under receiver operating curve (AUC) to validate the accuracy and discrimination of the model. Decision curve analysis (DCA) was used to validate the clinical application value of the model.
Results: A total of 27,073 patients were included in the study. These patients were randomly divided into a training set (N = 18,990) and a validation set (N = 8,083). In the training set, univariate and multivariate Cox regression analysis indicated that age, sex, histological tumor grade, T stage, tumor size, and surgical method are independent risk factors for CSS of patients. A new nomogram was constructed to predict patients' 1-, 3-, and 5-year CSS. The C-index of the training set and validation set were 0.818 (95% CI: 0.802-0.834) and 0.802 (95% CI: 0.777-0.827), respectively. The 1 -, 3 -, and 5-year AUC for the training and validation set ranged from 77.7 to 80.0. The calibration curves of the training set and the validation set indicated that the predicted value is highly consistent with the actual observation value, indicating that the model has good accuracy. DCA also suggested that the model has potential clinical application value.
Conclusion: We found that independent risk factors for CSS in middle-aged patients with nmRCC were age, sex, histological tumor grade, T stage, tumor size, and surgery. We have constructed a new nomogram to predict the CSS of middle-aged patients with nmRCC. This model has good accuracy and reliability and can assist doctors and patients in clinical decision making.
In recent years, renal cell carcinoma (RCC) incidence has gradually increased, accounting for 2-3% of adult malignant tumors (1). The incidence of RCC in the United States is about 9.1 per 100,000, and the mortality rate is 3.5 per 100,000 (2). It has been reported in the literature that 15% of patients with RCC diagnosed for the first time have developed distant metastases, and another 10-20% of patients with localized RCC eventually develop metastatic RCC (3, 4). The incidence of RCC in men is higher than that in women, about 1.65:1 (5, 6). In 2016, there were 6,700 new diagnoses of RCC in the United States, and 14,240 patients died of renal cancer (7). The prognosis of nmRCC is good, but the 5-year survival rate of metastatic RCC is about 10%, and the median survival time is only 10 months (8). A comprehensive treatment method based on surgery is advocated for localized RCC (9). However, 20-30% of patients with localized RCC still relapse after surgery (10). Therefore, evaluation of the progression, metastasis and prognosis of RCC is critical in clinical management.
At present, studies have shown that clinicopathological factors such as age, sex, and tumor size are related to the prognosis of RCC (11, 12). Guo et al. found that the right RCC has a better prognosis than the left (13). Wang et al. constructed a nomogram to predict the survival of RCC patients with bone metastases and found that age, sex, marriage, tumor histology grade, T stage, N stage, surgery, and radiotherapy are independent risks factors for patients (14). Li et al. developed a nomogram to predict the risk of distant metastasis in patients with RCC (15). Yue et al. found that age is a critical factor in the prognosis of patients with metastatic RCC; elderly patients have a worse prognosis than younger patients (16).
At present, artificial intelligence has been widely used in the medical field. Awais et al. (17) use texture analysis to classify abnormal areas of the mouth and promote the development of oral cancer treatment. Mishra et al. (18) use intelligent drive for multistage assessment of mental disorders to help patients with mental illness. Although various kinds of nomograms have been widely used in clinical practice, the accuracy and specificity of these nomgorams are very worrying. We aimed to establish a specific nomgogram to predict survival in middle-aged patients with renal cell carcinoma. This study used big data based on the Cox regression model to construct a simple nomogram, which is as convenient as possible for users to operate under the premise of ensuring accuracy.
RCC has become significant cancer endangering the health of the population. Accurate prediction of the survival of cancer patients is the key to improving the survival time and quality of life of patients with RCC. At present, using big medical data to establish a prediction model has become an essential means to predict the survival of cancer patients. The nomogram is a user-friendly graphical digital model that can accurately predict the occurrence of a given event based on the numerical estimation of multiple single variables (19). Middle-aged patients with RCC have a good prognosis without distant metastasis. However, accurate prognostic assessment can answer patient consultations and help doctors and patients make clinical decisions. Therefore, we aim to establish a nomogram to predict the CSS of middle-aged patients with nmRCC.
We downloaded the clinical-pathological data of the patients from the National Cancer Institute's Surveillance, Epidemiology, and Final Results (SEER) project, including patients who were diagnosed with nmRCC in the United States from 2010 to 2018 between 40 and 60 years old. The data of this study can be obtained from the SEER database (http://seer.cancer.gov/). The SEER database is a public database that contains 18 cancer registries and covers ~28% of the American population (20). Patient information can be obtained on the database, including demographic information, tumor characteristics, and survival status. Because the data we used is publicly available, and the patient's personal information is not identifiable, our study did not require ethical approval and informed consent. Our research method complies with the rules of the SEER database.
The patient's demographic information and clinical-pathological data include age, sex, race, year of diagnosis, marriage, tumor laterality, tumor histological type, histological grade, T stage, type of surgery, radiotherapy chemotherapy, and survival time. Inclusion criteria: (1) age 40-60 years; (2) pathological diagnosis of renal cell carcinoma (ICD-O-3 codes 8260, 8310, 8312, 8317); (3) diagnosis year 2010-2018. Exclusion criteria: (1) unknown race; (2) unknown tumor size; (3) unknown surgical method; (4) unknown T stage; (5) survival time <1 month; (6) unknown cause of death. The flow chart of patient screening is shown in Figure 1.
The year of diagnosis was divided into 2010-2014 and 2015-2018. The race included white, black, and other races (American Indian/AK Native, Asian/Pacific Islander). Tumor grades have grade I (highly differentiated), grade II (moderately differentiated), grade III (poorly differentiated), and grade IV (undifferentiated). The pathological types of RCC include renal clear cell carcinoma, renal papillary adenocarcinoma, renal chromophobe cell carcinoma, and unclassified renal cell carcinoma. According to the SEER operation code, the operation was divided into local tumor excision (code 10-27), partial nephrectomy (PN, code 30) and radical nephrectomy (RN, code 40-80).
The patients were randomly divided into a training set (70%) and a validation set (30%). In the training set, univariate and multivariate Cox regression models were used to analyze independent risk factors for survival of nmRCC patients, and the hazard ratio (HR) and 95% confidence interval (CI) were recorded.
The identified independent risk factors were used to construct a nomogram to predict 1-, 3-, and 5-year CSS in nmRCC patients. All independent risk factors were imported into the nomogram based on the Cox regression model. The risk weights of various variables and the degree of risk are accurately displayed in the nomogram.
The calibration curve was used to test the accuracy of the prediction model, and we used 1,000 bootstrap samples for internal validation. The 1-, 3-, and 5-year areas under the receiver operating curve (AUC) of the training set and the validation set were used to test the accuracy and discrimination of the prediction model. Similarly, we used the consistency index (C-index) to test the discriminative power of the model.
Decision curve analysis (DCA) is a new calculation method that estimates the net benefits under various risk thresholds to evaluate the clinical value of the model (21). DCA was used to assess the clinical application value of the nomogram and compare it with T staging. In addition, according to the nomogram score, patients were divided into a high-risk group and a low-risk group. Kaplan-Meier curve and log-rank test were used to compare the survival differences of patients in different groups.
The count data was described by frequency (%), and the chi-square test and non-parametric you test were used to compare groups. Measurement data (age, tumor size) were expressed using mean and standard deviation, and a non-parametric test (U-test) was used for differences between groups. The Cox regression model was used to analyze the risk factors of patient survival, and the Kaplan-Meier curve and log-rank test were used to compare the survival differences of patients between groups. All statistical analysis uses SPSS 26.0 and R software 4.1.0. P-value <0.05 was considered to be statistically different.
According to the inclusion and exclusion criteria, a total of 27,073 patients were included in the study. These patients were randomly divided into a training set (N = 18,990) and a validation set (N = 8,083). Table 1 shows the clinicopathological characteristics of all patients. The average age of the patients was 52.4 years, 20,993 patients were white (77.5%), 17,531 patients were male (64.8%), and 16,015 patients were married (59.2%). There were 14,784 (54.6%) patients diagnosed in 2010-2014. Patients with tumor grades I, II, III, and IV was 2,747 (10.1%), 12,412 (45.8%), 6,048 (22.3%), and 928 (3.43%), respectively. 14,571 (53.8%) tumors with T1a stage, 17,534 (64.8%) with the histopathological type of renal clear cell carcinoma, and the average tumor diameter were 46.0 mm. Most patients underwent surgery, 11,750 (43.4%) patients underwent PN, and 13,120 (48.5%) patients underwent RN. Most of the patients did not receive radiotherapy and chemotherapy, 26,702 (98.6%) patients did not receive chemotherapy, and 27,015 (99.8%) patients did not receive radiotherapy. There was no significant difference between the clinical-pathological information of the patients in the training set and the validation set.
All variables were included in univariate Cox regression analysis to screen out survival-related variables. We found that age (HR 1.05, 95%CI 1.03-1.06, p < 0.001), sex (HR 0.7, 95%CI 0.59-0.82, p < 0.001), tumor histological grade (HR 1.41, 95%CI 1.34-1.49, p < 0.001), T stage (HR 2.55, 95%CI 2.35-2.75, p < 0.001), tumor size (HR 1.01, 95%CI 1.01-1.01, p < 0.001), and surgery (HR 1.23, 95%CI 1.1-1.38, p < 0.001) were related to survival prognosis. These factors were included in the multivariate cox regression analysis and showed that all variables were independent prognostic risk factors (Table 2). In other words, these risk factors can be used as factors predicting CSS in patients with nmRCC.
Based on the independent risk factors screened out by univariate and multivariate Cox regression analysis, we constructed a new nomogram to predict the 1-year, 3-year, and 5-year CSS of middle-aged patients with nmRCC (Figure 2). The nomogram showed that tumor size and T stage are the most significant factors affecting the patient's CSS, followed by surgery, histological tumor grade, and the final age and sex have little effect on the survival and prognosis of patients.
The calibration curve showed that the 1-, 3-, and 5-year predicted values are highly consistent with the actual observed values in the training set, and the validation set are highly compatible with the existing experimental values, suggesting that our model has good accuracy (Figures 3A-F). The C-index in the training set and the validation set were 0.818 (95% CI: 0.802-0.834) and 0.802 (95% CI: 0.777-0.827), respectively, indicating that our prediction model has good discrimination. In the training set, the AUCs of the models that predict patients' 1-, 3-, and 5-year CSS are 0.796, 0.80, and 0.792, respectively (Figure 4A). In the validation set, the AUCs of the models that predict the patient's 1-, 3-, and 5-year CSS are 0.781, 0.795, and 0.777, respectively (Figure 4B). It also proved that the predictive model has good discrimination.
Figure 3. Calibration curves of the nomogram. (A–C) For 1-, 3-, and 5-year CSS in the training set; (D– F) For 1-, 3-, and 5-year CSS in the validation set.
DCA suggested that the nomogram has a better clinical application value in the training and validation set, and it is significantly better than T staging (Figures 5A,B). In addition, we had developed a risk stratification system. According to the score of each patient on the nomogram, all patients were divided into a low-risk group (total score ≤ 72.3) and a high-risk group (total score > 72.3).
Figure 5. DCA of the nomogram predicting CSS in the training set (A) and validation set (B). The Y-axis represents net income, and the X-axis represents threshold probability. The green line means no patients died, and the dark green line means all patients died. When the threshold probability is between 0 and 50%, the net benefit of the model exceeds all deaths or none.
According to the Kaplan-Meier curve, the high-risk group's 1-, 3-, and 5-year CSS rates were 97.2, 91.5, and 87.2%, respectively. The low-risk group's 1-, 3-, and 5-year CSS rates were 99.7, 98.7, and 97.8%. There was a significant difference in survival between the high-risk group and the low-risk patients in the training and validation set (Figure 6), indicating that our predictive model can accurately identify high-risk patients. In addition, we compared the survival differences of surgical methods in patients with different risk groups. We found that patients with surgery in the low-risk group had a higher survival rate than patients without surgery, including PN, RN, and local tumor excision (Figure 7A). However, although most patients chose RN in the high-risk group, patients with PN and local tumor excision have a higher survival rate than RN (Figure 7B).
Figure 6. Kaplan–Meier curves of CSS for patients in the low- and high-risk groups in the training set (A) and validation set (B).
Figure 7. Comparison of different surgical methods of Kaplan–Meier curves in the Low-risk group (A) and High-risk group (B).
Based on the nomogram we constructed, we developed a web application to predict the CSS of middle-aged patients with nmRCC. Visit https://xiudanpan.shinyapps.io/DynNomapp/ to enter the website. Enter the patient's clinical characteristics, and we can obtain the CSS of the patient at each time.
RCC is a common tumor of the urinary system in the world, the incidence of women ranks ninth, and the incidence of men ranks seventh (22, 23). Although surgical treatment, immunotherapy, targeted therapy, and other RCC treatment methods are developing rapidly. However, due to the widespread local recurrence, distant metastasis, and drug tolerance of RCC, the prognosis of RCC patients is not very optimistic (24). To improve RCC patients' prognosis and quality of life, more and more renal cancer surgery risk scoring standards and renal cancer prognostic risk stratification have been established (25–27). The prognosis of early RCC is relatively good. The study reported that early asymptomatic RCC prediction is significantly better than that of symptomatic RCC (28). With improved health awareness and the popularization of health examinations, symptomatic kidney cancer is rare, and patients with advanced kidney cancer are more common. The proportion of early asymptomatic kidney cancer is gradually increasing, with reports ranging from 46.2 to 61% (29). It may be because of the recent increase in abdominal imaging, which is the main reason for the early diagnosis of asymptomatic kidney cancer (30). One study found that frequent use of CT for abdominal scans is associated with the risk of nephrectomy (31).
This study focused on middle-aged nmRCC patients and established a prognostic nomogram for predicting the CSS of middle-aged nmRCC patients for the first time. Because middle-aged patients' remaining lives with nmRCC are still very long, an accurate prognosis can help patients improve their survival rate and quality of life. Our constructed nomogram can accurately predict patients' 1-, 3-, 5-year CSS. According to the univariate and multivariate analysis of patients, age, sex, histological grade, T stage, surgery, and tumor size are independent risk factors.
Similar to other studies, our results also found that age is a critical factor in the prognosis of patients, even in middle-aged patients (32). Because the increase of age will bring about the weakening of the immune system, further causing the deterioration of the tumor and reducing the survival time of the patient (33). In our study, men have a higher incidence of kidney cancer and a higher mortality rate. Sex as a prognostic factor of patients may be related to hormone levels in the body, such as androgens and testosterone can cause specific cancers (34, 35).
Previous studies have found that tumor characteristics are also critical factors for patient survival, such as histological tumor grade, T stage, N stage, and distant metastasis (36). Our study found that tumor size and histological tumor grade are independent risk factors for patient prognosis. The histological grade is related to the stemness of the tumor. Previous studies have found that high-grade tumors are related to bladder cancer and prostate cancer (37, 38). Because high-grade tumors are often highly malignant and aggressive tumors. In addition, tumor size is also associated with the patient's prognosis. The larger the tumor, the higher the risk of metastasis and invasion.
The TNM staging system is a standard staging system for all tumors. It is mainly determined by postoperative pathological results and clinical staging (39). According to the patient's tumor condition (T), lymph node (N), distant metastasis (M), the cancer is divided into different stages. Indeed, TNM staging is related to the patient's prognosis. The higher the stage, the worse the patient's prognosis. For nmRCC, there is no lymph node and distant metastasis, and only T staging can reflect the staging of the tumor. Our study found that the T stage is the most critical factor affecting the prognosis of patients. The higher the T stage, the worse the patient's prognosis. This also proved that T staging should be used as an essential component of the nomogram.
Tumor treatment mode is also an important prognostic factor for patients with RCC. Surgery, as the essential treatment method, is the most critical factor for the prognosis of renal cancer patients (40). The nomogram showed that patients with PN have the best prognosis, while those without surgery have the worst prognosis. Our risk stratification system suggested that most patients in the low-risk group choose PN and have a high survival rate. For high-risk patients, most patients choose RN. Although patients with RN and local tumor excision have a higher survival rate, this may be caused by selection bias. Because of more extensive and higher T-stage tumors, doctors and patients are more inclined to choose RN. And these patients will have worse outcomes.
This study used the identifiable variables in the SEER database to construct predictions of 1-, 3-, and 5-year CSS in middle-aged patients with nmRCC. The model has good accuracy and discrimination. The calibration curve of the nomogram indicated that the prediction accuracy of the prediction model is very high. The C-index and AUC of the nomogram are about 0.8, which stated that the discriminative accuracy of the prediction model is about 80% and proved that the model is reliable. This nomogram can predict the prognosis of middle-aged patients with nmRCC and provide a reliable basis for personalized treatment and monitoring.
This study used the identifiable variables in the SEER database to construct predictions of 1-, 3-, and 5-year CSS in middle-aged patients with nmRCC. The model has good accuracy and discrimination. The calibration curve of the nomogram indicated that the prediction accuracy of the prediction model is very high. The C-index and AUC of the nomogram are about 0.8, which stated that the discriminative accuracy of the prediction model is about 80% and proved that the model is reliable. This nomogram can predict the prognosis of middle-aged patients with nmRCC and provide a reliable basis for personalized treatment and monitoring.
This study also has some limitations. First of all, we did not include some possible clinical factors, such as BMI, smoking, drinking, hypertension, genetic markers, etc. But we had included important clinical-pathological information, such as tumor stage, surgery and other vital factors, so our results will not be too biased. Secondly, our study was a retrospective cases study, and there may be some deviations that are difficult to adjust. Further prospective studies are necessary to validate our prediction model. Finally, we only used the data in the SEER database for internal validation, and the subsequent external proof is needed to validate the model's accuracy.
We found that independent risk factors for CSS in middle-aged patients with nmRCC were age, sex, histological tumor grade, T stage, tumor size, and surgery. We have constructed a new nomogram to predict the CSS of patients. This model has good accuracy and reliability and can assist doctors and patients in clinical decision making.
Publicly available datasets were analyzed in this study. This data can be found at: https://seer.Cancer.gov/.
The data of this study is obtained from the SEER database. The patients' data is public and anonymous, so this study does not require ethical approval and informed consent.
JW, BZ, XL, and JT contributed to the conception and design. JW, BZ, and JT collected and analyzed the data. JW, BZ, XP, and JT drew the figures and tables. JT, XL, XP, and JW wrote the draft. JT, BZ, and XP contributed to manuscript writing and revision. All authors approved the final manuscript.
The authors declare that the research was conducted in the absence of any commercial or financial relationships that could be construed as a potential conflict of interest.
All claims expressed in this article are solely those of the authors and do not necessarily represent those of their affiliated organizations, or those of the publisher, the editors and the reviewers. Any product that may be evaluated in this article, or claim that may be made by its manufacturer, is not guaranteed or endorsed by the publisher.
1. Siegel RL, Miller KD, Jemal A. Cancer statistics, 2019. CA Cancer J Clin. (2019) 69:7-34. doi: 10.3322/caac.21551
2. Pantuck AJ, Zisman A, Belldegrun AS. The changing natural history of renal cell carcinoma. J Urol. (2001) 166:1611-23. doi: 10.1016/S0022-5347(05)65640-6
3. Janzen NK, Kim HL, Figlin RA, Belldegrun AS. Surveillance after radical or partial nephrectomy for localized renal cell carcinoma and management of recurrent disease. Urol Clin North Am. (2003) 30:843-52. doi: 10.1016/S0094-0143(03)00056-9
4. Schwaab T, Schwarzer A, Wolf B, Crocenzi TS, Seigne JD, Crosby NA, et al. Clinical and immunologic effects of intranodal autologous tumor lysate-dendritic cell vaccine with Aldesleukin (Interleukin 2) and IFN-{alpha}2a therapy in metastatic renal cell carcinoma patients. Clin Cancer Res. (2009) 15:4986-92. doi: 10.1158/1078-0432.CCR-08-3240
5. Woldrich JM, Mallin K, Ritchey J, Carroll PR, Kane CJ. Sex differences in renal cell cancer presentation and survival: an analysis of the National Cancer Database, 1993-2004. J Urol. (2008) 179:1709-13. doi: 10.1016/j.juro.2008.01.024
6. Bergström A, Hsieh CC, Lindblad P, Lu CM, Cook NR, Wolk A. Obesity and renal cell cancer–a quantitative review. Br J Cancer. (2001) 85:984-90. doi: 10.1054/bjoc.2001.2040
7. Siegel RL, Miller KD, Jemal A. Cancer statistics, 2016. CA Cancer J Clin. (2016) 66:7-30. doi: 10.3322/caac.21332
8. De Meerleer G, Khoo V, Escudier B, Joniau S, Bossi A, Ost P, et al. Radiotherapy for renal-cell carcinoma. Lancet Oncol. (2014) 15:e170-7. doi: 10.1016/S1470-2045(13)70569-2
9. Frank I, Blute ML, Cheville JC, Lohse CM, Weaver AL, Zincke H. An outcome prediction model for patients with clear cell renal cell carcinoma treated with radical nephrectomy based on tumor stage, size, grade and necrosis: the SSIGN score. J Urol. (2002) 168:2395-400. doi: 10.1016/S0022-5347(05)64153-5
10. Cho HJ, Kim SJ, Ha US, Hong SH, Kim JC, Choi YJ, et al. Prognostic value of capsular invasion for localized clear-cell renal cell carcinoma. Eur Urol. (2009) 56:1006-12. doi: 10.1016/j.eururo.2008.11.031
11. Wu J, Zhang P, Zhang G, Wang H, Gu W, Dai B, et al. Renal cell carcinoma histological subtype distribution differs by age, gender, and tumor size in coastal Chinese patients. Oncotarget. (2017) 8:71797-804. doi: 10.18632/oncotarget.17894
12. Jung EJ, Lee HJ, Kwak C, Ku JH, Moon KC. Young age is independent prognostic factor for cancer-specific survival of low-stage clear cell renal cell carcinoma. Urology. (2009) 73:137-41. doi: 10.1016/j.urology.2008.08.460
13. Guo S, Yao K, He X, Wu S, Ye Y, Chen J, et al. Prognostic significance of laterality in renal cell carcinoma: a population-based study from the surveillance, epidemiology, and end results (SEER) database. Cancer Med. (2019) 8:5629-37. doi: 10.1002/cam4.2484
14. Wang K, Wu Z, Wang G, Shi H, Xie J, Yin L, et al. Survival nomogram for patients with bone metastatic renal cell carcinoma: a population-based study. Int Braz J Urol. (2021) 47:333-49. doi: 10.1590/s1677-5538.ibju.2020.0195
15. Li Y, Chen P, Chen Z. A population-based study to predict distant metastasis in patients with renal cell carcinoma. Ann Palliat Med. (2021) 10:4273-88. doi: 10.21037/apm-20-2481
16. Yue G, Deyu L, Lianyuan T, Fengmin S, Mei G, Yajun H, et al. Clinical features and prognostic factors of patients with metastatic renal cell carcinoma stratified by age. Aging. (2021) 13:8290-305. doi: 10.18632/aging.202637
17. Awais M, Ghayvat H, Krishnan Pandarathodiyil A, Nabillah Ghani WM, Ramanathan A, Pandya S, et al. Healthcare professional in the loop (HPIL): classification of standard and oral cancer-causing anomalous regions of oral cavity using textural analysis technique in autofluorescence imaging. Sensors. (2020) 20:5780. doi: 10.3390/s20205780
18. Mishra S, Tripathy HK, Kumar Thakkar H, Garg D, Kotecha K, Pandya S. An explainable intelligence driven query prioritization using balanced decision tree approach for multi-level psychological disorders assessment. Front Public Health. (2021) 9:795007. doi: 10.3389/fpubh.2021.795007
19. Yan Y, Liu H, Mao K, Zhang M, Zhou Q, Yu W, et al. Novel nomograms to predict lymph node metastasis and liver metastasis in patients with early colon carcinoma. J Transl Med. (2019) 17:193. doi: 10.1186/s12967-019-1940-1
20. Cronin KA, Ries LA, Edwards BK. The surveillance, epidemiology, and end results (SEER) program of the National Cancer Institute. Cancer. (2014) 120(Suppl 23):3755-7. doi: 10.1002/cncr.29049
21. Vickers AJ, Cronin AM, Elkin EB, Gonen M. Extensions to decision curve analysis, a novel method for evaluating diagnostic tests, prediction models and molecular markers. BMC Med Inform Decis Mak. (2008) 8:53. doi: 10.1186/1472-6947-8-53
22. Rini BI, Campbell SC, Escudier B. Renal cell carcinoma. Lancet. (2009) 373:1119-32. doi: 10.1016/S0140-6736(09)60229-4
23. Scelo G, Larose TL. Epidemiology and risk factors for kidney cancer. J Clin Oncol. (2018) 36:JCO2018791905. doi: 10.1200/JCO.2018.79.1905
24. Siegel RL, Miller KD, Fuchs HE, Jemal A. Cancer statistics, 2021 [published correction appears in CA Cancer J Clin. (2021) 71:359]. CA Cancer J Clin. (2021) 71:7-33. doi: 10.3322/caac.21654
25. Beisland C, Guð*brandsdottir G, Reisæter LA, Bostad L, Hjelle KM. A prospective risk-stratified follow-up programme for radically treated renal cell carcinoma patients: evaluation after eight years of clinical use. World J Urol. (2016) 34:1087-99. doi: 10.1007/s00345-016-1796-4
26. Waldert M, Klatte T. Nephrometry scoring systems for surgical decision-making in nephron-sparing surgery. Curr Opin Urol. (2014) 24:437-40. doi: 10.1097/MOU.0000000000000085
27. Park DS, Hwang JH, Kang MH, Oh JJ. Association between RENAL nephrometry score and perioperative outcomes following open partial nephrectomy under cold ischemia [published correction appears in Can Urol Assoc J. (2014) Mar-Apr;8:84] [published correction appears in Can Urol Assoc J. (2014) 8:84]. Can Urol Assoc J. (2014) 8:E137-41. doi: 10.5489/cuaj.1372
28. Patard JJ, Leray E, Cindolo L, Ficarra V, Rodriguez A, De La Taille A, et al. Multi-institutional validation of a symptom based classification for renal cell carcinoma. J Urol. (2004) 172:858-62. doi: 10.1097/01.ju.0000135837.64840.55
29. Jayson M, Sanders H. Increased incidence of serendipitously discovered renal cell carcinoma. Urology. (1998) 51:203-5. doi: 10.1016/S0090-4295(97)00506-2
30. Maehara CK, Silverman SG, Lacson R, Khorasani R. Journal club: renal masses detected at abdominal CT: radiologists' adherence to guidelines regarding management recommendations and communication of critical results. AJR Am J Roentgenol. (2014) 203:828-34. doi: 10.2214/AJR.13.11497
31. Welch HG, Skinner JS, Schroeck FR, Zhou W, Black WC. Regional variation of computed tomographic imaging in the United States and the risk of nephrectomy. JAMA Intern Med. (2018) 178:221-7. doi: 10.1001/jamainternmed.2017.7508
32. Mao W, Zhang Z, Huang X, Fan J, Geng J. Marital status and survival in patients with penile cancer. J Cancer. (2019) 10:2661-9. doi: 10.7150/jca.32037
33. Zeng C, Wen W, Morgans AK, Pao W, Shu XO, Zheng W. Disparities by race, age, and sex in the improvement of survival for major cancers: results from the National Cancer Institute Surveillance, epidemiology, and end results (SEER) program in the United States, 1990 to 2010. JAMA Oncol. (2015) 1:88-96. doi: 10.1001/jamaoncol.2014.161
34. Huang X, Shu C, Chen L, Yao B. Impact of sex, body mass index and initial pathologic diagnosis age on the incidence and prognosis of different types of cancer. Oncol Rep. (2018) 40:1359-69. doi: 10.3892/or.2018.6529
35. Key T, Appleby P, Barnes I, Reeves G, Endogenous Hormones and Breast Cancer Collaborative Group. Endogenous sex hormones and breast cancer in postmenopausal women: reanalysis of nine prospective studies. J Natl Cancer Inst. (2002) 94:606-16. doi: 10.1093/jnci/94.8.606
36. Zeng Y, Mayne N, Yang CJ, D'Amico TA, Ng CSH, Liu CC, et al. A nomogram for predicting cancer-specific survival of TNM 8th edition stage I non-small-cell lung cancer. Ann Surg Oncol. (2019) 26:2053-62. doi: 10.1245/s10434-019-07318-7
37. Abdel-Rahman O. Bladder cancer mortality after a diagnosis of nonmuscle-invasive bladder carcinoma. Future Oncol. (2019) 15:2267-75. doi: 10.2217/fon-2018-0861
38. Liauw SL, Ham SA, Das LC, Rudra S, Packiam VT, Koshy M, et al. Prostate cancer outcomes following solid-organ transplantation: a SEER-medicare analysis. J Natl Cancer Inst. (2020) 112:847-54. doi: 10.1093/jnci/djz221
39. Amin MB, Greene FL, Edge SB, Compton CC, Gershenwald JE, Brookland RK, et al. The eighth edition AJCC cancer staging manual: continuing to build a bridge from a population-based to a more “personalized” approach to cancer staging. CA Cancer J Clin. (2017) 67:93-9. doi: 10.3322/caac.21388
Keywords: nomogram, middle-aged patients, nmRCC, cancer-specific survival, SEER, online application
Citation: Tang J, Wang J, Pan X, Liu X and Zhao B (2022) A Web-Based Prediction Model for Cancer-Specific Survival of Middle-Aged Patients With Non-metastatic Renal Cell Carcinoma: A Population-Based Study. Front. Public Health 10:822808. doi: 10.3389/fpubh.2022.822808
Received: 26 November 2021; Accepted: 17 January 2022;
Published: 24 February 2022.
Edited by:
Yi-Ju Tseng, National Central University, TaiwanReviewed by:
Sharnil Pandya, Symbiosis International University, IndiaCopyright © 2022 Tang, Wang, Pan, Liu and Zhao. This is an open-access article distributed under the terms of the Creative Commons Attribution License (CC BY). The use, distribution or reproduction in other forums is permitted, provided the original author(s) and the copyright owner(s) are credited and that the original publication in this journal is cited, in accordance with accepted academic practice. No use, distribution or reproduction is permitted which does not comply with these terms.
*Correspondence: Binyi Zhao, enE3MjAxMjhAMTYzLmNvbQ==
†These authors have contributed equally to this work and share first authorship
Disclaimer: All claims expressed in this article are solely those of the authors and do not necessarily represent those of their affiliated organizations, or those of the publisher, the editors and the reviewers. Any product that may be evaluated in this article or claim that may be made by its manufacturer is not guaranteed or endorsed by the publisher.
Research integrity at Frontiers
Learn more about the work of our research integrity team to safeguard the quality of each article we publish.