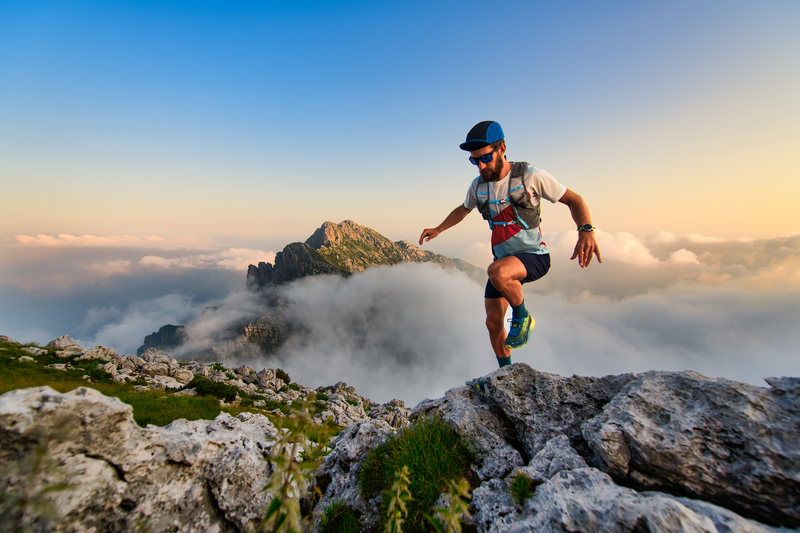
95% of researchers rate our articles as excellent or good
Learn more about the work of our research integrity team to safeguard the quality of each article we publish.
Find out more
ORIGINAL RESEARCH article
Front. Public Health , 20 December 2022
Sec. Aging and Public Health
Volume 10 - 2022 | https://doi.org/10.3389/fpubh.2022.1008137
Purpose: The purpose of this study was to investigate the clinical and non-clinical characteristics that may affect the early death rate of patients with metastatic colorectal carcinoma (mCRC) and develop accurate prognostic predictive models for mCRC.
Method: Medical records of 35,639 patients with mCRC diagnosed from 2010 to 2019 were obtained from the SEER database. All the patients were randomly divided into a training cohort and a validation cohort in a ratio of 7:3. X-tile software was utilized to identify the optimal cutoff point for age and tumor size. Univariate and multivariate logistic regression models were used to determine the independent predictors associated with overall early death and cancer-specific early death caused by mCRC. Simultaneously, predictive and dynamic nomograms were constructed. Moreover, logistic regression, random forest, CatBoost, LightGBM, and XGBoost were used to establish machine learning (ML) models. In addition, receiver operating characteristic curves (ROCs) and calibration plots were obtained to estimate the accuracy of the models. Decision curve analysis (DCA) was employed to determine the clinical benefits of ML models.
Results: The optimal cutoff points for age were 58 and 77 years and those for tumor size of 45 and 76. A total of 15 independent risk factors, namely, age, marital status, race, tumor localization, histologic type, grade, N-stage, tumor size, surgery, radiation, chemotherapy, bone metastasis, brain metastasis, liver metastasis, and lung metastasis, were significantly associated with the overall early death rate of patients with mCRC and the cancer-specific early death rate of patients with mCRC, following which nomograms were constructed. The ML models revealed that the random forest model accurately predicted outcomes, followed by logistic regression, CatBoost, XGBoost, and LightGBM models. Compared with other algorithms, the random forest model provided more clinical benefits than other models and can be used to make clinical decisions in overall early death and specific early death caused by mCRC.
Conclusion: ML algorithms combined with nomograms may play an important role in distinguishing early deaths owing to mCRC and potentially help clinicians make clinical decisions and follow-up strategies.
Colorectal carcinoma (CRC) is an aggressive malignant tumor and the third most common malignancy. It is the fourth leading contributor to cancer-related deaths in the world. In 2020, there were more than 1.1 million new CRC cases, and about 570,000 deaths were caused by CRC (1). Research has revealed that by 2030, the number of newly diagnosed patients with CRC is expected to increase to more than 2.2 million and the number of deaths to 1.1 million (2). Distant metastasis is the main leading cause of poor prognosis in patients with CRC, and about 25% of patients with CRC have been found to have distant metastasis at initial diagnosis (3). The risk of developing CRC depends on lifestyle, behavioral characteristics, and genetic factors. With the widespread knowledge of physical examination, the availability of treatments (surgical resection, chemotherapy, radiotherapy, and immunotherapy), and the discovery of early biomarkers, the prognosis of patients with CRC has improved significantly (4, 5). However, the prognosis of patients with mCRC is strikingly poor: The 5-year survival rate is only 10%, and the median survival time is about 5 months (6). Therefore, it is of great importance to identify risk factors of early death in patients with mCRC.
Clinical and pathological variables such as age, sex, race, and tumor size have been recognized as risk factors for cancer (7). The nomogram was found to be an advanced approach capable of predicting individual oncologic prognosis based on comprehensive characteristics (8). Moreover, as an emerging intersectional method, ML is adept at relating multiple variables and accurately predicting outcomes (9). Therefore, multiple ML predictive models have recently been used in disease diagnosis, prognostic prediction, and clinical decision-making (10, 11).
The purpose of this research was not only to use nomograms to evaluate the factors contributing to early death in patients with mCRC but also to find an approach with higher precision and clinical applicability for predicting early death in patients with mCRC based on machine learning algorithms, which could potentially help clinicians make clinical decisions and follow-up strategies.
Surveillance, Epidemiology, and End Results (SEER, https://seer.cancer.gov/) is the National Cancer Institute's open public database that contains cancer incidence and survival data of 17 established cancer registries across the United States and accounts for approximately 26.5% of incidence and survival rates of patients with cancer (12). In this study, SEER*Stat software (version 8.4.0) was used to extract clinical data of patients with mCRC from 2010 to 2019 (reference number 11788-Nov2021). The inclusion criteria for patients with mCRC in this investigation were as follows: (1) patients with tumor location codes of C18.0, C18.2–18.7, C19.9, and C20.9; (2) patients confirmed with stage IV CRC by histopathology; (3) patients aged 18–99 years old; (4) patients with only one primary site; and (5) patients with complete information on survival status. Patients diagnosed only by autopsy were excluded.
The screening process is shown in Figure 1. According to previous studies, early death was defined as death of patients within 3 months of diagnosis (13–15). All the included patients with mCRC were divided into a training cohort (accounting for 70%) and a validation cohort (accounting for 30%). X-tile was used to calculate the optimal cutoff point of patients' age and tumor size (16).
We compared the characteristics of the training and validation groups and analyzed the factors to predict early death in patients using univariate logistic analysis. Subsequently, significant variables were evaluated using stepwise multivariate logistic regression analysis, and the independent predictors associated with early death in patients were determined. According to the nomogram, the probability of early death in patients can be calculated. Moreover, the likelihood of early death in patients can be estimated by using the dynamic nomograms. To ensure the stability of the model, 10-fold cross-validation was used to evaluate the predictive ability of the model. Our model was then repeatedly tested and tuned, and the parameters to obtain the optimal model were determined. Independent predictors were included in five ML algorithms, and AUC was calculated to identify the top performing ML model. The differences between AUCs were compared by a bootstrap test. Calibration plots and DCA were used to assess calibration capability and clinical benefits, respectively.
Demographic and clinical factors were described by numbers and percentages. A pie chart was used to show the overall distribution of the data in the study. Pearson's chi-square test was used to evaluate the clinicopathological variables between the training and validation cohorts. Variables with a P < 0.05 in the univariate logistic analysis were screened for multivariate stepwise logistic regression to identify the possible independent risk factors. In addition, multicollinearity diagnostics in statistical modeling was performed by evaluating correlations, variance inflation factors, and eigenvalues. The forest plot obtained by using the R package “forestplot” showed the multivariate logistic analysis results of overall early death and cancer-specific early death, respectively. Nomograms were constructed from the results of univariate and multivariate analyses using the “rms” package. Simultaneously, a more flexible and better visualized dynamic nomogram was obtained through the “DynNom” package. In this study, three newly developed gradient boosting models (GBMs), namely, CatBoost, LightGBM, and XGBoost, and random forest and logistic regression models were implemented by “CatBoost,” “LightGBM,” “XGBoost,” “random forest,” and “rms” packages, respectively. The “pROC,” “rms,” and “rmda” packages were used to generate ROC, calibration, and DCA curves, respectively (https://github.com/mdbrown/rmda). All statistical analyses were performed using R software (version 4.2.1, http://www.r-project.org/).
A total of 35,639 patients with mCRC were included in this study, and the patients were randomly divided into the training cohort (n = 24,948) and validation cohort (n = 10,691). The results analyzed by X-tile software revealed that the optimal cutoff point for age was 58 and 77 years, and the optimal cutoff point for tumor size was 45 and 76 (Figure 2). The data distribution was displayed by pie chart (Supplementary Figure S1).
Figure 2. Estimation of the appropriate cutoff value for age (A) and tumor size (B) by X-tile analysis.
As shown in Table 1, 29.2% (10,396/35,639) of patients with mCRC have died within 3 months after diagnosis, and 26.8% (9,535/35,639) of the patients died of cancer. Most of the patients with mCRC were white (75.3%) and with household incomes of $55,000–$69,999 (39.6%) and >$70,000 (33.1%), and liver metastasis was the most common type (75%) when compared with bone (6.7%), brain (1.5%), and lung (26.7%) metastases. Only few of the patients with mCRC received radiation therapy (11.7%), whereas many of the patients preferred chemotherapy (63.3%). The tumor was more commonly located in the right colon (34.3%) than in the left colon (28.2%), transverse colon (6.3%), rectosigmoid (9.5%), and rectum (21.8%). The early death rate was higher in white people (76.2%) than in other ethnic groups and was higher in the right colon (39.2%) than in the left colon (26.1%), transverse colon (7.6%), rectosigmoid (9.1%), and rectum (17.9%). Treatments including surgery, radiation, and chemotherapy significantly reduced early death in patients with mCRC.
There were no significant differences in age, sex, marital status, race, median household income, tumor localization, histologic type, grade-stage, TN-stage (AJCC 8th version), tumor size, surgery, radiotherapy, chemotherapy, non-primary surgery, bone metastasis, brain metastasis, liver metastasis, and lung metastasis between the training and testing cohorts, with all p > 0.05 (Table 2). Therefore, the training and validation cohorts could be used for the follow-up research.
In the training cohort, the risk factors linked to the overall early death and cancer-specific early death of patients with mCRC were analyzed using univariate and multivariate logistic regression analyses. Univariate logistic analysis revealed that age at diagnosis, marital status, race, tumor localization, histologic type, grade, T-stage, N-stage, tumor size, surgery, non-primary surgery, radiation therapy, chemotherapy, bone, brain, liver, and lung metastasis were all associated with overall early death and cancer-specific early death of patients with mCRC all p < 0.05 (Table 3). The significant factors found by the univariate logistic analysis were included in the stepwise multivariate logistic regression, and the results illustrated that general characteristics (age, marital status, and race), tumor localization, histologic type, grade, N-stage, tumor size, and treatments (surgery, radiation, and chemotherapy), and metastases (bone, brain, liver, and lung) were independent risk factors of overall early death and cancer-specific early death of patients with mCRC, with all p < 0.05. The results of multivariate logistic regression were shown by forest plot (Figure 3). The results of multicollinearity diagnostic tests (pairwise correlations, variance inflation factors plot, and eigenvalues plot) revealed that there were no severe multicollinearity issues (Supplementary Figures S2, S3).
Table 3. Univariate logistic analysis of overall early death and cancer-specific early death in patients with mCRC.
Figure 3. Independent predictors of stepwise logistic regression models predict overall early death (A) and cancer-specific early death (B).
Predictive nomograms were constructed according to the results of the stepwise multivariate logistic regression analysis. In the nomogram prediction models, chemotherapy had the greatest predictive value, followed by brain metastases, surgery, tumor localization, grade, and bone metastases in overall early death and cancer-specific early death (Figure 4). The odds of early death in patients with mCRC can be predicted by calculating the scores of each factor. Dynamic nomograms for total early death that could assist researchers and clinicians can be accessed at https://xiaoz7474.shinyapps.io/DynNomapp_all_cause_early_death/, and those for cancer-specific early death can be accessed at https://xiaoz7474.shinyapps.io/DynNomapp_cancer_specific_early_death/.
Figure 4. Nomogram of overall early death (A) and cancer-specific early death (B) of patients with mCRC.
To determine the accuracy of our predictive nomogram model, novel ML algorithms were applied in the validation cohort (n = 10,691). The feature importance of random forest is shown in Figure 5. As shown in Figure 6, when it comes to overall early death and cancer-specific early death, the random forest model had the best performance with AUC values of 0.861 and 0.852, respectively, when compared with the XGBoost (AUC = 0.848, 0.838, respectively), LightGBM (AUC = 0.844, 0.834, respectively), CatBoost models (AUC = 0.851, 0.840, respectively), and logistic regression (AUC = 0.852, 0.842, respectively). According to the pairwise statistical comparisons between AUC values in all-cause early death (Table 4), the observed inter-individual differences in the overall performance of random forest, CatBoost, and logistic regression were statistically significantly higher than those of XGBoost and LightGBM. However, there is a slight difference in cancer-specific early death (Table 5), and the observed inter-individual differences in the overall performance of random forest were statistically significantly higher than those of logistic regression, XGBoost, CatBoost, and LightGBM. The learning rate and maximum depth used for each ML model are shown in Supplementary Table S1.
Figure 5. Feature importance of the random forest. Overall early death (A) and cancer-specific early death (B).
Table 4. P-values of the pairwise statistical comparisons of the ML model AUC (overall early death) values derived from the bootstrap test.
Table 5. P-values of the pairwise statistical comparisons of the ML model AUC (cancer-specific early death) values derived from the bootstrap test.
The calibration plots of the five algorithms were subsequently constructed. We found that the lines of the validation cohort of overall early death and cancer-specific early death were all around the 45° ideal line, which showed that these algorithms had a certain predictive value. Moreover, the overall early death and cancer-specific early death in patients with mCRC predicted by the random forest model had the strongest agreement with the observed results, followed by the logistic regression, CatBoost, XGBoost, and LightGBM models (Figure 7).
Figure 7. Calibration plots of ML models. Overall early death (A) and cancer-specific early death (B).
The DCA plots of overall early death and cancer-specific early death revealed that the random forest model most accurately predicted clinical outcomes, followed by the logistic regression, CatBoost, XGBoost, and LightGBM models (Figure 8).
Combining the results of ROC, calibration, and DCA curves, our study showed that the prediction models of novel ML algorithms constructed based on the aforementioned factors had higher precision and clinical applicability for predicting overall early death and cancer-specific early death in patients with mCRC than the logistic regression model.
CRC is the fourth leading contributor to cancer-related deaths in the world. With the rapid development of treatments and the prolonged median survival time of patients with CRC (17), the early death rate is still up to 20.7%, based on the SEER database, as revealed by this research. CRC is prone to distant metastases, and lung, liver, bone, and brain are the most frequently metastatic sites (18). mCRC in more than 65% of patients recur after surgical treatment (19). The long-term survival of patients with mCRC has attracted wide public attention; however, studies focusing on early death in patients with mCRC are rare and should be conducted (20, 21).
The risk of developing mCRC rests on different factors including lifestyle, behavioral characteristics, and genetic factors. Large cohort studies have found that the consumption of alcohol and red meat, smoking, obesity, low levels of physical activity, and inflammatory bowel diseases are risk factors of mCRC (22, 23). Donnelly et al. (24) used univariate and multivariable analyses and found that old age, being unmarried, and living alone formed the independent risk factors of CRC in the United Kingdom. Moreover, Tai et al. utilized Cox analysis and found that eight factors including age, grade, surgery, and primary site were significant prognostic factors of mCRC (20), which is consistent with our research. However, compared with previous studies, our study has some distinct advantages. First, the population included in this study was larger, which makes the results more reliable. Second, this is the first research that combined nomograms and ML models to estimate the prognosis of patients with mCRC. Third, the constructed models were validated via a validation cohort, which makes the models more stable and reliable.
Clinically, patients with mCRC are classified according to the TNM staging system, which is recognized as the standard method for cancer staging and provides the basis for therapeutic decisions (25). However, there are some limitations in the TNM staging system. When assessing patient prognosis, it only emphasizes distant metastases, lymph node involvement, and tumor site, while other factors such as tumor size, chemotherapy, and surgery are not considered (26, 27). Therefore, in this study, nomograms and ML models were integrated with different clinical features to comprehensively estimate survival of patients with mCRC.
The entire population included our research was obtained from the SEER database, and the patients were randomly divided into the training cohort (accounting for 70%) and validation cohort (accounting for 30%). The overall early death rate of patients with mCRC was 29.2%, and the cancer-specific death rate of patients with mCRC was 26.8%. Previous research revealed that clinical and non-clinical information including age, gender, and marital status were regarded as prognostic predictors for CRC (28). In addition to these factors, our research showed that the early death in patients with mCRC was mostly associated with chemotherapy and metastatic status. Consistent with the study of Ge (29), our results showed that the overall early death in patients with left-sided mCRC is better than that in patients with right-sided mCRC, with a hazard ratio of 0.84 and a p < 0.001. Researchers have found that microsatellite instability and gene expression are different for different sites of colon cancer (30, 31), which may explain why CRC at different sites has different prognoses. Our results illustrated that patients with mCRC of higher grades have higher hazard ratios. As it is known, the higher the grade, the higher the degree of malignancy and the worse the prognosis. The tumor histologic differentiation grade can provide a reference for prognosis judgment and clinical treatment. Previous studies have shown that surgery significantly improves the 5-year OS of patients with mCRC (32). Tumor size is also a vital variate factor in determining the prognosis of patients with CRC. Studies have illustrated the correlation between tumor size and survival in colon cancer (26, 33), and we found that tumor size was an independent prognostic factor in patients with mCRC.
Studies focusing on early death have been applied to many advanced cancers, and it is of great importance in cancer management. Wang et al. developed a nomogram to predict the early death of patients with stage IV CRC and found that the areas under the curve were up to 75.7% (34). In Zhu et al. (14) established a nomogram model, which is an insightful method in distinguishing the early death of patients with metastatic gastric cancer. These studies have illustrated the significant predictive ability of nomograms in predicting early death in patients with cancer.
In recent years, ML has been efficient at handling multiple variables and has been widely used in cancer detection and prediction (35, 36). In this research, ML models were constructed, and ROC, calibration, and DCA were utilized to evaluate the function of the models. The results revealed that the random forest model accurately predicted outcomes, followed by logistic regression, CatBoost, XGBoost, and LightGBM models. In summary, this research analyzed the risk factors of early death in patients with mCRC and used dynamic nomograms and novel ML algorithms to construct prognostic models. The models were efficient in predicting the prognosis of patients with mCRC and can potentially help clinicians make clinical decisions and follow-up strategies.
Although the results of this study are promising, there are several limitations to this study. First, the model is based on machine learning algorithms, so the clinical interpretation of the important features screened out by the model may be difficult. Second, the model is based on the SEER database, which only contains data of North American populations, so there may be gaps in population applicability, necessitating the inclusion of broader populations in future studies. Third, this study is retrospective, and thus, prospective clinical data are needed to provide more reliable evidence for the clinical application of this study.
Predictive nomograms and novel ML algorithms could provide a new method for accurately predicting the early death of patients with mCRC.
The original contributions presented in the study are included in the article/Supplementary material, further inquiries can be directed to the corresponding author.
Ethical review and approval was not required for the study on human participants in accordance with the local legislation and institutional requirements. Written informed consent for participation was not required for this study in accordance with the national legislation and the institutional requirements.
YZ and ZZ: conceptualization, project administration, and funding acquisition. YZ: methodology, investigation, and supervision. ZZ: software, formal analysis, data curation, and writing—original draft preparation. YZ, ZZ, and LW: validation. LW: resources. YZ and SW: manuscript—reviewing and editing. SW: visualization. All authors have read and agreed to the published version of the manuscript. All authors contributed to the article and approved the submitted version.
The authors declare that the research was conducted in the absence of any commercial or financial relationships that could be construed as a potential conflict of interest.
All claims expressed in this article are solely those of the authors and do not necessarily represent those of their affiliated organizations, or those of the publisher, the editors and the reviewers. Any product that may be evaluated in this article, or claim that may be made by its manufacturer, is not guaranteed or endorsed by the publisher.
The Supplementary Material for this article can be found online at: https://www.frontiersin.org/articles/10.3389/fpubh.2022.1008137/full#supplementary-material
1. Sung H, Ferlay J, Siegel RL, Laversanne M, Soerjomataram I, Jemal A, et al. Global cancer statistics 2020: GLOBOCAN estimates of incidence and mortality worldwide for 36 cancers in 185 countries. CA Cancer J Clin. (2021) 71:209–49. doi: 10.3322/caac.21660
2. Buccafusca G, Proserpio I, Tralongo AC, Giuliano SR, Tralongo P. Early colorectal cancer: diagnosis, treatment and survivorship care. Crit Rev Oncol Hematol. (2019) 136:20–30. doi: 10.1016/j.critrevonc.2019.01.023
3. Van Cutsem E, Oliveira J. Advanced colorectal cancer: ESMO clinical recommendations for diagnosis, treatment and follow-up. Ann Oncol. (2009) 20(Suppl. 4):61–3. doi: 10.1093/annonc/mdp130
4. Fan A, Wang B, Wang X, Nie Y, Fan D, Zhao X, et al. Immunotherapy in colorectal cancer: current achievements and future perspective. Int J Biol Sci. (2021) 17:3837–49. doi: 10.7150/ijbs.64077
5. Yoshino T, Arnold D, Taniguchi H, Pentheroudakis G, Yamazaki K, Xu R-H, et al. Pan-Asian adapted ESMO consensus guidelines for the management of patients with metastatic colorectal cancer: a JSMO-ESMO initiative endorsed by CSCO, KACO, MOS, SSO TOS. Ann Oncol. (2018) 29:44–70. doi: 10.1093/annonc/mdx738
6. Kawamura H, Yamaguchi T, Yano Y, Hozumi T, Takaki Y, Matsumoto H, et al. Characteristics and prognostic factors of bone metastasis in patients with colorectal cancer. Dis Colon Rectum. (2018) 61:673–8. doi: 10.1097/DCR.0000000000001071
7. Neazy SA, Mikwar Z, Sameer AS, Alghamdi K, Alowaydhi HM, Hashim RT, et al. Risk factors, clinical manifestations and treatment outcomes of colon cancer patients in National Guard Hospital in Jeddah, Saudi Arabia. Cureus. (2021) 13:e18150. doi: 10.7759/cureus.18150
8. Balachandran, VP, Gonen M, Smith JJ, DeMatteo RP. Nomograms in oncology: more than meets the eye. Lancet Oncol. (2015) 16:e173–80. doi: 10.1016/S1470-2045(14)71116-7
9. Wei L, Huang Y, Chen Z, Li J, Huang G, Qin X, et al. A novel machine learning algorithm combined with multivariate analysis for the prognosis of renal collecting duct carcinoma. Front Oncol. (2021) 11:777735. doi: 10.3389/fonc.2021.777735
10. May M. Eight ways machine learning is assisting medicine. Nat Med. (2021) 27:2–3. doi: 10.1038/s41591-020-01197-2
11. Goecks J, Jalili V, Heiser LM, Gray JW. How machine learning will transform biomedicine. Cell. (2020) 181:92–101. doi: 10.1016/j.cell.2020.03.022
12. Warren JL, Klabunde CN, Schrag D, Bach PB, Riley GF. Overview of the SEER-Medicare data: content, research applications, and generalizability to the United States elderly population. Med Care. (2002) 40:Iv-3-18. doi: 10.1097/00005650-200208001-00002
13. Zhang Z, Pu J, Zhang H. Development and validation of a simple-to-use nomogram to predict early death in metastatic pancreatic adenocarcinoma. Front Oncol. (2021) 11:729175. doi: 10.3389/fonc.2021.729175
14. Zhu Y, Fang X, Wang L, Zhang T, Yu D. A predictive nomogram for early death of metastatic gastric cancer: a retrospective study in the SEER database and China. J Cancer. (2020) 11:5527–35. doi: 10.7150/jca.46563
15. Chen T, Zhan X, Du J, Liu X, Deng W, Zhao S, et al. A simple-to-use nomogram for predicting early death in metastatic renal cell carcinoma: a population-based study. Front Surg. (2022) 9:871577. doi: 10.3389/fsurg.2022.871577
16. Camp RL, Dolled-Filhart M, Rimm DL. X-tile: a new bio-informatics tool for biomarker assessment and outcome-based cut-point optimization. Clin Cancer Res. (2004) 10:7252–9. doi: 10.1158/1078-0432.CCR-04-0713
17. Kou FR, Zhang YZ, Xu WR. Prognostic nomograms for predicting overall survival and cause-specific survival of signet ring cell carcinoma in colorectal cancer patients. World J Clin Cases. (2021) 9:2503–18. doi: 10.12998/wjcc.v9.i11.2503
18. Wang J, Li S, Liu Y, Zhang C, Li H, Lai B. Metastatic patterns and survival outcomes in patients with stage IV colon cancer: a population-based analysis. Cancer Med. (2020) 9:361–73. doi: 10.1002/cam4.2673
19. van der Stok EP, Spaander MCW, Grünhagen DJ, Verhoef C, Kuipers EJ. Surveillance after curative treatment for colorectal cancer. Nat Rev Clin Oncol. (2017) 14:297–315. doi: 10.1038/nrclinonc.2016.199
20. Tai Q, Xue W, Li M, Zhuo S, Zhang H, Fang F, et al. Survival nomogram for metastasis colon cancer patients based on SEER database. Front Genet. (2022) 13:832060. doi: 10.3389/fgene.2022.832060
21. Lee SHF, Rahman HA, Abidin N, Ong SK, Leong E, Naing L. Survival of colorectal cancer patients in Brunei Darussalam: comparison between 2002-09 and 2010-17. BMC Cancer. (2021) 21:477. doi: 10.1186/s12885-021-08224-6
22. Yuhara H, Steinmaus C, Cohen SE, Corley DA, Tei Y, Buffler PA. Is diabetes mellitus an independent risk factor for colon cancer and rectal cancer? Am J Gastroenterol. (2011) 106:1911–21. quiz 1922. doi: 10.1038/ajg.2011.301
23. Johnson CM, Wei C, Ensor JE, Smolenski DJ, Amos CI, Levin B, et al. Meta-analyses of colorectal cancer risk factors. Cancer Causes Control. (2013) 24:1207–22. doi: 10.1007/s10552-013-0201-5
24. Donnelly C, Hart N, McCrorie AD, Donnelly M, Anderson L, Ranaghan L, et al. Predictors of an early death in patients diagnosed with colon cancer: a retrospective case-control study in the UK. BMJ Open. (2019) 9:e026057. doi: 10.1136/bmjopen-2018-026057
25. Hari DM, Leung AM, Lee J-H, Sim M-S, Vuong B, Chiu CG, et al. AJCC Cancer Staging Manual 7th edition criteria for colon cancer: do the complex modifications improve prognostic assessment? J Am Coll Surg. (2013) 217:181–90. doi: 10.1016/j.jamcollsurg.2013.04.018
26. Feng H, Lyu Z, Zheng J, Zheng C, Wu DQ, Liang W, et al. Association of tumor size with prognosis in colon cancer: a Surveillance, Epidemiology, and End Results (SEER). Database Anal Surgery. (2021) 169:1116–23. doi: 10.1016/j.surg.2020.11.011
27. Guevara-Cuellar CA, Soto-Rojas VE, Echeverry-Molina MI, Gómez M, Martínez P. Optimal Allocation of Chemotherapy Schemes for Metastatic Colon Cancer in Colombia. Value Health Reg Issues. (2021) 26:105–12. doi: 10.1016/j.vhri.2021.01.006
28. Wong JCT, Lau JYW, Suen BY, Ng SC, Wong MCS, Tang RSY, et al. Prevalence, distribution, and risk factor for colonic neoplasia in 1133 subjects aged 40-49 undergoing screening colonoscopy. J Gastroenterol Hepatol. (2017) 32:92–7. doi: 10.1111/jgh.13450
29. Ge H, Yan Y, Xie M, Guo L, Tang D. Construction of a nomogram to predict overall survival for patients with M1 stage of colorectal cancer: a retrospective cohort study. Int J Surg. (2019) 72:96–101. doi: 10.1016/j.ijsu.2019.10.021
30. Glebov OK, Rodriguez LM, Nakahara K, Jenkins J, Cliatt J, Humbyrd C-J, et al. Distinguishing right from left colon by the pattern of gene expression. Cancer Epidemiol Biomarkers Prev. (2003) 12:755–62.
31. Papagiorgis PC, Zizi AE, Tseleni S, Oikonomakis IN, Nikiteas NI. The pattern of epidermal growth factor receptor variation with disease progression and aggressiveness in colorectal cancer depends on tumor location. Oncol Lett. (2012) 3:1129–35. doi: 10.3892/ol.2012.621
32. Chua TC, Morris DL. Therapeutic potential of surgery for metastatic colorectal cancer. Scand J Gastroenterol. (2012) 47:258–68. doi: 10.3109/00365521.2012.640823
33. Kornprat P, Pollheimer MJ, Lindtner RA, Schlemmer A, Rehak P, Langner C. Value of tumor size as a prognostic variable in colorectal cancer: a critical reappraisal. Am J Clin Oncol. (2011) 34:43–9. doi: 10.1097/COC.0b013e3181cae8dd
34. Wang X, Mao M, Xu G, Lin F, Sun P, Baklaushev VP, et al. The incidence, associated factors, and predictive nomogram for early death in stage IV colorectal cancer. Int J Colorectal Dis. (2019) 34:1189–201. doi: 10.1007/s00384-019-03306-1
35. Ngiam KY, Khor IW. Big data and machine learning algorithms for health-care delivery. Lancet Oncol. (2019) 20:e262–73. doi: 10.1016/S1470-2045(19)30149-4
Keywords: metastatic colorectal cancer, dynamic nomogram, novel machine learning, early death, SEER
Citation: Zhang Y, Zhang Z, Wei L and Wei S (2022) Construction and validation of nomograms combined with novel machine learning algorithms to predict early death of patients with metastatic colorectal cancer. Front. Public Health 10:1008137. doi: 10.3389/fpubh.2022.1008137
Received: 31 July 2022; Accepted: 28 November 2022;
Published: 20 December 2022.
Edited by:
Yutian Zou, Sun Yat-sen University Cancer Center (SYSUCC), ChinaCopyright © 2022 Zhang, Zhang, Wei and Wei. This is an open-access article distributed under the terms of the Creative Commons Attribution License (CC BY). The use, distribution or reproduction in other forums is permitted, provided the original author(s) and the copyright owner(s) are credited and that the original publication in this journal is cited, in accordance with accepted academic practice. No use, distribution or reproduction is permitted which does not comply with these terms.
*Correspondence: Shujing Wei, c2h1amluZ3dlaTAwMUAxNjMuY29t
†These authors have contributed equally to this work and share first authorship
Disclaimer: All claims expressed in this article are solely those of the authors and do not necessarily represent those of their affiliated organizations, or those of the publisher, the editors and the reviewers. Any product that may be evaluated in this article or claim that may be made by its manufacturer is not guaranteed or endorsed by the publisher.
Research integrity at Frontiers
Learn more about the work of our research integrity team to safeguard the quality of each article we publish.