- 1School of Finance and Economics, Jiangsu University, Zhenjiang, China
- 2School of Management, Jiangsu University, Zhenjiang, China
As an emerging form of medical organization, Chinese mobile healthcare (mHealth) platforms are inherently linked to the continuous use of users, which depends on the quality of the health information provided. However, improving the health information quality of mHealth platforms is still a problem that needs to be studied and solved in order to make the platforms sustainable. Based on the reputation mechanism, this study creates a behavioral evolutionary game model for health information providers (physicians) and managers (mHealth platforms), explores the evolution process and evolutionarily stable strategy of the behaviors in various situations, and uses numerical simulation technology to analyze mHealth platforms' constraints and the influencing factors of health information quality. This study presents three key findings. First, considering reputation, health information managers and mHealth platform providers should not unilaterally optimize health information. Instead, mHealth platforms should have active quality control, and physician groups should provide high-quality health information, which is the ideal evolution of the model. Second, the rewards that physicians receive from patients and mHealth platforms for providing quality health information, the reputation benefits, the penalties that physicians suffer for providing low-quality health information, and the increased probability and cost of rent-seeking behavior that physicians may choose can effectively promote the choice of physicians to provide high-quality health information. Third, the reputation gain of mHealth platforms, the probability of mHealth platforms being exposed, and increased losses suffered from exposure can effectively promote the choice of mHealth platforms to control the quality of health information. This study can provide a theoretical basis for mHealth platforms' health information quality control, which is conducive to the healthy and sustainable development of mHealth platforms and the improvement of user satisfaction.
1. Introduction
In the context of an increasingly aging population, the overall shortage and inefficient allocation of medical resources is an important issue, and people's awareness of healthcare has grown. This has led to the emergence of a large number of mobile healthcare (mHealth) platforms, developed in conjunction with 5G networks and smartphones. Currently, there are many concepts of mHealth. According to the World Health Organization (WHO), mHealth is defined as “the use of mobile wireless technologies for health” (1). According to a WHO's study, mHealth provides medical services and information via mobile communication technology. Specifically, in the mobile Internet field, it is a doctor–patient platform based on medical and health applications (apps) in mobile terminal systems, such as Ping An Good Doctor and Chunyu Doctor, in China. These platforms provide a new way for patients to access health information and for physicians to provide health information to the public (2). Its use can enhance access to healthcare and improve the health status of individuals (3). According to Media Research, in 2020, the number of China's mHealth users reached 661 million, and the size of China's mHealth market reached 54.47 billion yuan. mHealth has advantages that traditional healthcare cannot match, being able to overcome space, time, and even organizational barriers (4–6). It offers patients convenient, economical, and personalized access to medical services (such as appointment registration, online consultation, self-diagnosis, etc.), as well as health information (such as popular science articles, popular science videos, classic cases, etc.), which has great potential to improve the health of chronic disease patients (7–9). mHealth platforms are increasingly being seen as an innovative solution to the medical difficulties of 1.3 billion Chinese people, bringing tremendous value to hospital systems, patients, and government supervision, and potentially contributing to the development of the medical sector in the rest of the world (10).
However, mHealth platforms, as an emerging form of medical organizations, have also encountered many problems in the process of their rapid development. mHealth platforms are not well-structured, doctors' and patients' information is not symmetrical, and the participation rate of doctors is low (11). There is a general concern about the quality of health information available on mHealth platforms (12). A large amount of distorted health information can easily mislead the subjective perception of users, thus failing to meet their needs. For example, mHealth platforms can recklessly exaggerate certain health products, present so-called famous doctors, and pose a threat to patients' privacy. Online health information sources are scattered, carriers are diverse, and standards are lacking, which presents users with great challenges regarding inquiries regarding, and the selection of, health information, potentially even posing a threat to their lives. The sustainable and healthy development of mHealth platforms requires users' continuous use, which in turn requires these platforms to meet the needs of users, while the quality of health information on the platforms directly affects the continuous use of users. The quality of health information on these platforms is influenced by the behavior of the providers (physicians) and managers (mHealth platforms) of health information. The sustainability of mHealth platforms depends on the dynamic balance between the interests of health information providers and managers.
Therefore, how to optimize and improve the quality of health information on mHealth platforms so as to increase user satisfaction, increase user stickiness, achieve the dynamic balance of health information providers and managers, and ensure the sustainable development of mHealth platforms has become a major issue in mHealth services. The present study considers the reputation mechanism and constructs a game model for the behavioral evolution of health information providers (physicians) and managers (mHealth platforms). The main contributions of this study are as follows: First, it innovatively studies the sustainable development of mHealth platforms from the perspective of health information quality. Second, as opposed to previous studies that have analyzed the quality of health information from the perspective of the content, this study examines quality from the perspective of health information providers and managers. Third, this study introduces the reputation mechanism to reveal the impact of reputation on the quality of health information on mHealth platforms.
The remainder of the study is divided into the following sections: Section 2 reviews the literature; Section 3 describes the evolution of the game model construction and details the stability analysis; Section 4 discusses the simulation results; Section 5 describes this study's contributions and limitations.
2. Literature review
2.1. Mobile healthcare platforms
The rapid growth of mHealth platforms has entailed increasing research interest, with the debate regarding its advantages and disadvantages seemingly never-ending (13), while also being considered an important tool to promote the future of healthcare services (14). Using online health services provided by mobile platforms can improve users' health literacy and health self-management (15–17). The application of mHealth platforms can achieve the rational allocation of medical resources and improve the quality of medical services (18), thus realizing the rapid flow of medical information and the sharing of medical resources. The use of mHealth platforms by doctors and patients is a novel and effective method for tracking and managing chronic diseases (19). College students use mHealth platforms to find health-related information, including how to lose or control their weight (20) and to monitor their diet (21). Using online healthcare platforms, healthcare providers can provide many forms of professional support (22), especially in terms of professional health information (23). The focus of mHealth platform research has shifted to the platform-based medical health space, and the discussion has begun to focus on how to integrate the various elements and how to facilitate the development of mHealth platforms by coordinating the relationships between the players (10).
2.2. Health information quality
Regarding whether platforms will be able to attract and retain more users, the key question is whether they can provide high-quality health information. Quality health information needs to meet two criteria (24). First, it needs to be of high quality (25, 26). Second, the writing needs to be clear (27). Barry (28) was the first to explore a user-generated index system for evaluating the quality of web information. Eysenbach et al. (29) studied the literature related to the quality of health information on the Internet up to 2001 and derived the most commonly used criteria for evaluating the quality of health information on websites. These include accuracy, completeness, readability, site design, disclosure, and the reference(s) provided. However, the findings and conclusions concerning health-related websites vary widely due to differences in research methods, quality standards, study populations, and topic selection. Therefore, it is necessary to define a quality standard for health information. Zhang et al. (30) systematically analyzed 165 articles related to the quality of online health information from 2002 to 2013 and found that researchers typically evaluated quality in terms of content, format, and technical platform design. The quality of health information varies across medical fields and different websites, and overall information quality remains problematic. Future research needs to examine the quality of user-generated content and incorporate new media to facilitate consumer assessment of health information. Ewington et al. (31) systematically evaluated online health information regarding childbirth in terms of credibility, accuracy, readability, and content quality, and found that quality varied. Government websites have been found to have higher overall quality scores than non-government and commercially funded websites (32, 33), although there are significant differences between countries.
In terms of evaluating the quality of online health information, some scholars have used self-developed evaluation criteria, while others have directly used evaluation tools to rate the content attributes of health information. There are several popular tools for evaluating health websites, including the HON Code of Conduct for medical and health websites, issued by the Health on the Net Foundation (34); the DISCERN instrument, developed by the British Library at the University of Oxford and funded by the British National Health Service Executive Research and Development Program (35); the LIDA instrument, developed by Minervation in the UK healthcare field (36); and the JAMA instrument, created for the World Wide Web as a medical information delivery tool (37). In many cases, evaluation tools have been developed based on medical experts' input, which does not fully meet the needs of information users. Therefore, it is necessary to test the validity of the evaluation tools. It is generally agreed that online health information is poor in quality, so it is urgent that it should be improved. However, current research has mainly evaluated the quality of online health information, which is part of post-event supervision, lagging behind actual development, thereby posing a great danger in terms of faking health information. As a result, a monitoring mechanism for online health information is urgently needed, as well as a supervisory function before, during, and after the event.
2.3. Reputation mechanism
The reputation mechanism plays a central role in human societies and has aroused widespread interest in the realm of evolutionary game theory (38, 39). Reputation is seen as an important factor in building partnerships (40–42). Several behavioral experimental studies have demonstrated the important role of reputation in promoting cooperation (43). It is possible to use reputation as a signal to reveal the right direction for consumers, to reduce the degree of information asymmetry and the risk of adverse selection, to positively motivate the performance of physicians, and to improve the social influence and performance of platforms. Reputation enhancement can promote health knowledge sharing (44). The number of doctors' appointments is positively correlated with their reputation (45). Individuals with good reputations are able to earn more and they can influence the decisions of others, effectively promoting cooperation (46).
Providing quality health information on mHealth platforms is primarily the result of repeated games played by the providers and managers of health information content, aiming to maximize their own interests. This is a dynamic evolution relationship and matches the characteristics of the KMRW reputation model proposed by Kreps and Wilson (47). Thus, game theory is the most logical and explanatory analytical tool for analyzing reputation issues, and it also provides a quantitative measure of how reputation affects health information quality. Real-time evaluation of reputation levels of health information content providers and managers through a reputation mechanism is more effective than traditional post-event supervision in constraining their behavior and motivating them to provide high-quality services.
In conclusion, research on mHealth, network health information quality, reputation, and other aspects undertaken by domestic and foreign scholars has highlighted the significance of optimizing research on mHealth platforms' health information quality. At present, there are few studies on the quality of health information on mHealth platforms. Unlike previous studies, which have mainly focused on the content of the health information itself, the present study intends to introduce the reputation mechanism from the perspective of health information content providers and managers, construct an evolutionary game model, analyze the influencing factors of health information quality on mHealth platforms and of reputation on the optimization of health information quality, and investigate the sustainable development of mHealth platforms from the perspective of reputation.
3. Research methodology
3.1. Description of the research framework
According to evolutionary game theory, participants with limited rationality are unable to grasp fully their own powerful state due to information asymmetry and cognitive differences, etc., but can adjust their strategies by continuous learning, trial and error, imitation, and summarizing the experience of others. After repeated games, an equilibrium state is finally reached, which is the evolutionarily stable strategy (ESS). Neither providers nor managers of health information content on mHealth platforms are completely rational, and health information is essentially a trustworthy good that is difficult to judge accurately before use. It generally relies on the subjective reputation of information providers for judgment and decision-making. The quality of health information is actually a game between two groups of health information content providers and managers through random pairing and repeatedly adjusting their original strategies to maximize their own interests. This is consistent with the characteristics of evolutionary game theory, and the evolutionary game model can be constructed to analyze the health information quality of mHealth platforms. The framework model of this study was developed as follows:
1. According to the characteristics of health information content providers and managers on mHealth platforms, establish hypotheses and quantify the benefits of their strategies.
2. Construct a game pay-off matrix according to the hypotheses.
3. Analyze the stability of each strategy combination and its stability conditions.
4. Analyze the effect of each parameter change on the ESS.
5. Perform simulation analysis to verify the stability of the strategic combination.
In summary, due to information asymmetry, cognitive differences, and other factors, participants with bounded rationality may not fully understand their own powerful state. However, they may constantly learn, try again, copy, and sum up the experience of others to reach a stable equilibrium state, known as the ESS. The providers and managers of health information on mHealth platforms are not completely rational. Health information is essentially a trust item, which is difficult to evaluate accurately before using it. Usually, judgment and decision-making are based on a provider's subjective reputation (48). Essentially, health information quality is the result of providers and managers of health information content repeatedly playing through random pairings and continually tweaking their original strategies to maximize their own interests. The model developed in this study analyzes how health information quality can be optimized on mHealth platforms.
3.2. Model assumptions
Based on the characteristics of mHealth platform content providers and managers, the following assumptions are made:
3.2.1. Assumption 1
Game subjects and their behavioral strategies. Physicians are the main providers of health information content on mHealth platforms. mHealth platforms, as the main providers and managers of health information content, play a crucial role in the quality of the health information provided. Physicians may spend a lot of time and energy editing their knowledge to publish and share high-quality health information on mHealth platforms, while others may publish and share low-quality health information due to a lack of professional knowledge, poor recognition skills, profit-driven, and limited time and energy. Thus, the physician's strategy choice space is (high quality, low quality). mHealth platforms, as the managers of health information quality, may actively fulfill their social responsibility by investing human, financial, and material resources to analyze, filter, encrypt, and desensitize sensitive information, thus actively controlling health information quality. As a result, the mHealth platform's strategy selection space is (control, not control).
3.2.2. Assumption 2
Probability of adopting behavioral strategies for each subject. The game is asymmetric; in the initial state, the probability that the mHealth platform chooses the control strategy is x (0 ≤ x ≤ 1), and the probability that it chooses the no-control strategy is 1-x. The probability that physicians choose to provide high-quality health information is y (0 ≤ y ≤ 1), and the probability that they choose to provide low-quality health information is 1-y.
3.2.3. Assumption 3
Benefits for health information providers. For physicians providing health information on the mHealth platform, the basic cost is Cd (such as editing their existing knowledge into health science articles, popular science videos, sharing classic cases, answering patients' questions, guiding medical treatment, etc.). The extra effort is Ce (for example, providing more accurate and credible health information). The basic gain obtained by the physician for providing health information is Ed. When providing quality health information, the physician can obtain the reward W given by the patient and the reward M given by the mHealth platform, in addition to the reputation gain (R1). This gain (R1) is influenced by the reputation conversion coefficient α (α ≥ 0). The higher the reputation level of the physician, the greater the conversion factor α, and the greater the reputation benefits. The penalty suffered by the physician for providing low-quality health information when discovered by the health information manager is P. Further, the rent-seeking cost required to pay a gratuity to the manager in order to avoid the penalty may be CR, and the rent-seeking behavior is chosen with probability β (0 < β < 1).
3.2.4. Assumption 4
Benefits for health information managers. The basic benefit of the mHealth platform to control the quality of health information is Ep, and the cost to be paid is Cp. The mHealth platform generates revenue H by providing high-quality health information. A physician's contribution rate r (0 ≤ r ≤ 1), i.e., the rate of income generated by increased investment in physician information quality, impacts this income. Furthermore, the control of the quality of health information by the mHealth platform can also bring about for itself a reputation benefit R2, which is affected by its reputation conversion coefficient η (η ≥ 0). If doctors provide low-quality health information and the mHealth platform does not control it, the platform is exposed with a probability of b (0 ≤ b ≤ 1) and suffers a loss L.
3.3. Model building
Based on the above assumptions, the behavioral choice and game payment matrix for the “provider–manager” of health information on mHealth platforms is constructed (see Table 1).
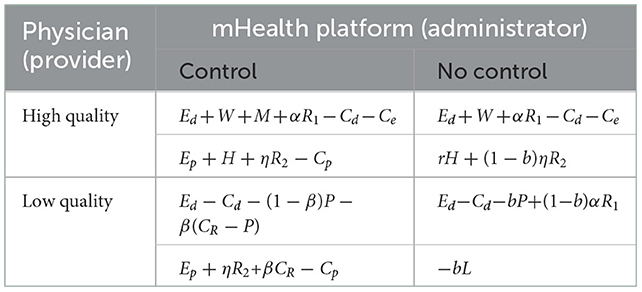
Table 1. Behavioral choices and game payment matrix for health information providers and managers of mHealth platforms.
Let the expected benefit of mHealth platforms choosing to control the quality of health information be Ex:
Let the expected benefit of mHealth platforms choosing not to control the quality of health information be E1-x:
Then, the average expected return of mHealth platforms is :
Similarly, the expected benefits of physicians choosing to provide high-quality health information is Ey:
The expected benefits of physicians choosing to provide low-quality health information is E1-y:
The average expected return for physicians is :
Thus, the replication dynamic equations for the mHealth platform and physician strategy are then obtained:
3.4. Stability analysis of evolutionary strategies
The health information manager–provider game system is obtained from the replicated dynamic equations F(x) andF(y). There are five partial equilibrium points in the plane M = {(x, y)|0 ≤ x ≤ 1, 0 ≤ y ≤ 1}. They are E1(0, 0), E2(0, 1), E3(1, 0), E4(1, 1), and , where:
These equalization points are not necessarily ESSs for the system. ESSs have the ability to prevent invasion and disturbance, as they are subject to bounded rationality that continuously adjusts the strategy according to the vested interests in terms of pursuing their own interests, eventually achieving a dynamic balance. According to the method proposed by Friedman, the stability of the equilibrium point of the evolutionary system of health information managers and providers can be verified by the local stability analysis of the Jacobi matrix of the system based on the replicated dynamic equations F(x) and F(y), and the Jacobi matrix of the system J:
When the equilibrium point satisfies the Jacobi matrix determinants detJ > 0 and trJ < 0, the equilibrium point is replicating the ESS of the dynamical system.
Since the parameter values cannot be determined, the stability of the equilibrium point needs to be further explored in a categorical manner. When conditions satisfy:
and
the following four cases can be classified according to the comparison of the gain conditions at the time of strategy selection between health information providers and managers.
3.4.1. Case 1
When Ep + ηR2 + βCR − Cp > −bL, W − Ce + bαR1 > −bP, and when αR1 + M + W − Ce + β(CR − P) + (1 − β)P > 0, Ep − Cp + (1 − r)H + bηR2 > 0; after the dynamic evolutionary game, the net benefits both of the health information providers and the managers of the mHealth platform are positive, and their evolutionary stability points are E4(1, 1). A physician will provide high-quality health information when the benefits of doing so outweigh the benefits of providing low-quality health information, regardless of whether the mHealth platform controls the quality of health information. In the same way, when controlling the quality of health information is more beneficial than not controlling it, regardless of whether doctors provide high-quality health information, the mHealth platform will decide to control it.
3.4.2. Case 2
When Ep + ηR2 + βCR − Cp > −bL, W − Ce + bαR1 < −bP, and when αR1 + M + W − Ce + β(CR − P) + (1 − β)P > 0, Ep − Cp + (1 − r)H + bηR2 > 0; after the dynamic evolutionary game, the mHealth platform has a positive net benefit and the physician has a negative net benefit. Its evolutionary stability point is E4(1, 1). Physicians commit a lot of time and effort to publishing and sharing high-quality health information when the mHealth platform does not control the quality. If physicians have a good reputation, the system will eventually form a positive relationship. mHealth platforms will eventually reach equilibrium when they control the quality of health information, regardless of whether the physician group provides high-quality information. If doctors initially provide low-quality health information, they will be punished under the condition that the mHealth platform actively monitors the quality of health information, and the punishment is greater than the rent-seeking cost paid by physicians to avoid punishment. Therefore, the system will eventually form a positive relationship by providing high-quality health information.
3.4.3. Case 3
When Ep + ηR2 + βCR − Cp < −bL, W − Ce + bαR1 < −bP, and when αR1 + M + W − Ce + β(CR − P) + (1 − β)P > 0, Ep − Cp + (1 − r)H + bηR2 > 0; after the dynamic evolutionary game, the mHealth platform and the physician both have a negative net benefit. Its evolutionary stability point is E1(0, 0) or E4(1, 1). When physician groups provide low-quality health information, mHealth platforms gain less by controlling the quality of health information than they lose by being exposed when they do not. When mHealth platforms do not control the quality of health information, the benefits of providing high-quality health information from physicians are less than the benefits of being exposed and penalized for providing low-quality health information. In such a situation, the system will eventually evolve into and end up in a prisoner's dilemma. Nevertheless, the interaction between the mHealth platform and doctors will eventually become positive as the platform controls the quality of health information and doctors provide high-quality health information.
3.4.4. Case 4
When Ep + ηR2 + βCR − Cp < −bL, W − Ce + bαR1 > −bP, and when αR1 + M + W − Ce + β(CR − P) + (1 − β)P > 0, Ep − Cp + (1 − r)H + bηR2 > 0; after the dynamic evolutionary game, the mHealth platform has a negative net benefit and the physician has a positive net benefit. Its evolutionary stability point is E4(1, 1). In the case of physician groups providing low-quality health information, the mHealth platform must invest in costs in order to control the quality of that information, resulting in a negative net income for the platform. The system will eventually evolve to a benign state due to the good reputation of the platform. Ultimately, the system will evolve to an equilibrium state if physician groups provide high-quality health information, regardless of whether the mHealth platform controls the quality of health information. This is due to the fact that if the mHealth platform does not control the quality of health information when low-quality health information provided by doctors is exposed, the loss is much greater than the short-term benefits for the platform. In the long run, mHealth platforms will choose to control the quality of health information so that the system will eventually interact in a positive way.
According to the local stability analysis method of the Jacobi matrix, the system equilibrium point (evolutionary stability) results were obtained (see Table 2).
From Table 2, it can be seen that when the reputation mechanism and punishment mechanism are considered, providing high-quality health information from physicians is more beneficial than providing low-quality health information, and controlling the quality of health information is more beneficial than having no control when the health information quality improves. The equilibrium points of system evolution are all in Cases 1–4. In other words, excluding the extra cost of providing high-quality health information, the benefits for physicians (including reputation gains, incentives given to physicians by the platform, and incentives given to physicians by patients) are greater than the penalties suffered by physicians for providing low-quality health information (the rent-seeking costs incurred to avoid the penalties). Further, the benefits of actively controlling the quality of health information by the mHealth platform (including reputation gain) are greater than the opportunity benefits gained from not controlling the quality of health information without exposure (including revenue and reputation benefits to the platform from quality information provided by physicians). In this case, both parties will adopt the strategy of providing high-quality health information and the manager will adopt the strategy of controlling the quality of health information after a period of game play as long as the benefits of one party not controlling the quality of health information are less than the benefits when controlling. The Jacobi matrix determinant columns detJ > 0 and trJ < 0 of the equilibrium points E2(0, 1) and E3(1, 0) are not constant and therefore cannot be an ESS for the system. As a result, health information managers and providers on mHealth platforms unilaterally optimizing the quality of health information is unreliable.
By varying each parameter's size, the evolution phase diagrams of the system are obtained (see Figure 1), allowing a more intuitive understanding of the evolution path.
3.5. Effect of parameter changes on evolutionarily stable strategies
When there are two possible equilibria of the system, changes in the magnitude of different parameter values cause the system to converge to different equilibria, as in Case 3. Both point E1(0, 0) and point E4(1, 1) are ESSs of the system, and whether the system converges to E1(0, 0) or E4(1, 1) requires further analysis depends on the variation of the parameters. From the phase diagram of the system evolution in Case 3 in Figure 1, it is clear that the key to the system evolution results lies in the position where the central point or saddle point E5 is located. The changes of the relevant parameters will lead to the movement of E5, thus playing the role of regulating the evolution direction. The effects of the changes of each parameter on the system evolution results are shown as follows:
(1) Parameter Ce. Ce is the additional cost for physicians to provide quality health information.. As Ce increases, E5 moves horizontally to the right, and the area of region E1E3E5E2 expands. Eventually, the system becomes more likely to converge to E1(0, 0). To put it another way, too much energy spent on providing high-quality health information will negatively affect physicians' enthusiasm, which will harm the system's benign evolution.
(2) Parameter W, M. W is the reward given by the patient when the physician provides quality health information. As W increases, E5 moves horizontally to the left and the area of region E2E5E3E4 expands. Eventually, the system becomes more likely to converge to E4(1, 1), indicating that patient affirmation facilitates physicians to provide quality health information. M is the reward given by the mHealth platform when physicians provide quality health information. As M increases, E5 moves horizontally to the left and the system becomes more likely to converge to E4(1, 1), indicating that incentives on mHealth platforms facilitate physicians' delivery of quality health information. The more rewards health information providers receive, the more conducive they are to the benign evolution of the system.
(3) Parameter R1, α, R2, η. R1 is the reputation gain that physicians receive when they provide quality information. As R1 increases, E5 moves horizontally to the left and the area of region E2E5E3E4 expands. Eventually, the system becomes more likely to converge to E4(1, 1). α is the conversion factor for physicians to provide quality information for reputation gain. As α increases, E5 moves horizontally to the left and the area of region E2E5E3E4 expands. Eventually, the system becomes more likely to converge to E4(1, 1). It can be seen that the parameters R1 and α are changing in the same direction in the system. R2 is the reputation benefit gained when the mHealth platform controls the quality of health information. As R2 increases, E5 moves vertically downward and the area of region E2E5E3E4 expands. Eventually, the system becomes more likely to converge to E4(1, 1).
η is the conversion coefficient of the mHealth platform to control the quality of health information for reputation gain. As η increases, E5 moves vertically downward and the area of region E2E5E3E4 expands, contributing to the benign evolution of the system. It can be seen that the parameters R2 and η are changing in the same direction in the system.
This shows that reputation mechanisms can motivate physicians to provide quality health information and monitor the quality of health information managed by mHealth platforms.
(4) Parameter P, β, CR. P is the penalty that physicians suffer when they provide low-quality health information that is detected by health information managers. When P increases, the value of y* is constant and the value of x* is influenced by the probability β of physicians choosing rent-seeking behavior. When , the value of x* becomes smaller, and E5 shifts horizontally to the left, making the area of the region E2E5E3E4 larger. Thus, the possibility of the system evolving to a stable point becomes greater. When , the change in the value of x* cannot be determined, the impact on the quality of health information on the mobile over medical platform cannot be judged at this time. As β grows, y* becomes smaller, and x* is affected by P and the rent-seeking cost CR.
When , x* becomes smaller, and E5 moves to the lower left corner, thereby expanding the region E2E5E3E4, which is conducive to the benign evolution of the system. When , x* becomes bigger; it is impossible to determine how the area of E2E5E3E4 changes, so the influence of β on the quality of the mHealth platform's health information cannot be judged. As the rent-seeking cost CR increases, the saddle point E5 moves horizontally to the left and vertically downward, and the area E1E3E5E2 where the system converges to the bad mode becomes smaller, which is beneficial to its benign evolution. The greater the rent-seeking cost, the more willing physicians are to provide quality health information.
(5) Parameter Ep, Cp. Ep is the revenue generated by physicians providing high-quality health information to mHealth platforms. As H increases, E5 moves vertically downward and the area of region E2E5E3E4 expands, contributing to the benign evolution of the system.
Ep is the basic benefit of mHealth platforms for controlling the quality of health information. As Ep increases, E5 moves vertically downward and the area of region E2E5E3E4 expands, contributing to the benign evolution of the system. Cp is the cost that mHealth platforms need to pay to control the quality of health information. As Cp increases, E5 moves vertically upward and the area of region E1E3E5E2 expands, thus not contributing to the benign evolution of the system.
(6) Parameter H,r. H is the revenue generated by physicians providing high-quality health information to mHealth platforms. As H increases, E5 moves vertically downward and the area of region E2E5E3E4 expands, contributing to the benign evolution of the system. r is the contribution of physician input. As r increases, E5 moves vertically upward and the area of region E2E5E3E4 expands, thus not being conducive to the benign evolution of the system. This shows that if mHealth platforms are not under control, no matter how much physicians invest, health information cannot be guaranteed to be of high quality.
(7) Parameter b, L. b reflects mHealth platforms choosing not to control the probability of being exposed when physicians provide low-quality health information. As b increases, the saddle point E5 moves horizontally to the left and vertically downward at the same time. The area of region E2E5E3E4 expands, contributing to the benign evolution of the system. L is the loss suffered by mHealth platforms due to the exposure of low-quality health information. As L increases, E5 moves vertically downward and the area of region E2E5E3E4 expands, contributing to the benign evolution of the system.
4. Simulation analysis
For a more intuitive analysis of the dynamic evolution process of the health information providers and managers of mHealth platforms, and to verify that the evolutionary game model above is valid, we use the MATLAB simulation tool to run numerical simulations for different situations and analyze their effects according to the constraints and copy the dynamic equations.
4.1. Case 1
Suppose W = 1, M = 1, α = 2, R1 = 2, Ce = 1, Ep = 1, Cp = 1, H = 1, r = 0.2, R2 = 1, η = 1, b = 0.2, L = 2, β = 0.2, CR = 2, and P = 4; when Ep + ηR2 + βCR − Cp > −bL, W − Ce + bαR1 > −bP and when αR1 + M + W − Ce + β(CR − P) + (1 − β)P > 0, Ep − Cp + (1 − r)H + bηR2 > 0. From Figure 2, it can be seen that the equilibrium point of the game between the health information providers and managers of the mHealth platform is (1, 1). Figure 2A illustrates that as long as the benefit of the mHealth platform controlling the quality of health information is greater than the benefit of not controlling the quality of health information, no matter what percentage of physicians initially choose to provide high-quality health information, health information managers actively monitor the quality of health information. As seen in Figures 2A, B, if the benefits of providing high-quality health information outweigh the benefits of providing low-quality health information, regardless of the proportion of the strategy initially chosen by the mHealth platform to control the quality of health information, physician groups who are rational economic people will actively provide high-quality health information.
4.2. Case 2
Suppose W = 1, M = 1, α = 2, R1 = 2, Ce = 5, Ep = 1, Cp = 1, H = 1, r = 0.2, R2 = 1, η = 1, b = 0.2, L = 2, β = 0.2, CR = 2, and P = 4; when Ep + ηR2 + βCR − Cp > −bL, W − Ce + bαR1 < −bP and when αR1 + M + W− Ce + β(CR − P) + (1 − β)P > 0, Ep − Cp + (1 − r)H + bηR2 > 0. From Figure 3, it can be seen that the equilibrium point of the game between health information providers and managers of the mHealth platform is (1, 1). Figure 3A shows that when the mHealth platform's proportion of strategies to control quality is low, the more physicians choose strategies to provide high-quality health information, and the faster the time spent reaching equilibrium, i.e., the more physicians invest in the system's benign evolution, the more favorable it is for the reputation mechanism of physicians to play a positive role. Figure 3B shows that if the mHealth platform chooses a high proportion of strategies to manage and control the quality of health information, no matter whether the physician group chooses a high or low proportion of strategies, the system will evolve to an equilibrium point. The time to reach the equilibrium point is different, and the punishment mechanism of the mHealth platform is beneficial.
4.3. Case 3
Suppose W = 1, M = 1, α = 2, R1 = 2, Ce = 5, Ep = 1, Cp = 3, H = 3, r = 0.2, R2 = 1, η = 1, b = 0.2, L = 2, β = 0.2, CR = 0.1, and P = 0.04; when Ep + ηR2 + βCR − Cp < −bL, W − Ce + bαR1 < −bP and when αR1 + M + W − Ce + β(CR − P) + (1 − β)P > 0, Ep − Cp + (1 − r)H + bηR2 > 0. As shown in Figure 4A, when the mHealth platform and physician group both have negative net incomes, the equilibrium point between the mHealth platform and manager is (1, 1). When the mHealth platform has a high proportion of strategies to control the quality of health information (x = 0.9, other parameters taken as in Figure 4A, compare Figures 4A,B), it is possible to evolve the system to the equilibrium point (1, 1), which validates the previous theoretical analysis.
Except for these parameters, other parameters take the same values as Figure 4A, and by comparing Figures 4A, 5 it can be seen that when a reputation gain is obtained (R1 = 9), the system can achieve benign evolution even if the mHealth platform does not have a high proportion of strategies to control the quality of health information. Also, the higher the proportion of physicians providing quality information, the shorter the time to converge to the equilibrium state. According to this study, when the control ratio of the mHealth platform is low, the reputation mechanism can positively guide the behavior of health information providers, prompting them to provide high-quality health information, which is conducive to the system's benign evolution. In the system, the parameters R1 and α change in the same direction. When the other parameters are the same as in Figure 4A and α = 9, the dynamic evolution diagram is the same in Figure 5. When the conversion coefficient of high-quality information to obtain reputation benefits increases, it is conducive to the benign evolution of the system. When the conversion coefficient of reputation gain from physicians providing quality information becomes low [e.g., Figure 4A, α = 2], it is not conducive to the benign evolution of the system. However, the quality of health information can still be guaranteed when the control ratio of mHealth platforms is high [e.g., Figure 4B, x = 0.9] and the proportion of physician groups providing quality health information is also high. This shows that when reputation mechanisms play a minor role, it takes the combined efforts of mHealth platforms and physician groups to evolve the system to a benign state.
From Figure 4A, we can see that the role of the reputation mechanism is not significant due to the small reputation gain and reputation conversion coefficient. When the rent-seeking cost of the physician population increases [except for the parameter CR = 2.9, other parameters take the same values as Figure 4A, compare Figures 4A, 6], it is conducive to the benign evolution of the system, indicating that the increase in the rent-seeking cost of physicians is conducive to reducing the rent-seeking behavior of physicians and motivating them to provide quality health information.
It is conducive to the benign evolution of the health information system when physicians provide low-quality health information and are punished for it (except for parameter P = 4, other parameters take the same values as in Figure 4A, compare Figures 4A, 7A). In situations where physicians provide low-quality health information (except for parameter b = 0.45, other parameters take the same values as in Figure 4A, compare Figures 4A, 7B), it is conducive to the system's benign evolution. An increase in the loss suffered by the mHealth platform due to low-quality health information is conducive to a benign evolution of the system (except for the parameter L = 4.85, other parameters take the same values as Figure 4A, compare Figures 4A, 7C), which indicates that a reasonable punishment mechanism is necessary for the optimization of the platform's health information quality.
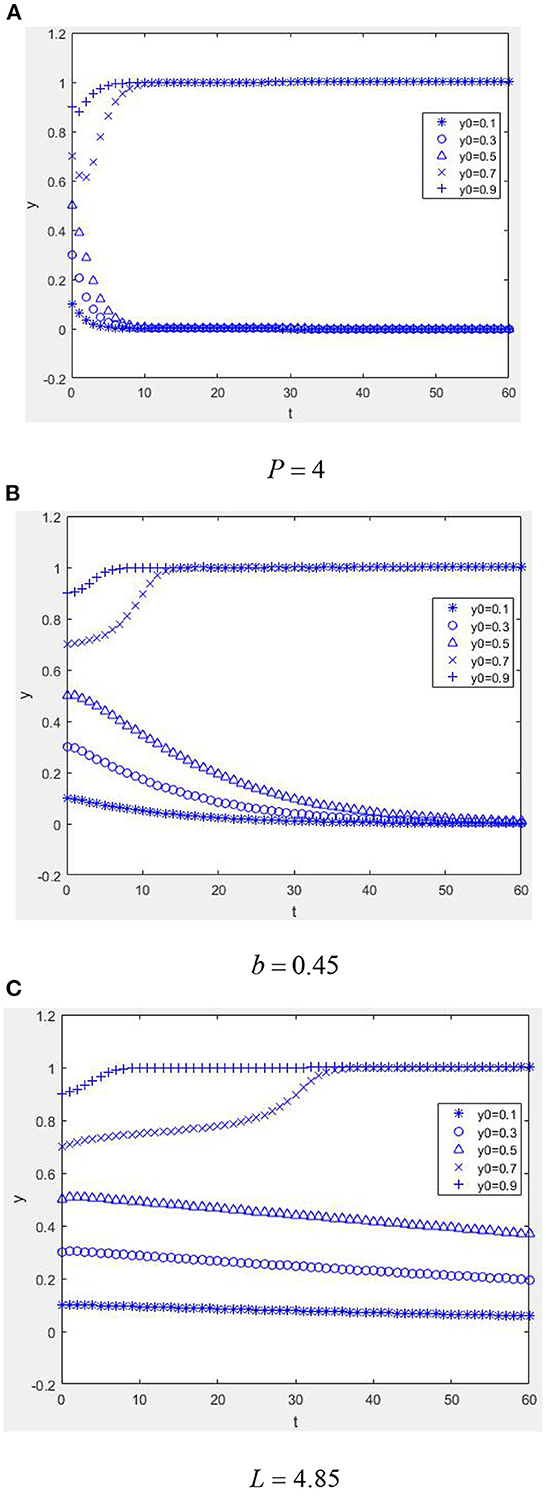
Figure 7. Dynamic evolution diagram under Case 3 (the role of punishment mechanism). (A) P = 4, (B) b = 0.45, and (C) L = 4.85.
4.4. Case 4
Suppose W = 1, M = 1, α = 2, R1 = 2, Ce = 1, Ep = 1, Cp = 3, H = 3, r = 0.2, R2 = 1, η = 1, b = 0.2, L = 2, β = 0.2, CR = 2, and P = 4; when Ep + ηR2 + βCR − Cp < −bL, W − Ce + bαR1 > −bP and when αR1 + M + W − Ce + β(CR − P) + (1 − β)P > 0, Ep − Cp + (1 − r)H + bηR2 > 0. From Figure 8, it can be seen that the equilibrium point of the game between health information providers and managers of the mHealth platform is (1, 1). It is shown in Figure 8A that even if the proportion of physicians providing high-quality health information strategies is low, the system can also evolve benignly if guided by the reputation mechanism of the mHealth platform. The higher the ratio, the quicker the system will converge to equilibrium. Based on Figures 8A, B, it can be seen that when the physician group has a net benefit, the equilibrium state will eventually be reached regardless of how much effort is devoted to controlling the quality of health information by the mHealth platform.
5. Conclusions and suggestions
5.1. Conclusions
We have introduced a behavioral game model between health information content providers and managers on mHealth platforms, examined the evolutionary process and ESS of the behaviors of both sides of the game in different situations, and used numerical simulation technology to analyze the quality of health information on mHealth platforms. The present research shows that it is unstable for managers and providers of health information on mHealth platforms to optimize health information quality unilaterally. The mHealth platform actively controls the quality of health information, and the physician community provides high-quality health information as the ideal evolutionary equilibrium of the model. The main factors that influence behavioral decisions on both sides are the rewards obtained by physicians for providing high-quality health information; physicians' reputation benefits and their conversion coefficient; punishments suffered by physicians; the probability of physicians choosing rent-seeking behavior; costs associated with rent-seeking behavior; physicians' investment contribution rate; the reputation benefits of mHealth platforms; the conversion coefficient of their reputation benefit; the probability of exposure and losses from exposure; the and cost and benefit of optimizing the quality of health information both for mHealth platforms and physicians.
5.2. Suggestions
The above findings have important implications for the improvement and sustainable development of health information quality on mHealth platforms.
First, it is necessary to focus on reputation mechanism construction to improve the effect of reputation incentives. mHealth platforms controlling the quality of health information and physicians providing quality health information can enhance the reputation of both parties. Further, reputation as a potential resource can bring more traffic to the platform and more patients to the physicians, thus creating more potential revenue. Therefore, mHealth platforms should pay attention to the construction of a reputation mechanism and increase the corresponding reputation incentive mechanism, such as guiding patients to actively give positive comments after getting help from physicians, setting a higher reputation conversion coefficient to ensure that physicians with a good reputation can get more benefits, and improving the effect of reputation incentives.
Second, it is necessary to increase the cost of rent-seeking and reduce the opportunity for rent-seeking. Public medical literacy should be improved, and physicians should be encouraged to monitor each other and expose physicians' rent-seeking behavior. Moreover, the relevant management should increase penalties for mHealth platforms that choose to harbor physicians' rent-seeking behavior to increase the cost of rent-seeking for physicians. Corresponding laws and supervision mechanisms should be established and improved to provide easy-to-operate and responsive supervision channels for the public and physicians to monitor health information on mHealth platforms. Eventually, a physician reputation file should be established. Violations such as rent-seeking should be recorded in the physician's reputation file to curb physicians' rent-seeking behavior and minimize opportunities for rent-seeking.
Third, it is necessary to improve the reward and punishment incentive mechanism and establish an information supervision mechanism. It is difficult to curb the rent-seeking behavior of doctors only through unilateral rewards and punishments because health information managers and providers on the mHealth platform are unable to optimize the quality of health information unilaterally. When considering reputation benefits, patients' affirmation of physicians and the mHealth platform's rewards for physicians are conducive to optimizing health information quality. Patients will ask physicians more paid questions, so the physicians will receive more benefits and will be more motivated. An increase in the punishment of doctors for providing inferior information, an increase in mHealth platform exposure, and losses from exposure are all also conducive to optimizing the quality of health information. A third-party quality watchdog can be introduced to ensure the timely exposure of false and misleading health information. Therefore, the reputation gains of physicians and mHealth platforms should be considered, and effective reward and punishment mechanisms and monitoring mechanisms should be developed.
The following limitations of this study require further in-depth exploration. First, the health information quality on the mHealth platform mainly depends on the providers and managers of health information, but the behavior of users of health information also indirectly influences this. Therefore, future research could consider introducing user behavior for a more comprehensive study. Second, this paper only has examined the sustainability of mHealth platforms from a theoretical and health information quality perspective, without analyzing specific cases. In the future, empirical methods can be considered to further explore this topic and improve its quality.
Data availability statement
The original contributions presented in the study are included in the article/Supplementary material, further inquiries can be directed to the corresponding author.
Author contributions
XS: conceptualization, methodology, investigation, validation, software, and writing the original draft. WZ: methodology, formal analysis, writing, reviewing, and editing. YF: writing, reviewing, editing, supervision, and funding acquisition. All authors contributed to the article and approved the submitted version.
Funding
This study was supported by the National Social Science Fund of China (20BTQ047), the Major Project of Philosophy and Social Science Research in Jiangsu Universities (2019SJZDA131), and the Project of Philosophy and Social Science Research in Jiangsu Universities (2018SJA1058).
Acknowledgments
The authors thank the National Social Science Foundation of China and the reviewers for their comments.
Conflict of interest
The authors declare that the research was conducted in the absence of any commercial or financial relationships that could be construed as a potential conflict of interest.
Publisher's note
All claims expressed in this article are solely those of the authors and do not necessarily represent those of their affiliated organizations, or those of the publisher, the editors and the reviewers. Any product that may be evaluated in this article, or claim that may be made by its manufacturer, is not guaranteed or endorsed by the publisher.
Supplementary material
The Supplementary Material for this article can be found online at: https://www.frontiersin.org/articles/10.3389/fpubh.2022.1059252/full#supplementary-material
References
1. World Health Organization. WHO Guideline: Recommendations on Digital Interventions for Health System Strengthening. World Health Organization (2019). Available online at: https://www.who.int/publications/i/item/9789241550505
2. Goh JM, Gao GD, Agarwal R. The creation of social value: can an online health community reduce rural-urban health disparities? MIS Q. (2016) 40:247–63. doi: 10.25300/MISQ/2016/40.1.11
3. Sun L, Buijsen M. Mobile health in China: does it meet availability, accessibility, acceptability and quality standards? Health Policy Technol. (2022) 11:100660. doi: 10.1016/j.hlpt.2022.100660
4. Silva BMC, Rodrigues JJPC, Diez ID, Lopez-Coronado M, Saleem K. Mobile-health: a review of current state in 2015. J Biomed Inform. (2015) 56:265–72. doi: 10.1016/j.jbi.2015.06.003
5. Akter S, Ray P. mHealth –an ultimate platform to serve the unserved. Yearb Med Inform. (2010) 19:94–100. doi: 10.1055/s-0038-1638697
6. Tachakra S, Wang XH, Istepanian RSH, Song YH. Mobile e-health: the unwired evolution of telemedicine. Telemed J e-health. (2003) 9:247–57. doi: 10.1089/153056203322502632
7. Ramirez V, Johnson E, Gonzalez C, Ramirez V, Rubino B, Rossetti G. Assessing the use of mobile health technology by patients: an observational study in primary care clinics. JMIR mhealth uhealth. (2016) 4:508–17. doi: 10.2196/mhealth.4928
8. Escoffery C, McGee R, Bidwell J, Sims C, Thropp EK, Frazier C, et al. A review of mobile apps for epilepsy self-management. Epilepsy Behav. (2018) 81:62–9. doi: 10.1016/j.yebeh.2017.12.010
9. Reychav I, Beeri R, Balapour A, Raban DR, Sabherwal R, Azuri J. How reliable are self-assessments using mobile technology in healthcare? The effects of technology identity and self-efficacy. Comput Hum Behav. (2019) 91:52–61. doi: 10.1016/j.chb.2018.09.024
10. Zheng S, Hakam B, Chen J. A dynamic equilibrium mechanism of core layer interests in the mobile medical platform ecosystem. Appl Bionics Biomech. (2021) 2022:8915055. doi: 10.1155/2022/8915055
11. Cui XM, Yao K, Hu JC. Transaction cost, network value and platform innovation-qualitative analysis based on 38 cases of platform practice. R and D Manage. (2014) 26:22–31.
12. Grundy QH, Wang ZC, Bero LA. Challenges in assessing mobile health app quality : a systematic review of prevalent and innovative methods. Am J Prev Med. (2016) 51:1051–9. doi: 10.1016/j.amepre.2016.07.009
13. Lampariello R, Labrique AB. Digital health: is the glass half-full or half-empty? Health Policy Technol. (2020) 9:266–7. doi: 10.1016/j.hlpt.2020.07.001
14. World Health Organisation. Global Diffusion of eHealth: Making Universal Health Coverage Achievable: Report of the Third Global Survey on eHealth. World Health Organisation (2016). Available online at: https://apps.who.int/iris/handle/10665/252529
15. Mano RS. Social media and online health services: a health empowerment perspective to online health information. Comput Hum Behav. (2014) 39:404–12. doi: 10.1016/j.chb.2014.07.032
16. Bond CS, Merolli M, Ahmed OH. Patient empowerment through social media. In:Shabbir SA, Elia G, Annie YS, , editors. Participatory Health Through Social Media. Academic Press (2016). p. 10–26. doi: 10.1016/B978-0-12-809269-9.00002-5
17. Cerezo PG, Juv'e-Udina ME, Delgado-Hito P. Concepts and measures of patient empowerment: a comprehensive review. Rev Escola Enfermagem USP. (2016) 50:664–71. doi: 10.1590/S0080-623420160000500018
18. Miao Y, Cui T, Jiang B. Research on service process design of mobile medical platform based on patient's emotional demand. Lect Notes Comput Sci. (2018) 10920:41–51. doi: 10.1007/978-3-319-91806-8_4
19. Lv Q, Jiang Y, Qi J, Zhang YL, Zhang X, Fang LK, et al. Using mobile apps for health management: a new health care mode in China. JMIR mHealth uHealth. (2019) 7:e10299. doi: 10.2196/10299
20. Choi W, Stvilia B. How do college students choose mobile health/wellness applications? Proc ASIST Ann Meet. (2014) 51:1–4. doi: 10.1002/meet.2014.14505101115
21. Slazus C, Ebrahim Z, Koen N. Mobile health apps: an assessment of needs, perceptions, usability, and efficacy in changing dietary choices. Nutrition. (2022) 101:111690. doi: 10.1016/j.nut.2022.111690
22. Brickwood KJ, Ahuja KDK, Watson G, O'Brien JA, Williams AD. Effects of activity tracker use with health professional support or telephone counseling on maintenance of physical activity and health outcomes in older adults: randomized controlled trial. JMIR mHealth uHealth. (2021) 9:e18686. doi: 10.2196/18686
23. Zhang X, Guo F, Xu T, Li Y. What motivates physicians to share free health information on online health platforms? Inform Process Manage. (2020) 57:102166. doi: 10.1016/j.ipm.2019.102166
24. Chu SKW, Huang H, Wong WNM, van Ginneken WF, Wu KM, Hung MY. Quality and clarity of health information on Q&A sites. Lib Inform Sci Res. (2018) 40:237–44. doi: 10.1016/j.lisr.2018.09.005
25. Adams SA. Revisiting the online health information reliability debate in the wake of “Web 2.0”: an inter-disciplinary literature and website review. Int J Med Inform. (2010) 79:391–400. doi: 10.1016/j.ijmedinf.2010.01.006
26. Williams P, Nicholas D, Huntington P, McLean F. Surfing for health: user evaluation of a health information website. Part one: background and literature review. Health Inform Lib J. (2002) 19:98–108. doi: 10.1046/j.1471-1842.2002.00374_2.x
27. Rien SY, Danielson DR. Credibility: a multidisciplinary framework. Ann Rev Inform Sci Technol. (2007) 41:307–64. doi: 10.1002/aris.2007.1440410114
28. Barry CL. Use-defined relevance criteria: an exploratory study. J Am Soc Inform Sci. (1994) 45:149–159. doi: 10.1002/(SICI)1097-4571(199404)45:3<149::AID-ASI5>3.0.CO;2-J
29. Eysenbach G, Powell J, Kuss O, Sa ER. Empirical studies assessing the quality of health information for consumers on the world wide web: a systematic review. J Am Med Assoc. (2002) 287:2691–700. doi: 10.1001/jama.287.20.2691
30. Zhang Y, Sun Y, Xie B. Quality of health information for consumers on the web: a systematic review of indicators, criteria, tools, and evaluation results. J Assoc Inform Sci Technol. (2015) 66:2071–84. doi: 10.1002/asi.23311
31. Ewington LJ, Vanes NK, Dewdney J, Al Wattar BH, Quenby S. Online health information on induction of labour: a systematic review and quality assessment study. Eur J Obstet Gynecol Reprod Biol. (2022) 271:177–82. doi: 10.1016/j.ejogrb.2022.02.010
32. Ng JY, Zhang CJ, Ahmed S. Dietary and herbal supplements for fatigue: a quality assessment of online consumer health information. Integrat Med Res. (2021) 10:100749. doi: 10.1016/j.imr.2021.100749
33. Sowter J, Astin F, Dye L, Marshall P, Knapp P. Assessment of the quality and content of website health information about herbal remedies for menopausal symptoms. Maturitas. (2016) 88:16–22. doi: 10.1016/j.maturitas.2016.02.016
34. Boyer C, Selby M, Scherrer JR, Appel RD. The health on the net code of conduct for medical and health websites. Comput Biol Med. (1998) 28:603–10. doi: 10.1016/S0010-4825(98)00037-7
35. Charnock D, Shepperd S, Needham G, Gann R. DISCERN:an instrument for judging the quality of written consumer health information on treatment choices. J Epidemiol Community Health. (1999) 53:105–11. doi: 10.1136/jech.53.2.105
36. Tavare AN, Alsafi A, Hamady MS. Analysis of the quality of information obtained about uterine artery embolization from the internet. Cardiovasc Interv Radiol. (2012) 35:1355–62. doi: 10.1007/s00270-012-0345-9
37. Silberg WM, Lundberg GD, Musacchio RA. Assessing, controlling, and assuring the quality of medical information on the internet: caveant lector et viewor-let the reader and viewer beware. J Am Med Assoc. (1997) 277:1244–5. doi: 10.1001/jama.277.15.1244
38. Milinski M, Semmann D, Krambeck HJ. Reputation helps solve the 'tragedy of the commons'. Nature. (2002) 415:424–6. doi: 10.1038/415424a
39. Fehr E. Human behaviour: don't lose your reputation. Nature. (2004) 432:449–50. doi: 10.1038/432449a
40. Fu F, Hauert C, Nowak MA, Wang L. Reputation-based partner choice promotes cooperation in social networks. Phys Rev. (2008) 78:026117. doi: 10.1103/PhysRevE.78.026117
41. Fu F, Wu T, Wang L. Partner switching stabilizes cooperation in coevolutionary prisoner's dilemma. Phys Rev. (2009) 79:036101. doi: 10.1103/PhysRevE.79.036101
42. Li Y. The evolution of reputation-based partner-switching behaviors with a cost. Sci Rep. (2014) 4:5957. doi: 10.1038/srep05957
43. Milinski M, Semmann D, Krambeck HJ, Marotzke J. Stabilizing the earth's climate is not a losing game: supporting evidence from public goods experiments. Proc Natl Acad Sci USA. (2006) 103:3994–8. doi: 10.1073/pnas.0504902103
44. Yan ZJ, Wang TM, Chen Y, Zhang H. Knowledge sharing in online health communities: a social exchange theory perspective. Inform Manage. (2016) 53:643–53. doi: 10.1016/j.im.2016.02.001
45. Liu XX, Guo XT, Wu H, Wu TS. The impact of individual and organizational reputation on physicians' appointments online. Int J Electro Comm. (2016) 20:551–77. doi: 10.1080/10864415.2016.1171977
46. Quan J, Zhou Y, Wang X, Yang JB. Information fusion based on reputation and payoff promotes cooperation in spatial public goods game. Appl Math Comput. (2020) 368:124805. doi: 10.1016/j.amc.2019.124805
47. Kreps DM, Wilson R. Reputation and imperfect information. J Econ Theory. (1982) 27:253–79. doi: 10.1016/0022-0531(82)90030-8
Keywords: sustainability, health information quality, evolutionary game theory, reputation mechanism, mobile healthcare platforms
Citation: Sun XY, Zhou WJ and Feng Y (2023) Mobile healthcare platforms' sustainability: The perspective of health information quality. Front. Public Health 10:1059252. doi: 10.3389/fpubh.2022.1059252
Received: 16 October 2022; Accepted: 08 December 2022;
Published: 06 January 2023.
Edited by:
Monica Lorena Sanchez Limon, Autonomous University of Tamaulipas, MexicoReviewed by:
Hector Aguirre, Universidad Autónoma de Tamaulipas, MexicoCesar Ivan Mellado Ibarra, Universidad Autónoma de Tamaulipas, Mexico
Copyright © 2023 Sun, Zhou and Feng. This is an open-access article distributed under the terms of the Creative Commons Attribution License (CC BY). The use, distribution or reproduction in other forums is permitted, provided the original author(s) and the copyright owner(s) are credited and that the original publication in this journal is cited, in accordance with accepted academic practice. No use, distribution or reproduction is permitted which does not comply with these terms.
*Correspondence: Xiaoyang Sun, c3Vubnlfc3VuQHVqcy5lZHUuY24=