- 1Health Economics Research Group, Menzies Institute for Medical Research, University of Tasmania, Hobart, TAS, Australia
- 2Department of Pharmacology, Thai Nguyen University of Medicine and Pharmacy, Thai Nguyen University, Thai Nguyen, Vietnam
- 3Tasmanian Data Linkage Unit, Menzies Institute for Medical Research, University of Tasmania, Hobart, TAS, Australia
- 4Centre for Health Policy, School of Population and Global Health, The University of Melbourne, Melbourne, VIC, Australia
Aims: Our study aimed to identify the common themes, knowledge gaps and to evaluate the quality of data linkage research on diabetes in Australia.
Methods: This systematic review was developed in accordance with the Preferred Reporting Items for Systematic Reviews and Meta-Analyses (the PRISMA Statement). Six biomedical databases and the Australian Population Health Research Network (PHRN) website were searched. A narrative synthesis was conducted to comprehensively identify the common themes and knowledge gaps. The guidelines for studies involving data linkage were used to appraise methodological quality of included studies.
Results: After screening and hand-searching, 118 studies were included in the final analysis. Data linkage publications confirmed negative health outcomes in people with diabetes, reported risk factors for diabetes and its complications, and found an inverse association between primary care use and hospitalization. Linked data were used to validate data sources and diabetes instruments. There were limited publications investigating healthcare expenditure and adverse drug reactions (ADRs) in people with diabetes. Regarding methodological assessment, important information about the linkage performed was under-reported in included studies.
Conclusions: In the future, more up to date data linkage research addressing costs of diabetes and its complications in a contemporary Australian setting, as well as research assessing ADRs of recently approved antidiabetic medications, are required.
Introduction
Diabetes mellitus is a chronic disease placing a heavy socioeconomic burden not only on patients and their families, but also on society. In 2017–2018, the National Health Survey reported that 1.2 million Australians were living with diabetes with the rate doubling over the preceding 30 years (1). In addition, type 2 diabetes mellitus (T2DM) was ranked as the 13th leading cause of disease burden in Australia (2). The total healthcare expenditure on people with diabetes was estimated to be A$2.7 billion (2.3% total disease expenditure) in 2015–2016 (3). In this context, the provision of information related to diabetes has been considered to be a national priority (4).
Health data are increasingly stored in large administrative electronic databases (5). Although having been developed primarily for administrative purposes, such as providing billing information and tracking health care reimbursement (6, 7), there has been an increasing trend to use these databases for research purposes based on their specific advantages compared to clinical databases (8). Over time, the need for comprehensive datasets to perform high-quality research led to the development of a novel tool maximizing the usefulness of electronic databases for research: data linkage.
Data linkage, or record linkage as it is also known, is a process that matches records representing the same person or entity derived from different data sources in order to generate new and more comprehensive datasets for different purposes, and particularly research (9). Data linkage has been started since the 1970s in Australia and developed gradually after the establishment of the two first data linkage units: The Western Australian Data Linkage System (WADLS) in 1995, and the Centre for Health Record Linkage (CHeReL) in New South Wales in 2006 (10). These units, especially the WADLS, have contributed to many successful linkage projects that have been considered as necessary information to support policy making. To help realise the potential of data linkage, the Australian Government and state authorities invested approximately A$93 million to establish the PHRN in 2009. The PHRN comprises a network of data linkage units located in each Australian state or territory and a national data linkage unit operated by the Australian Institute of Health and Welfare. After the establishment of the PHRN, the number of data linkage studies has increased substantially, including studies focusing on endocrine disorders, such as diabetes (11, 12).
Data linkage enables researchers to obtain a more comprehensive range of information as the data are collected from different sources (13). More importantly, there is a wide range of data sources from administrative, registry, and clinical databases that have been linked, or can be linked, within states or nationally. However, targeted topics of published data linkage studies have mostly focused on service utilization and disease outcomes (5, 14). Until now, although the potential of data linkage in supporting research on chronic diseases, particularly diabetes is undeniable, data linkage usage, as well as quality of data linkage studies on diabetes, have not been properly examined. This systematic review was performed to synthesize the common themes, knowledge gaps, and to evaluate the quality of data linkage publications on diabetes in Australia.
Methods
Information Sources
This systematic review was developed in accordance with the PRISMA Statement (15). The protocol for this review was registered for the International Prospective Register Of Systematic Reviews (PROSPERO, RRID: SCR019061) with registration number CRD42020158030.
Using predefined search strategies (Supplementary Appendix 1), the following six key databases were searched to identify relevant articles published until 31st December 2020: MEDLINE (MEDLINE, RRID: SCR_002185), EMBASE (EMBASE, RRID: SCR_001650), Web of science, Scopus, Econlit, and Google scholar (Google Scholar, RRID: SCR_008878). The search strategies were based partly on previous strategies constructed by Tew et al. (11). Given that our systematic review focused on data linkage, we also searched manually for studies published on the PHRN website. To ensure the comprehensiveness of the search, reference lists of included studies and related systematic reviews were scanned to obtain any other relevant studies.
Study Selection and Eligibility Criteria
All search results were catalogued in EndNote X8 (EndNote, RRID: SCR_014001). After removing duplicates, screening and selection of papers were managed in Covidence (Covidence, RRID: SCR_016484). We used two screening stages to identify included studies against the inclusion and exclusion criteria: screening of titles and abstracts, and full text screening. Both the first and second screening were performed independently by two reviewers. Prior to formal screening, the review team worked together to screen a small sample of studies to ensure the consistency across reviewers. Discrepancies between reviewers were resolved through discussion to reach consensus.
Studies included in the review satisfied the following criteria:
1. Published in English;
2. Used Australian linked data;
3. Focused on diabetes: primary outcomes directly related to diabetes and/or study population involved people with diabetes.
Studies were excluded if they included at least one of the following:
1. Used linked data from other countries;
2. Included other diseases/health conditions without investigating their relationship with diabetes.
3. Did not report any health outcomes;
4. Did not have full text available;
5. Were duplicate publications; protocols, conference abstracts, case reports, reviews, comments and letters without original data; or animal studies.
Data Extraction and Methodological Assessment
Data were extracted using a pre-designed form in Microsoft Excel. The form included the following key elements: Author, publication year, jurisdiction(s), data linkage unit, datasets, linkage method, population, sample size, timeframe, study design, diabetes type, main outcomes, main findings, advantages and disadvantages of using linked data reported by authors. One reviewer independently extracted data from included studies, then a second reviewer conducted an audit from a random 10% of papers. This process was also applied for critical appraisal.
The guidelines for studies involving data linkage were used to appraise methodological quality of included studies (16). The guidelines include 14 reporting items belonging to four domains. In order to calculate quality scores, we applied the approach of Patel et al. (17) with modifications. Because each paper used a different number of databases, for the first domain we calculated the average number of points achieved in each item. The total number of points obtained from all items divided by the number of applicable items was the quality score of each study. Categorising studies as “low,” “medium,” and “high” quality was based on the first and third quartiles of quality scores. We calculated Spearman correlation coefficient to assess if there was any trend in quality score over time.
Results
The systematic search identified 5,759 studies. After screening and hand-searching, 118 studies were included in the final analysis (Figure 1). Characteristics of included studies are presented in Supplementary Appendix 2.
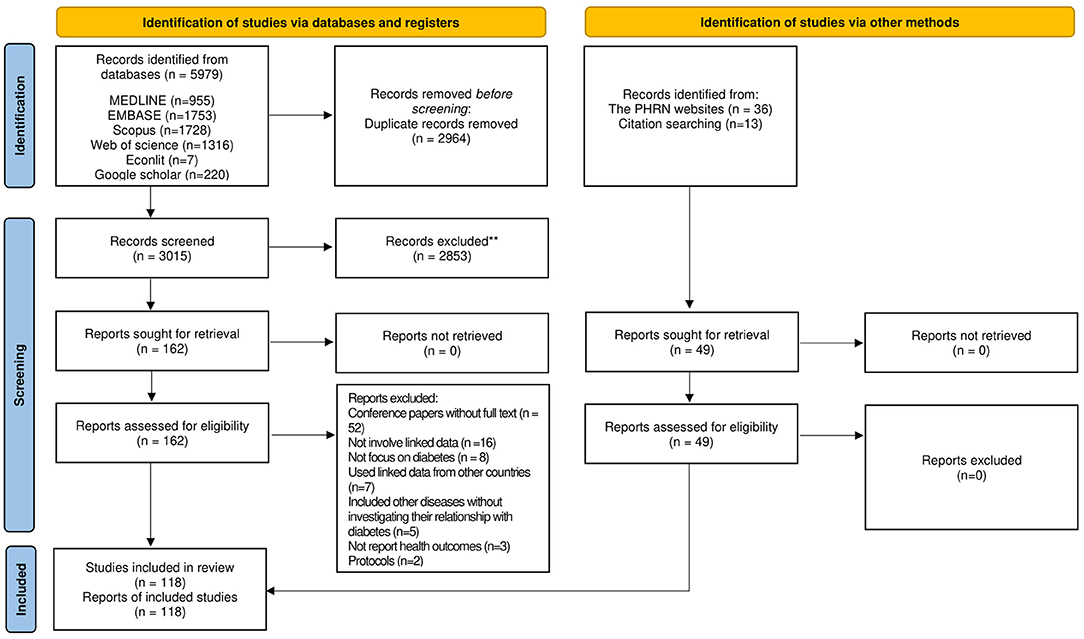
Figure 1. PRISMA 2020 flow diagram for new systematic reviews which included searches of databases, registers and other sources. Figure is adopted from Page et al. (18).
General Finding
Jurisdiction of Linkage
The number of data linkage publications on diabetes across Australia has increased over the years, reaching a peak in 2015–2016 and decreasing slightly from 2017 to 2020 (Figure 2). There were 13 national data linkage studies. Studies involving linked data from multiple states were counted as one publication for each state. Most of the contributions (n = 108; 51.6%) came from WA (19–90) (n = 72; 34.4%) and NSW (72–83, 86, 91–114) (n = 36; 17.2%). Studies also originated from QLD (72–83, 86, 115–124) (n = 23; 11.0%), SA (72–83, 86, 125–131) (n = 20; 9.6%), and VIC (72–83, 86, 129, 132–135) (n = 18; 8.6%). A small number of publications were performed in the NT (72–83, 86, 136) (n = 14; 6.7%), TAS (72–83) (n = 13; 6.2%) (86), and the ACT (72–83) (n = 13; 6.2%).
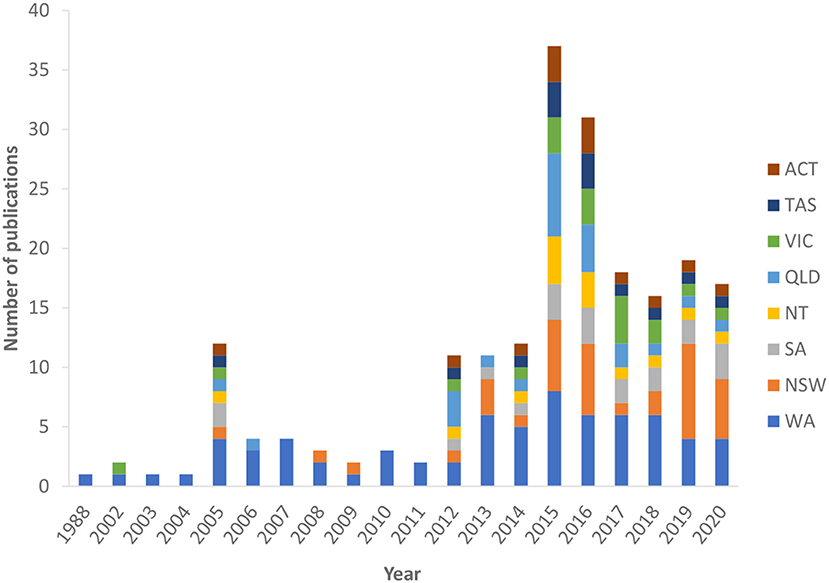
Figure 2. Number of data linkage publications on diabetes by state and publication year. WA, Western Australia; NSW, New South Wales; SA, South Australia; NT, Northern Territory; QLD, Queensland; VIC, Victoria; TAS, Tasmania; ACT, Australian Capital Territory. Studies involving linked data from multiple states were counted as one publication for each state.
Most included articles (n = 77; 65.3%) used data that was linked by a single data linkage organization. However, there were 15 articles (12.7%) using data linked by two organizations. The Western Australian Data Linkage System (WADLS) performed data linkage for nearly half of the included articles (n = 53; 44.9%) (19–21, 24–32, 34, 35, 37, 39–61, 63–71, 84, 85, 87–90), 34 articles (28.8%) used data linked by other linkage units belonging to the PHRN network (73–76, 79–81, 86, 91–101, 104, 105, 107–114, 126, 127, 129–131), and only four articles (3.7%) used data linked by non-PHRN units (33, 103, 106, 135). More importantly, whether the linkages were carried out by an organization or researchers themselves, the name of the organization performing the linkage was not explicitly reported in 26 articles (22.0%) (22, 23, 36, 38, 62, 72, 77, 78, 82, 83, 102, 115–125, 128, 132, 134, 136).
Method of Linkage and Data Sources
The methods of linkage were not provided in most of the included studies (n = 78; 66.1%) (19–21, 23–35, 37, 38, 40–47, 49, 51, 52, 54–59, 61, 63–65, 68–71, 75, 79, 81–83, 85–91, 93, 94, 96–98, 101, 102, 104, 106, 107, 112, 113, 116, 118, 120–122, 124, 125, 127, 128, 133–135). The probabilistic method was predominantly used in the remaining studies (n = 31; 26.3%) (22, 36, 39, 48, 50, 53, 60, 62, 66, 67, 72–74, 76, 77, 80, 92, 99, 100, 103, 105, 108, 117, 119, 123, 126, 129–132), while limited studies were undertaken using deterministic linkage (n = 2; 1.9%) (115, 136) or a combination of these two methods (n = 7; 5.9%) (78, 95, 109–111, 114).
There were a wide range of Australian datasets linked (Figure 3). Amongst them, the most common databases linked were hospital (n = 86; 72.9%), registry of death (n = 75; 63.6%) and study-specific databases (n = 57; 48.3%). Other commonly linked datasets were the Medicare Benefits Schedule (MBS; n = 24; 20.3%), perinatal (n = 19; 16.1%); diabetes register (n = 18; 15.3%); Pharmaceutical Benefits Scheme (PBS; n = 18; 15.3%); electoral roll (n = 14; 11.9%); other disease registers (n = 12, 10.2%), and clinical/laboratory databases (n = 13; 11.0%). Meanwhile, other datasets (n = 20; 16.9%), such as medical records, birth register, emergency department, and general practice databases were less common.
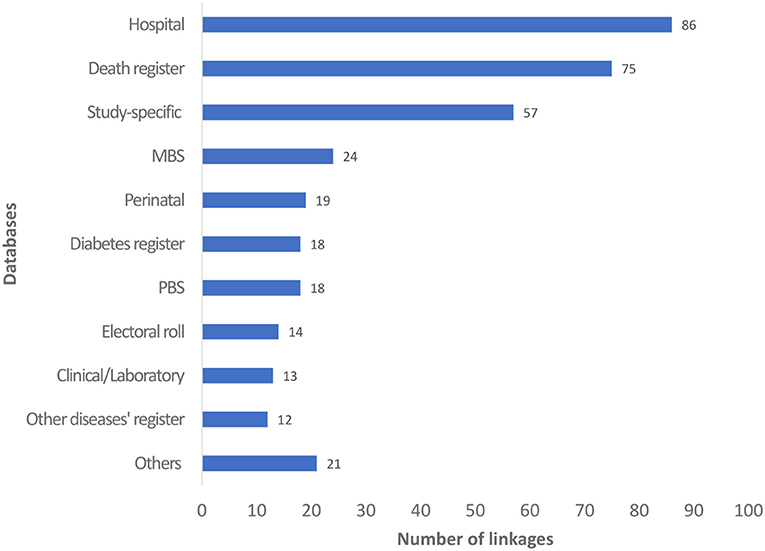
Figure 3. Number of linkages by databases. MBS, Medicare Benefits Schedule; PBS, Pharmaceutical Benefits Scheme.
Timeframe and Diabetes Type
The timeframe of included studies was determined based on the time intervals that data were collected from all linked databases. If the authors did not report their timeframe exactly by date, month and year, the default date of 1st January for the beginning and 31st December for the end of studies was assumed. There were 13 articles (11.0%) that reported a time horizon ≤ 5 years (38, 58, 95, 100, 102, 106, 110, 117, 120, 122, 132, 135, 136). Thirty-five studies (29.7%) used a time frame of 6–10 years (23, 31, 36, 37, 40, 55, 59, 62, 64, 69, 81, 83, 91, 94, 99, 103–105, 107, 109, 111, 115, 116, 118, 119, 121, 123–125, 127, 129, 134). The remaining studies adopted even longer time horizons: 11–15 years (n = 37; 31.4%) (20–22, 24, 25, 27, 30, 32, 33, 35, 49, 50, 52, 54, 56, 57, 67, 72– 78, 80, 82, 85, 86, 88, 89, 93, 96, 97, 101, 108, 112, 114, 128, 130), and ≥16 years (n = 33; 28.0%) (79).
Diabetes type was ascertained by either International Classification of Diseases (ICD) codes used to identify patients or specific types of diabetes mentioned in the papers. T2DM was investigated in most included papers, either alone (n = 44; 37.3%) (19, 20, 24–32, 35, 40, 42–44, 47, 49, 52, 54, 56, 57, 59, 62, 64, 68–71, 75, 85–87, 89–92, 98, 103, 110, 113, 115, 120, 125) or together with other types of diabetes (n = 44; 37.3%) (21–23, 34, 36, 37, 39, 41, 46, 48, 51, 58, 61, 63, 65–67, 72–74, 77, 78, 80, 81, 84, 88, 93, 94, 96, 97, 99–101, 104, 106, 109, 111, 114, 117, 123, 124, 127, 128, 136). Fourteen articles (11.9%) focused on type 1 diabetes mellitus (T1DM) (33, 45, 50, 53, 55, 60, 76, 107, 108, 126, 130, 131, 133, 134) and 10 other articles (8.5%) looked at gestational diabetes (GDM) (38, 102, 105, 116, 118, 121, 122, 129, 132, 135). However, there was a small number of papers (n = 6; 5.1%) that did not specify the type of diabetes covered (79, 82, 83, 95, 112, 119).
Quality of Linked Data
While several measurements can be used to ascertain quality of linkage results, including linkage specificity, sensitivity, false-positive and false-negative rates, most studies reported false-positive and false-negative rates that were relatively low (<1%). However, there were some concerns about missing and incorrect links (23, 67, 129). Other concerns related to the quality of the source information (39, 56, 76, 103, 121, 136), often from administrative databases such as the accuracy of coding (40, 41, 66, 118–120), change in coding system (48, 122), recorder bias (132) or lack of clinical data (21, 22, 34, 45, 46, 72–75, 77, 79, 94, 100, 108, 116, 121, 124, 127).
Common Themes of Research
There were six common themes of research that emerged amongst publications reviewed in our study. Each of these common themes are discussed in detail below. Because of the large degree of heterogeneity amongst different studies, especially in relation to the target populations and outcomes measurements, we could not perform a meta-analysis.
Health Outcomes in People With Diabetes
Nearly half of the included studies (n = 40; 33.9%) focused on health outcomes in people with diabetes; in some cases these were examined in the context of other diseases.
Publications Investigating Diabetes Alone
Health outcomes targeted were mortality (25, 26, 39, 48, 64, 72, 75–77, 79, 80, 92, 98, 127), hospital admissions (23, 90), and pregnancy outcomes (106, 122, 128, 132) (Supplementary Table 1a-Appendix 2).
These studies found that although there was a downward trend in mortality (72, 77), people with diabetes still had a higher risk of death (39, 48, 79, 127), greater number of years of life lost (127) and shorter life expectancy (76) compared to people without diabetes. In addition, diagnosis at younger ages (72, 75, 92), being Indigenous (25, 98), living in the major urban and remote areas (80) and having complications (such as myocardial infarction and peripheral arterial disease) (54, 64) were some specific factors associated with an increased risk of death from diabetes.
In terms of hospitalization, people with diabetes had higher hospitalization rates for both diabetic and non-diabetic causes compared to the general population (23, 90). Specific to pregnancy outcomes, data linkage studies suggested negative maternal and neonatal outcomes in women having diabetes in pregnancy and their infants such as gestational hypertension, induction of labor, and caesarean section (106, 122, 128, 132).
Publications Investigating Diabetes in Relation to Other Diseases or Health Conditions
Because of the higher incidence of some diseases in people with diabetes, association between diabetes and psychiatric disorders (60), dementia (28, 29), infections (43, 119), cancer (42, 73), hip fracture (47), tendon rupture (44), tuberculosis (81), and pancreatitis (41) was reported. There were diseases and health conditions (mental illness, cancer, infection, burn) that solely or in combination with diabetes, were associated with negative outcomes such as increased diabetes-related hospitalization rates (34, 46, 51) or increased mortality (68, 73, 74) (Supplementary Table 1b-Appendix 2).
Incidence of Diabetes/Diabetic Complications and Their Risk Factors
There were 11 studies (9.3%) reporting incidence or prevalence of diabetes and its complications, either alone (66, 87, 130) or in combination with investigating predictors (19, 40, 45, 88, 108, 113, 114, 131), and 21 other studies (17.8%) only focused on risk factors. Most of these papers investigated multiple risk factors simultaneously (19, 32, 40, 45, 57, 59, 105, 108, 114, 123, 124). The remaining papers focused specifically on socio-demographic (49), clinical (55, 59, 70, 78, 88, 133), lifestyle (50, 56, 93, 96, 97, 101), or perinatal factors (53, 107, 126) (Supplementary Table 2-Appendix 2).
Risk Factors for Diabetes
Results of papers exploring risk factors for T1DM suggested that maternal smoking during pregnancy was associated with lower risk of childhood T1DM (50, 131), but were inconsistent in terms of whether caesarean section (107, 126) and increasing birth weight (53, 107) were determinants of T1DM in children. Papers that investigated the risks associated with T2DM and GDM found that smoking (56, 113), being overweight (56, 113, 124) and using statins (78) were factors associated with T2DM. Having metabolic syndrome pre-pregnancy, or GDM in the previous pregnancy, were strong predictors of GDM (105, 123).
Risk Factors for Diabetic Complications
Most of included papers looked at chronic complications, either microvascular (40, 45, 93, 96, 97, 101, 114, 133) or macrovascular complications (19, 49, 55, 57, 59, 70, 85). Only three articles focused on acute complications (32, 88, 108).
In terms of socio-demographic factors, educational status (higher than primary level) (32), ethnicity (Asian or Southern European) (40), sex (women) (45, 108), older age (40, 114) and living in regional or remote areas (108, 114) were associated with some diabetic complications - especially acute and ophthalmic complications. In terms of clinical factors, having other complications (19, 32, 40, 57, 85, 114), longer diabetes duration (32, 40, 133), poor glycaemic (19, 45, 57, 88, 133) and poor lipid control (40, 55) were also strongly associated with increased risk of developing complications, and particularly ophthalmic and foot complications. In terms of lifestyle factors, while physical activity, high consumption of cheese and whole-meal bread showed a positive effect on preventing ophthalmic complications in people with diabetes (96, 97), increasing consumption of red meat and poultry showed harmful effects (101).
Validation of Data Sources and Diabetic Instruments
Linked data was used in six articles (5.1%) to validate data accuracy. When cross referenced with other sources, the accuracy of using administrative data to identify diabetes status was relatively high (sensitivity and specificity were both up to 99%) (84, 118). Cross referencing with administrative databases demonstrated that self-reporting of diabetes was a reliable source to identify patients (109). In addition, using linked data was a method which could improve data completeness (33, 103) and reduce bias by supporting exclusion of ineligible subjects (67).
There were 11 studies (9.3%) that successfully developed and/or validated diabetes instruments. These included a new measure of continuity of primary care (21), a stratification strategy to classify diabetes severity (65), a simple instrument to predict vascular disease severity in people with T2DM (115), risk equations to predict life expectancy (62) and mortality (22) for T2DM people after occurrence of major complications, an Australian risk equation to predict CVD for T2DM people (24), the Framingham and United Kingdom Prospective Diabetes Study cardiovascular risk equations (27), three measurement approaches of regularity of general practice (GP) contacts (61), and the International Association of Diabetes Study Groups criteria to diagnose GDM (38, 102, 135).
Health Service Utilization in People With Diabetes
Articles that focused on the relationship between primary care and hospitalization (n = 6; 5.1%) used different approaches when exploring the concept “continuity of care”. While the two oldest papers only measured the number of GP visits (frequency) (91, 136), more recent publications measured either the dispersion of GP visits over time (regularity) (94) or the combination between regularity and frequency (37, 95, 111). Although most of them suggested the completely inverse association between primary care use and hospitalization (37, 91, 94, 95), two other papers emphasized the importance of maintaining adequate levels of GP contact on reducing hospitalization (111, 136).
The remaining articles (n = 8; 6.8%) suggested that people with diabetes exerted a high demand on the health service (83, 100), however discrepancies between Australians and overseas-born Australians (110), between patients with different socioeconomic statuses (86) were noted. The rates of participation in diabetes-related screening, such as postpartum glucose screening after GDM (116, 121, 129) and screening for diabetic retinopathy (112) were relatively low.
Intervention and Medications in People With Diabetes
Three studies (2.5%) compared mortality or hospitalization between people with diabetes with and without the interventions to explore their effects. While an integrated primary–secondary model of care were proven to be effective (reduced hospitalization) (120), self-monitoring of blood glucose showed no effects (35).
The effects of medications were investigated in five studies (4.2%). Papers focusing on ADRs of antidiabetic medications found that metformin was associated with hypomagnesemia (71) but was not associated with either lactic acidosis (52) nor adverse maternal and neonatal outcomes (104). In addition, continuous subcutaneous insulin infusion and multiple daily injections showed no difference in adverse pregnancy outcomes (134).
Healthcare Costs in People With Diabetes
Seven (5.9%) studies estimated healthcare costs. All costs estimated were direct costs covering several areas of expenditure, but mainly hospital and primary care. The majority were partial economic evaluations (30, 31, 36, 69, 99, 117); only one study performed a cost-effectiveness analysis (125). Overall, healthcare costs in people with diabetes were much higher than people without diabetes (99, 117). Additionally, this expenditure increased exponentially over time because of increasing diabetes prevalence and complications (30, 31, 36, 69).
Methodological Assessment
After applying the quality assessment guidelines for studies involving data linkage to evaluate the quality of included studies, we identified 32 high-quality, 55 medium-quality and 31 low-quality studies (Figure 4). The Spearman correlation coefficient (0.11) suggested a slightly upward trend in quality scores over time but was not considered statistically significant (P = 0.2357).
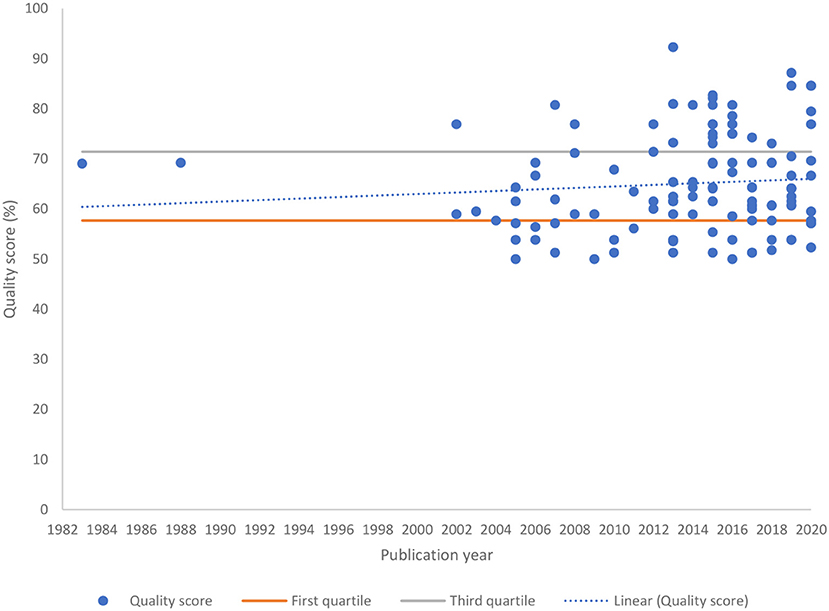
Figure 4. Quality scores of included studies against the guidelines for studies involving data linkage.
Discussion
The results of this review were consistent with previous systematic reviews which showed an upward trend in the number of published papers using Australian linked data over the years, and a variation of research output amongst different states (11, 12). The years 2015 and 2016 saw an expansion in the number of data linkage studies on diabetes in all jurisdictions, and particularly WA and NSW. This could be explained by results from linkage between the National Diabetes Services Scheme (NDSS) and the National Death Index, coinciding with the development of two large data linkage studies: The Fremantle Diabetes Study in WA, and the 45 and Up study in NSW.
There are two main methods used to link data: deterministic and probabilistic matching. Deterministic linkage often requires a set of complete and accurate variables or a unique identifier to create exact matches, whereas probabilistic linkage uses mathematical algorithms to estimate the probability that each pair of records linked refers to the same individual in the population (137). Choosing linkage methods depends on the availability and quality of variables in the datasets. However, with the absence of a unique personal identifier amongst different data sources in Australia (138), it is evident that probabilistic matching was more prevalent.
Databases linked in studies were not restricted to several data sources used commonly in data linkage studies, such as administrative databases [hospital morbidity, death register, Medicare Benefits Schedule (MBS) and Pharmaceutical Benefits Scheme (PBS) claims databases], and register databases [the NDSS, other diseases' registries (tuberculosis, cancer, mental diseases)]. They also included study databases (such as the Fremantle Diabetes, the 45 and Up study's databases) and clinical/laboratory databases. Administrative databases were the sources of information related to mortality, hospital admission and medications. In some studies, linkage containing state electoral roll records or birth registers was performed to identify large comparison cohorts (29, 40–44, 47) or even ascertain migration status of the study population (65). With regard to registry databases, while linkage with the NDSS supported population-based research on diabetes, linkage with other disease registers enabled researchers to investigate the complicated relationship between diabetes and other diseases. Study and clinical/laboratory databases provided researchers with unique variables that were not available in administrative databases including details of lifestyle, and glycaemic and lipid control (HbA1c, HDL cholesterol, LDL cholesterol, triglycerides). Combining all these sources into one comprehensive data set has allowed researchers to answer multiple research questions that would otherwise be difficult to answer from one single source. Based on the diverse information available from linked data, many aspects of diabetes were explored. Data linkage studies supported findings from previous studies with strong evidence based on their large sample sizes, long duration of follow-up, a variety of information related to potential confounders, and outcomes accurately captured from many sources.
Negative Health Outcomes in People With Diabetes and Their Consequences
In our review, health outcomes in people with diabetes was the most common topic studied. This may be due to the feasibility of ascertaining outcomes data through frequently linked database, such as hospital admission and mortality (12). Another explanation is the potential of linked data to investigate outcomes necessitating prolonged durations to develop, and particularly in chronic diseases such as diabetes.
Data linkage studies found associations between diabetes and negative health outcomes. These included higher risk of death and hospitalization, and higher tendency for negative outcomes in pregnancy. In addition, associations between diabetes and mental illness, cancer, and infections were observed. As a result, demand on health services from people with diabetes was large and the healthcare costs were substantial and increasing exponentially.
Confirmed and Conflicting Risk Factors for Diabetes and Its Complications
Findings related to risk factors for diabetes and its complications from previous non-data linkage studies have been inconsistent with insufficient power to detect small effects cited as a feature (53). Data linkage studies with community-based samples confirmed the importance of potential risk factors for diabetes (overweight, smoking) and diabetic complications (having other diabetic complications, poor glycaemic and lipid control) reported previously, and suggested conflicting risk factors. These included the association between maternal smoking, caesarean section, increasing birth weight and T1DM in children. This controversial information should be assessed further in future research.
Data Linkage as a Validation Tool
The potential of linked data as a tool to validate and improve the accuracy of data sources has been mentioned in literature (139, 140). The most frequently linked databases, administrative databases, were the focus of many validation studies using ICD codes (141). More importantly, due to the availability of disparate data sources, data linkage facilitates validation of case-finding algorithms that are constructed using a variety of information, such as a combination of ICD codes and other criteria (MBS and PBS claims, self-reported data…) to identify patients with targeted diseases (141). Apart from studies validating data accuracy, research that was specific to diabetes has been undertaken using linked data to develop and validate diabetes-related instruments which have potential utility in either clinical practice or future research.
The Association Between Primary Care and Hospitalization
Investigating the association between primary care and hospitalization was very challenging in the past as data are stored in different databases (91). With the development of data linkage methods, it is now possible for researchers to explore this relationship comprehensively. Over time, approaches to measure continuity of primary care have been improved, from monitoring only the frequency of visits to the more recent shift in perspective focusing on the effects of both regularity and frequency of GP visits on hospitalization. Data linkage studies revealed a greater understanding of the connexion between primary care and hospitalization, to help support health policymakers to make evidence-based decisions towards strengthening primary care in diabetes.
Future Research
The advantages of using linked data to investigate some specific topics, such as healthcare costs and ADRs have been discussed in previous studies (36, 142–145). However, in our review, there were few articles reporting healthcare costs which were either outdated or focused on specific groups which would not provide a comprehensive picture of total costs related to diabetes care. In terms of ADRs, until now, published studies involving linked data have explored ADRs of only a few older antidiabetic medications with well-known ADRs. Given that diabetes imposes a large economic burden on the healthcare system due to its complications and comorbidities, accurately estimating the true economic burden of diabetes is very challenging. In the future, researchers who are interested in data linkage should take into account the availability of linking hospital, study-specific databases, MBS and PBS claims databases to investigate many aspects of healthcare use and expenditure related to diabetes. In addition, assessing ADRs of recently approved medications, such as glucagon-like peptide-1 agonist, dipeptidyl peptidase four inhibitors, and sodium glucose cotransporter 2 should be conducted if there is no profound evidence gathered from existing studies, given the capacity of data linkage to support further examination of the associations between medications and ADRs that were previously established through clinical trials.
In Australia, a systematic review regarding the use of linked hospital data from 1995 onwards identified circulatory diseases, cancer, and mental health diseases as some of the most common topics researched in data linkage studies (11). Similar to diabetes, these diseases could be used as resources for data linkage research.
Ensuring the Quality of Linked Data
Besides widely recognised advantages listed, there were certain limitations of data linkage that were mostly related to the quality of linked data mentioned above. However, reporting these important details was often ignored in publications. Although some of these limitations are unavoidable, ensuring the quality of linked data is an essential component as it can impact the accuracy and transparency of results (146). Lacking a unique personal identifier amongst different data sources in Australia could be an obstacle for performing linkage with a perfect match. Nevertheless, efforts could be made to increase the quality of linked data, such as developing and opereting a regular quality assurance process for each administrative database.
Methodological Assessment
There was under-reporting of important information about the linkage, such as methods of linkage, variables used to link data, and quality of linked data. Given the increasing number of data linkage publications, it is important for authors to be careful to report their data transparently and consistently. Adopting guidelines for evaluating the quality of studies using linked data is recommended. However, the quality scores should be interpreted cautiously as some criteria in the guidelines were not applicable for all included studies (16). For example, in the second domain of the guidelines, there is a criterion for reporting changes to coding systems of included datasets; while in some studies no such changes occurred, or the datasets did not require coding. This problem can be resolved by making this criterion optional.
Strengths and Limitations
This is the first systematic review focusing on data linkage studies on diabetes in Australia. Using search strategies combined with hand searching, we identified 118 relevant studies. Additionally, our study used a new, validated tool designed to appraise the quality of data linkage studies.
However, there were some unavoidable limitations in conducting this review. Firstly, there was potential that the search strategies may have missed relevant studies if studies only described data linkage usage in their full texts, but not in their titles and abstracts. Secondly, this study could not provide further quantitative pooled data, or meta-analysis data to support our findings, due to heterogeneity.
Conclusion
Our review identified the widespread use of data linkage to address questions related to diabetes in Australia. While some studies have investigated the costs of diabetes and its complications in Australia, more timely research based on data linkage are required to address costs of diabetes and its complications in a contemporary Australian setting. In addition, data linkage studies assessing ADRs of recently approved medications should also be undertaken.
Although we cannot use only evidence gathered from data linkage studies to represent all available data for diabetes, this review will provide a comprehensive picture of what type of evidence that we would expect from data linkage research and whether there are any specific advantages of using data linkage to study diabetes. Findings from this review will contribute to supporting practitioners and policymakers in decision-making and guiding future data linkage research.
Data Availability Statement
The data that support this study are available in the article and accompanying online Supplementary Material. Further inquiries can be directed to the corresponding author/s.
Author Contributions
ND conceived the study, performed the literature search, study selection, data extraction and methodological assessment, and prepared the manuscript. IC performed study selection, assisted with data extraction, methodological assessment, and preparation of the manuscript. BdG conceived the study and assisted with preparation of the manuscript. JC conceived the study, assisted with study selection and preparation of the manuscript. BS provided opinion related to data linkage and assisted with preparation of the manuscript. AP conceived the study and assisted with preparation of the manuscript. All authors read and approved the final manuscript.
Conflict of Interest
The authors declare that the research was conducted in the absence of any commercial or financial relationships that could be construed as a potential conflict of interest.
Publisher's Note
All claims expressed in this article are solely those of the authors and do not necessarily represent those of their affiliated organizations, or those of the publisher, the editors and the reviewers. Any product that may be evaluated in this article, or claim that may be made by its manufacturer, is not guaranteed or endorsed by the publisher.
Supplementary Material
The Supplementary Material for this article can be found online at: https://www.frontiersin.org/articles/10.3389/fpubh.2022.757987/full#supplementary-material
Abbreviations
ADRs, adverse drug reactions; GDM, gestational diabetes; ICD, International Classification of Diseases; MBS, Medicare Benefits Schedule; NDSS, National Diabetes Services; PBS, Pharmaceutical Benefits Scheme; PHRN, Population Health Research Network; T1DM, type 1 diabetes mellitus; T2DM, type 2 diabetes mellitus; WADLS, Western Australian Data Linkage System.
References
1. Australian Bureau of Statistic. National Health Survey: First Results, 2017-18. Commonwealth of Australia (2018). Available online at: https://www.abs.gov.au/AUSSTATS/abs@.nsf/Lookup/4364.0.55.001Main+Features702017-18?OpenDocument (accessed May 8, 2020).
2. Australian Institute of Health Welfare. Australian Burden of Disease Study Impact and causes of illness and death in Australia 2015. (2019). Available online at: https://www.aihw.gov.au/getmedia/c076f42f-61ea-4348-9c0a-d996353e838f/aihw-bod-22.pdf.aspx?inline=true (accessed July 9, 2020).
3. Australian Institute of Health Welfare. Diabetes Commonwealth of Australia. (2019). Available online at: https://www.aihw.gov.au/reports/diabetes/diabetes/contents/impact (accessed February 15, 2021).
4. Colagiuri S, Colagiuri R, Ward J. National diabetes strategy and implementation plan. Diabetes. (1998). Available online at: https://citese erx.ist.psu.edu/viewdoc/download?doi=10.1.1.138.617&rep=rep1&type=pdf (accessed May 8, 2020).
5. Webb P, Bain C, Page A. Essential Epidemiology: An Introduction For Students and Health Professionals. Cambridge: Cambridge University Press (2017). doi: 10.1017/9781316275948
6. Hashimoto RE, Brodt ED, Skelly AC, Dettori JR. Administrative database studies: goldmine or goose chase? Evid Based Spine Care J. (2014) 5:74–6. doi: 10.1055/s-0034-1390027
7. Maetens A, De Schreye R, Faes K, Houttekier D, Deliens L, Gielen B, et al. Using linked administrative and disease-specific databases to study end-of-life care on a population level. BMC Palliat Care. (2016) 15:1–10. doi: 10.1186/s12904-016-0159-7
8. Johnson EK, Nelson CP. Values and pitfalls of the use of administrative databases for outcomes assessment. J Urol. (2013) 190:17–8. doi: 10.1016/j.juro.2013.04.048
9. Gu L, Baxter R, Vickers D, Rainsford C. Record linkage: Current practice and future directions. (2003). Available online at: http://citeseerx.ist.psu.edu/viewdoc/download?doi=10.1.1.14.8119&rep=rep1&type=pdf (accessed May 8, 2020).
10. Moore HC, Guiver T, Woollacott A, De Klerk N, Gidding HF. Establishing a process for conducting cross-jurisdictional record linkage in Australia. Aust N Z J Public Health. (2016) 40:159–64. doi: 10.1111/1753-6405.12481
11. Tew M, Dalziel KM, Petrie DJ, Clarke PM. Growth of linked hospital data use in Australia: a systematic review. Aust Health Rev. (2016) 41:394–400. doi: 10.1071/AH16034
12. Young A, Flack F. Recent trends in the use of linked data in Australia. Aust Health Rev. (2018) 42:584–90. doi: 10.1071/AH18014
13. Bohensky MA, Jolley D, Sundararajan V, Evans S, Pilcher DV, Scott I, et al. Data linkage: a powerful research tool with potential problems. BMC Health Serv Res. (2010) 10:1–7. doi: 10.1186/1472-6963-10-346
15. Moher D, Liberati A, Tetzlaff J, Altman DG, PRISMA Group. Preferred reporting items for systematic reviews and meta-analyses: the PRISMA statement. Ann Intern Med. (2009) 151:264–9. doi: 10.7326/0003-4819-151-4-200908180-00135
16. Bohensky MA, Jolley D, Sundararajan V, Evans S, Ibrahim J, Brand C. Development and validation of reporting guidelines for studies involving data linkage. Aust N Z J Public Health. (2011) 35:486–9. doi: 10.1111/j.1753-6405.2011.00741.x
17. Patel K, Kouvonen A, Close C, Väänänen A, O'Reilly D, Donnelly M. What do register-based studies tell us about migrant mental health? A scoping review. Syst Rev. (2017) 6:1–11. doi: 10.1186/s13643-017-0463-1
18. Page MJ, McKenzie JE, Bossuyt PM, Boutron I, Hoffmann TC, Mulrow CD, et al. The PRISMA 2020 statement: an updated guideline for reporting systematic reviews. BMJ. (2021) 372:n71. doi: 10.1136/bmj.n71
19. Baba M, Davis WA, Davis TM. A longitudinal study of foot ulceration and its risk factors in community-based patients with type 2 diabetes: the Fremantle Diabetes Study. Diabetes Res Clin Pract. (2014) 106:42–9. doi: 10.1016/j.diabres.2014.07.021
20. Bruce DG, Davis WA, Starkstein SE, Davis TME. A prospective study of depression and mortality in patients with type 2 diabetes: the Fremantle Diabetes Study. Diabetologia. (2005) 48:2532–9. doi: 10.1007/s00125-005-0024-3
21. Ha NT, Harris M, Preen D, Robinson S, Moorin R. A time-duration measure of continuity of care to optimise utilisation of primary health care: a threshold effects approach among people with diabetes. BMC Health Serv Res. (2019) 19:276. doi: 10.1186/s12913-019-4099-9
22. Hayes AJ, Davis WA, Davis TM, Clarke PM. Adapting and validating diabetes simulation models across settings: accounting for mortality differences using administrative data. J Diabetes Complications. (2013) 27:351–6. doi: 10.1016/j.jdiacomp.2012.12.006
23. De Klerk NH, Armstrong BK. Admission to hospital for road trauma in patients with diabetes mellitus. J Epidemiol Commun Health. (1983) 37:232–7. doi: 10.1136/jech.37.3.232
24. Davis WA, Knuiman MW, Davis TME. An Australian cardiovascular risk equation for type 2 diabetes: the Fremantle Diabetes Study. Intern Med J. (2010) 40:286–92. doi: 10.1111/j.1445-5994.2009.01958.x
25. Davis TME, McAullay D, Davis WA, Bruce DG. Characteristics and outcome of type 2 diabetes in urban Aboriginal people: the Fremantle Diabetes Study. Intern Med J. (2007) 37:59–63. doi: 10.1111/j.1445-5994.2006.01247.x
26. Tan ED, Davis WA, Davis TM. Characteristics and prognosis of Asian patients with type 2 diabetes from a multi-racial Australian community: the Fremantle Diabetes Study. Intern Med J. (2013) 43:1125–32. doi: 10.1111/imj.12246
27. Davis WA, Colagiuri S, Davis TME. Comparison of the Framingham and United Kingdom Prospective Diabetes Study cardiovascular risk equations in Australian patients with type 2 diabetes from the Fremantle Diabetes Study. Med J Aust. (2009) 190:180–4. doi: 10.5694/j.1326-5377.2009.tb02343.x
28. Bruce DG, Davis TME, Davis WA. Dementia complicating type 2 diabetes and the influence of premature mortality: the Fremantle Diabetes Study. Acta Diabetol. (2019) 56:767–76. doi: 10.1007/s00592-019-01322-9
29. Davis WA, Zilkens RR, Starkstein SE, Davis TM, Bruce DG. Dementia onset, incidence and risk in type 2 diabetes: a matched cohort study with the Fremantle Diabetes Study Phase I. Diabetologia. (2017) 60:89–97. doi: 10.1007/s00125-016-4127-9
30. Davis WA, Lewin G, Davis TM, Bruce DG. Determinants and costs of community nursing in patients with type 2 diabetes from a community-based observational study: the Fremantle Diabetes Study. Int J Nurs Stud. (2013) 50:1166–71. doi: 10.1016/j.ijnurstu.2012.11.013
31. Davis WA, Knuiman MW, Hendrie D, Davis TME. Determinants of diabetes-attributable non-blood glucose-lowering medication costs in type 2 diabetes: the Fremantle Diabetes Study. Diabetes Care. (2005) 28:329–36. doi: 10.2337/diacare.28.2.329
32. Davis TME, Brown SGA, Jacobs IG, Bulsara M, Bruce DG, Davis WA. Determinants of severe hypoglycemia complicating type 2 diabetes: the Fremantle Diabetes Study. J Clin Endocrinol Metab. (2010) 95:2240–7. doi: 10.1210/jc.2009-2828
33. Glatthaar C, Whittall DE, Welborn TA, Gibson MJ, Brooks BH, Ryan MM, et al. Diabetes in Western Australian children: descriptive epidemiology. Med J Aust. (1988) 148:117–23. doi: 10.5694/j.1326-5377.1988.tb112770.x
34. Duke JM, Randall SM, Fear MW, Boyd JH, Rea S, Wood FM. Diabetes mellitus after injury in burn and non-burned patients: a population based retrospective cohort study. Burns. (2018) 44:566–72. doi: 10.1016/j.burns.2017.10.019
35. Davis WA, Bruce DG, Davis TM. Does self-monitoring of blood glucose improve outcome in type 2 diabetes? The Fremantle Diabetes Study. Diabetologia. (2007) 50:510–5. doi: 10.1007/s00125-006-0581-0
36. Clarke P, Leal J, Kelman C, Smith M, Colagiuri S. Estimating the cost of complications of diabetes in Australia using administrative health-care data. Value Health. (2008) 11:199–206. doi: 10.1111/j.1524-4733.2007.00228.x
37. Ha NT, Harris M, Preen D, Robinson S, Moorin R. Identifying patterns of general practitioner service utilisation and their relationship with potentially preventable hospitalisations in people with diabetes: the utility of a cluster analysis approach. Diabetes Res Clin Pract. (2018) 138:201–10. doi: 10.1016/j.diabres.2018.01.027
38. Laafira A, White SW, Griffin CJ, Graham D. Impact of the new IADPSG gestational diabetes diagnostic criteria on pregnancy outcomes in Western Australia. Aust N Z J Obstet Gynaecol. (2016) 56:36–41. doi: 10.1111/ajo.12394
39. Nedkoff L, Knuiman M, Hung J, Briffa TG. Improving 30-day case fatality after incident myocardial infarction in people with diabetes between 1998 and 2010. Heart. (2015) 101:1318–24. doi: 10.1136/heartjnl-2015-307627
40. Drinkwater JJ, Davis TME, Turner AW, Bruce DG, Davis WA. Incidence and determinants of intraocular lens implantation in type 2 diabetes: the fremantle diabetes study phase II. Diabetes Care. (2019) 42:288–96. doi: 10.2337/dc18-1556
41. Davis TM, Drinkwater J, Davis WA. Incidence and precipitants of hospitalization for pancreatitis in people with diabetes: the Fremantle Diabetes Study. Diabetic Med. (2014) 31:913–9. doi: 10.1111/dme.12448
42. Magliano DJ, Davis WA, Shaw JE, Bruce DG, Davis TME. Incidence and predictors of all-cause and site-specific cancer in type 2 diabetes: the Fremantle Diabetes Study. Eur J Endocrinol. (2012) 167:589–99. doi: 10.1530/EJE-12-0053
43. Hamilton EJ, Martin N, Makepeace A, Sillars BA, Davis WA, Davis TME. Incidence and predictors of hospitalization for bacterial infection in community-based patients with type 2 diabetes: the Fremantle Diabetes Study. PLoS ONE. (2013) 8:e60502. doi: 10.1371/journal.pone.0060502
44. Zakaria MHB, Davis WA, Davis TME. Incidence and predictors of hospitalization for tendon rupture in Type 2 diabetes: the Fremantle Diabetes Study. Diabet Med. (2014) 31:425–30. doi: 10.1111/dme.12344
45. Cooper MN, de Bock MI, Carter KW, de Klerk NH, Jones TW, Davis EA. Incidence of and risk factors for hospitalisations due to vascular complications: a population-based type 1 diabetes cohort (n = 1316) followed into early adulthood. J Diabetes Complications. (2017) 31:843–9. doi: 10.1016/j.jdiacomp.2016.11.022
46. Duke JM, Randall SM, Fear MW, Boyd JH, O'Halloran E, Rea S, et al. Increased admissions for diabetes mellitus after burn. Burns. (2016) 42:1734–9. doi: 10.1016/j.burns.2016.06.005
47. Hamilton E, Davis WA, Bruce DG, Davis TME. Influence of premature mortality on the link between type 2 diabetes and hip fracture: the Fremantle Diabetes Study. J Clinical Endocrinol Metab. (2017) 102:551–9. doi: 10.1210/jc.2016-3570
48. Nedkoff L, Knuiman M, Hung J, Briffa TG. Long-term all-cause and cardiovascular mortality following incident myocardial infarction in men and women with and without diabetes: temporal trends from 1998 to 2009. Eur J Prev Cardiol. (2016) 23:1273–81. doi: 10.1177/2047487316634279
49. Bruce DG, Van Minnen K, Davis WA, Mudhar J, Perret M, Subawickrama DP, et al. Maternal family history of diabetes is associated with a reduced risk of cardiovascular disease in women with type 2 diabetes: the Fremantle Diabetes Study. Diabetes Care. (2010) 33:1477–83. doi: 10.2337/dc10-0147
50. Haynes A, Cooper MN, Bower C, Jones TW, Davis EA. Maternal smoking during pregnancy and the risk of childhood type 1 diabetes in Western Australia. Diabetologia. (2014) 57:469–72. doi: 10.1007/s00125-013-3122-7
51. Mai Q, Holman CD, Sanfilippo FM, Emery JD, Preen DB. Mental illness related disparities in diabetes prevalence, quality of care and outcomes: a population-based longitudinal study. BMC Med. (2011) 9:118. doi: 10.1186/1741-7015-9-118
52. Kamber N, Davis WA, Bruce DG, Davis TM. Metformin and lactic acidosis in an Australian community setting: the Fremantle Diabetes Study. Med J Aus. (2008) 188:446–9. doi: 10.5694/j.1326-5377.2008.tb01713.x
53. Haynes A, Bower C, Bulsara MK, Finn J, Jones TW, Davis EA. Perinatal risk factors for childhood Type 1 diabetes in Western Australia—a population-based study (1980–2002). Diabet Med. (2007) 24:564–70. doi: 10.1111/j.1464-5491.2007.02149.x
54. Norman PE, Davis WA, Bruce DG, Davis TME. Peripheral arterial disease and risk of cardiac death in type 2 diabetes: the Fremantle Diabetes Study. Diabet Care. (2006) 29:575–80. doi: 10.2337/diacare.29.03.06.dc05-1567
55. Davis TME, Bruce DG, Davis WA. Predictors of first stroke in type 1 diabetes: the Fremantle Diabetes Study. Diabet Med. (2005) 22:551–3. doi: 10.1111/j.1464-5491.2005.01462.x
56. Burke V, Zhao Y, Lee AH, Hunter E, Spargo RA, Gracey M, et al. Predictors of type 2 diabetes and diabetes-related hospitalisation in an Australian Aboriginal cohort. Diabetes Res Clin Pract. (2007) 78:360–8. doi: 10.1016/j.diabres.2007.04.007
57. Davis WA, Norman PE, Bruce DG, Davis TME. Predictors, consequences and costs of diabetes-related lower extremity amputation complicating type 2 diabetes: the Fremantle Diabetes Study. Diabetologia. (2006) 49:2634–41. doi: 10.1007/s00125-006-0431-0
58. Hart J, Hamilton EJ, Makepeace A, Davis WA, Latkovic E, Lim EM, et al. Prevalence, risk factors and sequelae of Staphylococcus aureus carriage in diabetes: the Fremantle Diabetes Study Phase II. J Diabetes Complications. (2015) 29:1092–7. doi: 10.1016/j.jdiacomp.2015.06.005
59. Gillett M, Davis WA, Jackson D, Bruce DG, Davis TME. Prospective evaluation of carotid bruit as a predictor of first stroke in type 2 diabetes. Stroke. (2003) 34:2145–51. doi: 10.1161/01.STR.0000087360.91794.11
60. Cooper MN, Lin A, Alvares GA, de Klerk NH, Jones TW, Davis EA. Psychiatric disorders during early adulthood in those with childhood onset type 1 diabetes: rates and clinical risk factors from population-based follow-up. Pediatr Diabetes. (2017) 18:599–606. doi: 10.1111/pedi.12469
61. Youens D, Harris M, Robinson S, Preen DB, Moorin RE. Regularity of contact with GPs: measurement approaches to improve valid associations with hospitalization. Fam Pract. (2019) 36:650–6. doi: 10.1093/fampra/cmz002
62. Hayes AJ, Leal J, Kelman CW, Clarke PM. Risk equations to predict life expectancy of people with type 2 diabetes mellitus following major complications: a study from Western Australia. Diabetic Med. (2011) 28:428–35. doi: 10.1111/j.1464-5491.2010.03189.x
63. Davis WA, Starkstein SE, Bruce DG, Davis TME. Risk of suicide in Australian adults with diabetes: the Fremantle Diabetes Study. Intern Med J. (2015) 45:976–80. doi: 10.1111/imj.12853
64. Davis TME, Fortun P, Mulder J, Davis WA, Bruce DG. Silent myocardial infarction and its prognosis in a community-based cohort of type 2 diabetic patients: the Fremantle Diabetes Study. Diabetologia. (2004) 47:395–9. doi: 10.1007/s00125-004-1344-4
65. Ha NT, Harris M, Robinson S, Preen D, Moorin R. Stratification strategy for evaluating the influence of diabetes complication severity index on the risk of hospitalization: a record linkage data in Western Australia. J Diabetes Complications. (2017) 31:1175–80. doi: 10.1016/j.jdiacomp.2017.03.015
66. Kurowski JR, Nedkoff L, Schoen DE, Knuiman M, Norman PE, Briffa TG. Temporal trends in initial and recurrent lower extremity amputations in people with and without diabetes in Western Australia from 2000 to 2010. Diabetes Res Clin Pract. (2015) 108:280–7. doi: 10.1016/j.diabres.2015.02.008
67. Youens D, Preen DB, Harris MN, Moorin RE. The importance of historical residential address information in longitudinal studies using administrative health data. Int J Epidemiol. (2018) 47:69–80. doi: 10.1093/ije/dyx156
68. Davis WA, Starkstein SE, Bruce DG, Davis TM. The interactive effects of type 2 diabetes mellitus and schizophrenia on all-cause mortality: the Fremantle Diabetes Study. J Diabetes Complications. (2015) 29:1320–2. doi: 10.1016/j.jdiacomp.2015.08.019
69. Davis WA, Knuiman MW, Hendrie D, Davis TME. The obesity-driven rising costs of type 2 diabetes in Australia: projections from the Fremantle Diabetes Study. Intern Med J. (2006) 36:155–61. doi: 10.1111/j.1445-5994.2006.01014.x
70. Peters KE, Davis WA, Beilby J, Hung J, Bruce DG, Davis TME. The relationship between circulating adiponectin, ADIPOQ variants and incident cardiovascular disease in type 2 diabetes: the Fremantle Diabetes Study. Diabetes Res Clin Pract. (2018) 143:62–70. doi: 10.1016/j.diabres.2018.06.005
71. Peters KE, Chubb SAP, Davis WA, Davis TME. The Relationship between hypomagnesemia, metformin therapy and cardiovascular disease complicating type 2 diabetes: the Fremantle Diabetes Study. PLoS ONE. (2013) 8:e74355. doi: 10.1371/journal.pone.0074355
72. Harding JL, Shaw JE, Peeters A, Davidson S, Magliano DJ. Age-specific trends from 2000–2011 in all-cause and cause-specific mortality in type 1 and type 2 diabetes: a cohort study of more than one million people. Diabetes Care. (2016) 39:1018–26. doi: 10.2337/dc15-2308
73. Harding JL, Shaw JE, Peeters A, Cartensen B, Magliano DJ. Cancer risk among people with type 1 and type 2 diabetes: disentangling true associations, detection bias, and reverse causation. Diabetes Care. (2015) 38:264–70. doi: 10.2337/dc14-1996
74. Magliano DJ, Harding JL, Cohen K, Huxley RR, Davis WA, Shaw JE. Excess risk of dying from infectious causes in those with type 1 and type 2 diabetes. Diabetes Care. (2015) 38:1274–80. doi: 10.2337/dc14-2820
75. Huo L, Magliano DJ, Ranciere F, Harding JL, Nanayakkara N, Shaw JE, et al. Impact of age at diagnosis and duration of type 2 diabetes on mortality in Australia 1997-2011. Diabetologia. (2018) 61:1055–63. doi: 10.1007/s00125-018-4544-z
76. Huo L, Harding JL, Peeters A, Shaw JE, Magliano DJ. Life expectancy of type 1 diabetic patients during 1997-2010: a national Australian registry-based cohort study. Diabetologia. (2016) 59:1177–85. doi: 10.1007/s00125-015-3857-4
77. Harding JL, Shaw JE, Peeters A, Guiver T, Davidson S, Magliano DJ. Mortality trends among people with type 1 and type 2 diabetes in Australia: 1997-2010. Diabetes Care. (2014) 37:2579–86. doi: 10.2337/dc14-0096
78. Jones M, Tett S, Peeters G, Mishra GD, Dobson A. New-onset diabetes after statin exposure in elderly women: the Australian Longitudinal Study on Women's Health. Drugs Aging. (2017) 34:203–9. doi: 10.1007/s40266-017-0435-0
79. Wubishet BL, Harris ML, Forder PM, Acharya SH, Byles JE. Predictors of 15-year survival among Australian women with diabetes from age 76-81. Diabetes Res Clin Pract. (2019) 150:48–56. doi: 10.1016/j.diabres.2019.02.016
80. Magliano DJ, Cohen K, Harding JL, Shaw JE. Residential distance from major urban areas, diabetes and cardiovascular mortality in Australia. Diabetes Res Clin Pract. (2015) 109:271–8. doi: 10.1016/j.diabres.2015.05.029
81. Dobler CC, Flack JR, Marks GB. Risk of tuberculosis among people with diabetes mellitus: an Australian nationwide cohort study. BMJ Open. (2012) 2:e000666. doi: 10.1136/bmjopen-2011-000666
82. Tatoulis J, Wynne R, Skillington PD, Buxton BF. Total arterial revascularization: a superior strategy for diabetic patients who require coronary surgery. Ann Thorac Surg. (2016) 102:1948–55. doi: 10.1016/j.athoracsur.2016.05.062
83. Young AF, Lowe JM, Byles JE, Patterson AJ. Trends in health service use for women in Australia with diabetes. Aust N Z J Public Health. (2005) 29:422–8. doi: 10.1111/j.1467-842X.2005.tb00221.x
84. Nedkoff L, Knuiman M, Hung J, Sanfilippo FM, Katzenellenbogen JM, Briffa TG. Concordance between administrative health data and medical records for diabetes status in coronary heart disease patients: a retrospective linked data study. BMC Med Res Methodol. (2013) 13:121. doi: 10.1186/1471-2288-13-121
85. Drinkwater JJ, Davis TM, Hellbusch V, Turner AW, Bruce DG, Davis WA. Retinopathy predicts stroke but not myocardial infarction in type 2 diabetes: the Fremantle Diabetes Study Phase II. Cardiovasc Diabetol. (2020) 19:1–11. doi: 10.1186/s12933-020-01018-3
86. Morton JI, Ilom?ki J, Magliano DJ, Shaw JE. The association of socioeconomic disadvantage and remoteness with receipt of type 2 diabetes medications in Australia: a nationwide registry study. Diabetologia. (2021) 64:349–60. doi: 10.1007/s00125-020-05304-3
87. Davis TM, Hunt K, McAullay D, Chubb SA, Sillars BA, Bruce DG, et al. Continuing disparities in cardiovascular risk factors and complications between aboriginal and Anglo-Celt Australians with type 2 diabetes: the Fremantle Diabetes Study. Diabetes Care. (2012) 35:2005–11. doi: 10.2337/dc12-0225
88. Davis TM, Davis W. Incidence and associates of diabetic ketoacidosis in a community-based cohort: the Fremantle Diabetes Study Phase II. BMJ Open Diabetes Res Care. (2020) 8:e000983. doi: 10.1136/bmjdrc-2019-000983
89. Davis TM, Davis WA. Influence of renin-angiotensin system inhibitors on lower–respiratory tract infections in type 2 diabetes: the Fremantle Diabetes Study Phase II. Diabetes Care. (2020) 43:2113–20. doi: 10.2337/dc20-0895
90. Brameld KJ, Ward A, Gavin AL, Holman CD. Health outcomes in people with type 2 diabetes. A record linkage study. Aust Fam Physician. (2002) 31:775–8, 82. Available online at: https://search.informit.org/doi/pdf/10.3316/informit.380827334379246
91. Comino EJ, Tran DT, Taggart JR, Liaw ST, Ruscoe W, Snow JM, et al. A preliminary study of the relationship between general practice care and hospitalisation using a diabetes register, CARDIAB. Aust Health Rev. (2013) 37:210–7. doi: 10.1071/AH12175
92. Al-Saeed AH, Constantino MI, Molyneaux L, D'Souza M, Limacher-Gisler F, Luo C, et al. An inverse relationship between age of type 2 diabetes onset and complication risk and mortality: the impact of youth-onset type 2 diabetes. Diabetes Care. (2016) 39:823–9. doi: 10.2337/dc15-0991
93. Han X, Wu C, Yan X, Keel S, Shang X, Zhang L, et al. Are smoking intensity and cessation related to cataract surgical risk in diabetic patients? Findings from the 45 and Up Study. Eye. (2019) 34:383–91. doi: 10.1038/s41433-019-0550-8
94. Moorin RE, Youens D, Preen DB, Harris M, Wright CM. Association between continuity of provider-adjusted regularity of general practitioner contact and unplanned diabetes-related hospitalisation: a data linkage study in New South Wales, Australia, using the 45 and Up Study cohort. BMJ Open. (2019) 9:e027158. doi: 10.1136/bmjopen-2018-027158
95. Comino EJ, Islam MDF, Tran DT, Jorm L, Flack J, Jalaludin B, et al. Association of processes of primary care and hospitalisation for people with diabetes: a record linkage study. Diabetes Res Clin Pract. (2015) 108:296–305. doi: 10.1016/j.diabres.2015.02.003
96. Wu C, Han X, Yan X, Shang X, Zhang L, He M. Associations between physical activity and cataract treated surgically in patients with diabetes: findings from the 45 and Up Study. Br J Ophthalmol. (2019) 103:1099–105. doi: 10.1136/bjophthalmol-2018-312407
97. Yan X, Han X, Wu C, Keel S, Shang X, Zhang L, et al. Does daily dietary intake affect diabetic retinopathy progression? 10-year results from the 45 and Up Study. Br J Ophthalmol. (2019) 104:1774–80. doi: 10.1136/bjophthalmol-2018-312990
98. Alharbi TJ, Constantino MI, Molyneaux L, Wu T, Twigg SM, Yue DK, et al. Ethnic specific differences in survival of patients with type 2 diabetes: analysis of data collected from an Australian multi-ethnic cohort over a 25 year period. Diabetes Res Clin Pract. (2015) 107:130–8. doi: 10.1016/j.diabres.2014.09.037
99. Stewart Williams J, Ling R, Searles AM, Doran CM, Byles J. Identification of higher hospital costs and more frequent admissions among mid-aged Australian women who self-report diabetes mellitus. Maturitas. (2016) 90:58–63. doi: 10.1016/j.maturitas.2016.04.008
100. Comino EJ, Harris MF, Islam MD, Tran DT, Jalaludin B, Jorm L, et al. Impact of diabetes on hospital admission and length of stay among a general population aged 45 year or more: a record linkage study. BMC Health Serv Res. (2015) 15:12. doi: 10.1186/s12913-014-0666-2
101. Wu C, Han X, Yan X, Keel S, Shang X, Zhang L, et al. Impact of diet on the incidence of cataract surgery among diabetic patients: findings from the 45 and Up Study. Curr Eye Res. (2019) 44:385–92. doi: 10.1080/02713683.2018.1548024
102. Cheung NW, Jiang S, Athayde N. Impact of the IADPSG criteria for gestational diabetes, and of obesity, on pregnancy outcomes. Aust N Z J Obstet Gynaecol. (2018) 58:553–9. doi: 10.1111/ajo.12772
103. Dennis S, Taggart J, Yu H, Jalaludin B, Harris MF, Liaw ST. Linking observational data from general practice, hospital admissions and diabetes clinic databases: can it be used to predict hospital admission? BMC Health Serv Res. (2019) 19:526. doi: 10.1186/s12913-019-4337-1
104. Kelty EA, Tran D, Atkinson AA, Preen DB, Havard A. Maternal and neonatal health outcomes associated with the use of gliclazide and metformin for the treatment of diabetes in pregnancy: a record linkage study. Diabetes Technol Ther. (2019) 17:96–102. doi: 10.1089/dia.2019.0241
105. Khambalia AZ, Ford JB, Nassar N, Shand AW, McElduff A, Roberts CL. Occurrence and recurrence of diabetes in pregnancy. Diabetic Med. (2013) 30:452–6. doi: 10.1111/dme.12124
106. Shand AW, Bell JC, McElduff A, Morris J, Roberts CL. Outcomes of pregnancies in women with pre-gestational diabetes mellitus and gestational diabetes mellitus; a population-based study in New South Wales, Australia, 1998–2002. Diabetic Med. (2008) 25:708–15. doi: 10.1111/j.1464-5491.2008.02431.x
107. Algert CS, McElduff A, Morris JM, Roberts CL. Perinatal risk factors for early onset of Type 1 diabetes in a 2000-2005 birth cohort. Diabetic Med. (2009) 26:1193–7. doi: 10.1111/j.1464-5491.2009.02878.x
108. Ampt A, van Gemert T, Craig ME, Donaghue KC, Lain SB, Nassar N. Using population data to understand the epidemiology and risk factors for diabetic ketoacidosis in Australian children with type 1 diabetes. Pediatr Diabetes. (2019) 20:901–8. doi: 10.1111/pedi.12891
109. Comino EJ, Tran DT, Haas M, Flack J, Jalaludin B, Jorm L, et al. Validating self-report of diabetes use by participants in the 45 and Up Study: a record linkage study. BMC Health Serv Res. (2013) 13:481. doi: 10.1186/1472-6963-13-481
110. Tran DT, Jorm LR, Havard A, Harris MF, Comino EJ. Variation in the use of primary care services for diabetes management according to country of birth and geography among older Australians. Prim Care Diabetes. (2016) 10:66–74. doi: 10.1016/j.pcd.2015.07.001
111. Ha NT, Harris M, Preen D, Moorin R. Time protective effect of contact with a general practitioner and its association with diabetes-related hospitalisations: a cohort study using the 45 and Up Study data in Australia. BMJ Open. (2020) 10:e032790. doi: 10.1136/bmjopen-2019-032790
112. Gibson AA, Humphries J, Gillies M, Nassar N, Colagiuri S. Adherence to eye examination guidelines among individuals with diabetes: an analysis of linked health data. Clin Exp Ophthalmol. (2020) 48:1229–38. doi: 10.1111/ceo.13830
113. Zhang H, Rogers K, Sukkar L, Jun M, Kang A, Young T, et al. Prevalence, incidence and risk factors of diabetes in Australian adults aged ≥45 years: a cohort study using linked routinely-collected data. J Clin Transl Endocrinol. (2020) 22:100240. doi: 10.1016/j.jcte.2020.100240
114. Sukkar L, Kang A, Hockham C, Young T, Jun M, Foote C, et al. Incidence and associations of chronic kidney disease in community participants with diabetes: a 5-year prospective analysis of the EXTEND45 study. Diabetes Care. (2020) 43:982–90. doi: 10.2337/dc19-1803
115. Gibson OR, Segal L, McDermott RA A. simple diabetes vascular severity staging instrument and its application to a Torres Strait Islander and Aboriginal adult cohort of north Australia. BMC Health Serv Res. (2012) 12:185. doi: 10.1186/1472-6963-12-185
116. Chamberlain C, Fredericks B, McLean A, Oldenburg B, Mein J, Wolfe R. Associations with low rates of postpartum glucose screening after gestational diabetes among Indigenous and non-Indigenous Australian women. Aust N Z J Public Health. (2015) 39:69–76. doi: 10.1111/1753-6405.12285
117. Clarke P, Kelman C, Colagiuri S. Factors influencing the cost of hospital care for people with diabetes in Australia. J Diabetes Complications. (2006) 20:349–55. doi: 10.1016/j.jdiacomp.2005.08.004
118. Chamberlain C, Fredericks B, McLean A, Davis B, Eades S, Stewart K, et al. Gestational diabetes mellitus in Far North Queensland, Australia, 2004 to 2010: midwives' perinatal data most accurate source. Aust N Z J Public Health. (2013) 37:556–61. doi: 10.1111/1753-6405.12148
119. Li M, McDermott R. High absolute risk of severe infections among Indigenous adults in rural northern Australia is amplified by diabetes - a 7 year follow up study. J Diabetes Complications. (2016) 30:1069–73. doi: 10.1016/j.jdiacomp.2016.04.022
120. Zhang J, Donald M, Baxter KA, Ware RS, Burridge L, Russell AW, et al. Impact of an integrated model of care on potentially preventable hospitalizations for people with Type 2 diabetes mellitus. Diabetic Med. (2015) 32:872–80. doi: 10.1111/dme.12705
121. Chamberlain C, McLean A, Oats J, Oldenburg B, Eades S, Sinha A, et al. Low rates of postpartum glucose screening among indigenous and non-indigenous women in Australia with gestational diabetes. Matern Child Health J. (2015) 19:651–63. doi: 10.1007/s10995-014-1555-3
122. Chamberlain CR, Wilson AN, Amir LH, O'Dea K, Campbell S, Leonard D, et al. Low rates of predominant breastfeeding in hospital after gestational diabetes, particularly among Indigenous women in Australia. Aust N Z J Public Health. (2017) 41:144–50. doi: 10.1111/1753-6405.12629
123. Campbell SK, Lynch J, Esterman A, McDermott R. Pre-pregnancy predictors of diabetes in pregnancy among Aboriginal and Torres Strait Islander women in North Queensland, Australia. Matern Child Health J. (2012) 16:1284–92. doi: 10.1007/s10995-011-0889-3
124. Chamberlain CR, Oldenburg B, Wilson AN, Eades SJ, O'Dea K, Oats JJ, et al. Type 2 diabetes after gestational diabetes: greater than fourfold risk among Indigenous compared with non-Indigenous Australian women. Diabetes Metab Res Rev. (2016) 32:217–27. doi: 10.1002/dmrr.2715
125. Afzali HHA, Gray J, Beilby J, Holton C, Banham D, Karnon J, et al. risk-adjusted economic evaluation of alternative models of involvement of practice nurses in management of type 2 diabetes. Diabetic Med. (2013) 30:855–63. doi: 10.1111/dme.12195
126. Begum M, Pilkington R, Chittleborough C, Lynch J, Penno M, Smithers L. Caesarean section and risk of type 1 diabetes: whole-of-population study. Diabetic Med. (2019) 36:1686–93. doi: 10.1111/dme.14131
127. Abdelhamid YA, Plummer MP, Finnis ME, Biradar V, Bihari S, Kar P, et al. Long-term mortality of critically ill patients with diabetes who survive admission to the intensive care unit. Crit Care Resusc. (2017) 19:303–9. Available online at: https://search.informit.org/doi/pdf/10.3316/informit.275220376490335
128. Sharpe PB, Chan A, Haan EA, Hiller JE. Maternal diabetes and congenital anomalies in South Australia 1986-2000: a population-based cohort study. Birth Defects Res A Clin Mol Teratol. (2005) 73:605–11. doi: 10.1002/bdra.20172
129. Boyle DIR, Versace VL, Dunbar JA, Scheil W, Janus E, Oats JJN, et al. Results of the first recorded evaluation of a national gestational diabetes mellitus register: challenges in screening, registration, and follow-up for diabetes risk. PloS ONE. (2018) 13:e0200832. doi: 10.1371/journal.pone.0200832
130. Begum M, Chittleborough C, Pilkington R, Mittinty M, Lynch J, Penno M, et al. Incidence of type 1 diabetes by socio-demographic characteristics among South Australian children: whole-of-population study. J Paediatr Child Health. (2020) 56:1952–8. doi: 10.1111/jpc.15104
131. Begum M, Pilkington RM, Chittleborough CR, Lynch JW, Penno M, Smithers LG. Effect of maternal smoking during pregnancy on childhood type 1 diabetes: a whole-of-population study. Diabetologia. (2020) 63:1162–73. doi: 10.1007/s00125-020-05111-w
132. Stone CA, McLachlan KA, Halliday JL, Wein P, Tippett C. Gestational diabetes in Victoria in 1996: incidence, risk factors and outcomes. Med J Aust. (2002) 177:486–91. doi: 10.5694/j.1326-5377.2002.tb04916.x
133. White M, Sabin MA, Magnussen CG, O'Connell MA, Colman PG, Cameron F. Long term risk of severe retinopathy in childhood-onset type 1 diabetes: a data linkage study. Med J Aust. (2017) 206:398–401. doi: 10.5694/mja16.00712
134. Abell SK, Suen M, Pease A, Boyle JA, Soldatos G, Regan J, et al. Pregnancy outcomes and insulin requirements in women with type 1 diabetes treated with continuous subcutaneous insulin infusion and multiple daily injections: cohort Study. Diabetes Technol Ther. (2017) 19:280–7. doi: 10.1089/dia.2016.0412
135. Abell SK, Teede HJ. The IADPSG diagnostic criteria identify women with increased risk of adverse pregnancy outcomes in Victoria. Aust N Z J Obstet Gynaecol. (2017) 57:564–8. doi: 10.1111/ajo.12676
136. Zhao Y, Connors C, Lee AH, Liang W. Relationship between primary care visits and hospital admissions in remote indigenous patients with diabetes: a multivariate spline regression model. Diabetes Res Clin Pract. (2015) 108:106–12. doi: 10.1016/j.diabres.2015.01.013
137. Karmel R. Data Linkage Protocols Using a Statistical Linkage Key: AIHW Cat. no CS1. Canberra: Australian Institute of Health and Welfare. (2005).
138. Harron K, Dibben C, Boyd J, Hjern A, Azimaee M, Barreto ML, et al. Challenges in administrative data linkage for research. Big Data Soc. (2017) 4:2053951717745678. doi: 10.1177/2053951717745678
139. Neville SE, Taylor LK, Moore H, Madden R, Ring I, Pulver LJ, et al. Using linkage between hospital and ABS mortality data to enhance reporting of deaths among Aboriginal and Torres Strait Islander peoples. Aust N Z J Public Health. (2011) 35:543–8. doi: 10.1111/j.1753-6405.2011.00738.x
140. Rowe SL, Cowie BC. Using data linkage to improve the completeness of Aboriginal and Torres Strait Islander status in communicable disease notifications in Victoria. Aust N Z J Public Health. (2016) 40:148–53. doi: 10.1111/1753-6405.12434
141. Shiff NJ, Jama S, Boden C, Lix LM. Validation of administrative health data for the pediatric population: a scoping review. BMC Health Serv Res. (2014) 14:1–7. doi: 10.1186/1472-6963-14-236
142. Colvin L, Slack-Smith L, Stanley FJ, Bower C. Pharmacovigilance in pregnancy using population-based linked datasets. Pharmacoepidemiol Drug Saf. (2009) 18:211–25. doi: 10.1002/pds.1705
143. Gavrielov-Yusim N, Friger M. Use of administrative medical databases in population-based research. J Epidemiol Community Health. (2014) 68:283–7. doi: 10.1136/jech-2013-202744
144. Burgess CL, Holman CDAJ, Satti AG. Adverse drug reactions in older Australians, 1981–2002. Med J Aust. (2005) 182:267–70. doi: 10.5694/j.1326-5377.2005.tb06698.x
145. Grzeskowiak LE, Gilbert AL, Morrison JL. Conception and beyond: using population-based record linkage to monitor long-term effects of medications used during pregnancy. J Pharm Pract Res. (2010) 40:46–9. doi: 10.1002/j.2055-2335.2010.tb00725.x
Keywords: data linkage, record linkage, linked data, linked records, medical record linkage, diabetes, Australia
Citation: Dinh TTN, Cox IA, de Graaff B, Campbell JA, Stokes B and Palmer AJ (2022) A Comprehensive Systematic Review of Data Linkage Publications on Diabetes in Australia. Front. Public Health 10:757987. doi: 10.3389/fpubh.2022.757987
Received: 13 August 2021; Accepted: 25 April 2022;
Published: 25 May 2022.
Edited by:
Amanuel Tesfay Gebremedhin, Curtin University, AustraliaReviewed by:
Amanuel Tesfay Gebremedhin, Curtin University, AustraliaMichael Goldacre, University of Oxford, United Kingdom
Copyright © 2022 Dinh, Cox, de Graaff, Campbell, Stokes and Palmer. This is an open-access article distributed under the terms of the Creative Commons Attribution License (CC BY). The use, distribution or reproduction in other forums is permitted, provided the original author(s) and the copyright owner(s) are credited and that the original publication in this journal is cited, in accordance with accepted academic practice. No use, distribution or reproduction is permitted which does not comply with these terms.
*Correspondence: Andrew J. Palmer, YW5kcmV3LnBhbG1lckB1dGFzLmVkdS5hdQ==