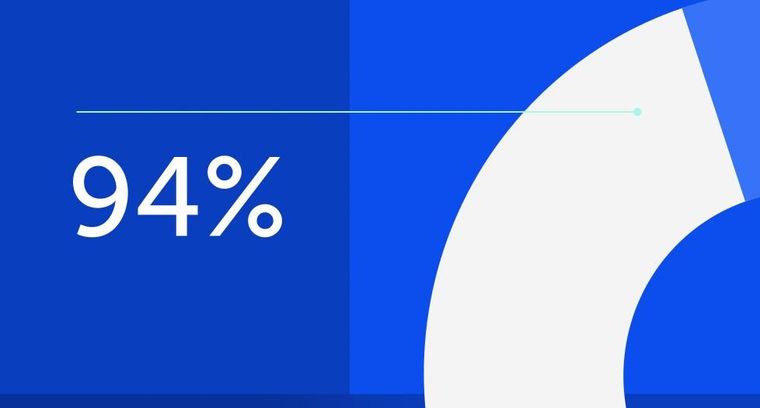
94% of researchers rate our articles as excellent or good
Learn more about the work of our research integrity team to safeguard the quality of each article we publish.
Find out more
ORIGINAL RESEARCH article
Front. Public Health, 13 January 2023
Sec. Infectious Diseases: Epidemiology and Prevention
Volume 10 - 2022 | https://doi.org/10.3389/fpubh.2022.1031372
This article is part of the Research TopicChlamydia Trachomatis Infection: Epidemiology, Prevention, Clinical, and Basic Science ResearchView all 13 articles
Background: Chlamydia trachomatis (chlamydia) is one of the most common sexually transmitted infections (STI) globally, and re-infections are common. Current Australian guidelines recommend re-testing for chlamydia 3 months after treatment to identify possible re-infection. Patient-delivered partner therapy (PDPT) has been proposed to control chlamydia re-infection among heterosexuals. We aimed to identify determinants and the prediction of chlamydia re-testing and re-infection within 1 year among heterosexuals with chlamydia to identify potential PDPT candidates.
Methods: Our baseline data included 5,806 heterosexuals with chlamydia aged ≥18 years and 2,070 re-tested for chlamydia within 1 year of their chlamydia diagnosis at the Melbourne Sexual Health Center from January 2, 2015, to May 15, 2020. We used routinely collected electronic health record (EHR) variables and machine-learning models to predict chlamydia re-testing and re-infection events. We also used logistic regression to investigate factors associated with chlamydia re-testing and re-infection.
Results: About 2,070 (36%) of 5,806 heterosexuals with chlamydia were re-tested for chlamydia within 1 year. Among those retested, 307 (15%) were re-infected. Multivariable logistic regression analysis showed that older age (≥35 years old), female, living with HIV, being a current sex worker, patient-delivered partner therapy users, and higher numbers of sex partners were associated with an increased chlamydia re-testing within 1 year. Multivariable logistic regression analysis also showed that younger age (18–24 years), male gender, and living with HIV were associated with an increased chlamydia re-infection within 1 year. The XGBoost model was the best model for predicting chlamydia re-testing and re-infection within 1 year among heterosexuals with chlamydia; however, machine learning approaches and these self-reported answers from clients did not provide a good predictive value (AUC < 60.0%).
Conclusion: The low rate of chlamydia re-testing and high rate of chlamydia re-infection among heterosexuals with chlamydia highlights the need for further interventions. Better targeting of individuals more likely to be re-infected is needed to optimize the provision of PDPT and encourage the test of re-infection at 3 months.
Chlamydia trachomatis (chlamydia) is the most common bacterial sexually transmitted infection (STI) globally (1, 2). An estimated 131 million incident cases of chlamydia infections are acquired globally among people aged 15–49 years annually (3). After treatment, chlamydia re-infections are common, occurring in about 10–20% of patients within 12 months (4–8). Identifying and treating chlamydia re-infection promptly among women is vital because re-infection is associated with a higher risk of pelvic inflammatory disease (9). A study in Australia reported that the chlamydia re-infection rate was 22.3 (95%CI: 13.2–37.6) per 100 person-years among women aged 16–25 years (8). Australian guidelines recommend chlamydia re-testing at 3 months after treatment to identify possible re-infection (10, 11). Chlamydia re-testing was relatively low (40%) within 1.5–12 months (12). Therefore, developing innovative measures that increase repeat testing for chlamydia following chlamydia infection is important.
Treatment of partners is necessary for heterosexuals with chlamydia to prevent re-infection and interrupt the chain of chlamydia transmission (13). Patient Delivery Partner Therapy (PDPT) has been proposed as a possible strategy for reducing re-infection and preventing the sequelae of STIs (14). PDPT involves providing antibiotic treatment to the sexual partners of patients diagnosed with a treatable sexually transmitted infection by giving the prescription or medication to the patient's sexual partner(s) via the diagnosed patient (15). The purpose of PDPT is to enable sexual partners who may be reluctant to attend a health provider to be treated earlier, thus reducing the risk of re-infecting the diagnosed patient. Two observational studies found that PDPT may lower women's re-infection risk (16, 17). In Australia, PDPT is permissible for heterosexual men and women diagnosed with chlamydia (18). Identifying heterosexuals with chlamydia at high risk of re-infection who could benefit from PDPT is important for implementing PDPT.
Predictive models can assist clinical decision-making (19). Developing a predictive model for predicting chlamydia re-testing and re-infection within 1 year among heterosexuals could be used to identify potential PDPT candidates and prioritize chlamydia re-infection screening and sexual health service planning. Machine learning approaches have some advantages in prediction, including not requiring statistical inferences and assumptions (20), improving accuracy by exploiting complex interactions between risk factors (21), handling a mass of predictors and combining them in a non-linear and highly interactive way (22). Despite these advantages, to our knowledge, only one study used machine learning to predict STI re-infection. Our study used machine learning algorithms to predict the risk of acquiring ≥1 or ≥2 additional STIs (combinations of gonorrhea, chlamydia, and syphilis) diagnoses within 1 or 2 years after the initial diagnosis among patients (not limited to heterosexuals) in Massachusetts, USA (23). No studies have used machine learning to predict chlamydia re-testing or re-infection among heterosexuals. Our research team has also used machine learning approaches to predict the uptake of HIV/STI testing among men who have sex with men receiving reminders for testing (24).
We aimed to predict chlamydia re-testing and re-infection within 1 year among heterosexuals with chlamydia using machine learning algorithms. We also used univariable and multivariable logistic regression to identify variables associated with chlamydia re-testing and re-infection.
Our analysis used electronic health records (EHRs) data between January 2, 2015, and May 15, 2020, at the Melbourne Sexual Health Center (MSHC). We included individuals who were (1) heterosexuals with chlamydia (including heterosexual males and females) and (2) aged 18 years and above.
Based on the literature review and expert discussion, we selected potential predictors. Potential predictors were selected from data routinely collected through a computer-assisted self-interviewing system used when patients enter the clinic (25) and from other routinely collected data items in the clinical setting. Self-reported predictors at baseline (i.e., at the time of their diagnosis of chlamydia) were included, such as demographics (age at the consultation, gender, country of birth, access to Medicare, ever sex worker, current sex worker), sexual practices (numbers of sex partners in the last 3 months, sex overseas in the last 12 months, condoms used with sex partners in the last 3 months), self-reported past STI (i.e., chlamydia, genital herpes, genital warts, gonorrhea, syphilis), HIV infection, use of PDPT (i.e., the diagnosed client receiving an extra prescription of antibiotics to give to their sexual partner), injecting drug use in the last 3 months, triage reason as asymptomatic screening, triage reason as STI symptoms, and triage reason as contact of any STI (summarized in Table 1). In the machine learning analysis, we did not include PDPT users.
Table 1. Factors associated with chlamydia re-testing within 1 year among 5,806 heterosexual individuals with chlamydia.
The primary outcome was chlamydia re-infection, defined as the first new chlamydia diagnosis using nucleic acid amplification testing from any anatomical site, including the oropharynx, urethra/urine, or anorectum, at least 30 days after and within 365 days after a positive chlamydia diagnosis. The selection of chlamydia re-infection from 30 to 365 days after a positive chlamydia diagnosis is consistent with a Chlamydia trachomatis re-infection study among female adolescents (26). In this study, chlamydia re-testing was defined as those heterosexuals who had new chlamydia diagnosis results (either positive or negative) at least 30 days after and within 365 days after a positive chlamydia diagnosis.
Statistical analysis was conducted with R 4.0.3. Descriptive statistics were used to summarize the patient characteristics. We also performed risk factor analysis using univariable and multivariable logistic regression analyses to calculate the unadjusted odds ratio (OR) and adjusted OR. Variables with p < 0.20 in the univariable logistic regression analysis were included in the multivariable logistic regression analysis to identify independent risk factors. All statistics were performed using a two-sided test, and statistical significance was considered at p < 0.05.
Machine learning algorithms were conducted with Python 3.9.7. We developed 10 commonly utilized machine learning models, including Logistic Regression (LR), K-Nearest Neighbors (KNN), Adaptive Boosting Classifier (AdaBoost), SVM with a Radial Basis Function Kernel (SVM), Gaussian Naive Bayes (GaussianNB), Gradient Boosting Machine (GBM), Light Gradient Boosting Decision Machine (LightGBM), Extreme Gradient Boosting (XGBoost), Random Forest (RF), and Multi-Layer Perceptron (MLP). LR, GBM, AdaBoost, GaussianNB, KNN, SVM, RF, and MLP was built using the scikit-learn library. LightGBM was built using the LightGBM library. XGBoost was built using the xgboost library. We performed a 3 × 10 (3 outer folds, 10 inner folds) nested cross-validation to avoid overfitting and improve generalizability (25, 27). We used the Bayesian optimisation method for tuning the hyperparameters. The performance of machine learning models was measured by the area under the receiver operating characteristics curve (AUC) and accuracy on the independent testing dataset. We also calculated the variable importance of chlamydia re-testing and re-infection within 1 year to investigate the effect of different predictors on prediction (24, 25, 28). First, we obtained the results of variable importance analysis from each fold in the outer loop of nested cross-validation. Then based on the value of AUC, we chose the one closest to the mean AUC and reported the results of this variable importance analysis.
Ethical approval was granted by the Alfred Hospital Ethics Committee, Australia (project number: 277/20).
Our study data included 5,806 heterosexual patients diagnosed with chlamydia. Among 5,806 patients, 35.7% (2,070/5,806) were re-tested for chlamydia within 1 year. A total of 14.8% (307/2,070) heterosexuals with chlamydia were re-infected with chlamydia within 1 year. Further details of the sociodemographic characteristics and sexual practices are provided in Tables 1, 2.
Table 2. Factors associated with chlamydia re-infection within 1 year among 2,070 re-tested heterosexual individuals.
The potential factors that were included in the multivariable logistic regression analysis of chlamydia re-testing within 1 year among heterosexuals with chlamydia were age, gender, HIV infection, triage reason as asymptomatic screening, triage reason as contact of STI infection, current sex work, use of PDPT, country of birth, access to Medicare, injecting drug use in the past 3 months, had sex with a partner in the past 3 months, numbers of sex partners in the past 3 months, condoms used with sex partners in the last 3 months. Chlamydia re-testing within 1 year among heterosexuals was associated with male gender [adjusted odds ratios (aOR) = 0.74, 95%CI 0.66, 0.84], living with HIV (aOR = 2.27, 95%CI 1.18, 4.50), presentation as contact of STI infection (aOR = 0.79, 95%CI 0.69, 0.91), being a current sex worker (aOR = 2.50, 95%CI 1.88, 3.33), users of patient delivered partner therapy (aOR = 1.32, 95%CI 1.02, 1.70), older age (≥35 years old vs. 18–24 years, aOR = 1.27, 95%CI 1.04, 1.55), unknown/missing on accessing to Medicare (aOR = 0.41, 95%CI 0.34, 0.50), and higher numbers of partners in the last 3 months (>10 vs.1, aOR = 1.46, 95%CI 1.06, 2.01) (Table 1).
The potential factors that were included in the multivariable logistic regression analysis of chlamydia re-infection within 1 year among heterosexuals with chlamydia were age, gender, HIV infection, past genital herpes, past gonorrhea, current sex worker, sex overseas in the past 12 months, country of birth, access to Medicare, injecting drug use in the past 3 months, had sex with a partner in the past 3 months, numbers of sex partners in the past 3 months, and condoms used with sex partners in the past 3 months. Chlamydia re-infection within 1 year among heterosexuals was associated with male gender (aOR = 1.55, 95%CI 1.16, 2.08), living with HIV (aOR = 4.02, 95%CI 1.64, 9.87), and older age (≥35 years old vs. 18–24 years, aOR = 0.58, 95%CI 0.37, 0.89) (Table 2).
The best model for predicting chlamydia re-testing within 1 year among heterosexuals with chlamydia was the XGBoost [mean 58.5% (SD 0.1%)]. Adaboost, SVM, LightGBM, and XGBoost performed better than LR in predicting chlamydia re-testing within 1 year among heterosexuals with chlamydia. However, machine learning approaches did not provide a good predictive value for chlamydia re-testing on the testing data (AUC < 60.0%). Further details of model performance are provided in Table 3 and Supplementary Table 1. Variable importance analysis using XGBoost showed that the top 10 identified predictors for chlamydia re-testing within 1 year among heterosexuals with chlamydia included current sex worker, access to Medicare, condoms used with sex partners, age, gender, triage reason as contact of STI infection, injecting drug use, sex overseas, numbers of sex partners, and triage reason as STI symptoms (Figure 1).
Table 3. Machine learning model evaluation of chlamydia re-testing and re-infection within1 year among heterosexual individuals with chlamydia on the testing data set (mean/SD).
Figure 1. Variable importance in the prediction of chlamydia re-testing within 1 year among heterosexuals by XGBoost.
The best model for predicting chlamydia re-infection within 1 year among heterosexuals with chlamydia was the XGBoost [mean 58.5% (SD 1.1%)]. Adaboost and XGBoost performed better than LR in predicting chlamydia re-infection within 1 year among heterosexuals with chlamydia. However, machine learning approaches did not provide a good predictive value for chlamydia re-infection on the testing data (all AUC < 60.0%). Further details of model performance are provided in Table 3 and Supplementary Table 1. Variable importance analysis using XGBoost showed that the top 10 identified predictors for chlamydia re-infection within 1 year among heterosexuals with chlamydia included gender, number of sex partners, age, past gonorrhea, past chlamydia, injecting drug use, past genital warts, condoms used with sex partners, sex overseas, and country of birth (Figure 2).
Figure 2. Variable importance in the prediction of chlamydia re-infection within 1 year among heterosexuals by XGBoost.
To our knowledge, this is the first study using machine learning approaches to predict chlamydia re-testing and re-infection within 1 year among heterosexuals with chlamydia. Our study found a relatively low chlamydia re-testing rate (36%) within 1 year among heterosexuals with chlamydia. Our finding was similar to a previous study reporting that about 40% were re-tested within 12 months in 25 general practice clinics in the Australian Collaboration for Chlamydia Enhanced Sentinel Surveillance system (12). Our study also found a relatively high rate of chlamydia re-infection (15%) within 1 year among heterosexuals with chlamydia. Our result is consistent with previous studies reporting that the chlamydia re-infection rate was about 10–20% of patients within 12 months (4–8). The low rate of chlamydia re-testing and high rate of chlamydia re-infection suggest that further targeted and personalized interventions are warranted among heterosexuals with chlamydia. Our logistic regression analysis showed the determinants of chlamydia re-testing and re-infection within 1 year among heterosexuals with chlamydia. Our machine learning predictive models proposed a new way of using risk prediction models to increase the low chlamydia re-testing rate and reduce the high rate of chlamydia re-infection. Despite the relatively poor prediction of chlamydia re-testing and re-infection among heterosexuals, our findings suggest that researchers could develop a wide range of machine learning algorithms to find the best algorithm for prediction. Our study also provides implications for further data collection in machine learning analysis. In this study, we used routinely collected data from the clinical setting. To improve the performance of learning models in predicting chlamydia re-testing and re-infection, researchers could pay more attention to the quality, comprehensiveness, and accuracy of data collection to avoid “garbage in, garbage out” (29). Our study provides implications for selecting machine learning algorithms and predictors for future machine learning studies in predicting chlamydia re-testing and re-infection among heterosexuals with chlamydia. Better targeting interventions for those at increased risk of re-infection may help reduce their risk or encourage re-testing to diagnose and treat their infection earlier.
We aimed to develop machine learning models to predict chlamydia re-testing behavior within 1 year among heterosexuals with chlamydia using routine questions in the clinical setting. There are already some studies that have utilized machine learning algorithms to predict sexual-health behaviors, including sexual recidivism (30), sexual desire (31), reasons for not using a condom (32), and HIV risk behaviors (33). However, limited studies use machine learning algorithms to predict sexual health service utilization behaviors. We noted a previous study that reported that machine learning approaches could predict HIV/STI re-testing after receiving clinic reminder messages among MSM (24). Another study used machine learning algorithms to predict HIV testing behavior among participants from substance use disorder treatment programs (34). Our study is the first to use machine learning approaches to predict chlamydia re-testing. Therefore, it is difficult to compare our results to others. Despite the poor prediction of chlamydia re-testing behavior, developing good predictive models for this is still necessary and important. Predicting sexual healthcare utilization behaviors (e.g., future chlamydia re-testing) may benefit the management and planning of sexual health services for high-risk populations or focus prevention interventions in advance. We hope our work could encourage more machine learning research to predict chlamydia re-testing behavior among heterosexuals with chlamydia.
We intended to develop predictive models for chlamydia re-infection within 1 year among heterosexuals using machine learning approaches. Predicting future chlamydia re-infection could inform prioritizing interventions. A previous study used machine learning algorithms that could predict chlamydia (AUC = 0.67) acquisition within 1 year among males and females (28). However, our machine learning predictive models indicated that existing self-reported and routinely collected EHRs data could not provide a high predictive value for chlamydia re-infection events within 1 year among heterosexuals with chlamydia (AUC < 0.6). Despite this, our study still provides implications for future machine learning studies focusing on predicting chlamydia re-infection. For example, our results showed that Adaboost, and XGBoost performed better than conventional logistic regression in predicting chlamydia re-infection within 1 year among heterosexuals. Besides, the low predictive performances suggest that existing self-reported and routinely collected EHR data may not include some important predictors for chlamydia re-infection, such as sexual networks and background chlamydia prevalence. Another machine learning study indicated that their models lacked data for specific sexual practices (23). Our models did not include other potential factors because these data were not routinely collected EHRs data in the clinic, such as employment status (8), cervical infection, contact with uncircumcised partners and resuming sex with an untreated partner (35, 36).
Better targeting of interventions to improve re-testing is needed, especially for those with an increased likelihood of chlamydia re-infection. Our study findings are consistent with previous studies, which also found chlamydia re-testing was associated with higher numbers of sex partners (37) and among heterosexual females (38). We also observed that sex workers showed a higher re-testing rate, probably because they had a legal requirement for 3 monthly testing. Our variable importance analyses suggest other possible predictors for future predictive models of chlamydia re-testing using machine learning approaches. The top identified predictors for chlamydia re-testing were being a current sex worker, access to Medicare, condoms used with sex partners, age, gender, triage reason as contact of STI infection, injecting drug use, sex overseas, number of sex partners, and presentation as symptomatic. We hope our preliminary work encourages more machine learning research to explore the effect of introducing additional predictors on predicting chlamydia re-testing among heterosexuals.
Our study found that the chlamydia re-infection rate was greater among individuals of younger age (18–24 years), male gender, and living with HIV. These factors were consistent with previous studies on risk factors for chlamydia infection. A previous study showed that people living with HIV had more repeat chlamydia infections in the Netherlands (39). Young age at first infection was associated with an increased risk of subsequent chlamydia infection within 1 year among female adolescents in the US (26). A previous study found that being a sex worker was associated with chlamydia (40). These findings may provide clinical and public health researchers and policymakers with a deeper understanding of the drivers of chlamydia re-infection among heterosexuals at the population level. Besides, our variable importance analyses suggest possible important predictors for future predictive models of chlamydia re-infection using machine learning approaches. Consistent with previous studies on risk factors of chlamydia infection, we found that factors such as condom use (41), having sex with a female or male partner (42), and sex workers (40) were among the important predictors included in the machine learning models.
Our study has some limitations. First, only about 36% of individuals diagnosed with chlamydia returned for another chlamydia test within 1 year. We did not have information on whether the remaining individuals were tested elsewhere, so this may be an underestimate and may lead to a selection bias in our study. Among those retested, about 15% were re-infected. It is possible that those at higher risk of re-infection may be more likely to be retested. Therefore, the chlamydia re-infection (15%) may be overestimated. Second, our findings were from a single sexual clinic; therefore, studies from different settings (e.g., those attending general practice) would be needed to verify our results. Third, the validity of the predictive factors depends on the accuracy of the self-reported information, subject to participants' recall and non-response bias. There has been substantial work on the CASI system to ensure its validity and accuracy (43). Fourth, we did not include gonorrhea and chlamydia coinfection status in this study. A previous study suggested that gonorrhea and chlamydia coinfection may increase the risk of chlamydia re-infection (36). Last, we included data up to May 2020, which might introduce selection bias due to the COVID-19 lockdown in Melbourne. The first COVID-19 lockdown started on March 30 and ended on May 12, 2020. Thus, our study included a small number of clinic consultations during the COVID-19 lockdown period. A previous study showed that the re-testing patterns and sexual practices might have changed due to COVID-19 (44).
The chlamydia re-testing rate within 1 year was relatively low among heterosexuals with chlamydia; however, the re-infection rate of chlamydia was relatively high. Our study highlights the need for innovative interventions to increase chlamydia re-testing and reduce re-infection among heterosexuals with chlamydia. Our findings elaborate on the determinants of chlamydia re-testing and re-infection within 1 year among heterosexuals with chlamydia. To our knowledge, it is the first demonstration of machine learning algorithms to identify heterosexuals with chlamydia at high risk of re-infection who could benefit from patient-delivered partner therapy. XGBoost model can improve the prediction of chlamydia re-testing and re-infection compared with traditional logistic regression. Our machine learning predictive models may provide a promising way to develop an innovative method to increase the low chlamydia re-testing rate and reduce the high rate of chlamydia re-infection.
The data analyzed in this study is subject to the following licenses/restrictions: The data is not publicly available due to privacy or ethical restrictions but will be made available on reasonable request to the corresponding author, with the permission of the Alfred Hospital Ethics Committee. Restrictions apply to the availability of these data, which were used under ethical approval for this study. Requests to access these datasets should be directed to JO, amFzb24ub25nQG1vbmFzaC5lZHU=.
The studies involving human participants were reviewed and approved by the Alfred Hospital Ethics Committee, Australia (project number: 277/20). As this was a retrospective study involving minimal risk to the privacy of the study subjects, informed consent was waived by the Alfred Hospital Ethics Committee. All identifying details of the study subjects were removed before any computational analysis.
JO, XX, and LZ conceived and designed the study. XX did data cleaning, established the models and coding, and wrote the first draft and editing. EC, JO, and LZ contributed to data cleaning. EC, CF, MC, IA, JG, JH, NC, LZ, and JO contributed to the interpretation of data and manuscript revision. All authors contributed to the preparation of the manuscript and approved the final manuscript.
This work was supported by Australian National Health and Medical Research Council Emerging Leadership Investigator Grant (Grant GNT1172873 to EC; GNT1193955 to JO), the Australian National Health and Medical Research Council Leadership Investigator Grant (Grant GNT1172900 to CF), National Natural Science Foundation of China (Grant 81950410639 to LZ), Outstanding Young Scholars Funding (Grant 3111500001 to LZ), Xi'an Jiaotong University Basic Research and Profession Grant (Grant xtr022019003 to LZ, Grant xzy032020032 to LZ), Epidemiology modeling and risk assessment (Grant 20200344 to LZ), and Xi'an Jiaotong University Young Talent Support Grant (Grant YX6J004 to LZ).
The authors would like to acknowledge Afrizal Afrizal from Melbourne Sexual Health Center for data extraction. The authors would also like to thank Zhen Yu and Zongyuan Ge at Monash University for their insightful discussion of machine learning techniques.
The authors declare that the research was conducted in the absence of any commercial or financial relationships that could be construed as a potential conflict of interest.
All claims expressed in this article are solely those of the authors and do not necessarily represent those of their affiliated organizations, or those of the publisher, the editors and the reviewers. Any product that may be evaluated in this article, or claim that may be made by its manufacturer, is not guaranteed or endorsed by the publisher.
The Supplementary Material for this article can be found online at: https://www.frontiersin.org/articles/10.3389/fpubh.2022.1031372/full#supplementary-material
1. Institute. TK. HIV, viral hepatitis and sexually transmissible infections in Australia: Annual surveillance report 2018. Available online at: https://kirby.unsw.edu.au/report/hiv-viral-hepatitis-and-sexually-transmissible-infections-australia-annual-surveillance (accessed May 07, 2017).
2. Rowley J, Vander Hoorn S, Korenromp E, Low N, Unemo M, Abu-Raddad LJ, et al. Chlamydia, gonorrhoea, trichomoniasis and syphilis: global prevalence and incidence estimates, 2016. Bul World Health Organ. (2019) 97:548–62. doi: 10.2471/BLT.18.228486
3. World Health Organization. Global Health Sector Strategy on Sexually Transmitted Infections 2016–2021: Toward Ending STIs. Geneva: World Health Organization (2016).
4. Geisler WM, Lensing SY, Press CG, Hook EW III. Spontaneous resolution of genital Chlamydia trachomatis infection in women and protection from reinfection. J Infect Dis. (2013) 207:1850–6. doi: 10.1093/infdis/jit094
5. Hosenfeld CB, Workowski KA, Berman S, Zaidi A, Dyson J, Mosure D, et al. Repeat infection with Chlamydia and gonorrhea among females: a systematic review of the literature. Sex Transm Dis. (2009) 36:478–89. doi: 10.1097/OLQ.0b013e3181a2a933
6. Xu F, Stoner BP, Taylor SN, Mena L, Tian LH, Papp J, et al. Use of home-obtained vaginal swabs to facilitate rescreening for Chlamydia trachomatis infections: two randomized controlled trials. Obstet Gynecol. (2011) 118(2 Pt 1):231–9. doi: 10.1097/AOG.0b013e3182246a83
7. Dunne EF, Chapin JB, Rietmeijer CA, Kent CK, Ellen JM, Gaydos CA, et al. Rate and predictors of repeat Chlamydia trachomatis infection among men. Sex Transm Dis. (2008) 35(11 Suppl.):S40–4. doi: 10.1097/OLQ.0b013e31817247b2
8. Walker J, Tabrizi SN, Fairley CK, Chen MY, Bradshaw CS, Twin J, et al. Chlamydia trachomatis incidence and re-infection among young women–behavioural and microbiological characteristics. PLoS ONE. (2012) 7:e37778. doi: 10.1371/journal.pone.0037778
9. Price MJ, Ades AE, De Angelis D, Welton NJ, Macleod J, Soldan K, et al. Risk of pelvic inflammatory disease following Chlamydia trachomatis infection: analysis of prospective studies with a multistate model. Am J Epidemiol. (2013). 178:484–92. doi: 10.1093/aje/kws583
10. Munari SC, Goller JL, Hellard ME, Hocking JS. Chlamydia prevention and management in Australia: reducing the burden of disease. Med J Aust. (2022) 217:499–501. doi: 10.5694/mja2.51749
11. Ong JJ, Bourne C, Dean JA, Ryder N, Cornelisse VJ, Murray S, et al. Australian sexually transmitted infection (STI) management guidelines for use in primary care 2022 update. Sex Health. (2022). doi: 10.1071/SH22134. [Epub ahead of print].
12. Bowring AL, Gouillou M, Guy R, Kong FYS, Hocking J, Pirotta M, et al. Missed opportunities—low levels of chlamydia retesting at Australian general practices, 2008–2009. Sex Transm Infect. (2012) 88:330–4. doi: 10.1136/sextrans-2011-050422
13. Lang AS, Jansen K, Sailer A, Bremer V, Dudareva S. Not again! Effect of previous test results, age group and reason for testing on (re-) infection with Chlamydia trachomatis in Germany. BMC Infect Dis. (2018) 18:424. doi: 10.1186/s12879-018-3323-2
14. Khan A, Fortenberry JD, Juliar BE, Tu W, Orr DP, Batteiger BE. The prevalence of chlamydia, gonorrhea, and trichomonas in sexual partnerships: implications for partner notification and treatment. Sex Transm Dis. (2005) 32:260–4. doi: 10.1097/01.olq.0000161089.53411.cb
15. Layton E, Goller JL, Coombe J, Temple-Smith M, Tomnay J, Vaisey A, et al. 'It's literally giving them a solution in their hands': the views of young Australians towards patient-delivered partner therapy for treating chlamydia. Sex Transm Infect. (2021) 97:256–60. doi: 10.1136/sextrans-2020-054820
16. Kissinger P, Brown R, Reed K, Salifou J, Drake A, Farley TA, et al. Effectiveness of patient delivered partner medication for preventing recurrent Chlamydia trachomatis. Sex Transm Infect. (1998) 74:331–3. doi: 10.1136/sti.74.5.331
17. Ramstedt K, Forssman L, Johannisson G. Contact tracing in the control of genital Chlamydia trachomatis infection. Int J STD AIDS. (1991) 2:116–8. doi: 10.1177/095646249100200208
18. Goller JL, Coombe J, Bourne C, Bateson D, Temple-Smith M, Tomnay J, et al. Patient-delivered partner therapy for chlamydia in Australia: can it become part of routine care? Sex Health. (2020) 17:321–9. doi: 10.1071/SH20024
20. Bzdok D, Altman N, Krzywinski M. Statistics versus machine learning. Nat Methods. (2018) 15:233–4. doi: 10.1038/nmeth.4642
21. Weng SF, Reps J, Kai J, Garibaldi JM, Qureshi N. Can machine-learning improve cardiovascular risk prediction using routine clinical data? PLoS ONE. (2017) 12:e0174944. doi: 10.1371/journal.pone.0174944
22. Mullainathan S, Spiess J. Machine learning: an applied econometric approach. J Econ Perspect. (2017) 31:87–106. doi: 10.1257/jep.31.2.87
23. Elder HR, Gruber S, Willis SJ, Cocoros N, Callahan M, Flagg EW, et al. Can machine learning help identify patients at risk for recurrent sexually transmitted infections? Sex Transm Dis. (2021) 48:56–62. doi: 10.1097/OLQ.0000000000001264
24. Xu X, Fairley CK, Chow EPF, Lee D, Aung ET, Zhang L, et al. Using machine learning approaches to predict timely clinic attendance and the uptake of HIV/STI testing post clinic reminder messages. Sci Rep. (2022) 12:8757. doi: 10.1038/s41598-022-12033-7
25. Bao Y, Medland NA, Fairley CK, Wu J, Shang X, Chow EPF, et al. Predicting the diagnosis of HIV and sexually transmitted infections among men who have sex with men using machine learning approaches. J Infect. (2020) 82:48–59. doi: 10.2139/ssrn.3550064
26. Gaydos CA, Wright C, Wood BJ, Waterfield G, Hobson S, Quinn TC. Chlamydia trachomatis reinfection rates among female adolescents seeking rescreening in school-based health centers. Sex Transm Dis. (2008) 35:233–7. doi: 10.1097/OLQ.0b013e31815c11fe
27. Parvandeh S, Yeh HW, Paulus MP, McKinney BA. Consensus features nested cross-validation. Bioinformatics. (2020) 36:3093–8. doi: 10.1093/bioinformatics/btaa046
28. Xu X, Ge Z, Chow EP, Yu Z, Lee D, Wu J, et al. A machine-learning-based risk-prediction tool for HIV and sexually transmitted infections acquisition over the next 12 months. J Clin Med. (2022) 11:1818. doi: 10.3390/jcm11071818
29. Rose LT, Fischer KW. Garbage in, garbage out: having useful data is everything. Measur Interdiscipl Res Perspect. (2011) 9:222–6. doi: 10.1080/15366367.2011.632338
30. Ozkan T, Clipper SJ, Piquero AR, Baglivio M, Wolff K. Predicting sexual recidivism. Sex Abuse. (2020) 32:375–99. doi: 10.1177/1079063219852944
31. Vowels LM, Vowels MJ, Mark KP. Uncovering the most important factors for predicting sexual desire using explainable machine learning. J Sex Med. (2021) 18:1198–216. doi: 10.1016/j.jsxm.2021.04.010
32. Govindan B, Maduravasagam K. Deploying machine learning to find out the reasons for not using condom in a questionnaire-based study of 120 patients. Indian J Sex Transm Dis AIDS. (2018) 39:50–4. doi: 10.4103/ijstd.IJSTD_64_17
33. Wray TB, Luo X, Ke J, Pérez AE, Carr DJ, Monti PM. Using smartphone survey data and machine learning to identify situational and contextual risk factors for HIV risk behavior among men who have sex with men who are not on PrEP. Prev Sci. (2019) 20:904–13. doi: 10.1007/s11121-019-01019-z
34. Pan Y, Liu H, Metsch LR, Feaster DJ. Factors associated with HIV testing among participants from substance use disorder treatment programs in the US: a machine learning approach. AIDS Behav. (2017) 21:534–46. doi: 10.1007/s10461-016-1628-y
35. Whittington WL, Kent C, Kissinger P, Oh MK, Fortenberry JD, Hillis SE, et al. Determinants of persistent and recurrent Chlamydia trachomatis infection in young women: results of a multicenter cohort study. Sex Transm Dis. (2001) 28:117–23. doi: 10.1097/00007435-200102000-00011
36. Russell AN, Zheng X, O'Connell CM, Taylor BD, Wiesenfeld HC, Hillier SL, et al. Analysis of factors driving incident and ascending infection and the role of serum antibody in chlamydia trachomatis genital tract infection. J Infect Dis. (2016) 213:523–31. doi: 10.1093/infdis/jiv438
37. Visser M, van Aar F, Koedijk FD, Kampman CJ, Heijne J. Repeat Chlamydia trachomatis testing among heterosexual STI outpatient clinic visitors in the Netherlands: a longitudinal study. BMC Infect Dis. (2017) 17:782. doi: 10.1186/s12879-017-2871-1
38. Guy R, Wand H, Franklin N, Fairley CK, Chen MY, O'Connor CC, et al. Re-testing for chlamydia at sexual health services in Australia, 2004–08. Sexual Health. (2011) 8:242–7. doi: 10.1071/SH10086
39. Wijers J, Dukers-Muijrers N, Hoebe C, Wolffs PFG, van Liere G. The characteristics of patients frequently tested and repeatedly infected with Chlamydia trachomatis in Southwest Limburg, the Netherlands. BMC Public Health. (2020) 20:1239. doi: 10.1186/s12889-020-09334-9
40. Vajdic CM, Middleton M, Bowden FJ, Fairley CK, Kaldor JM. The prevalence of genital Chlamydia trachomatis in Australia 19972004: a systematic review. Sexual Health. (2005) 2:169–83. doi: 10.1071/SH05018
41. Jasumback CL, Perry SH, Ness TE, Matsenjwa M, Masangane ZT, Mavimbela M, et al. Point-of-care testing to guide treatment and estimate risk factors for sexually transmitted infections in adolescents and young people with human immunodeficiency virus in Eswatini. Open Forum Infect Dis. (2020) 7:ofaa052. doi: 10.1093/ofid/ofaa052
42. Allan-Blitz LT, Leon SR, Bristow CC, Konda KA, Vargas SK, Flores JA, et al. High prevalence of extra-genital chlamydial or gonococcal infections among men who have sex with men and transgender women in Lima, Peru. Int J STD AIDS. (2017) 28:138–44. doi: 10.1177/0956462416630909
43. Fairley CK, Sze JK, Vodstrcil LA, Chen MY. Computer-assisted self interviewing in sexual health clinics. Sex Transm Dis. (2010) 37:665–8. doi: 10.1097/OLQ.0b013e3181f7d505
Keywords: Chlamydia trachomatis, re-testing, re-infection, heterosexual, predictive model, machine learning, variable importance, risk factors
Citation: Xu X, Chow EPF, Fairley CK, Chen M, Aguirre I, Goller J, Hocking J, Carvalho N, Zhang L and Ong JJ (2023) Determinants and prediction of Chlamydia trachomatis re-testing and re-infection within 1 year among heterosexuals with chlamydia attending a sexual health clinic. Front. Public Health 10:1031372. doi: 10.3389/fpubh.2022.1031372
Received: 29 August 2022; Accepted: 23 December 2022;
Published: 13 January 2023.
Edited by:
Nilu Goonetilleke, University of North Carolina at Chapel Hill, United StatesReviewed by:
Haidong Lu, Yale University, United StatesCopyright © 2023 Xu, Chow, Fairley, Chen, Aguirre, Goller, Hocking, Carvalho, Zhang and Ong. This is an open-access article distributed under the terms of the Creative Commons Attribution License (CC BY). The use, distribution or reproduction in other forums is permitted, provided the original author(s) and the copyright owner(s) are credited and that the original publication in this journal is cited, in accordance with accepted academic practice. No use, distribution or reproduction is permitted which does not comply with these terms.
*Correspondence: Lei Zhang, bGVpLnpoYW5nMUBtb25hc2guZWR1; Jason J. Ong,
amFzb24ub25nQG1vbmFzaC5lZHU=
†These authors have contributed equally to this work
Disclaimer: All claims expressed in this article are solely those of the authors and do not necessarily represent those of their affiliated organizations, or those of the publisher, the editors and the reviewers. Any product that may be evaluated in this article or claim that may be made by its manufacturer is not guaranteed or endorsed by the publisher.
Research integrity at Frontiers
Learn more about the work of our research integrity team to safeguard the quality of each article we publish.