- Department of Military Medical Psychology, Air Force Medical University, Xi'an, China
Background: Behavioral inhibition/activation systems (BIS/BAS) and impulsivity are associated with problematic smartphone use (PSU). However, no studies to date have explored how the subdomains of BIS/BAS and the dimensions of impulsivity relate to the components of PSU in a joint framework. This study aimed to examine the relationships between the three constructs at a fine-grained level and identify the central nodes and bridge nodes of their relationships using network analysis.
Methods: A regularized partial correlation network of PSU, BIS/BAS, and impulsivity communities was estimated to investigate the connections between variables and determine the expected influence and bridge expected influence for each variable based on data from 325 Chinese adults. PSU, BIS/BAS, and impulsivity were assessed using the Smartphone Application-Based Addiction Scale (SABAS), BIS/BAS scales, and Barratt Impulsiveness Scale-Version 11 (BIS-11), respectively.
Results: In addition to connections within each community, network analysis revealed that there were connections between different communities, especially connections to PSU. I2 “motor impulsivity” was strongly associated with PSU2 “conflict” and PSU6 “relapse”; BASR “BAS-reward responsiveness” was strongly associated with PSU5 “withdrawal.” Nodes BASR “BAS-reward responsiveness” and PSU6 “relapse” were the most central variables, while nodes BASR “BAS-reward responsiveness” and I2 “motor impulsivity” were the strongest bridge variables.
Conclusion: The connections between the subdomains of BIS/BAS and the components of PSU and between the dimensions of impulsivity and the components of PSU may be particularly important in the development and maintenance of PSU. The central variables identified here, along with the bridge variables, could be promising and effective targets for the prevention and intervention of PSU.
Introduction
The number of smartphone users has surged in recent years because smartphones offer a wide range of functions such as communication, online education, and entertainment. Taking the Chinese population as an example, the China Internet Network Information Center (CNNIC) reported that 1,029 million Chinese people used mobile phones to access the Internet as of December 2021, accounting for 99.7% of Chinese netizens (1). According to a report from Pew Research Center, in 2018, 96% of adults owned a smartphone in South Korea, which was the highest rate of smartphone ownership among the 39 countries surveyed (2). However, with the rapid growth of smartphone users, a related issue—problematic smartphone use (PSU)—has ensued. PSU refers to the compulsive and dependent use of smartphones, leading to negative consequences or impaired daily function (3, 4). The PSU has become a public concern. It has been reported that the median prevalence of PSU amongst children and young people was 23.3% (5). Following suggestions by previous researchers, the current study does not discriminate between PSU and other terms used to describe excessive smartphone use such as “smartphone addiction”; these terms are here collectively referred to as PSU (6, 7). Cumulative evidence indicates that PSU can be related to physical health problems (e.g., headaches, neck and thumb pain) (8–10) and psychological problems (e.g., anxiety and depression, sleep disorders, loneliness, and impulsivity) (11–15). Given the high prevalence and adverse results related to PSU, it is of great significance to reveal the pathogenesis of PSU. According to the Interaction of Person-Affect-Cognition-Execution (I-PACE) model, individuals' predisposing variables (e.g., specific personality trait, neurobiological characteristics, reduced executive functioning and inhibition control, etc.) make them more susceptible to developing PSU (16, 17). Importantly, two candidate transdiagnostic risk factors for PSU are behavioral inhibition/activation systems and impulsivity.
Gray's reinforcement sensitivity theory (RST) has often been used to explain an individual's predisposition to addictive behaviors (18–21). RST attempts to illuminate individual differences in personality traits from the perspective of neurophysiological mechanisms. It holds that there are two brain systems that are sensitive to punishment and rewards, respectively, and control individual behavior: the Behavioral Inhibition System (BIS) and the Behavioral Activation System (BAS) (18, 22, 23). The BIS regulates aversive motivation and is activated by punishment and by the termination of rewards, inhibiting behavior that may result in negative consequences; the BAS regulates appetitive motivation and is activated by rewards and by the termination of punishment, increasing approach behavior and generating positive emotional feelings (20, 22, 24, 25). Growing evidence indicates that BIS/BAS can be associated with addiction or addiction-like behavior, such as substance use disorder (26, 27), Internet addiction (28, 29), and Internet gaming disorder (IGD) (20, 30). Additionally, it has been reported in a prospective study that there are bidirectional interactions between addiction and BIS/BAS (31). Importantly for this study, although few related studies have been conducted, some studies have revealed that BIS/BAS is closely related to PSU and can be risk factors for PSU (25, 32–34). For example, a study has found that BIS is significantly associated with PSU through copula regression analysis (33). Overall, existing researches establish the close relationships between BIS/BAS and PSU.
Impulsivity is a multi-dimensional construct involving actions carried out quickly and without foresight, preferences for risk taking, failures of inhibitory processes, a lack of planning, and a tendency to accept small immediate rewards rather than large delayed rewards and that often results in undesirable consequences (35–39). Impulsive behavior is not always maladaptive: for example, functional impulsivity helps to complete tasks in a limited period of time, and can lead to good outcomes (36, 40). However, excessive and persistent impulsivity is a prominent risk factor for addiction (36, 40–42). It has also been reported that there is a bidirectional relationship between impulsivity and addictive behavior (43). Impulsivity is not only an important predictor of substance use disorders (36, 44, 45) but also a vulnerability factor for non-substance-related addictive disorders (40, 42, 46). Critical for this study, prior studies have revealed that impulsivity is one of the most predictive factors of PSU (3, 25, 40, 41, 47–49). For example, it has been reported that dysfunctional impulsivity is directly connected to PSU, and also indirectly connected to PSU through the mediation of sensation seeking (e.g., thrill and adventure seeking) through stepwise regression (40). Hence, impulsivity has tight relationships with PSU; in particular, it is important for developing, reinforcing, and maintaining the symptoms of PSU. Furthermore, a close relationship between the BAS of the BIS/BAS and impulsivity has been shown in substance use disorders (50, 51).
Prior studies have investigated the relationships between PSU and the BIS/BAS (25) and between PSU and impulsivity based on total scores on scales (41, 47, 49). However, this practice may obscure the variation between individual psychopathological variables and fail to reveal the relationships between the variables at a fine-grained level (52, 53). In fact, of PSU, BIS/BAS, and impulsivity, none are simple unidimensional constructs. PSU consists of six components according to the addiction components model, namely salience (preoccupation with the behavior), mood modification (mood changes brought about by the behavior), tolerance (increasing engagement in the behavior over time), withdrawal (negative feelings and physical symptoms when the behavior is blocked), conflict (interpersonal and intrapersonal relationship problems because of the behavior), and relapse (reversion to the behavior after a period of abstinence) (54, 55). As for BIS/BAS, in addition to BIS, BAS alone is comprised of three subdomains, including reward responsiveness (receptivity to actual or potential rewards), drive (persistent pursuit of goals), and fun seeking (desire for new and potentially rewarding experiences) (18, 22). Impulsivity is composed of three dimensions, including inattention (not focusing on the task at hand), motor impulsiveness (acting on the spur of the moment), and lack of planning (not planning and thinking carefully) (35, 56). Therefore, the single summative score of BIS/BAS, impulsivity, or PSU is based on the notion of variable equivalence and masks the heterogeneity between individual variables, as well as obscure the specific relationships between variables (57, 58). In order to better understand the psychopathology behind PSU, BIS/BAS, and impulsivity at a fine-grained level (especially between PSU and BIS/BAS and between PSU and impulsivity) and to pinpoint effective intervention and prevention targets for PSU, studies are warranted to investigate the relationships among the individual variables of these constructs.
Network analysis is a new and promising data-driven approach that can satisfy this requirement. It involves estimating and visualizing the complex relationships and characteristics of a system in network form (24, 52, 59). The network can consist of individual disorder symptoms (52, 59) or non-symptom factors that may contribute to the development and maintenance of a disorder, such as psychophysiological variables, cognitive process, behaviors, or different personality traits (60, 61). From the perspective of the network theoretical model, psychological constructs are characterized as networks emerging from the interactions between different variables (60, 62). Variables, whether symptoms or non-symptoms, are regarded as the nodes in the psychopathology network; the partial correlations between different variables are represented as node-to-node edges (62, 63).
Network analysis provides further understanding of the mechanisms underlying the development and maintenance of disorders (62, 64). This approach also makes it possible to identify central nodes that greatly affect other nodes across the whole network, as well as bridge nodes, which connect with nodes of other network communities (63–65). The term “community” is used to represent a group of nodes corresponding to a specific construct based on psychological theory or clinical criteria rather than based on any network analytical methods such as community detection (65, 66). Because central nodes have the greatest influence on the overall network and bridge nodes are critical to maintaining the co-occurrence of mental disorders and facilitating the contagion of one disorder to another, these nodes have been identified as more effective targets for prevention and treatment than other nodes (53, 63–65). Network analysis has been used to investigate three or more co-occurring constructs in a joint framework, including problematic Internet and smartphone use, primary emotional systems, and need satisfaction (67), as well as depressive symptoms, parental stress, and mechanistic variables (68).
However, to the best of our knowledge, no study has yet investigated the relationships between PSU, BIS/BAS, and impulsivity in a joint framework using network analysis. To fill that research gap, we used a network analysis approach to examine the interactions between PSU, BIS/BAS, and impulsivity, especially the relationships between subdomains of BIS/BAS and components of PSU and between dimensions of impulsivity and components of PSU. We constructed a PSU-BIS/BAS-Impulsivity network to explore the links among the three communities. We also calculated the expected influence (EI) and bridge expected influence (BEI) of each of the network's nodes to identify central nodes that maintain the whole network and bridge nodes that contribute to the co-occurrence of these constructs. Based on previous studies that BIS/BAS and impulsivity are vulnerability factors of PSU, we hypothesized that subdomains of BIS/BAS and dimensions of impulsivity both exist connections to components of PSU in addition to edges within each community. We also hypothesized that there exist influential nodes in this network that play important roles in the development and maintenance of PSU. In this study, we aimed to advance our understanding of the psychopathological pathways leading from BIS/BAS and impulsivity to PSU, and to determine effective prevention and therapeutic targets for PSU. Considering that no published studies have investigated the relationships between PSU, BIS/BAS, and impulsivity using network analysis, our work is largely exploratory.
Methods
Participants
This study was cross-sectional and conducted in China from 27 April 2022 to 16 May 2022. The current study used an online survey hosted on the Wenjuanxing platform (www.wjx.cn), which has been used successfully in previous studies (24, 69). The first part of the survey included the anonymity statement and informed consent form. Participants all gave their informed consent and were allowed to exit the study at any time; they were also encouraged to give honest responses to the survey. A total of 343 participants were recruited through convenience sampling based on WeChat. The inclusion criteria were: (1) healthy adults (aged 18 years or above); (2) college students (undergraduates, masters, or doctors); (3) consent to participate in the study. The exclusion criteria were: (1) a history of organic brain damage or mental disorders; (2) the time used to complete the survey was <100 s, indicating indiscriminate response without consideration. The final sample consisted of 325 participants. The study was reviewed and approved by the Medical Ethics Committee of Tangdu Hospital of the Fourth Military Medical University.
Measures
In addition to demographic variables such as age, gender, and education, participants were asked to complete scales in the online survey assessing PSU, behavioral inhibition/activation systems (BIS/BAS), and impulsivity.
Problematic smartphone use
PSU was measured using the Chinese version of the Smartphone Application-Based Addiction Scale (SABAS) (70–72). The scale consists of 6 items, with each scored from 1 = strongly disagree to 6 = strongly agree; a higher score indicates a higher level of severity of PSU. The 6 items correspond one-to-one to the 6 core criteria of the components model of addiction, namely salience, conflict, mood modification, tolerance, withdrawal, and relapse (54, 55). The Cronbach's α coefficient for this scale was 0.83 in our sample.
Behavioral inhibition/activation systems
BIS/BAS was measured using the Chinese version of the BIS/BAS scales (22, 73). The scale has 18 items in total (items 1 and 18 deleted) that are rated on a 4-point Likert-type scale ranging from 1 = strongly agree to 4 = strongly disagree (32, 51, 73, 74). The scale includes one subscale assessing BIS (5 items) and 3 subscales assessing BAS (13 items): reward responsiveness (BASR, 4 items), drive (BASD, 4 items), and fun seeking (BASF, 5 items). The Cronbach's α coefficient was 0.82 for BIS, 0.87 for BAS, 0.78 for BASR, 0.75 for BASD, and 0.65 for BASF in the current study.
Impulsivity
The valid Chinese version of the Barratt Impulsiveness Scale-Version 11 (BIS-11) was used to assess impulsivity (56, 75). This scale has been widely used in previous studies (76–78). The scale consists of 30 items and can be separated into three dimensions (each with 10 items), namely motor impulsivity, attentional impulsivity, and non-planning impulsivity. Each item is scored from 1 = never to 5 = always; however, the items of the non-planning and attentional impulsivity dimensions are inversely scored (75). The scores for each dimension and the BIS-11 all range from 0 to 100 after being converted (75). The higher the score, the higher the impulsivity. The internal consistency of the BIS-11 in this study was fairly good; the Cronbach's α coefficients of the motor impulsivity dimension, attentional impulsivity dimension, and non-planning impulsivity dimension were 0.86, 0.89, and 0.83, respectively.
Analytical procedure
SPSS 26.0 and RStudio software (version 4.1.1) were used to analyze the data. SPSS 26.0 was first used to calculate descriptive metrics and Cronbach's α coefficients. Following previous studies (53, 79, 80), the informativeness of each variable was estimated via mean of standard deviation. RStudio was then used to perform network analysis.
We estimated the structure of the networks in the current study via Gaussian graphical model (GGM) (81), namely the network structure of PSU components, BIS/BAS subdomains, and impulsivity dimensions (PSU-BIS/BAS-Impulsivity network). Within a GGM, edges are undirected and represent the partial correlation between two nodes after controlling for all remaining nodes. As recommended by a previous study (82), the estimation of network structure was based on Spearman correlations. Moreover, in order to regularize the GGM, we employed the graphical least absolute shrinkage and selection operator (LASSO) method (83). By shrinking all edges and punishing the edges of trivially small partial correlation coefficients to zero, this regularization process helps to remove spurious edges and to obtain a more stable, sparse, and easy-to-interpret network (82, 83). Furthermore, the hyperparameter of the Extended Bayesian Information Criterion (EBIC) was set to 0.5 to determine the optimal network model (82, 84, 85). The network layout was visualized using the Fruchterman-Reingold algorithm (86). In this part of the analytical procedure, we constructed and visualized the network structure using R-package qgraph (87).
Both the traditional centrality and bridge centrality measures were reported as raw values. We calculated EI, which was used in this study as the traditional centrality index, using R-package qgraph (87). EI is a more suitable than other node centrality measures for evaluating the centrality of each node and determining central nodes in a network with both negative and positive associations (88). EI is defined as the sum of all the edge weights connecting to a given node. A higher EI indicates a more important and influential node in the network. Moreover, to identify bridge nodes in the network that connect different communities or disorders (e.g., PSU, BIS/BAS, and impulsivity), BEI was computed as the bridge centrality indicator using R-package networktools (65). BEI refers to the sum of the edge weights connecting a specific node with nodes in the other communities; a higher BEI value represents a higher likelihood of contagion between communities (65). In our study, we defined three communities in the network in advance: we set the six components of PSU to form one community, the four subdomains of BIS/BAS to constitute the second community, and the three impulsivity dimensions to form the third community.
We used R-package bootnet to evaluate the robustness and accuracy of the PSU-BIS/BAS-Impulsivity network (86). First, to assess the stability of centrality measures, including node EI and node BEI, we calculated the correlation stability (CS) coefficient using a case-dropping bootstrap approach (1,000 bootstrap samples). The CS coefficient indicates sufficient stability if it is above at least 0.25 (an acceptable value), and preferably >0.5 (86). Second, we examined the accuracy of edge weights with a non-parametric bootstrap method (1,000 bootstrap samples) to compute the 95% confidence interval. A narrower 95% confidence interval indicates a more reliable network (80, 89). Finally, we applied bootstrapped difference tests (1,000 bootstrap samples) to evaluate differences in the centrality indices and edge weights.
Results
Descriptive statistics
The mean age of the participants was 21.49 years (SD = 3.73, range = 18–36 years). All participants had received a college education or above. There was an approximately equal gender distribution; 54.8% of the participants were female (n = 178), and males made up 45.2% (n = 147). The participants reported that they spent an average time of 6.62 h (SD = 3.59) on their smartphones per day. Abbreviations, mean scores, standard deviations, skewness, and kurtosis for each variable of the three constructs are shown in Table 1.
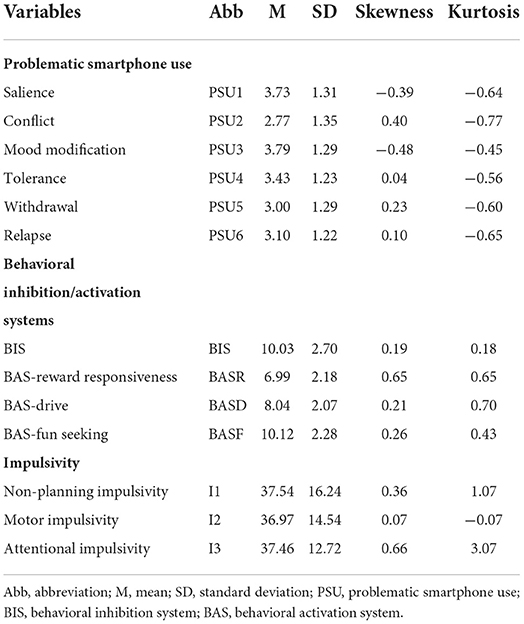
Table 1. Abbreviations, mean scores, standard deviations, skewness, and kurtosis for the study variables.
Network analysis
The level of variable informativeness was checked. No variable was found to be poorly informative, namely 2.5 SD below the mean level of informativeness (M ± SD = 1.28 ± 0.05, 2.31 ± 0.28, and 14.50 ± 1.76 for PSU, BIS/BAS, and impulsivity, respectively). Figure 1 shows the PSU-BIS/BAS-Impulsivity network. In the network with 13 nodes, 52 out of the 78 edges were non-zero, including 14 negative edges and 38 positive edges. The six strongest, positive edges in the final network were identified. Within the PSU community, the two strongest edges were between the nodes PSU1 “salience” and PSU3 “mood modification” (weight = 0.34) and between PSU4 “tolerance” and PSU6 “relapse” (weight = 0.46). Within the BIS/BAS community, the three strongest edges were the edges between the nodes BASD “BAS-drive” and BASF “BAS-fun seeking,” between BASR “BAS-reward responsiveness” and BASF “BAS-fun seeking,” and between BASR “BAS-reward responsiveness” and BASD “BAS-drive” (weight = 0.37, 0.33, 0.32, respectively). Within the impulsivity community, a particularly strong positive edge occurred between nodes I1 “non-planning impulsivity” and I3 “attentional impulsivity” (weight = 0.63). The cross-community edges were weaker than the within-community edges. Nevertheless, some relatively strong edges were identified. I2 “motor impulsivity” was positively associated with PSU2 “conflict” (weight = 0.19) and PSU6 “relapse” (weight = 0.09). BASR “BAS-reward responsiveness” was positively associated with PSU5 “withdrawal” (weight = 0.1). In addition to associations with PSU, some dimensions of impulsivity were positively correlated with subdomains of BIS/BAS. I1 “non-planning impulsivity” was linked to BASD “BAS-drive” (weight = 0.16). I2 “motor impulsivity” was linked to BASR “BAS-reward responsiveness” (weight = 0.16). All edge weights of the PSU-BIS/BAS-Impulsivity network can be seen in Supplementary Table 1. The bootstrapped 95% confidence interval for estimated edge weights is narrow, indicating that the edges were estimated accurately and reliably (see Supplementary Figure 1). The bootstrapped difference test for edge weights is shown in Supplementary Figure 2, revealing that the weights of the six strongest edges were significantly higher than ~84–100% of the weights of other edges.
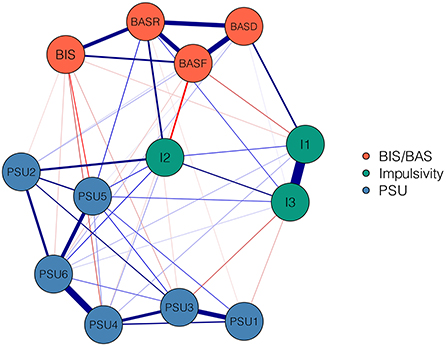
Figure 1. Network Structure of PSU, BIS/BAS, and impulsivity variables. Blue edges represent positive relations, whereas red edges represent negative relations. Thickness of edge indicates strength of relationship. The weights of edges are in Supplementary Table 1. PSU1, salience; PSU2, conflict; PSU3, mood modification; PSU4, tolerance; PSU5, withdrawal; PSU6, relapse; BIS, Behavioral Inhibition System; BASR, Behavioral Activation System-reward responsiveness; BASD, Behavioral Activation System-drive; BASF, Behavioral Activation System-fun seeking; I1, non-planning impulsivity; I2, motor impulsivity; I3, attentional impulsivity.
The results of the traditional centrality index (i.e., EI) are shown in Figure 2A. The nodes BASR “BAS-reward responsiveness” (EI = 1.22) and PSU6 “relapse” (EI = 1.13) exhibited extremely high EI, marking them as the most central nodes in the network. BIS had the lowest EI and was thus the least central node (EI = 0.17). The CS coefficient for EI was 0.59, exceeding the preferably recommended threshold of 0.5; therefore, the estimation of node EI had a good level of stability (see Supplementary Figure 3). The bootstrapped difference test showed that the EIs of BASR “BAS-reward responsiveness” and PSU6 “relapse” were significantly higher than ~75–92% of the other node EIs; no significant difference was observed in the EIs of these two nodes (see Supplementary Figure 4). To sum up, compared with other nodes, BASR “BAS-reward responsiveness” and PSU6 “relapse” were the most important and influential nodes in the network.
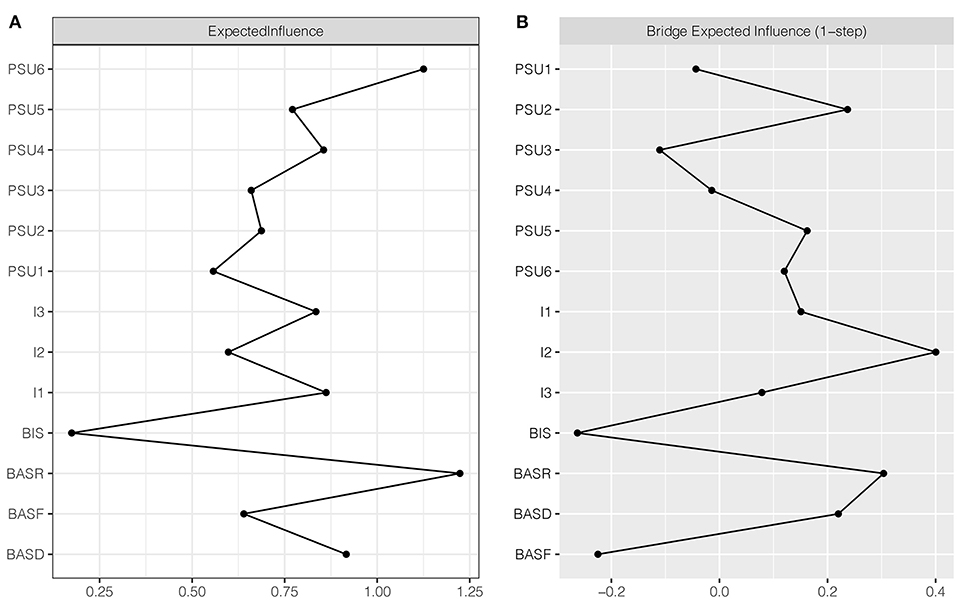
Figure 2. Centrality plot depicting the expected influence and bridge expected influence of each node in the network (raw value). (A) Expected influence. (B) Bridge expected influence. PSU1, salience; PSU2, conflict; PSU3, mood modification; PSU4, tolerance; PSU5, withdrawal; PSU6, relapse; BIS, Behavioral Inhibition System; BASR, Behavioral Activation System-reward responsiveness; BASD, Behavioral Activation System-drive; BASF, Behavioral Activation System-fun seeking; I1, non-planning impulsivity; I2, motor impulsivity; I3, attentional impulsivity.
The BEI for each node in the network is shown in Figure 2B. Two bridge nodes exhibited the highest BEI values. One was node BASR “BAS-reward responsiveness” (BEI = 0.3) in the BIS/BAS community; the other was node I2 “motor impulsivity” (BEI = 0.4) in the impulsivity community. The CS coefficient of node BEI was 0.52, indicating that the estimation of BEI was adequately stable (see Supplementary Figure 5). The bootstrapped difference test showed that the BEIs of I2 “motor impulsivity” and BASR “BAS-reward responsiveness” were significantly higher than about 42–67% of the BEIs of the other nodes (see Supplementary Figure 6). Bridge centrality emphasizes the importance of I2 “motor impulsivity” and BASR “BAS-reward responsiveness,” which had enormous influence on the interactions between different communities.
Discussion
To the best of our knowledge, this is the first study to use network analysis to investigate the relationships between PSU, BIS/BAS, and impulsivity. Based on this approach, this study developed a complex network consisting of three communities: the symptoms of PSU, the subdomains of BIS/BAS, and the dimensions of impulsivity. The strongest edges were observed within each community and there were some relatively weak edges connecting variables between the communities. These results suggest that individual variables of BIS/BAS and impulsivity are linked to specific pathways to develop and maintain PSU. The current study also explored the central nodes and bridge nodes that played important roles in this PSU-BIS/BAS-Impulsivity network. The perspective of network analysis helps to better understand how the dimensions of impulsivity and the subdomains of BIS/BAS are linked to the components of PSU and revealed effective targets for the prevention and treatment of PSU.
This study revealed that the strongest edges appeared within the communities rather than connecting different communities. This is consistent with prior studies that have shown that the strongest edges exist within each community when detecting relationships between variables in a network consisting of two or more communities (24, 53, 63, 90–93). For the PSU community, the two strongest positive edges were between PSU1 “salience” and PSU3 “mood modification” and between PSU4 “tolerance” and PSU6 “relapse”; this has also been found in a prior network research (24). The three strongest edges found within the BIS/BAS community are similar to the results found by other studies using network analysis (94). They were between BASD “BAS-drive” and BASF “BAS-fun seeking,” between BASR “BAS-reward responsiveness” and BASF “BAS-fun seeking,” and between BASR “BAS-reward responsiveness” and BASD “BAS-drive.” For the impulsivity community, the strongest edge was between I1 “non-planning impulsivity” and I3 “attentional impulsivity,” which is consistent with prior studies that revealed a positive correlation between non-planning impulsivity and attentional impulsivity (95). Additionally, it has been held that non-planning and attentional impulsivity should be regarded as forms of cognitive impulsivity, and thus conceptually different from motor impulsivity (96, 97). This may account for the strong relationship observed between non-planning and attentional impulsivity. Altogether, it is unsurprising that the strongest edges existed within each community rather than connecting different communities, because the variables of each community are sub-components of each psychological construct; these variables have close interactions with each other from a theoretical perspective.
In addition to within-community edges, we found that some variables in the impulsivity and BIS/BAS communities were associated with components of PSU (i.e., cross-community edges), which is consistent with our hypothesis. These findings provide a fine-grained understanding of the links between BIS/BAS and PSU and between impulsivity and PSU. For example, I2 “motor impulsivity” had relatively strong connections with PSU2 “conflict” and PSU6 “relapse,” which could partly account for why individuals with high impulsivity are predisposed to developing PSU (25, 41, 47, 49, 98). It has been reported that response inhibition is closely related to motor impulsivity (99–101). Response inhibition (a component of inhibition control) helps to inhibit impulsive action and to resist temptations, which facilitates adaptive and goal-directed behaviors (102–104). Excessive motor impulsivity may lead to difficulty with response inhibition. Hence, failing to resist temptations may result in relapse and acting impulsively may contribute to conflicts with others (24, 105, 106). Another important edge identified in this study was the positive connection between BASR “BAS-reward responsiveness” and PSU5 “withdrawal,” which is similar to the finding that BASR is a predictor of PSU (25). The current study further explored the possible path linking BAS and PSU, which lies in reward responsiveness and withdrawal. However, this result contradicts a previous study that showed reward responsiveness to be negatively correlated with Internet addiction (20). This inconsistency may arise from a difference in samples: the previous study was based on participants with IGD. IGD can lead to functional and structural alterations of the brain that influence reward responsiveness, contributing to differences from the healthy population (107–110). Since no studies have investigated the relationships between individual variables of BIS/BAS or impulsivity and components of PSU, the current study only provided preliminary insight into an issue certainly worth further study. In addition to edges linking to PSU, there were some edges connecting dimensions of impulsivity and subdomains of BIS/BAS. For example, I1 “non-planning impulsivity” was associated with BASD “BAS-drive,” and I2 “motor impulsivity” was associated with BASR “BAS-reward responsiveness.” These findings are in line with the view that impulsivity is conceptually related to BAS (18, 22, 111, 112). Prior studies based on measuring scales have also reported that BAS is associated with impulsivity (51, 113).
Consistent with our hypothesis that there existed influential nodes (i.e., key central nodes or bridge nodes) in the PSU-BIS/BAS-Impulsivity network, there were two key central nodes with the highest EIs (62, 88): BASR “BAS-reward responsiveness” and PSU6 “relapse.” This result demonstrates that these two variables have the greatest influence within the network and may play the most important role in activating other variables and maintaining the current psychopathological network. This finding aligns with those of previous studies, which have shown that BAS-reward responsiveness exhibits extremely high centrality (24). However, this result differs from prior studies on the centrality indices for components of PSU; relapse was identified as the central node in our study, whereas mood modification was the most central node in an earlier study (24). However, another qualitative examination has revealed that non-addicted smartphone users and addicted users are not different in the components of mood modification and relapse, but are instead different in the other four components of PSU (114). It would seem that mood modification and relapse may not be the dominant components in the development of PSU. Considering that the results of previous studies are inconsistent and confusing, our study is largely exploratory and more studies are warranted.
The BEI is used to evaluate the importance of bridge nodes when analyzing co-occurring constructs (65). Bridge nodes that connect theoretically independent constructs are crucial to understanding the development and maintenance of psychological comorbidities (63, 65). In the current study, I2 “motor impulsivity” and BASR “BAS-reward responsiveness” were identified as key bridge nodes. What interests us most was that I2 “motor impulsivity” had the highest BEI value and was more related to the PSU community than BASR “BAS-reward responsiveness.” This result suggests that motor impulsivity has a significant impact on BAS and the development and maintenance of PSU. These findings echo prior studies that have reported that impulsivity is closely related to PSU (3, 25, 41, 48, 49). Moreover, these findings also accord with some studies that have shown that impulsivity is associated with BAS (51, 113). Our results verify the relationships between impulsivity and PSU from the perspective of network analysis.
These aforementioned findings have important theoretical and clinical implications. Regarding the theoretical implications, this research revealed some edges appeared between BIS/BAS and PSU and between impulsivity and PSU such as edges between I2 “motor impulsivity” and PSU2 “conflict” and between BASR “BAS-reward responsiveness” and PSU5 “withdrawal.” These findings are of importance to figure out specific role played by different components of BIS/BAS or impulsivity in the development and maintenance of symptoms of PSU. In other words, our study shed light on the possible pathological pathways linking BIS/BAS and impulsivity to PSU. Regarding the clinical implications, network theory holds that interventions on critical central nodes may effectively disrupt the overall network and reduce the severity of the entire network, facilitating the intervention and treatment (59, 62, 64, 89). Additionally, targeting key bridge nodes may disrupt the connection between co-occurring constructs and reduce the adverse effects of one disorder on others (i.e., prevent the contagion of one disorder to others), benefiting the treatment outcomes (63–65, 115). In this study, preventing the contagion between communities may decrease the risky interactions between them that may co-lead to PSU. Regarding central nodes, we underscore the importance of taking reward responsiveness and relapse into consideration when attempting to prevent and treat PSU. As for bridge nodes, future intervention of PSU should focus on motor impulsivity and reward responsiveness. Consequently, reward responsiveness of BIS/BAS, relapse of PSU, and motor impulsivity may be effective targets for intervention and treatment of PSU.
The current study has provided preliminary insights into the fine-grained relationships between PSU, BIS/BAS, and impulsivity. Nonetheless, it has some limitations that warrant consideration. First, the results were based on data collected using self-reported scales. Although participants were encouraged to give honest responses, self-reports may nonetheless lead to subjective biases and social approval effects (91, 115). This reminds us to interpret our results cautiously. Second, the study is cross-sectional and cannot verify the causality linking the variables. Future studies should examine the causal relationships using a longitudinal experimental design. Third, similar to the second limitation, although we identified the central and bridge nodes that play important roles in the network and regard them as effective intervention targets (62, 65), it is necessary to verify using further longitudinal or experimental studies whether interventions on these variables will succeed. Fourth, we chose only healthy adults and obtained the network structure and centrality indices, and thus one should be cautious when extending the results to clinical samples. Fifth, similar to fourth limitation, given that this study used a convenient sampling method and enrolled a relatively small sample, the findings are specific to the present sample, and the generalizability of our findings to other populations needs to be established via replications in other samples. Sixth, redundant nodes refer to nodes that were highly overlapping and most likely measure the same underlying variable (116). Although it is theoretically justified that none of the variables in our study were redundant (117), it is recommended to conduct statistical redundancy analysis in such future studies. Finally, the network and its characteristics in the current study are specific to the scales we used. We assessed PSU using the SAMAS, BIS/BAS using the BIS/BAS scales, and impulsivity using the BIS-11 scale. This means that the current study did not capture all aspects of these constructs, and only provided a limited picture of the relationships between BIS/BAS or impulsivity and PSU. Future studies can consider other facets of these constructs and integrate them into a unified framework to investigate how BIS/BAS and impulsivity develop and maintain PSU.
Conclusions
This study is the first to estimate the network structure of PSU, BIS/BAS, and impulsivity based on individual variables and investigate the relationships among these constructs using network analysis. In addition to connections within each community, we found some connections between PSU and BIS/BAS and between PSU and impulsivity. This result showed that there are psychopathological pathways linking BIS/BAS and impulsivity with PSU on a fine-grained level. This study also identified some variables that are critical to the development and maintenance of PSU, including the central nodes BASR “BAS-reward responsiveness” and PSU6 “relapse,” as well as the bridge nodes I2 “motor impulsivity” and BASR “BAS-reward responsiveness”; these findings have important clinical implications, providing promising and effective targets for the prevention and intervention of PSU.
Data availability statement
The raw data supporting the conclusions of this article will be made available by the authors, without undue reservation.
Ethics statement
The studies involving human participants were reviewed and approved by the Medical Ethics Committee of Tangdu Hospital of the Fourth Military Medical University. The patients/participants provided their written informed consent to participate in this study.
Author contributions
ZG, SW, and XZ conceived the study. ZG, YH, and RQ performed the data collection. TY performed data analysis. ZG and YH wrote the draft of the manuscript. LR, SW, and XZ obtained funding and contributed to the manuscript revision. All authors contributed to the article and approved the submitted version.
Funding
This work was supported by the Key project of PLA Logistics Research Program during the 14th Five-Year Plan period (BKJ21J013).
Acknowledgments
The authors would like to thank MogoEdit (https://www.mogoedit.com) for its English editing during the preparation of this manuscript.
Conflict of interest
The authors declare that the research was conducted in the absence of any commercial or financial relationships that could be construed as a potential conflict of interest.
Publisher's note
All claims expressed in this article are solely those of the authors and do not necessarily represent those of their affiliated organizations, or those of the publisher, the editors and the reviewers. Any product that may be evaluated in this article, or claim that may be made by its manufacturer, is not guaranteed or endorsed by the publisher.
Supplementary material
The Supplementary Material for this article can be found online at: https://www.frontiersin.org/articles/10.3389/fpubh.2022.1014548/full#supplementary-material
References
1. CNNIC. The 49th Statistical Report on China's Internet Development (2022). Available online at: http://wwwcnniccn/gywm/xwzx/rdxw/20172017_7086/202202/t20220225_71724htm (accessed July 6, 2022).
2. Pew Research Center. Explore Global Rates of Internet Use, Smartphone Ownership Social Media Use over Time (2018). Available online at: https://wwwpewresearchorg/global/2018/06/19/social-media-use-continues-to-rise-in-developing-countries-but-plateaus-across-developed-ones/#table (accessed July 6, 2022).
3. Billieux J. Problematic use of the mobile phone: a literature review and a pathways model. Curr Psychiatry Rev. (2012) 8:299–307. doi: 10.2174/157340012803520522
4. Horwood S, Anglim J. Personality and problematic smartphone use: a facet-level analysis using the five factor model and hexaco frameworks. Comput Human Behav. (2018) 85:349–59. doi: 10.1016/j.chb.2018.04.013
5. Sohn SY, Rees P, Wildridge B, Kalk NJ, Carter B. Prevalence of problematic smartphone usage and associated mental health outcomes amongst children and young people: a systematic review, meta-analysis and grade of the evidence. BMC Psychiatry. (2019) 19:356. doi: 10.1186/s12888-019-2350-x
6. Panova T, Carbonell X. Is smartphone addiction really an addiction? J Behav Addict. (2018) 7:252–9. doi: 10.1556/2006.7.2018.49
7. Huang S, Lai X, Xue Y, Zhang C, Wang Y. A network analysis of problematic smartphone use symptoms in a student sample. J Behav Addict. (2020) 9:1032–43. doi: 10.1556/2006.2020.00098
8. Demir YP, Sumer MM. Effects of smartphone overuse on headache, sleep and quality of life in migraine patients. Neurosciences. (2019) 24:115–21. doi: 10.17712/nsj.2019.2.20180037
9. Lee JI, Song HS. The correlation analysis between hours of smartphone use and neck pain in the gachon university students. Acupuncture. (2014) 31:99–109. doi: 10.13045/acupunct.2014028
10. Inal E, Demirci K, Çetintürk A, Akgönül M, Savaş S. Effects of smartphone overuse on hand function, pinch strength, and the median nerve: smartphone overuse. Muscle Nerve. (2015) 52:183–8. doi: 10.1002/mus.24695
11. Kim S, Park J, Kim H, Pan Z, Lee Y, McIntyre R. The relationship between smartphone addiction and symptoms of depression, anxiety, and attention-deficit/hyperactivity in south korean adolescents. Ann Gen Psychiatry. (2019) 18:1. doi: 10.1186/s12991-019-0224-8
12. Cui G, Yin Y, Li S, Chen L, Liu X, Tang K, et al. Longitudinal relationships among problematic mobile phone use, bedtime procrastination, sleep quality and depressive symptoms in chinese college students: a cross-lagged panel analysis. BMC Psychiatry. (2021) 21:449. doi: 10.1186/s12888-021-03451-4
13. Yayan E, Suna Dag Y, Düken M. The effects of technology use on working young loneliness and social relationships. Perspect Psychiatr Care. (2019) 55:194–200. doi: 10.1111/ppc.12318
14. Amiri M, Dowran B, Salimi H, Zarghami MH. The problematic use of mobile phone and mental health: a review study in Iran. J Educ Health Promot. (2020) 9:290. doi: 10.4103/jehp.jehp_268_20
15. Li Y, Li G, Liu L, Wu H. Correlations between mobile phone addiction and anxiety, depression, impulsivity, and poor sleep quality among college students: a systematic review and meta-analysis. J Behav Addict. (2020) 9:551–71. doi: 10.1556/2006.2020.00057
16. Brand M, Young KS, Laier C, Wölfling K, Potenza MN. Integrating psychological and neurobiological considerations regarding the development and maintenance of specific internet-use disorders: an interaction of person-affect-cognition-execution (I-Pace) model. Neurosci Biobehav Rev. (2016) 71:252–66. doi: 10.1016/j.neubiorev.2016.08.033
17. Brand M, Wegmann E, Stark R, Müller A, Wölfling K, Robbins TW, et al. The interaction of person-affect-cognition-execution (I-Pace) model for addictive behaviors: update, generalization to addictive behaviors beyond internet-use disorders, and specification of the process character of addictive behaviors. Neurosci Biobehav Rev. (2019) 104:1–10. doi: 10.1016/j.neubiorev.2019.06.032
18. Gray JA. A critique of eysenck's theory of personality. In:Eysenck HJ, , editor. A Model for Personality. Berlin: Springer Berlin Heidelberg (1981). p. 246–76.
19. Gray JA. The psychophysiological basis of introversion-extraversion. Behav Res Ther. (1970) 8:249–66. doi: 10.1016/0005-7967(70)90069-0
20. Xiang H, Tian X, Zhou Y, Chen J, Potenza MN, Zhang Q. The relationship between behavioral inhibition and behavioral activation systems, impulsiveness, and internet gaming disorder among students of different ages. Front Psychiatry. (2021) 11:560142. doi: 10.3389/fpsyt.2020.560142
21. Andreassen CS, Torsheim T, Brunborg GS, Pallesen S. Development of a facebook addiction scale. Psychol Rep. (2012) 110:501–17. doi: 10.2466/02.09.18.PR0.110.2.501-517
22. Carver CS, White TL. Behavioral inhibition, behavioral activation, and affective responses to impending reward and punishment: the bis/bas scales. J Pers Soc Psychol. (1994) 67:319–33.
23. Franken I, Muris P. Bis/Bas personality characteristics and college students' substance use. Person Ind Differen. (2006) 40:1497–503. doi: 10.1016/j.paid.2005.12.005
24. Gao L, Zhao W, Chu X, Chen H, Li W. A network analysis of the relationships between behavioral inhibition/activation systems and problematic mobile phone use. Front Psychiatry. (2022) 13:832933. doi: 10.3389/fpsyt.2022.832933
25. Kim Y, Jeong J-E, Cho H, Jung D-J, Kwak M, Rho MJ, et al. Personality factors predicting smartphone addiction predisposition: behavioral inhibition and activation systems, impulsivity, and self-control. PLoS ONE. (2016) 11:e0159788. doi: 10.1371/journal.pone.0159788
26. Perry RI, Krmpotich T, Thompson LL, Mikulich-Gilbertson SK, Banich MT, Tanabe J. Sex modulates approach systems and impulsivity in substance dependence. Drug Alcohol Depend. (2013) 133:222–7. doi: 10.1016/j.drugalcdep.2013.04.032
27. Balconi M, Finocchiaro R, Campanella S. Reward sensitivity, decisional bias, and metacognitive deficits in cocaine drug addiction. J Addict Med. (2014) 8:399–406. doi: 10.1097/adm.0000000000000065
28. Nam CR, Lee DH, Lee JY, Choi AR, Chung SJ, Kim D-J, et al. The role of resilience in internet addiction among adolescents between sexes: a moderated mediation model. J Clin Med. (2018) 7:222. doi: 10.3390/jcm7080222
29. Li Q, Dai W, Zhong Y, Wang L, Dai B, Liu X. The mediating role of coping styles on impulsivity, behavioral inhibition/approach system, and internet addiction in adolescents from a gender perspective. Front Psychol. (2019) 10:2402. doi: 10.3389/fpsyg.2019.02402
30. Rho MJ, Lee H, Lee T-H, Cho H, Jung D, Kim D-J, et al. Risk factors for internet gaming disorder: psychological factors and internet gaming characteristics. Int J Environ Res Public Health. (2018) 15:40. doi: 10.3390/ijerph15010040
31. Yen J-Y, Yen C-F, Chen C-S, Chang Y-H, Yeh Y-C, Ko C-H. The bidirectional interactions between addiction, behaviour approach and behaviour inhibition systems among adolescents in a prospective study. Psychiatry Res. (2012) 200:588–92. doi: 10.1016/j.psychres.2012.03.015
32. Jeong B, Lee JY, Kim BM, Park E, Kwon J-G, Kim D-J, et al. Associations of personality and clinical characteristics with excessive internet and smartphone use in adolescents: a structural equation modeling approach. Addict Behav. (2020) 110:106485. doi: 10.1016/j.addbeh.2020.106485
33. Lee M, Chung S, Lee Y, Park S, Kwon J, Kim D, et al. Investigation of correlated internet and smartphone addiction in adolescents: copula regression analysis. Int J Environ Res Public Health. (2020) 17:5806. doi: 10.3390/ijerph17165806
34. Jiang Z, Zhao X. Brain behavioral systems, self-control and problematic mobile phone use: the moderating role of gender and history of use. Pers Individ Dif. (2017) 106:111–6. doi: 10.1016/j.paid.2016.10.036
35. Moeller FG, Barratt ES, Dougherty DM, Schmitz JM, Swann AC. Psychiatric aspects of impulsivity. Am J Psychiatry. (2001) 158:1783–93. doi: 10.1176/appi.ajp.158.11.1783
36. Dalley JW, Everitt BJ, Robbins TW. Impulsivity, compulsivity, and top-down cognitive control. Neuron. (2011) 69:680–94. doi: 10.1016/j.neuron.2011.01.020
37. Bari A, Robbins TW. Inhibition and impulsivity: behavioral and neural basis of response control. Prog Neurobiol. (2013) 108:44–79. doi: 10.1016/j.pneurobio.2013.06.005
38. Nigg JT. Annual research review: on the relations among self-regulation, self-control, executive functioning, effortful control, cognitive control, impulsivity, risk-taking, and inhibition for developmental psychopathology. J Child Psychol Psychiatry. (2017) 58:361–83. doi: 10.1111/jcpp.12675
39. Morris LA, O'Callaghan C, Le Heron C. Disordered decision making: a cognitive framework for apathy and impulsivity in huntington's disease. Mov Disord. (2022) 37:1149–63. doi: 10.1002/mds.29013
40. De P, Albeniz Garrote G, Rubio L, Medina Gomez B, Buedo-Guirado C. Smartphone abuse amongst adolescents: the role of impulsivity and sensation seeking. Front Psychol. (2021) 12:746626. doi: 10.3389/fpsyg.2021.746626
41. De-Sola J, Talledo H, Rubio G, Rodriguez de Fonseca F. Psychological factors and alcohol use in problematic mobile phone use in the spanish population. Front Psychiatry. (2017) 8:11. doi: 10.3389/fpsyt.2017.00011
42. Dalley J, Robbins T. Fractionating impulsivity: neuropsychiatric implications. Nat Rev Neurosci. (2017) 18:158–71. doi: 10.1038/nrn.2017.8
43. Kaiser A, Bonsu JA, Charnigo RJ, Milich R, Lynam DR. Impulsive personality and alcohol use: bidirectional relations over one year. J Stud Alcohol Drugs. (2016) 77:473–82. doi: 10.15288/jsad.2016.77.473
44. Rodríguez-Cintas L, Daigre C, Grau-López L, Barral C, Pérez-Pazos J, Voltes N, et al. Impulsivity and addiction severity in cocaine and opioid dependent patients. Addict Behav. (2016) 58:104–9. doi: 10.1016/j.addbeh.2016.02.029
45. Mayer JT, Chopard G, Nicolier M, Gabriel D, Masse C, Giustiniani J, et al. Can transcranial direct current stimulation (Tdcs) improve impulsivity in healthy and psychiatric adult populations? A systematic review. Prog Neuro Psychopharmacol Biol Psychiatry. (2020) 98:109814. doi: 10.1016/j.pnpbp.2019.109814
46. Weinstein A, Abu H, Timor A, Mama Y. Delay discounting, risk-taking, and rejection sensitivity among individuals with internet and video gaming disorders. J Behav Addict. (2016) 5:674–82. doi: 10.1556/2006.5.2016.081
47. Billieux J, Linden M, Rochat L. The role of impulsivity in actual and problematic use of the mobile phone. Appl Cogn Psychol. (2010) 22:1195–210. doi: 10.1002/acp.1429
48. De-Sola Gutiérrez J, Rodríguez de. Fonseca F, Rubio G. Cell-phone addiction: a review. Front Psychiatry. (2016) 7:175. doi: 10.3389/fpsyt.2016.00175
49. Peterka-Bonetta J, Sindermann C, Elhai JD, Montag C. Personality associations with smartphone and internet use disorder: a comparison study including links to impulsivity and social anxiety. Front Public Health. (2019) 7:127. doi: 10.3389/fpubh.2019.00127
50. Dawe S, Loxton N. The role of impulsivity in the development of substance use and eating disorders. Neurosci Biobehav Rev. (2004) 28:343–51. doi: 10.1016/j.neubiorev.2004.03.007
51. Che Q, Yang P, Gao H, Liu M, Zhang J, Cai T. Application of the Chinese version of the bis/bas scales in participants with a substance use disorder: an analysis of psychometric properties and comparison with community residents. Front Psychol. (2020) 11:912. doi: 10.3389/fpsyg.2020.00912
52. Beard C, Millner AJ, Forgeard MJ, Fried EI, Hsu KJ, Treadway MT, et al. Network analysis of depression and anxiety symptom relationships in a psychiatric sample. Psychol Med. (2016) 46:3359–69. doi: 10.1017/s0033291716002300
53. Cai H, Bai W, Sha S, Zhang L, Chow IHI, Lei S-M, et al. Identification of central symptoms in internet addictions and depression among adolescents in macau: a network analysis. J Affect Disord. (2022) 302:415–23. doi: 10.1016/j.jad.2022.01.068
54. Griffiths MD. A 'components' model of addiction within a biopsychosocial framework. J Subst Use. (2005) 10:191–7. doi: 10.1080/14659890500114359
55. Csibi S, Griffiths M, Demetrovics Z, Szabo A. Analysis of problematic smartphone use across different age groups within the 'components model of addiction'. Int J Ment Health Addict. (2019) 19:616–31. doi: 10.1007/s11469-019-00095-0
56. Patton JH, Stanford MS, Barratt ES. Factor structure of the barratt impulsiveness scale. J Clin Psychol. (1995) 51:768–74.
57. Fried EI. Problematic assumptions have slowed down depression research: why symptoms, not syndromes are the way forward. Front Psychol. (2015) 6:309. doi: 10.3389/fpsyg.2015.00309
58. Fried EI, Epskamp S, Nesse RM, Tuerlinckx F, Borsboom D. What are 'good' depression symptoms? Comparing the centrality of dsm and non-dsm symptoms of depression in a network analysis. J Affect Disord. (2016) 189:314–20. doi: 10.1016/j.jad.2015.09.005
59. Borsboom D, Cramer AO. Network analysis: an integrative approach to the structure of psychopathology. Annu Rev Clin Psychol. (2013) 9:91–121. doi: 10.1146/annurev-clinpsy-050212-185608
60. Jones PJ, Heeren A, McNally RJ. Commentary: a network theory of mental disorders. Front Psychol. (2017) 8:1305. doi: 10.3389/fpsyg.2017.01305
61. Marcus DK, Preszler J, Zeigler-Hill V. A network of dark personality traits: what lies at the heart of darkness? J Res Person. (2017) 2017:56–62. doi: 10.1016/j.jrp.2017.11.003
62. Borsboom D. A network theory of mental disorders. World Psychiatry. (2017) 16:5–13. doi: 10.1002/wps.20375
63. Haws JK, Brockdorf AN, Gratz KL, Messman TL, Tull MT, DiLillo D. Examining the associations between ptsd symptoms and aspects of emotion dysregulation through network analysis. J Anxiety Disord. (2022) 86:102536. doi: 10.1016/j.janxdis.2022.102536
64. Byrne ME, Tanofsky-Kraff M, Lavender JM, Parker MN, Shank LM, Swanson TN, et al. Bridging executive function and disinhibited eating among youth: a network analysis. Int J Eating Disord. (2021) 54:721–32. doi: 10.1002/eat.23476
65. Jones PJ, Ma R, McNally RJ. Bridge centrality: a network approach to understanding comorbidity. Multivariate Behav Res. (2021) 56:353–67. doi: 10.1080/00273171.2019.1614898
66. Hoffman M, Steinley D, Gates KM, Prinstein MJ, Brusco MJ. Detecting clusters/communities in social networks. Multivariate Behav Res. (2018) 53:57–73. doi: 10.1080/00273171.2017.1391682
67. Rozgonjuk D, Davis KL, Montag C. The roles of primary emotional systems and need satisfaction in problematic internet and smartphone use: a network perspective. Front Psychol. (2021) 12:709805. doi: 10.3389/fpsyg.2021.709805
68. Skjerdingstad N, Johnson MS, Johnson SU, Hoffart A, Ebrahimi OV. Feelings of worthlessness links depressive symptoms and parental stress: a network analysis during the COVID-19 pandemic. Eur Psychiatry. (2021) 64:e50. doi: 10.1192/j.eurpsy.2021.2223
69. Yuan H, Ren L, Ma Z, Li F, Liu J, Jin Y, et al. Network structure of ptsd symptoms in chinese male firefighters. Asian J Psychiatr. (2022) 72:103062. doi: 10.1016/j.ajp.2022.103062
70. Csibi S, Griffiths MD, Cook B, Demetrovics Z, Szabo A. The psychometric properties of the smartphone application-based addiction scale (Sabas). Int J Ment Health Addict. (2018) 16:393–403. doi: 10.1007/s11469-017-9787-2
71. Leung H, Pakpour AH, Strong C, Lin YC, Tsai MC, Griffiths MD, et al. Measurement invariance across young adults from Hong Kong and Taiwan among three internet-related addiction scales: bergen social media addiction scale (Bsmas), smartphone application-based addiction scale (Sabas), and internet gaming disorder scale-short form (Igds-Sf9) (Study Part a). Addict Behav. (2020) 101:105969. doi: 10.1016/j.addbeh.2019.04.027
72. Yam C-W, Pakpour AH, Griffiths MD, Yau W-Y, Lo C-LM, Ng JMT, et al. Psychometric testing of three Chinese online-related addictive behavior instruments among Hong Kong university students. Psychiatr Q. (2019) 90:117–28. doi: 10.1007/s11126-018-9610-7
73. Li Y, Zhang Y, Jiang Y, Li H, Mi S, Yi G, et al. The Chinese version of the bis/bas scale: reliability and validity. Chinese Mental Health Journal. (2008) 22:613–6. doi: 10.3724/SP.J.1041.2008.00418
74. Wang X, Zhang R, Chen X, Liu K, Wang L, Zhang J, et al. Psychopathological correlates and emotion regulation as mediators of approach and avoidance motivation in a Chinese military sample. Front Psychiatry. (2019) 10:149. doi: 10.3389/fpsyt.2019.00149
75. Li XY, Phillips MR, Dong XU, YaLi Z, ShaoJie Y, YongSheng T, et al. Reliability and validity of an adapted Chinese version of barratt impulsiveness scale. Chin Mental Health J. (2011) 25:610–5. doi: 10.1007/s12583-011-0163-z
76. Raiha S, Yang GC, Wang LX, Dai WN, Wu HY, Meng GT, et al. Altered reward processing system in internet gaming disorder. Front Psychiatry. (2020) 11:599141. doi: 10.3389/fpsyt.2020.599141
77. Ran HL, Fang D, Donald AR, Wang R, Che YS, He XT, et al. Impulsivity mediates the association between parenting styles and self-harm in chinese adolescents. BMC Public Health. (2021) 21:332. doi: 10.1186/s12889-021-10386-8
78. Wang YG, Chen S, Xu ZM, Shen ZH, Wang YQ, He XY, et al. Family history of suicide and high motor impulsivity distinguish suicide attempters from suicide ideators among college students. J Psychiatr Res. (2017) 90:21–5. doi: 10.1016/j.jpsychires.2017.02.006
79. Marchetti I. Hopelessness: a network analysis. Cognit Ther Res. (2019) 43:611–9. doi: 10.1007/s10608-018-9981-y
80. Mullarkey M, Marchetti I, Beevers C. Using network analysis to identify central symptoms of adolescent depression. J Clin Child Adol Psychol. (2019) 48:656–68. doi: 10.1080/15374416.2018.1437735
81. Epskamp S, Waldorp L, Mõttus R, Borsboom D. The gaussian graphical model in cross-sectional and time-series data. Multivariate Behav Res. (2018) 53:453–80. doi: 10.1080/00273171.2018.1454823
82. Epskamp S, Fried E. A tutorial on regularized partial correlation networks. Psychol Methods. (2018) 23:617–34. doi: 10.1037/met0000167
83. Friedman J, Hastie T, Tibshirani R. Sparse inverse covariance estimation with the graphical lasso. Biostatistics. (2008) 9:432–41. doi: 10.1093/biostatistics/kxm045
84. Foygel R, Drton M. Extended bayesian information criteria for gaussian graphical models. Adv Neural Inf Process Syst. (2010) 23:2020–8. doi: 10.48550/arXiv.1011.6640
85. Chen J, Chen Z. Extended bayesian information criteria for model selection with large model spaces. Biometrika. (2008) 95:759–71. doi: 10.1093/biomet/asn034
86. Epskamp S, Borsboom D, Fried EI. Estimating psychological networks and their accuracy: a tutorial paper. Behav Res Methods. (2018) 50:195–212. doi: 10.3758/s13428-017-0862-1
87. Epskamp S, Cramer A, Waldorp LJ, Schmittmann VD, Borsboom D. Qgraph: network visualizations of relationships in psychometric data. J Stat Softw. (2012) 48:367–71. doi: 10.18637/jss.v048.i04
88. Robinaugh D, Millner A, McNally R. Identifying highly influential nodes in the complicated grief network. J Abnorm Psychol. (2016) 125:747–57. doi: 10.1037/abn0000181
89. Yang Y, Zhang D-Y, Li Y-L, Zhang M, Wang P-H, Liu X-H, et al. Prevalence, correlates, and network analysis of internet addiction symptoms among chinese pregnant and postpartum women. J Affect Disord. (2022) 298:126–33. doi: 10.1016/j.jad.2021.10.092
90. Ren L, Wang Y, Wu L, Wei Z, Cui L-B, Wei X, et al. Network structure of depression and anxiety symptoms in chinese female nursing students. BMC Psychiatry. (2021) 21:279. doi: 10.1186/s12888-021-03276-1
91. Ren L, Wei Z, Li Y, Cui L-B, Wang Y, Wu L, et al. The relations between different components of intolerance of uncertainty and symptoms of generalized anxiety disorder: a network analysis. BMC Psychiatry. (2021) 21:448. doi: 10.1186/s12888-021-03455-0
92. Yuan GF, Shi W, Elhai JD, Montag C, Chang K, Jackson T, et al. Gaming to cope: applying network analysis to understand the relationship between posttraumatic stress symptoms and internet gaming disorder symptoms among disaster-exposed Chinese young adults. Addict Behav. (2022) 124:107096. doi: 10.1016/j.addbeh.2021.107096
93. Park S-C, Kim D. The centrality of depression and anxiety symptoms in major depressive disorder determined using a network analysis. J Affect Disord. (2020) 271:19–26. doi: 10.1016/j.jad.2020.03.078
94. Lin S-Y, Eaton NR, Schleider JL. Unpacking associations between mood symptoms and screen time in preadolescents: a network analysis. J Abnorm Child Psychol. (2020) 48:1635–47. doi: 10.1007/s10802-020-00703-x
95. Jakuszkowiak-Wojten K, Landowski J, Wiglusz MS, Cubala WJ. Impulsivity and panic disorder: an exploratory study of psychometric correlates. Psychiatr Danub. (2015) 27(Suppl. 1):S456–8.
96. Antonelli F, Ray N, Strafella A. Impulsivity and Parkinson's disease: more than just disinhibition. J Neurol Sci. (2011) 310:202–7. doi: 10.1016/j.jns.2011.06.006
97. Koh J, Kaneoke Y, Donishi T, Ishida T, Sakata M, Hiwatani Y, et al. Increased large-scale inter-network connectivity in relation to impulsivity in Parkinson's disease. Sci Rep. (2020) 10:11418. doi: 10.1038/s41598-020-68266-x
98. Lane HY, Chang CJ, Huang CL, Chang YH. An investigation into smartphone addiction with personality and sleep quality among university students. Int J Environ Res Public Health. (2021) 18:7588. doi: 10.3390/ijerph18147588
99. Veit R, Schag K, Schopf E, Borutta M, Kreutzer J, Ehlis AC, et al. Diminished prefrontal cortex activation in patients with binge eating disorder associates with trait impulsivity and improves after impulsivity-focused treatment based on a randomized controlled impuls trial. NeuroImage Clin. (2021) 30:102679. doi: 10.1016/j.nicl.2021.102679
100. Poulton A, Chen LPE, Dali G, Fox M, Hester R. Web-based independent versus laboratory-based stop-signal task performance: within-subjects counterbalanced comparison study. J Med Internet Res. (2022) 24:e32922. doi: 10.2196/32922
101. Whelan R, Conrod P, Poline J, Lourdusamy A, Banaschewski T, Barker G, et al. Adolescent impulsivity phenotypes characterized by distinct brain networks. Nat Neurosci. (2012) 15:920–5. doi: 10.1038/nn.3092
102. Verbruggen F, Logan GD. Response inhibition in the stop-signal paradigm. Trends Cogn Sci. (2008) 12:418–24. doi: 10.1016/j.tics.2008.07.005
103. Diamond A. Executive functions. Annu Rev Psychol. (2013) 64:135–68. doi: 10.1146/annurev-psych-113011-143750
104. Verbruggen F, Aron AR, Band GPH, Beste C, Bissett PG, Brockett AT, et al. A consensus guide to capturing the ability to inhibit actions and impulsive behaviors in the stop-signal task. Elife. (2019) 8:e46323. doi: 10.7554/eLife.46323
105. Czapla M, Simon J, Richter B, Kluge M, Friederich H, Herpertz S, et al. The impact of cognitive impairment and impulsivity on relapse of alcohol-dependent patients: implications for psychotherapeutic treatment. Addict Biol. (2016) 21:873–84. doi: 10.1111/adb.12229
106. Lo Buono V, Palmeri R, Stroscio G, Corallo F, Di Lorenzo G, Sorbera C, et al. The effect on deep brain stimulation of subthalamic nucleus and dopaminergic treatment in Parkinson disease. Medicine. (2020) 99:e21578. doi: 10.1097/md.0000000000021578
107. Yao Y-W, Liu L, Worhunsky PD, Lichenstein S, Ma S-S, Zhu L, et al. Is monetary reward processing altered in drug-naive youth with a behavioral addiction? findings from internet gaming disorder. NeuroImage Clin. (2020) 26:102202. doi: 10.1016/j.nicl.2020.102202
108. Lee D, Park J, Namkoong K, Kim IY, Jung Y-C. Gray matter differences in the anterior cingulate and orbitofrontal cortex of young adults with internet gaming disorder: surface-based morphometry. J Behav Addict. (2018) 7:21–30. doi: 10.1556/2006.7.2018.20
109. Ma S-S, Worhunsky PD, Xu J-S, Yip SW, Zhou N, Zhang J-T, et al. Alterations in functional networks during cue-reactivity in internet gaming disorder. J Behav Addict. (2019) 8:277–87. doi: 10.1556/2006.8.2019.25
110. Zhang J-T, Yao Y-W, Potenza MN, Xia C-C, Lan J, Liu L, et al. Altered resting-state neural activity and changes following a craving behavioral intervention for internet gaming disorder. Sci Rep. (2016) 6:28109. doi: 10.1038/srep28109
111. Newman JP. Reaction to punishment in extraverts and psychopaths: implications for the impulsive behavior of disinhibited individuals. J Res Pers. (1987) 21:464–80.
112. Wallace JF, Newman JP, Bachorowski JA. Failures of response modulation: impulsive behavior in anxious and impulsive individuals. J Res Pers. (1991) 25:23–44.
113. Caseras X. The measurement of individual differences in behavioural inhibition and behavioural activation systems: a comparison of personality scales. Person Ind Differen. (2003) 34:999–1013. doi: 10.1016/S0191-8869(02)00084-3
114. Jameel S, Shahnawaz MG, Griffiths MD. Smartphone addiction in students: a qualitative examination of the components model of addiction using face-to-face interviews. J Behav Addict. (2019) 8:780–93. doi: 10.1556/2006.8.2019.57
115. Huang S, Lai X, Li Y, Luo Y, Wang Y. Understanding juveniles' problematic smartphone use and related influencing factors: a network perspective. J Behav Addict. (2021) 10:811–26. doi: 10.1556/2006.2021.00048
116. Christensen AP, Golino H, Silvia PJ. A psychometric network perspective on the validity and validation of personality trait questionnaires. Eur J Pers. (2020) 34:1095–108. doi: 10.1002/per.2265
117. Rhemtulla M, van Bork R, Cramer AOJ. Cross-Lagged Network Models. Multivariate Behavioral Research (2022). Available online at: https://osfio/r24q6 (accessed September 15, 2022).
Keywords: problematic smartphone use, behavioral inhibition system, behavioral activation system, impulsivity, network analysis
Citation: Guo Z, He Y, Yang T, Ren L, Qiu R, Zhu X and Wu S (2022) The roles of behavioral inhibition/activation systems and impulsivity in problematic smartphone use: A network analysis. Front. Public Health 10:1014548. doi: 10.3389/fpubh.2022.1014548
Received: 08 August 2022; Accepted: 27 September 2022;
Published: 19 October 2022.
Edited by:
Yonghui Li, Institute of Psychology (CAS), ChinaReviewed by:
Shiva Soraya, Iran University of Medical Sciences, IranDongfang Wang, South China Normal University, China
Copyright © 2022 Guo, He, Yang, Ren, Qiu, Zhu and Wu. This is an open-access article distributed under the terms of the Creative Commons Attribution License (CC BY). The use, distribution or reproduction in other forums is permitted, provided the original author(s) and the copyright owner(s) are credited and that the original publication in this journal is cited, in accordance with accepted academic practice. No use, distribution or reproduction is permitted which does not comply with these terms.
*Correspondence: Xia Zhu, emh1eGlhJiN4MDAwNDA7Zm1tdS5lZHUuY24=; Shengjun Wu, d3VzaGomI3gwMDA0MDtmbW11LmVkdS5jbg==
†These authors share first authorship