- 1Military Medical Psychology School, Fourth Military Medical University, Xi'an, China
- 2BrainPark, Turner Institute for Brain and Mental Health and School of Psychological Sciences, Monash University, Clayton, VI, Australia
- 3Department of Developmental Psychology for Armyman, School of Medical Psychology, Army Medical University, Chongqing, China
Background: The relationship between different dimensions of empathy and individual symptoms of depression during the COVID-19 pandemic remains unclear, despite the established link between empathy and depression. The network analysis offers a novel framework for visualizing the association between empathy and depression as a complex system consisting of interacting nodes. In this study, we investigated the nuanced associations between different dimensions of empathy and individual symptoms of depression using a network model during the pandemic.
Methods: 1,177 students completed the Chinese version of the Interpersonal Reactivity Index (IRI), measuring dimensions of empathy, and the Chinese version of the Patient Health Questionnaire-9 (PHQ-9), measuring symptoms of depression. First, we investigated the nuanced associations between different dimensions of empathy and individual depressive symptoms. Then, we calculated the bridge expected influence to examine how different dimensions of empathy may activate or deactivate the symptoms of depression cluster. Finally, we conducted a network comparison test to explore whether network characteristics such as empathy-depression edges and bridge nodes differed between genders.
Results: First, our findings showed that personal distress was positively linked to symptoms of depression. These symptoms involved psychomotor agitation or retardation (edge weight = 0.18), sad mood (edge weight = 0.12), trouble with concentrating (edge weight = 0.11), and guilt (edge weight = 0.10). Perspective-taking was found to be negatively correlated with trouble with concentrating (edge weight = −0.11). Empathic concern was negatively associated with suicidal thoughts (edge weight = −0.10) and psychomotor agitation or retardation (edge weight = −0.08). Fantasy was not connected with any symptoms of depression. Second, personal distress and empathic concern were the most positive and negative influential nodes that bridged empathy and depression (values of bridge expected influence were 0.51 and −0.19 and values of predictability were 0.24 and 0.24, respectively). The estimates of the bridge expected influence on the nodes were adequately stable (correlation stability coefficient = 0.75). Finally, no sex differences in the studied network characteristics were observed.
Conclusions: This study applied network analysis to reveal potential pathways between different dimensions of empathy and individual symptoms of depression. The findings supported the existing theoretical system and contribute to the theoretical mechanism. We have also made efforts to suggest interventions and preventions based on personal distress and empathic concern, the two most important dimensions of empathy for depressive symptoms. These efforts may help Chinese university students to adopt better practical methods to overcome symptoms of depression during the COVID-19 pandemic.
1. Introduction
The emergence of the COVID-19 pandemic and related restriction measures have created a social alienation lifestyle and multiple psychological stressors. These transitions have exacerbated mental health concerns among the general population, particularly symptoms of depression. Symptoms of depression are characterized by anhedonia, sad mood, trouble with sleeping, fatigue, eating problems, guilt, trouble with concentrating, psychomotor agitation or retardation, and suicidal thoughts (1). These symptoms have been shown to affect long-term cognitive performance and quality of life (2). Therefore, exploring possible risks, protective factors, and ways to alleviate symptoms of depression is a public health priority (3).
Empathy, the ability of one to share and understand the internal states of others (4), has been identified as a key factor in understanding depression (5, 6). Theoretically, Zahnwaxler and colleagues proposed that empathy may exaggerate a sense of responsibility for what happens to others (e.g., parents' suffering). It then produces an uncomfortable pervasive sense of guilt, exacerbating symptoms of depression (7, 8). Further, Tone and Tully (9) argued that empathic neurobiological processes would form personal distress and guilt, after being moderated by intraindividual and interindividual factors. It could then lead toward internalizing problems in the form of anxiety and depression. However, empirical studies found inconsistent results regarding the influence of empathy on depression, before and during the pandemic. On one hand, the overly active empathetic reaction may increase the likelihood of symptoms of depression due to unrealistic guilt from failure of alleviating suffering of others (10, 11). On the other hand, empathy can help people to understand their problems from various perspectives, hence cope better during the pandemic (12). For example, empathy can better buffer adverse outcomes of pressure and promote the demonstration impact of hope (12).
The internal heterogeneity of empathy could partially explain the inconsistency of the reported associations between empathy and depression. Empathy is a multi-faceted construct that includes four dimensions. These dimensions are: perspective taking (an inclination to adopt other people's psychological opinions spontaneously), empathic concern (a feeling of concerned and compassion for the unfortunate of others), fantasy (the tendency to realistically imagine the emotions and behaviors of characters in literary or media works), and personal distress (the uneasiness and anxiety in the face of tense interpersonal settings) (13). Different dimensions of empathy are related differently to depression (14). For example, perspective-taking reflecting the cognitive component of empathy has been shown to predict depression negatively, while personal distress reflecting the affective aspect of empathy has been shown to predict depression positively (15).
Depression heterogeneity is another potential reason for the inconsistency. Depression is a heterogeneous syndrome characterized by various cognitive, affective, and somatic symptoms (16). Emerging studies have demonstrated that predisposing variables are related differently to co-occurring symptoms (17–20). Thus, it is plausible to assume that different dimensions of empathy may have unique symptom pathways to co-occurring symptoms of depression. For example, perspective-taking involves the frontal brain areas, which might explain the trouble with concentrating symptom of depression (21). However, personal distress may be particularly relevant to the guilt symptom (9). Research examining the relationship between empathy and depression has focused almost exclusively on the construct level (using sum scores) despite empirical evidence suggesting that it is critical to analyze specific symptoms of depression. Focusing on a construct level may conceal important or unique symptom pathways linking empathy and depression. Therefore, moving from sum-scores analysis to dimension and symptom-level analysis may facilitate a more valuable understanding of the relationship between the two.
Network analysis is a statistical method especially suitable for dimension and symptom-level analysis. In the network approach, neuropsychological conditions result from direct interactions between symptoms rather than being underpinned by latent variables (22). Under external factors (e.g., psychological and societal mechanisms), some psychopathological constructs may be activated and transmit the effect to other constructs to generate a feedback loop (22). Recently, more studies have shown that network analysis is a valuable tool to reveal complex associations, such as the underlying mechanisms between symptoms of a given neuropsychological condition and its risk factors (11, 23–26). Utilizing partial correlations in network models allows researchers to explore unique pairwise interactions between variables and adjust for multicollinearity and generate hypotheses on potential causal effects (27, 28).
There are two key advantages of using network analysis in the present study. The first is contributing to a related theoretical framework. Network analysis represents a significant innovation to explore and visualize the interplay between different constructs in a bottom-up data-driven manner and contributes to the generation of hypotheses (27). Thus, network analysis may help expand the current theoretical research on depression and empathy from a latent variable level (total score) to a symptom/dimension level. The second is providing the potential targets for related interventions and preventions. Network analysis offers the bridge expected influence index (e.g., the sum value of all connecting edges in the other communities for a specific node) to identify influential nodes that bridge predisposing variables and symptoms of psychopathology. Nodes with high bridge expected influence may more likely confer risks to other communities (29). Therefore, predisposing variables with high bridge centrality indexes may be potential targets for related preventions and interventions.
During the pandemic, symptoms of depression among university students have increased significantly (3), and empathy is regarded as critical for healthy self-regulation (30, 31). Further, although studies have found that females may be more susceptible to empathy and depression (32, 33), whether sex differences play a role between the two constructs remains unclear (21). For instance, personal distress could explain why females are more prone to depression but the relationship between empathic concern and depression was not different between sexes (21, 34, 35). Additionally, no sex differences were observed in cognitive components of empathy, such as perspective-taking (21, 35–37). To shed light on these gaps, we sought to construct an integrated network combining dimensions of empathy and symptoms of depression. There were three aims in the current research: (1) to investigate the relationship between different dimensions of empathy and symptoms of depression; (2) to identify positive and negative influential nodes that bridge empathy and depression by using bridge centrality indexes; and (3) to explore whether there are sex differences in the above network characteristics.
2. Methods
2.1. Ethics statement
The data collection procedure followed the Declaration of Helsinki and was approved by the Ethics Committee of the First Affiliated Hospital of the Fourth Military Medical University (Project No. KY20202063-F-2).
2.2. Participants
We recruited 1,204 Chinese students using a convenience sampling method between 12 March and 20 September 2021. By intentionally constraining the sampling frame, this sampling method is considered generalized to Chinese university students as subpopulations. To avoid the generalizability of the sample being too narrow and external validity being low, we selected universities of different levels and college students of different majors in Shaanxi and Jilin Province of China. All participants provided their consent before completing a questionnaire through a Chinese online platform (Wenjuanxing). We included two honesty check questions (please select “not at all” for this question and please select “describes me very well” for this question) to detect low-quality data. An incorrect response in at least one of the two questions was considered invalid data (n = 27). Each item in the questionnaire required a response, hence, there were no missing items in any participants. The final valid data was 1,177 (505 males, 672 females; mean age = 20.38 years; SD = 2.09). Upon completing the questionnaire, participants received one to five Chinese Yuan, values drawn at random.
2.3. Measures
2.3.1. Dimensions of empathy
The Interpersonal Reactivity Index (IRI) measures empathy and is composed of four dimensions: perspective-taking, empathic concern, fantasy, and personal distress (13). Our study used the Chinese version of the IRI. The scale is comprised of 22 items and scored from 0, does not describe me very well, to 4, describes me very well (38). The current sample's Cronbach 's alpha values were as follows: full scale (0.76), perspective-taking subscale (0.76), empathic concern subscale (0.64), fantasy subscale (0.63) and personal distress subscale (0.78).
2.3.2. Symptoms of depression
The Patient Health Questionnaire-9 (PHQ-9) is a brief, valid, self-administered screening tool widely used to measure depression (39). The Chinese version of the PHQ-9 was used in the current study (40). It has nine items providing continuous scores of symptoms of depression. Participants reported to what degree each item applies to them on a 4-point Likert scale ranging from 0, not at all, to 3, nearly every day. The Cronbach 's alpha of PHQ-9 was 0.87 in this study.
2.4. Statistical analysis
Statistical analyzes were conducted through R-statistical software. We modeled the empathy-depression network via Gaussian graphical models [GGMs; (41)]. All dimensions of empathy and symptoms of depression were depicted as nodes. An edge between any two nodes demonstrated a partial correlation of the two variables after conditioning on all other network variables. This study used Spearman correlations as input when estimating the network structure, which is recommended for non-normally distributed data (28, 42). Due to the high sample size (n = 1,177) and the expectation that there may be many weak bridging edges between dimensions of empathy and symptoms of depression, this study used the unregularized model selection approach (42, 43). Following recent recommendations (42), the network estimation in this study was based on the ggmModSelect technique in the R-package qgraph (44). The Fruchterman-Reingold algorithm was used for visualizing the layout (45). The network was visualized via the R-package qgraph (44).
We calculated the bridge expected influence (the sum of edge weights from a given node in one community to another) to identify influential nodes that bridge dimensions of empathy and symptoms of depression (29). Higher bridge expected influence values implied increased cross-cluster connectivity (29). Two communities were pre-defined before analysis: the dimensions of empathy community (four dimensions from the IRI scale) and the symptoms of depression community (nine items from the PHQ-9 scale). The bridge expected influence was calculated through the R-package networktools (29). Further, we calculated the mean node predictability to quantify the mean explained variance of the estimated network via R-package mgm (46).
The accuracy of edge weights was assessed by plotting the 95% confidence interval (with 2,000 bootstrap samples) for each edge within the presented networks and calculating bootstrapped difference tests for each edge weight. The stability of bridge expected influence was evaluated by computing the correlation stability (CS)-coefficient via a case-dropping bootstrap approach (with 2,000 bootstrap samples) and computing bootstrapped difference tests for bridge expected influence. According to a previous recommendation (47), the ideal CS-coefficient is above 0.5 and should be at least 0.25. The procedures mentioned above were conducted via the R-package bootnet (47).
Finally, we used the R-package NetworkComparisonTest to compare whether sex differences existed in the empathy-depression network (permutations = 1,000; 48). Specifically, we compared global strength (summed edge weights of the networks), between-community edge weight and bridge expected influence of the empathy-depression network in male and female participants. Since we had no prior hypotheses about differences in edges, corrections for multiple comparisons were not used when testing them in the current exploratory setting (48).
3. Results
3.1. Sample characteristic and descriptive statistics of variables
Table 1 displayed the sample characteristics. Table 2 showed the mean scores and standard deviations for each variable selected in the present network.
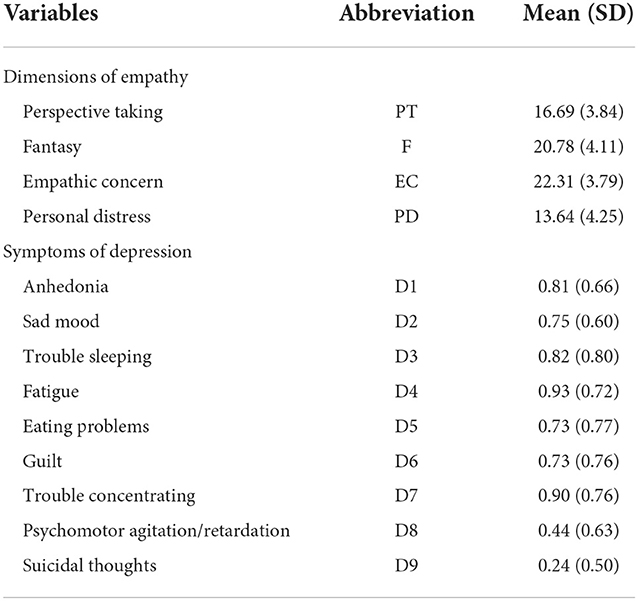
Table 2. Abbreviation, mean scores and standard deviations for each variable selected in the present empathy-depression network.
3.2. Relations between dimensions of empathy and symptoms of depression
Figure 1A presented the empathy-depression network. There were 7 of 36 (19.4%) possible between-community edges (four positive edges and three negative edges; weight range from −0.11 to 0.18) within the network. The positive between-community edges were PD (personal distress)—D8 (psychomotor agitation or retardation; edge weight = 0.18), PD—D2 (sad mood; weight = 0.12), PD—D7 (trouble with concentrating; weight = 0.11), PD—D6 (guilt; weight = 0.10). The negative between-community edges were PT (perspective-taking)—D7 (trouble with concentrating; weight = −0.11), EC (empathic concern)—D9 (suicidal thoughts; weight = −0.10), EC—D8 (psychomotor agitation or retardation, weight = −0.08). There was no edge between F (fantasy) and symptoms of depression. Supplementary Figure 1 showed the bootstrapped 95% confidence interval of edge weights within the empathy-depression network. Supplementary Figure 2 showed the bootstrapped difference test for edge weights.
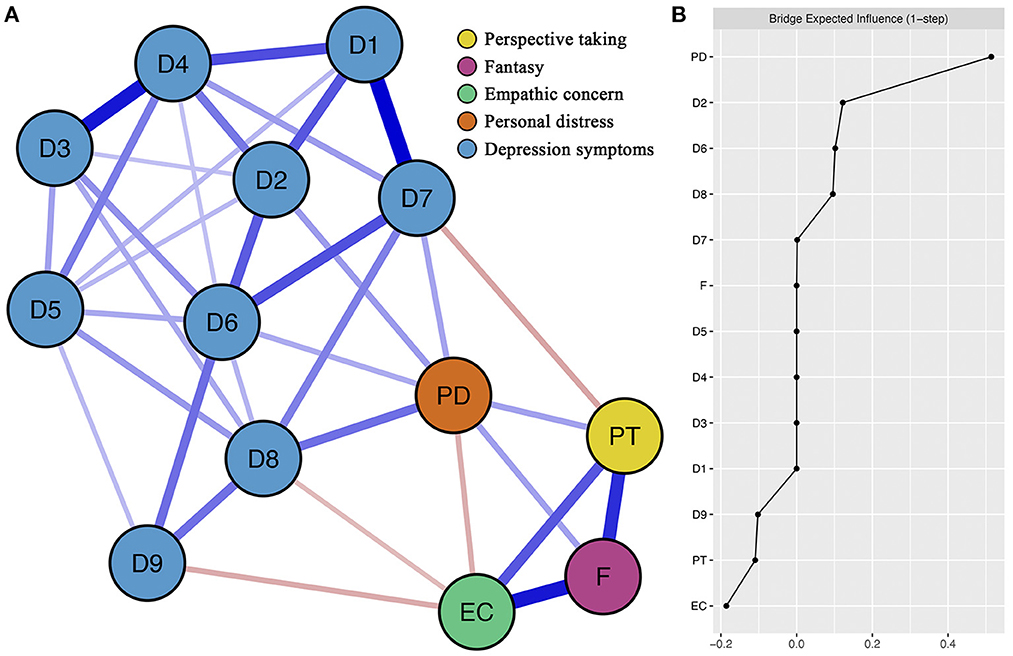
Figure 1. Network structure of different dimensions of empathy and different symptoms of depression. (A) Blue edges represent positive correlations, and red edges represent negative correlations. The thickness of the edge reflects the magnitude of the correlation. The text of variables selected in the network can be seen in Table 2. (B) Centrality plot depicting the bridge expected influence of each variable in the network.
3.3. Bridge expected influence
Figure 1B showed the bridge expected influence values of each node. PD had the highest positive bridge expected influence (value = 0.51). EC and PT had the highest negative bridge expected influences (value = −0.19 and −0.11, respectively). The CS-coefficient of bridge expected influence (value = 0.75) was larger than 0.5, indicating these centrality indexes were adequately stable (Supplementary Figure 3). Supplementary Figure 4 showed the bootstrapped difference tests for node bridge expected influence. Moreover, the results of other centrality indexes (i.e., expected influence, strength, closeness and betweenness) within empathy-depression network can be found in Supplementary Figures 5–7. Mean node predictability of the final empathy-depression network was 0.35. This indicated that on average, 35% of the variance of nodes in the present network can be explained by their neighboring nodes. More details about node predictability can be found in Supplementary Table 1.
3.4. Network comparison between genders
We did not find significant sex differences in the network global strength (Global strength [S] = 0.27, male = 5.65, female = 5.38, p = 0.31) between-community edges and node bridge expected influence.
4. Discussion
There is a lack of understanding of how each dimension of empathy may relate to individual symptoms of depression, especially during the COVID-19 pandemic. In the present study, we addressed this gap by showing specific associations between dimensions of empathy and symptoms of depression via network analysis. The findings add to the literature by showing that the related protective and risk factors differed considerably for symptoms of depression (49–55). Further, we calculated the bridge expected influence to detect influential nodes connecting dimensions of empathy and symptoms of depression. Our results indicate that personal distress has the highest positive bridge expected influence, while empathic concern has the highest negative bridge expected influence. Lastly, we found no sex differences in the structure of the empathy-depression network. These results contribute to the clarity of the empathy-depression theoretical framework and provide potential targets for related preventions and interventions.
4.1. Relations between dimensions of empathy and symptoms of depression
In concert with theoretical assumptions by Zahnwaxler et al. (7, 8), this study found that personal distress was positively associated with guilt. Individuals characterized by personal distress may experience excessive self-criticism when viewing aversive events (56), increasing the likelihood of feeling guilt during such events (57). The ongoing pandemic has heightened people's psychological distress while also causing them to suffer from experiences of guilt. For example, social workers might experience guilt and anxiety when they face people who died from the epidemic (58). Similarly, patients with COVID-19 may feel guilty about being around others and have negative emotional distress due to the stigmatization of the disease (59). Their families and medical staff could also feel guilty for not sharing their pain (60).
Echoing the theoretical model of Tone and Tully (9), our study showed positive relationships between personal distress and symptoms of depression, such as psychomotor agitation or retardation, trouble with concentrating, and sad mood. Individuals prone to personal distress and empathic reactions show an inclination to experience physiological hyperarousal and focus their cognitive resources on self-soothing (61). Nevertheless, these individuals are more sensitive to negative emotions in challenging situations (61). As a result, these individuals are more predisposed to the symptoms of depression above. In the context of the pandemic, people with a high level of personal distress may pay too much attention to their distress and get caught in negative moods (62).
Compared to personal distress, perspective-taking was negatively correlated to trouble with concentrating. These findings were consistent with a previous study (63). For instance, attentional shifts that correspond to depression and perspective-taking require switching between externally and internally focused viewpoints (63, 64). As such, individuals who are good at perspective-taking can switch back to ongoing things that require attention easily during situations that may cause trouble with concentrating. Hence, they experience less trouble with concentrating (65).
Empathic concern was negatively associated with psychomotor agitation or retardation and suicidal thoughts. According to the emotion-specificity hypothesis, psychomotor agitation or retardation is associated with deficits in emotion recognition (66), which may be due to the low ability of empathic concern (67). Meanwhile, the impairment in empathic concern reflecting negative ruminations could make people more likely to have thoughts of death (68). As the pandemic has brought an increase in suicides and a decrease in empathy (69), strategies to show more empathic concern for others might reduce depression, particularly suicidal thoughts, during the continuity of the pandemic (70).
Fantasy was not connected with any symptoms of depression. Our result was in line with previous studies, which found no significant association between fantasy and depression (71, 72). Indeed, in contrast to the other three dimensions directly related to empathy, fantasy does not directly represent empathy (32). Although IRI is considered the most appropriate tool to measure empathy to date, Baron-Cohen and Wheelwright (32) argued that the content measured by the fantasy subscale is broader than empathy itself. This discrepancy should be explicitly examined in future research.
4.2. Bridge expected influence
In the present network, node bridge expected influence might cast light on the particular role played by different dimensions of empathy in the context of depression. Personal distress has the highest positive bridge expected influence suggesting that it is the most positively influential node that bridges empathy and depression. Thus, from a network perspective, personal distress is the most critical dimension of empathy in activating risk for symptoms of depression and targeting personal distress may be more effective at reducing symptoms of depression than targeting other dimensions of empathy. Mindfulness-based stress reduction (MBSR) is an 8–10 weeks group intervention to develop mindfulness through intensive practice (73). Previous studies have shown that MBSR effectively reduces personal distress (74). The significant effects of MBSR on symptoms of depression have been demonstrated in a range of clinical and non-clinical populations (74). Particularly during the epidemic, MBSR has shown promising results for medical staff (75), isolated patients (76), unemployed people (77), and university students (78) to reduce distress and depression. This study supports the scientific application of MBSR and recommends it as an intervention for depression treatment of young adults deeply affected by personal distress.
Empathic concern has the strongest negative bridge expected influence, indicating that it is the most negatively influential node that bridges empathy and depression. Thus, empathetic concern may be the most critical dimension of empathy in activating protection for symptoms of depression. Compassion Cultivation Training (CCT) is an 8-week intervention to enhance compassion and empathy (79). Compared to MBSR, which takes an implicit approach to teaching empathy as a mindfulness practice attitude, CCT takes a more straightforward approach to training empathy. Although both CCT and MBSR can improve the ability of empathy and reduce depression, CCT is especially effective in promoting empathic concern and identification with humanity (79). Our results recommend CCT as an effective program to improve empathic concern and prevent depression. Incorporating CCT into the psychoeducation program may have a protective effect on the mental health of young adults facing the risk of depression.
4.3. Network comparison between genders
Although previous studies have shown that empathy and depression may be affected by sex, we found that sex had no significant influence on the relationship, nor bridge centrality. These results were in concert with previous work (21, 80), which means there is insufficient research to conclude sex differences in the relationship (21). Despite sex having been found to influence empathy and depression, this effect might not extend to the link between the two. Overall, the network structure results' theoretical contribution and the bridge influence's expected clinical implications in this study are suitable for both females and males, and further evidence is needed to draw more convincing conclusions in the future.
4.4. Limitations
One key limitation of the current study is the usage of cross-sectional data. We can only estimate undirected networks and cannot determine the direction of edges. Future work could use longitudinal data to ascertain the causality dynamically. Second, all study variables were measured at a group level. Thus, the research conclusions could not be applied to a single individual. Third, most of the participants in this study were graduating university students. Therefore, the conclusions of this study are more applicable to the young adult group facing the pressure of further education and employment under the pandemic, and the generalizability to populations in other age groups or geographical locations should be further verified. Fourth, non-probability sampling strategies were used in this study; probability sampling strategies are recommended in the future to yield an unbiased sample representative of the target population. Fifth, like other existing studies that examine how external field factors may act directly upon symptom networks (e.g., emotion regulation and intolerance of uncertainty) (17, 23, 26, 52, 53), we focused on one particular construct of the external field (i.e., empathy). Future research could add potential covariates (e.g., age, exercise, smoking, and chronic diseases) (50) into the model to further improve its validity. Finally, since there was no pre-pandemic data, the specific impact of the pandemic on the relationship between empathy and depression cannot be fully determined.
5. Conclusion
The present study advances the understanding of the relationship between empathy and depression through the network approach. Our results showed that different dimensions of empathy have specific relations with different symptoms of depression. From the network perspective, this study supports previous theoretical models of the relationship between empathy and depression while also discovers more diverse pathways, such as psychomotor agitation or retardation, trouble with concentrating, and sad mood. These explored pathways provide a new insight for the improvement of theoretical mechanisms related to empathy and depression. Moreover, our findings suggest that preventions and interventions that target personal distress and empathic concern of empathy may be promising in reducing depression from the context of empathy during the COVID-19 pandemic.
Data availability statement
The raw data supporting the conclusions of this article will be made available by the authors, without undue reservation.
Ethics statement
The studies involving human participants were reviewed and approved by Ethics Committee of the First Affiliated Hospital of the Fourth Military Medical University. The questionnaire was completed online through a Chinese platform (Wenjuanxing) after participants provided written informed consent.
Author contributions
JL and LR developed study idea and design. JL and PW collected data. JL, CL, LR, and XL wrote the original draft of this manuscript. JL, CL, LR, TW, and KL revised the manuscript. XL, CL, and LR give guidance to the manuscript. All authors contributed to revising subsequent versions of the paper.
Funding
LR's involvement in this research was funded by the Fourth Military Medical University (2021JSTS30). XL's involvement in this research was funded by the Fourth Military Medical University (BWS16J012).
Acknowledgments
We would like to thank all the individuals who participated in the study.
Conflict of interest
The authors declare that the research was conducted in the absence of any commercial or financial relationships that could be construed as a potential conflict of interest.
Publisher's note
All claims expressed in this article are solely those of the authors and do not necessarily represent those of their affiliated organizations, or those of the publisher, the editors and the reviewers. Any product that may be evaluated in this article, or claim that may be made by its manufacturer, is not guaranteed or endorsed by the publisher.
Supplementary material
The Supplementary Material for this article can be found online at: https://www.frontiersin.org/articles/10.3389/fpubh.2022.1034119/full#supplementary-material
References
1. Marguilho M, Nobre A. Effects of the Covid-19 pandemic on mental health what do we know so far? Eur Psychiatry. (2021) 64:S312. doi: 10.1192/j.eurpsy.2021.839
2. Poletti S, Palladini M, Mazza MG, De Lorenzo R, Furlan R, Ciceri F, et al. Long-term consequences of Covid-19 on cognitive functioning up to 6 months after discharge: role of depression and impact on quality of life. Eur Arch Psychiatry Clin Neurosci. (2021) 272:773–82. doi: 10.1007/s00406-021-01346-9
3. Koelen JA, Mansueto AC, Finnemann A, de Koning L, van der Heijde CM, Vonk P, et al. Covid-19 and mental health among at-risk university students: a prospective study into risk and protective factors. Int J Methods Psychiatric Res. (2022) 31:e1901. doi: 10.1002/mpr.1901
4. Weisz E, Cikara M. Strategic regulation of empathy. Trends Cogn Sci. (2021) 25:213–27. doi: 10.1016/j.tics.2020.12.002
5. Guadagni V, Umilta A, Iaria G. Sleep quality, empathy, and mood during the isolation period of the Covid-19 pandemic in the canadian population: females and women suffered the most. Front Global Women's Health. (2020) 1:585938. doi: 10.3389/fgwh.2020.585938
6. Han J, Choi J-H, Lee S-O, Kim Y-R, Soo KS. The effect of empathy on anxiety and depression in Covid-19 disaster: through risk perception and indirect trauma. J Korea Contents Associat. (2021) 21:609–25. doi: 10.5392/JKCA.2021.21.11.609
7. Zahnwaxler C, Radkeyarrow M. The origins of empathic concern. Motiv Emot. (1990) 14:107–30. doi: 10.1007/BF00991639
8. Zahnwaxler C, Hulle CV. Empathy, guilt, and depression: when caring for others becomes costly to children. Pathol Altruism. (2012) 321–44. doi: 10.1093/acprof:oso/9780199738571.003.0224
9. Tone EB, Tully EC. Empathy as a “risky strength”: a multilevel examination of empathy and risk for internalizing disorders. Dev Psychopathol. (2014) 26:1547–65. doi: 10.1017/S0954579414001199
10. O'Connor LE, Berry JW, Lewis T, Mulherin K, Crisostomo PS. Empathy and depression: the moral system on overdrive. Empathy Mental Illness. (2007) 49:75. doi: 10.1017/CBO9780511543753.005
11. Hughes EK, Gullone E, Watson SD. Emotional functioning in children and adolescents with elevated depressive symptoms. J Psychopathol Behav Assess. (2011) 33:335–45. doi: 10.1007/s10862-011-9220-2
12. Hu YX, Ye BJ, Im H. Hope and post-stress growth during Covid-19 pandemic: the mediating role of perceived stress and the moderating role of empathy. Personal Individual Differ. (2021) 178:110831. doi: 10.1016/j.paid.2021.110831
13. Davis MH. Measuring individual-differences in empathy—evidence for a multidimensional approach. J Pers Soc Psychol. (1983) 44:113–26. doi: 10.1037/0022-3514.44.1.113
14. Yan Z, Zeng X, Su J, Zhang X. The dark side of empathy: meta-analysis evidence of the relationship between empathy and depression. Psych J. (2021) 10:794–804. doi: 10.1002/pchj.482
15. Neumann DL, Chan RCK, Wang Y, Boyle GJ. Cognitive and affective components of empathy and their relationship with personality dimensions in a Chinese sample. Asian J Social Psychol. (2016) 19:244–53. doi: 10.1111/ajsp.12138
16. Fried EI, Nesse RM. Depression sum-scores don't add up: why analyzing specific depression symptoms is essential. BMC Med. (2015) 13:1–11. doi: 10.1186/s12916-015-0325-4
17. Liu C, Ren L, Li K, Yang W, Li Y, Rotaru K, et al. Understanding the association between intolerance of uncertainty and problematic smartphone use a network analysis. Front Psychiatry. (2022) 13:917833. doi: 10.3389/fpsyt.2022.917833
18. Li K, Zhan X, Ren L, Liu N, Zhang L, Li L, et al. The association of abuse and depression with suicidal ideation in Chinese adolescents: a network analysis. Front Psychiatry. (2022) 13:853951. doi: 10.3389/fpsyt.2022.853951
19. Ren L, Wang Y, Wu L, Wei Z, Cui L-B, Wei X, et al. Network structure of depression and anxiety symptoms in Chinese female nursing students. BMC Psychiatry. (2021) 21:1–12. doi: 10.1186/s12888-021-03276-1
20. Wei Z, Ren L, Wang X, Liu C, Cao M, Hu M, et al. Network of depression and anxiety symptoms in patients with epilepsy. Epilepsy Res. (2021) 175:106696. doi: 10.1016/j.eplepsyres.2021.106696
21. Schreiter S, Pijnenborg GH, Aan Het Rot M. Empathy in adults with clinical or subclinical depressive symptoms. J Affect Disord. (2013) 150:1–16. doi: 10.1016/j.jad.2013.03.009
22. Borsboom D. A network theory of mental disorders. World Psychiatry. (2017) 16:5–13. doi: 10.1002/wps.20375
23. Ren L, Wei ZH, Li Y, Cui LB, Wang YF, Wu L, et al. The relations between different components of intolerance of uncertainty and symptoms of generalized anxiety disorder: a network analysis. BMC Psychiatry. (2021) 21:1–11. doi: 10.1186/s12888-021-03455-0
24. Wei X, Jiang H, Wang H, Geng J, Gao T, Lei L, et al. The relationship between components of neuroticism and problematic smartphone use in adolescents: a network analysis. Personal Individual Differ. (2022) 186:111325. doi: 10.1016/j.paid.2021.111325
25. Ren L, Yang Z, Wang YD, Cui LB, Jin YC, Ma ZJ, et al. The relations among worry, meta-worry, intolerance of uncertainty and attentional bias for threat in men at high risk for generalized anxiety disorder: a network analysis. BMC Psychiatry. (2020) 20:1–11. doi: 10.1186/s12888-020-02849-w
26. Feng TW, Ren L, Liu C, li KL, Wu L, Wei XY, et al. The relations between different components of intolerance of uncertainty and symptoms of depression during the COVID-19 pandemic: a network analysis. Front Psychiatry. (2022) 13. doi: 10.3389/fpsyt.2022.993814
27. Borsboom D, Cramer AO. Network analysis: an integrative approach to the structure of psychopathology. Annu Rev Clin Psychol. (2013) 9:91–121. doi: 10.1146/annurev-clinpsy-050212-185608
28. Epskamp S, Fried EI. A tutorial on regularized partial correlation networks. Psychol Methods. (2018) 23:617–34. doi: 10.1037/met0000167
29. Jones PJ, Ma R, McNally RJ. Bridge centrality: a network approach to understanding comorbidity. Multivariate Behav Res. (2021) 56:353–67. doi: 10.1080/00273171.2019.1614898
30. Zhang H, Yang Q, Bei Y, Destech Publicat Inc. The relationship between university students' empathy ability and their abiding by social morality, joint conference of international conference on social science and contemporary humanity development (SSCHD). In: International Conference on Management, Economics and Social Development (ICMESD). Wuhan: China (2015). p. 69–73.
31. Euna L. A study on empathy education for the improvement of university students' empathy ability: with the focus on the ewha womans university course 'homo empathicus: communication, empathy, trust'. J Edu Culture. (2017) 23:149–68. doi: 10.24159/joec.2017.23.5.149
32. Baron-Cohen S, Wheelwright S. The empathy quotient: an investigation of adults with asperger syndrome or high functioning autism, and normal sex differences. J Autism Dev Disord. (2004) 34:163–75. doi: 10.1023/B:JADD.0000022607.19833.00
33. Nolen-Hoeksema S. Gender differences in depression. Curr Dir Psychol Sci. (2001) 10:173–6. doi: 10.1111/1467-8721.00142
34. Grynberg D, Luminet O, Corneille O, Grezes J. Berthoz S. Alexithymia in the interpersonal domain: a general deficit of empathy?. Personal Individual Differ. (2010) 49:845–50. doi: 10.1016/j.paid.2010.07.013
35. Laurent SM, Hodges SD. Gender roles and empathic accuracy: the role of communion in reading minds. Sex Roles. (2009) 60:387–98. doi: 10.1007/s11199-008-9544-x
36. Gadassi R, Mor N, Rafaeli E. Depression and empathic accuracy in couples: an interpersonal model of gender differences in depression. Psychol Sci. (2011) 22:1033–41. doi: 10.1177/0956797611414728
37. Zaki J, Bolger N, Ochsner K. It takes two—the interpersonal nature of empathic accuracy. Psychol Sci. (2008) 19:399–404. doi: 10.1111/j.1467-9280.2008.02099.x
38. Zhang F, Dong Y, Wang K. Reliability and validity of the chinese version of the interpersonal reactivity index-C. Chin J Clin Psychol. (2010) 18:155–7.
39. Kroenke K, Spitzer RL, Williams JBW. The Phq-9—validity of a brief depression severity measure. J Gen Intern Med. (2001) 16:606–13. doi: 10.1046/j.1525-1497.2001.016009606.x
40. Sun XY, Li YX, Yu CQ, Li LM. Reliability and validity of depression scales of chinese version: a systematic review. Zhonghua liu xing bing xue za zhi. (2017) 38:110–6.
41. Epskamp S, Waldorp LJ, Mottus R, Borsboom D. The Gaussian graphical model in cross-sectional and time-series data. Multivariate Behav Res. (2018) 53:453–80. doi: 10.1080/00273171.2018.1454823
42. Isvoranu AM, Epskamp S. Which estimation method to choose in network psychometrics? Deriving guidelines for applied researchers. Psychol Methods. (2021). doi: 10.1037/met0000439
43. Williams DR, Rast P. Back to the basics: rethinking partial correlation network methodology. Br J Math Stat Psychol. (2020) 73:187–212. doi: 10.1111/bmsp.12173
44. Epskamp S, Cramer AOJ, Waldorp LJ, Schmittmann VD, Borsboom D. Qgraph: network visualizations of relationships in psychometric data. J Stat Softw. (2012) 48:1–18. doi: 10.18637/jss.v048.i04
45. Fruchterman TMJ, Reingold EM. Graph drawing by force-directed placement. Softw Practice Exp. (1991) 21:1129–64. doi: 10.1002/spe.4380211102
46. Haslbeck JMB, Fried EI. How predictable are symptoms in psychopathological networks? A reanalysis of 18 published datasets. Psychol Med. (2017) 47:2267–76. doi: 10.1017/S0033291717001258
47. Epskamp S, Borsboom D, Fried EI. Estimating psychological networks and their accuracy: a tutorial paper. Behav Res Methods. (2018) 50:195–212. doi: 10.3758/s13428-017-0862-1
48. Van Borkulo CD, Van Bork R, Boschloo L, Kossakowski JJ, Tio P, Schoevers RA, et al. Comparing network structures on three aspects: a permutation test. Psychol Methods. (2022). doi: 10.1037/met0000476
49. Triolo F, Murri MB, Calderon-Larranaga A, Vetrano DL, Sjoberg L, Fratiglioni L, et al. Bridging late-life depression and chronic somatic diseases: a network analysis. Transl Psychiatry. (2021) 11:1–8. doi: 10.1038/s41398-021-01686-z
50. Fried EI, von Stockert S, Haslbeck JMB, Lamers F, Schoevers RA, Penninx BWJH. Using network analysis to examine links between individual depressive symptoms, inflammatory markers, and covariates. Psychol Med. (2020) 50:2682–90. doi: 10.1017/S0033291719002770
51. Skjerdingstad N, Johnson MS, Johnson SU, Hoffart A, Ebrahimi OV. Feelings of worthlessness links depressive symptoms and parental stress: a network analysis during the COVID-19 pandemic. Eur Psychiatry. (2021) 64:1. doi: 10.1192/j.eurpsy.2021.2223
52. Everaert J, Joormann J. Emotion regulation difficulties related to depression and anxiety: a network approach to model relations among symptoms, positive reappraisal, and repetitive negative thinking. Clin Psychol Sci. (2019) 7:1304–18. doi: 10.1177/2167702619859342
53. Liang S, Liu C, Rotaru K, Li K, Wei X, Yuan S, et al. The relations between emotion regulation, depression and anxiety among medical staff during the late stage of COVID-19 pandemic: a network analysis. Psychiatry Res. (2022) 317:114863. doi: 10.1016/j.psychres.2022.114863
54. Yang Y, Zhang SF, Yang BX, Li W, Sha S, Jia FJ, et al. Mapping network connectivity among symptoms of depression and pain in wuhan residents during the late-stage of the COVID-19 pandemic. Front Psychiatry. (2022) 13. doi: 10.3389/fpsyt.2022.814790
55. Fried EI, Nesse RM, Zivin K, Guille C, Sen S. Depression is more than the sum score of its parts: individual dsm symptoms have different risk factors. Psychol Med. (2014) 44:2067–76. doi: 10.1017/S0033291713002900
56. Kim H, Han S. Does personal distress enhance empathic interaction or block it? Pers Individ Dif. (2018) 124:77–83. doi: 10.1016/j.paid.2017.12.005
57. Gambin M, Sharp C. The relations between empathy, guilt, shame and depression in inpatient adolescents. J Affect Disord. (2018) 241:381–7. doi: 10.1016/j.jad.2018.08.068
58. Davies H, Cheung M. Covid-19 and first responder social workers: an unexpected mental health storm. Soc Work. (2022) 67:114–22. doi: 10.1093/sw/swac007
59. Hamama L, Levin-Dagan N. People who contracted covid-19: the mediating role of shame and guilt in the link between threatening illness perception and mental health measures. Anxiety Stress Coping. (2022) 35:72–85. doi: 10.1080/10615806.2021.1964073
60. Dhavale P, Koparkar A, Fernandes P. Palliative care interventions from a social work perspective and the challenges faced by patients and caregivers during Covid-19. Indian J Palliat Care. (2020) 26:S58–62. doi: 10.4103/IJPC.IJPC_149_20
61. Cristea IA, Legge E, Prosperi M, Guazzelli M, David D, Gentili C. Moderating effects of empathic concern and personal distress on the emotional reactions of disaster volunteers. Disasters. (2014) 38:740–52. doi: 10.1111/disa.12075
62. Ma XL, Wang XC. The role of empathy in the mechanism linking parental psychological control to emotional reactivities to Covid-19 pandemic: a pilot study among chinese emerging adults. Personal Individual Differ. (2021) 168:1103999. doi: 10.1016/j.paid.2020.110399
63. Goodhew SC, Edwards M. Attentional control both helps and harms empathy. Cognition. (2021) 206:104505. doi: 10.1016/j.cognition.2020.104505
64. Judah MR, Grant DM, Mills AC, Lechner WV. Factor structure and validation of the attentional control scale. Cogn Emot. (2014) 28:433–51. doi: 10.1080/02699931.2013.835254
65. Wilshire CE, Ward T, Clack S. Symptom descriptions in psychopathology: how well are they working for us? Clin Psychol Sci. (2021) 9:323–39. doi: 10.1177/2167702620969215
66. Rojahn J, Rabold DE. Schneider F. Emotion specificity in mental-retardation. Am J Mental Retard. (1995) 99:477–86.
67. Israelashvili J, Sauter D, Fischer A. Two facets of affective empathy: concern and distress have opposite relationships to emotion recognition. Cogn Emot. (2020) 34:1112–22. doi: 10.1080/02699931.2020.1724893
68. Ekinci O, Ekinci A. Relationship between empathic responding and its clinical characteristics in patients with major depressive disorder. Dusunen Adam J Psychiatry Neurol Sci. (2016) 29:145–54. doi: 10.5350/DAJPN2016290206
69. Spitzer M. Open schools! weighing the effects of viruses and lockdowns on children. Trends Neurosci Edu. (2021) 22:100151. doi: 10.1016/j.tine.2021.100151
70. Slavich GM, Roos LG, Zaki J. Social belonging, compassion, and kindness: key ingredients for fostering resilience, recovery, and growth from the Covid-19 pandemic. Anxiety Stress Coping. (2022) 35:1–8. doi: 10.1080/10615806.2021.1950695
71. Cusi AM, MacQueen GM, Spreng RN, McKinnon MC. Altered empathic responding in major depressive disorder: relation to symptom severity, illness burden, and psychosocial outcome. Psychiatry Res. (2011) 188:231–6. doi: 10.1016/j.psychres.2011.04.013
72. O'Connor LE, Berry JW, Weiss J, Gilbert P. Guilt, fear, submission, and empathy in depression. J Affect Disord. (2002) 71:19–27. doi: 10.1016/S0165-0327(01)00408-6
73. Song Y, Lindquist R. Effects of mindfulness-based stress reduction on depression, anxiety, stress and mindfulness in Korean nursing students. Nurse Educ Today. (2015) 35:86–90. doi: 10.1016/j.nedt.2014.06.010
74. Birnie K, Speca M, Carlson LE. Exploring self-compassion and empathy in the context of mindfulness-based stress reduction (Mbsr). Stress Health. (2010) 26:359–71. doi: 10.1002/smi.1305
75. Liu Y, Luo SX, Ye JL, Chen YZ Li JF, Li YX. The use of online MBSR audio in medical staff during the COVID-19 in China. Eur Rev Med Pharmacol Sci. (2020) 24:10874–8. doi: 10.26355/eurrev_202010_23451
76. Gu Y, Li C, Song Y, Lu G, Yan J. Effects of mindfulness-based stress reduction using Tencent Meeting on anxiety and depression of isolated patients with COVID-19. Chin Nurs Manage. (2021) 21:707–12.
77. Wasson RS, Luberto CM, Murthi M, McDonald SB, Pallerla H, Novak BK, et al. Feasibility and acceptability of a community-based modified mindfulness-based stress reduction program for the under- and unemployed. Global Adv Health Med. (2020) 9:2164956120973636. doi: 10.1177/2164956120973636
78. MacDonald HZ, Neville T. Promoting university students' mindfulness, mental health, and self-compassion in the time of COVID-19: feasibility and efficacy of an online, interactive mindfulness-based stress reduction randomized trial. J College Student Psychotherapy. (2022) 10:1–19. doi: 10.1080/87568225.2022.2028329
79. Brito-Pons G, Campos D, Cebolla A. Implicit or explicit compassion? Effects of compassion cultivation training and comparison with mindfulness-based stress reduction. Mindfulness. (2018) 9:1494–508. doi: 10.1007/s12671-018-0898-z
Keywords: COVID-19, empathy, depression, network analysis, symptom level, bridge nodes, sex differences
Citation: Li J, Liu C, Wulandari T, Wang P, Li K, Ren L and Liu X (2022) The relationship between dimensions of empathy and symptoms of depression among university students during the COVID-19 pandemic: A network analysis. Front. Public Health 10:1034119. doi: 10.3389/fpubh.2022.1034119
Received: 01 September 2022; Accepted: 29 November 2022;
Published: 22 December 2022.
Edited by:
Zheng Zhu, Fudan University, ChinaCopyright © 2022 Li, Liu, Wulandari, Wang, Li, Ren and Liu. This is an open-access article distributed under the terms of the Creative Commons Attribution License (CC BY). The use, distribution or reproduction in other forums is permitted, provided the original author(s) and the copyright owner(s) are credited and that the original publication in this journal is cited, in accordance with accepted academic practice. No use, distribution or reproduction is permitted which does not comply with these terms.
*Correspondence: Lei Ren, rl_fmmu@163.com; Xufeng Liu, lxf_fmmu@163.com
†These authors have contributed equally to this work