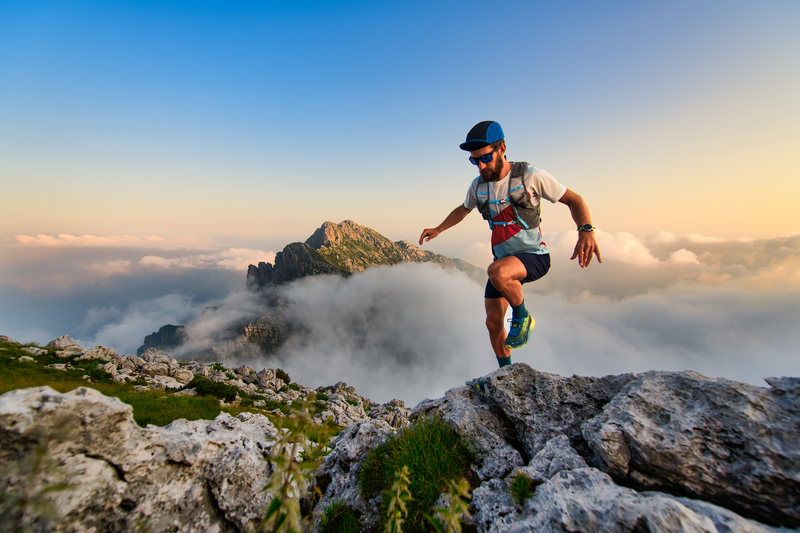
94% of researchers rate our articles as excellent or good
Learn more about the work of our research integrity team to safeguard the quality of each article we publish.
Find out more
ORIGINAL RESEARCH article
Front. Public Health , 26 September 2022
Sec. Aging and Public Health
Volume 10 - 2022 | https://doi.org/10.3389/fpubh.2022.1006955
Background: Sepsis remains the leading cause of postoperative death in elderly patients and is defined as organ dysfunction with proven or suspected infection according to Sepsis-3 criteria. To better avoid potential non-linear associations between the risk factors, we firstly used a tree-based analytic methods to explore the putative risk factors of geriatric sepsis based on the criteria in the study.
Methods: Data of 7,302 surgical patients aged ≥ 65 years at the Third Affiliated Hospital of Sun Yat-sen University from January 2015 to September 2020 were collected. An analytic method that combined tree-based analysis with the method of Mantel-Haenszel and logistic regression was adopted to assess the association between 17 putative risk factors and postoperative sepsis defined by the Sepsis-3 guideline by controlling 16 potential confounding factors.
Results: Among the 16 potential covariates, six major confounders were statistically identified by the tree-based model, including cerebrovascular diseases, preoperative infusion of red blood cells, pneumonia, age ≥ 75, malignant tumor and diabetes. Our analysis indicated that emergency surgery increases the risk of postoperative sepsis in elderly patients by more than six times. The type of surgery is also a crucial risk factor for sepsis, particularly transplantation and neurosurgery. Other risk factors were duration of surgery > 120 min, administration of steroids, hypoalbuminemia, elevated creatinine, blood urea nitrogen, hematocrit, platelets, glucose, white blood cell count, abnormal neutrophil-to-lymphocyte ratio and elevated hsCRP-to-albumin ratio.
Conclusions: Our study uses an effective method to explore some risk factors for postoperative sepsis in elderly by adjusting many potential confounders and it can provide information for intervention design.
Sepsis is defined as organ dysfunction in patients with proven or suspected infection (1) and about 30% occurs after surgery (2). Sepsis remains the leading cause of postoperative mortality in surgical patients (3), particularly the elderly patients that are more susceptible to sepsis and septic shock (4, 5). The incidence of sepsis has been reported to be disproportionately higher in the elderly, and both short- and long-term survival and prognosis in older adult with postoperative sepsis were significantly worse compared with that in the young population (6, 7). As the population ages, age of patients undergoing surgery has been increasing, severe sepsis has been termed “a quintessential disease of aged” (8). Thus, geriatric sepsis after surgery represents a substantial health care burden worldwide and early prediction and intervention help to improve the outcomes of geriatric postoperative sepsis (9).
Previous definitions of sepsis were based largely on systemic inflammatory response syndrome (SIRS) and resulted in about 12.5% missed diagnosis (10) as well as misdiagnosis due to high sensitivity. The newly proposed Sepsis-3 criteria emphasize the importance of organ dysfunction determined by Sequential Organ Failure Assessment (SOFA) scores (1) and prognosis, and recognized in most countries. Limited studies have been carried out in the elderly patients and identified potential risk factors for geriatric postoperative sepsis on previous definitions of sepsis, such as age, comorbidities, and dependent function (11, 12). However, such studies were limited by previous definitions of sepsis. Moreover, earlier studies used the univariate analysis or logistic regression analysis with a hypothesis of linear associations between the risk factors and sepsis. However, multiple known and suspected confounding risk factors (such as age and comorbidities) must be considered during the analysis and potentially non-linear associations or complicated relationships between the potential risk factors and sepsis would exaggerate or conceal their underlying effects.
Machine learning is a novel method to address the complicated and non-linear interactions and tree-based model analysis is widely recognized as it takes into account the predictive value of all factors sequentially in a hierarchy of importance (13). Moreover, tree-based model typically produces a simple and easily interpreted decision tree, which can also stratify the population according to some confounding factors. Nevertheless, application of the tree-based model to analyze risk factors for postoperative sepsis in elderly patients has not been validated so far. Thus, tree-based analysis was used in this study to address effect of the potential confounding factors and further explore the putative risk factors of geriatric sepsis based on the Sepsis-3 criteria, which enable early prediction and intervention to improve the outcomes of geriatric surgery patients.
This retrospective cohort study was approved by the Research Ethics Committee at the Third Affiliated Hospital of Sun Yat-sen University, Guangzhou, China (No. [2019]02-609-02). All the relevant data were retrieved from the electronic health record system, a database created by extracting medical records from the hospital information system, laboratory information system, picture archiving and communication system, and Docare Anesthesia System. Consecutive elderly patients (aged ≥ 65 yrs) (14) undergoing surgery between January 2015 and September 2020 were eligible for inclusion. Patients who received general or regional anesthesia without intubation, had incomplete anesthetic and postoperative data were excluded.
Based on previous literature and our clinical experience, we collected 17 putative risk factors for postoperative sepsis that were explored in other surgical population, including the preoperative laboratory variables [white blood cell counts (WBC), hematocrit (HCT), red blood cell distribution width (RDW), platelets (PLT), blood glucose (GLU), albumin (ALB), blood urea nitrogen (BUN), serum creatinine (SCr), hypersensitive C-reactive protein (hsCRP), hypersensitive C-reactive protein-to-albumin ratio (CAR), neutrophil-to-lymphocyte ratio (NLR)], and intraoperative variables (timing of surgery, types of surgery, duration of surgery, administration of dexmedetomidine, ulinastatin and steroids). Among them, preoperative laboratory variables were divided into groups according to clinical significance, normal reference of results in our hospital, or previous studies (see for details in Supplementary Table 1).
Meanwhile, we also enrolled 16 potential confounding factors that have been studied in the literature to confirm the association between putative risk factors and postoperative sepsis (11, 15–17), including demographic characteristics [age (65–74 or ≥ 75 years), sex, body mass index (< 18.5, 18.5–24 or ≥ 24 kg/m2), smoking and drinking], preoperative comorbidities [diabetes, coronary disease, cerebrovascular diseases, malignant tumor, pneumonia, hepatic failure, acute renal failure and numbers of preoperative comorbidities (0, 1–2 or ≥ 3)], and preoperative management related to acquired immunosuppression or immune dysregulation [chemical therapy, dialysis and infusion of red blood cells (RBC)].
In this current study, sepsis was defined as life-threatening organ dysfunction caused by a dysregulated host response to infection and the diagnosis of sepsis was a SOFA score increase of ≥ 2 based on the Sepsis-3 criteria in the presence of proven or suspected infection (1). Infections were confirmed by microbiological cultures (excluding samples that are contaminated or thought to indicate colonialization) were classified as suspected if antibiotics were administered in addition to those used as standard prophylaxis, or if the surgeons documented a suspected infection in the EPR's clinical notes. Daily SOFA scores were calculated after surgery until discharge. Patients were divided into sepsis group and non-sepsis group based on the sepsis criteria1.
As mentioned above, all continuous variables were divided into groups for the explainability in the clinical work (Table 1). Before grouping, we used means and modes to fill in missing values for continuous covariates and categorical covariates, respectively. All data were expressed as numbers (percentage) as all the continuous data were converted into categorical variables after grouping. Pearson's chi-square test was applied to compared categorical data.
To better find the effects of confounding factors on the incidence of postoperative sepsis, we applied a tree-based model (18, 19), also known as a classification and regression tree, to stratify the study population into relatively homogeneous subgroups based on a subset of the 16 confounding factors selected in the study. Tree-based model considers the divide and conquer method of decision tree induction. The algorithm identifies the most important independent variable and sets it as the root node, before forking to the next best variable. The algorithm works in a top-down fashion, from the root node to the internal nodes and finally to the terminal leaf nodes. In this study, we found the risk factors that best divided the sample into two groups at first. The best was defined as the partition that maximizes the sum of squares between groups (or, equivalently, minimizes the sum of squares of errors within groups). This process is applied recursively to each subgroup separately until the subgroup reaches the minimum sample (set to 20 in our analysis, the default value in part) or until the model fit cannot be improved. As the generated model is often too complex and may over-fit the data, the second stage of the process involves using cross-validation to prune the complete tree. We used stratified 10-fold cross-validation to assess model fit across a range of model complexity and select the optimal (pruned) tree by examining cross-validation errors in relation to model complexity. We followed the default parameter selection and finally chose the highest point of test scores in red to build the tree (see Supplementary Figure 1). In this study, three tree models were derived from different confounding factors. Model 1 based on demographic factors, model 2 based on demographic factors and preoperative comorbidities, and model 3 based on all the potential confounding factors were developed. Akaike information criterion (AIC) that focus on model accuracy and complexity was adopted for model selection.
To further control for confounders, we used the Mantel-Haenszel method (20, 21) to adjust for the effect of 17 putative risk factors on postoperative sepsis and derived adjusted relative risks (RRs). Similarly, we explored the marginal association between sepsis and 17 potential risk factors using a univariate logistic analysis. In addition, we explored the relationship between confounding factors and postoperative sepsis through following tree model screening.
For sensitivity analysis, logistic regression (LR) was performed and the adjusted odd ratios (ORs) were calculated to explore the association between the 17 potential risk factors and sepsis. Notably, the significant confounding factors were also taken into consideration.
All P-values were two sided, and P < 0.05 was considered as statistically significant. The construction and pruning of the tree model were done in Python 3.8, and the other statistical analyses were performed using R version 3.6.2 software (Vienna, Austria).
A total of 16,141 patients aged ≥ 65 yrs were collected, of whom 8,839 were excluded because they did not meet the inclusion criteria, leaving 7,302 to be enrolled in the final analysis (Figure 1). The mean age was 70 yrs, with approximately three quarters of patients falling into the 65–74 yrs group. The majority of patients were male (4,162, 57.0%). Three hundred and ninety-seven (5.4%) patients developed postoperative sepsis. The clinical characteristics of the patients were presented in Table 1, including 17 putative risk factors and 16 covariates.
The marginal association between sepsis and the 17 putative risk factors were shown in Table 2. We found that 15 of 17 variables were significantly associated with sepsis. Emergency surgery showed a significant marginal correlation as 25.19% of patients who underwent emergency surgery developed sepsis compared to 3.90% of patients who underwent elective surgery. The relative risk of sepsis in patients undergoing emergency surgery was 8.30 (95% CI 6.57–10.45) compared to the group undergoing elective surgery. Patients undergoing neurosurgery (RR 4.16, 95% CI 3.12–5.52) or transplantation (RR 6.33, 95% CI 3.64–10.65) had a higher risk of postoperative sepsis compared to those undergoing abdominal and genitourinary surgery; however, patients undergoing orthopedic surgery (RR 0.48, 95% CI 0.31–0.72) or head and neck surgery (RR 0.12, 95% CI 0.05–0.28) had a lower risk. Duration of surgery > 120 min (RR 2.96, 95% CI 2.33–3.80) and preoperative hypoalbuminemia (RR 3.12, 95% CI, 2.53–3.84) were associated with a higher risk of sepsis. Patients with elevated CAR or NLR were at higher risk of suffering postoperative sepsis (RR 3.46, 95% CI, 2.74–4.35 and RR 2.67, 95% CI 2.16–3.29, respectively). Meanwhile, intraoperative administration of steroids, ulinastatin, preoperative abnormal WBC, HCT, and PLT, elevated RDW, BUN, SCr, and GLU were also associated with postoperative sepsis.
The goodness of fit of the 3 tree models was ranked according to AIC, with the one having the lowest AIC being the best. Model 1 showed an AIC of 3056.8, model 2 showed an AIC of 2943.7, and model 3 showed the lowest AIC of 2907.4. Therefore, model 3 derived from all the potential confounders is the best tree model of postoperative sepsis in the elderly and detailed in Figure 2. Among the 16 potential covariates, six main confounding factors were statistically identified by the tree model, including cerebrovascular diseases, preoperative infusion of RBC, pneumonia, age ≥ 75 yrs, malignant tumor and diabetes. We used these six variables for classification and generated seven subgroups bases on the magnitude of risk. Each subgroup was designated by a probabilistic combination of potential confounders and the incidence of postoperative sepsis. Specifically, the total population was first divided into two groups based on the presence or absence of preoperative cerebrovascular diseases. Notably, the incidence of sepsis was 30.3% and 5.0% in patients with and without cerebrovascular diseases, respectively [RR 6.01, 95%CI (3.91–9.04), Figure 3]. The pattern of risk observed in each subgroup revealed interactions between a set of potential confounding variables. For example, we found that patients without cerebrovascular diseases, preoperative infusion of RBC or pneumonia, the risk of development of postoperative sepsis depended on the age. Patients aged ≥ 75 yrs had a higher risk of postoperative sepsis compared to patients aged 65–74 yrs [RR 1.92, 95% CI (1.49–2.45), Figure 3]. There was also an association between diabetes, malignant tumors and age, reflected in the fact that comorbid diabetes increases the risk of postoperative sepsis in patients aged 65–74 yrs with malignant tumors but not cerebrovascular diseases, preoperative transfusion of red blood cells or pneumonia. The model showed that among the 16 potential confounders identified, the presence of cerebrovascular disease was the most important risk factor for the incidence of sepsis. However, in patients without cerebrovascular disease, the risk was dependent on age and other preoperative conditions. It is worth mentioning that interactions between variables may be found in specific subgroup.
Besides, model 1 indicated that age ≥ 75 yrs and drinking were confounding factors for postoperative sepsis in the elderly patients if only demographic factors were taken into account. Model 2 showed that cerebrovascular diseases, pneumonia, age ≥ 75 yrs, and diabetes were confounding factors for postoperative sepsis if both demographic factors and comorbidities were considered (Supplementary Figure 2).
To further control for the six stratified confounders shown in model 3, we then applied the Mantel-Haenszel method in the stratified sample to derive adjusted relative risks for 17 hypothetical risk factors. We found that 14 of the factors had a significant effect. Patients undergoing emergency surgery had a higher risk of sepsis after surgery under general anesthesia (RR 6.66, 95% CI 5.13–8.64); We found that the risk of postoperative sepsis for neurosurgery (RR 3.85, 95% CI 2.73–5.42) and transplantation (RR 4.27, 95% CI 2.44–7.47) surgery were higher; however, the risk was lower for orthopedic surgery (RR 0.47, 95% CI 0.31–0.72) and head and neck surgery (RR 0.16, 95% CI 0.07–0.36) compared to abdominal and urogenital surgery. In addition, patients with hypoalbuminemia, duration of surgery > 120 min, abnormal WBC, and elevated NLR, CAR, creatinine also suffered a greater risk of postoperative sepsis (Table 2). Additionally, the relative risks estimated from the method of Mantel-Haenszel based on the model 1, model 2, and model 3 gave very close answers except PLT.
For sensitivity analysis, we also applied logistic regression to obtain the adjusted odds ratio of 17 hypothetical risk factors, as shown in the last two columns in Table 2. Notably, we found a first-order interaction between pneumonia and age (P = 0.0418), so we considered the first-order interactions between these two variables as well as the main effects of the other four confounding factors. After performing the positive and stepwise regression analysis among the 17 potential risk factors, we found the adjusted ORs were consistent with the adjusted RRs of the risk factors. Therefore, for this study, it seems unwarranted to adjust the risks for the 17 potential risk factors. However, this does not mean that it is not necessary to calculate the adjusted RR because we cannot draw this conclusion without them.
Early prediction and rapid treatment are crucial to improving outcomes of patients with postoperative sepsis (22), especially in the elderly patients that are more susceptible to sepsis and associated with higher mortality (23). Early studies have recognized many risk factors for sepsis, but few of them are carried out in elderly patients based on the Sepsis-3 Criteria and most of them assumed a linear relationship between risk factors and sepsis. This report has utilized a tree-based method to explore 17 assumed putative risk factors for geriatric postoperative sepsis by adjusting 16 potential confounding factors, considering the intricate interactions among variables. Accordingly, our study has demonstrated that several modifiable and unmodifiable risk factors are related to postoperative sepsis. Risk factors, such as emergency surgery (6.7 times), longer duration of surgery (2.9 times), Steroids (2.2 times), preoperative higher CAR (2.4 times), higher NLR (2.1 times) hypoalbuminemia (2.3 times) and elevated SCr (2.6 times) increased the risk of postoperative sepsis in the elderly patients significantly.
In the current study, three tree models were constructed for control of confounding factors for postoperative sepsis based on including different variables. In the tree-based analysis, the model 3 included all the covariates while the other models only included partial confounding factors. Model 3 was optimal, with the minimum AIC of 2907.4. As showed in Figure 3, the elderly patients with cerebrovascular diseases were at higher risk of developing sepsis than those without sepsis, which is previously reported in some studies based on Sepsis-1 criteria (24, 25). However, the role of cerebrovascular diseases in sepsis remains uncertain (26). Our study gives positive support to this argument. Specifically, with or without cerebrovascular diseases was chosen as the first splitter variable in our model, which suggests the strongest significance in risk for postoperative sepsis for the aged surgical patients. Transfusion may significantly increase risk of infection by down-regulating of immunologic functions or bacterial contamination of blood components (7, 27), and has been reported to be a risk factor for postoperative sepsis. Interestingly, our study showed that preoperative blood transfusion was a risk factor only for those without cerebrovascular diseases. Besides, age ≥ 75 yrs, pneumonia, diabetes and malignant tumor were also found to be associated with postoperative sepsis in elderly patients in the study.
Compared with the multivariable logistic regression analysis, non-parametric tree-based methods allow for the underlying complicated relationships between the risk factors. Tree-based methods also select the variables in order of importance and subgroup the population based on the risk. Since a large number of predictors are considered stratification factors/variables (particularly categorical ones), tree-based model is an appropriate alternative method, in view of laborious work in logistic regression model. In this study, we aim to address specific hypotheses by estimating adjusted odds ratio and relative risks for postoperative sepsis in the surgical elderly patients. Therefore, we do not conduct a pure tree-based model based on both the potential risk factors and confounding factors to find out the risk population. Instead, we used both logistic regression and the Mantel-Haenszel method after the tree-based analysis for assessment to examine the effects of risk factors (presented as RRs or ORs).
Our study also reported several strong factors associated with postoperative sepsis in the elderly surgical patients, including emergency surgery, duration of surgery, type of surgery and intraoperative administration of steroids, which are consistent with previous reported risk factors (15, 16, 28–32). Notably, we found that emergency surgery increased the risk of postoperative sepsis over 6-fold after regarding the confounders, and this was greater than other investigations that reported twice (29) or three-time risk (15) of emergency surgery contributing to postoperative sepsis in adults. The results revealed that in aged patients undergoing emergency surgery, more early intervention should be adopted purposefully to prevent postoperative sepsis.
Besides the emergency surgery, multiple preoperative laboratory results had been reported to predict sepsis but the causative interactions remain confusing (33). Meanwhile, most of risk factors of sepsis are not well defined and focus on the susceptibility to infection (34, 35). In the study, we applied the Mantel-Haenszel method in the stratified sample to derive adjusted relative risks and odds ratios for 17 hypothetical risk factors and found that preoperative abnormal WBC and HCT, elevated RDW, BUN, SCr and GLU were significantly associated with postoperative sepsis. All the variables were explainable according to our clinical experience. For instance, decreased renal function (elevated BUN and SCr) contributes to immunocompromised status through retention of uremic toxins and malnutrition, hence, increases development of infection and sepsis (36, 37). We also found that poor GLU control (preoperative GLU > 10 mmol/L) (38) was a risk factor of sepsis and this might be attributed to the effect of glycemia on altering the immune system through decrease of secretion of inflammatory cytokines, depression in neutrophils and T cells function, resulting in the risk for infection and delaying the recovery from infection (39). The results in our study also confirmed the association between sepsis and inflammatory biomarkers, including increased WBC, RDW (40), NLR (41, 42) and CAR (43). Notably, there exists little difference among the adjusted RRs obtained by Mantel-Haenszel method based on different tree models and adjusted ORs obtained by logistic regression, indicating that our results were stable and reliable to predict postoperative sepsis in the elderly.
Consistent with earlier study (44), our results showed hypoalbuminemia an indicator of malnutrition and inflammation that increases the risk for infection and sepsis, but no significant correlation between increased hsCRP and postoperative sepsis was found. Instead, elevated CAR was shown to indicate higher risk of sepsis, compared with hypoalbuminemia and the other inflammatory biomarkers. As we earlier reported, we think this might be attributed to the low specificity of hsCRP and the CAR is thought to integrate information provided by hsCRP and albumin, and higher CAR is often associated with nutritional deficiency and severe inflammation, which makes it superior to other systemic inflammation biomarkers.
In this study, we revealed several factors that need to be addressed and modified to decrease the risk for developing postoperative sepsis. For examples, decrease of unnecessary blood transfusion, correcting hypoalbuminemia, well control of preoperative blood glucose and infection status, and shortening the operation time may decrease the risk of postoperative sepsis. Although we found the intraoperative administration of ulinastatin was associated with postoperative sepsis, which is inconsistent with its anti-inflammatory effect (45), we think this might be attributed to the fact that patients received intra-operative ulinastatin might have worse perioperative conditions and received a higher grade of surgery.
Our study may have several limitations. Firstly, the risk factor analysis was developed in a single-center study, and future multi-center study will be needed for generalization and validation. Secondly, the study was performed retrospectively, for which possible residual confounding may occur and we couldn't explore the exact effect of ulinastatin administration on patients with the same condition. Thirdly, analysis was restricted to the variables available from the electronic health record system; hence, some potential factors such as bacterial species and infection site cannot be evaluated. Finally, the cut-off points of the preoperative laboratory test were grouped mostly according to the normal ranges in our hospital instead of the optimal cut-off point. We consider the little variation of results caused technique for testing in different hospitals and attention will be attracted by abnormal results.
In conclusion, we developed a tree-based analysis for postoperative sepsis defined by the newly introduced Sepsis-3 criteria in elderly patients, taking non-linear interactions into consideration, and revealed many risk factors for postoperative sepsis among the surgical aged patients, including preoperative clinical laboratory results and surgery-related factors. It provides evidence for clinical decision-making by modification of some preoperative risk factors and early identification of high-risk surgical patients after surgery.
The raw data supporting the conclusions of this article will be made available by the authors, without undue reservation.
The studies involving human participants were reviewed and approved by the Ethnic Committee of The Third Affiliated Hospital of Sun Yat-sen University (No. [2019]02-609-02). Written informed consent for participation was not required for this study in accordance with the national legislation and the institutional requirements.
ZL, SZ, and ZH designed this research. XP and CC collected and anonymized the original data. XP, CC, and JC designed and guided the process of data cleaning, model building, and statistics. CC, DY, CM, and YW defined the rules of data extraction and interpreted patient data. ZL and JC performed data cleaning and built the tree-based model. XP and CC equally contributed in writing the manuscript. DY, CC, and JC revised the manuscript. All authors read and approved the final manuscript.
This study was supported by the National Natural Science Foundation of China (Grant Nos. 82102297, 82072218, and 81974296), and Natural Science Foundation of Guangdong Province (Grant Nos. 2018A0303130224, 2019A1515110020, 2020A1515010153, and 2022A1515012603), Science and Technology Program of Guangzhou, China (Grant No. 202002020047), Young Talent Support Project of Guangzhou Association for Science and Technology (Grant No. QT20220101257), and the Fundamental Research Funds for the Central Universities of China (Grant No. 22qntd3401).
The authors declare that the research was conducted in the absence of any commercial or financial relationships that could be construed as a potential conflict of interest.
All claims expressed in this article are solely those of the authors and do not necessarily represent those of their affiliated organizations, or those of the publisher, the editors and the reviewers. Any product that may be evaluated in this article, or claim that may be made by its manufacturer, is not guaranteed or endorsed by the publisher.
The Supplementary Material for this article can be found online at: https://www.frontiersin.org/articles/10.3389/fpubh.2022.1006955/full#supplementary-material
1. ^For Original Research articles, please note that the Material and Methods section can be placed in any of the following ways: before Results, before Discussion or after Discussion.
1. Singer M, Deutschman CS, Seymour CW, Shankar-Hari M, Annane D, Bauer M, et al. The third international consensus definitions for sepsis and septic shock (Sepsis-3). JAMA. (2016) 315:801–10. doi: 10.1001/jama.2016.0287
2. Angus DC, Linde-Zwirble WT, Lidicker J, Clermont G, Carcillo J, Pinsky MR. Epidemiology of severe sepsis in the United States: analysis of incidence, outcome, and associated costs of care. Crit Care Med. (2001) 29:1303–10. doi: 10.1097/00003246-200107000-00002
3. Bateman BT, Schmidt U, Berman MF, Bittner EA. Temporal trends in the epidemiology of severe postoperative sepsis after elective surgery: a large, nationwide sample. Anesthesiology. (2010) 112:917–25. doi: 10.1097/ALN.0b013e3181cea3d0
4. Rhee C, Dantes R, Epstein L, Murphy DJ, Seymour CW, Iwashyna TJ, et al. Incidence and trends of sepsis in US hospitals using clinical vs claims data, 2009–2014. JAMA. (2017) 318:1241–9. doi: 10.1001/jama.2017.13836
5. Vogel TR, Dombrovskiy VY, Carson JL, Graham AM, Lowry SF. Postoperative sepsis in the United States. Ann Surg. (2010) 252:1065–71. doi: 10.1097/SLA.0b013e3181dcf36e
6. Brakenridge SC, Efron PA, Cox MC, Stortz JA, Hawkins RB, Ghita G, et al. Current epidemiology of surgical sepsis: discordance between inpatient mortality and 1-year outcomes. Ann Surg. (2019) 270:502–10. doi: 10.1097/SLA.0000000000003458
7. Mankowski RT, Anton SD, Ghita GL, Brumback B, Cox MC, Mohr AM, et al. Older sepsis survivors suffer persistent disability burden and poor long-term survival. J Am Geriatr Soc. (2020) 68:1962–9. doi: 10.1111/jgs.16435
8. Iwashyna TJ, Cooke CR, Wunsch H, Kahn JM. Population burden of long-term survivorship after severe sepsis in older Americans. J Am Geriatr Soc. (2012) 60:1070–7. doi: 10.1111/j.1532-5415.2012.03989.x
9. Castellanos-Ortega A, Suberviola B, García-Astudillo LA, Holanda MS, Ortiz F, Llorca J, et al. Impact of the surviving sepsis campaign protocols on hospital length of stay and mortality in septic shock patients: results of a three-year follow-up quasi-experimental study. Crit Care Med. (2010) 38:1036–43. doi: 10.1097/CCM.0b013e3181d455b6
10. Kaukonen KM, Bailey M, Pilcher D, Cooper DJ, Bellomo R. Systemic inflammatory response syndrome criteria in defining severe sepsis. N Engl J Med. (2015) 372:1629–38. doi: 10.1056/NEJMoa1415236
11. Bouza C, López-Cuadrado T, Amate-Blanco JM. Characteristics, incidence and temporal trends of sepsis in elderly patients undergoing surgery. Br J Surg. (2016) 103:e73–82. doi: 10.1002/bjs.10065
12. Bohl DD, Iantorno SE, Saltzman BM, Tetreault MW, Darrith B, Della Valle CJ. Sepsis within 30 days of geriatric hip fracture surgery. J Arthroplasty. (2017) 32:3114–9. doi: 10.1016/j.arth.2017.05.024
13. Loh W-Y. Classification and regression trees. WIREs Data Mining Knowl Discov. (2011) 1:14–23. doi: 10.1002/widm.8
14. Orimo H. [Reviewing the definition of elderly]. Nihon Ronen Igakkai Zasshi. (2006) 43:27–34. doi: 10.3143/geriatrics.43.27
15. Plaeke P, De Man JG, Coenen S, Jorens PG, De Winter BY, Hubens G. Clinical- and surgery-specific risk factors for post-operative sepsis: a systematic review and meta-analysis of over 30 million patients. Surg Today. (2020) 50:427–39. doi: 10.1007/s00595-019-01827-4
16. Zuckerman SL, Lakomkin N, Smith JS, Shaffrey CI, Devin CJ. Incidence and predictors of all-cause mortality within one year after adult spinal deformity surgery. J Spine Surg. (2018) 4:333–41. doi: 10.21037/jss.2018.05.28
17. Wang Y, Gu Y, Huang F, Liu D, Zhang Z, Zhou N, et al. Risk factors for sepsis based on sepsis-3 criteria after orthotopic liver transplantation. Mediators Inflamm. (2018) 2018:8703172. doi: 10.1155/2018/8703172
18. Xiang S, Li L, Wang L, Liu J, Tan Y, Hu J, et al. A decision tree model of cerebral palsy based on risk factors. J Matern Fetal Neonatal Med. (2021) 34:3922–7. doi: 10.1080/14767058.2019.1702944
19. Duan L, He J, Li M, Dai J, Zhou Y, Lai F, et al. Based on a decision tree model for exploring the risk factors of smartphone addiction among children and adolescents in china during the COVID-19 pandemic. Front Psychiatry. (2021) 12:652356. doi: 10.3389/fpsyt.2021.652356
20. Mangat HS, Chiu YL, Gerber LM, Alimi M, Ghajar J, Härtl R. Hypertonic saline reduces cumulative and daily intracranial pressure burdens after severe traumatic brain injury. J Neurosurg. (2015) 122:202–10. doi: 10.3171/2014.10.JNS132545
21. Bruno E, Nicoletti A, Quattrocchi G, Filippini G, Colosimo C, Zappia M. Pregabalin for essential tremor. Cochrane Database Syst Rev. (2016) 10:Cd009682. doi: 10.1002/14651858.CD009682.pub2
22. Rhodes A, Evans LE, Alhazzani W, Levy MM, Antonelli M, Ferrer R, et al. Surviving sepsis campaign: international guidelines for management of sepsis and septic shock: 2016. Intensive Care Med. (2017) 43:304–77. doi: 10.1007/s00134-017-4683-6
23. Rudd KE, Johnson SC, Agesa KM, Shackelford KA, Tsoi D, Kievlan DR, et al. Global, regional, and national sepsis incidence and mortality, 1990–2017: analysis for the Global Burden of Disease Study. Lancet. (2020) 395:200–11. doi: 10.1016/S0140-6736(19)32989-7
24. Sood A, Abdollah F, Sammon JD, Arora N, Weeks M, Peabody JO, et al. Postoperative sepsis prediction in patients undergoing major cancer surgery. J Surg Res. (2017) 209:60–9. doi: 10.1016/j.jss.2016.09.059
25. Kim M, Brady JE Li G. Interaction effects of acute kidney injury, acute respiratory failure, and sepsis on 30-day postoperative mortality in patients undergoing high-risk intraabdominal general surgical procedures. Anesth Analg. (2015) 121:1536–46. doi: 10.1213/ANE.0000000000000915
26. Gabriel V, Grigorian A, Nahmias J, Pejcinovska M, Smith M, Sun B, et al. Risk factors for post-operative sepsis and septic shock in patients undergoing emergency surgery. Surg Infect. (2019) 20:367–72. doi: 10.1089/sur.2018.186
27. Remy KE, Hall MW, Cholette J, Juffermans NP, Nicol K, Doctor A, et al. Mechanisms of red blood cell transfusion-related immunomodulation. Transfusion. (2018) 58:804–15. doi: 10.1111/trf.14488
28. Upile T, Jerjes W, Mahil J, Upile N, Sudhoff H, Wright A, et al. An explanation for the worsened prognosis in some cancer patients of perioperative transfusion: the time-dependent release of biologically active growth factors from stored blood products. Eur Arch Otorhinolaryngol. (2011) 268:1789–94. doi: 10.1007/s00405-011-1525-y
29. Moore LJ, Moore FA, Todd SR, Jones SL, Turner KL, Bass BL. Sepsis in general surgery: the 2005-2007 national surgical quality improvement program perspective. Arch Surg. (2010) 145:695–700. doi: 10.1001/archsurg.2010.107
30. Ninh A, Wood K, Bui AH, Leitman IM. Risk factors and outcomes for sepsis after appendectomy in adults. Surg Infect. (2019) 20:601–6. doi: 10.1089/sur.2019.003
31. Howitt SH, Herring M, Malagon I, McCollum CN, Grant SW. Incidence and outcomes of sepsis after cardiac surgery as defined by the Sepsis-3 guidelines. Br J Anaesth. (2018) 120:509–16. doi: 10.1016/j.bja.2017.10.018
32. Shankar-Hari M, Harrison DA, Rubenfeld GD, Rowan K. Epidemiology of sepsis and septic shock in critical care units: comparison between sepsis-2 and sepsis-3 populations using a national critical care database. Br J Anaesth. (2017) 119:626–36. doi: 10.1093/bja/aex234
33. Fleuren LM, Klausch TLT, Zwager CL, Schoonmade LJ, Guo T, Roggeveen LF, et al. Machine learning for the prediction of sepsis: a systematic review and meta-analysis of diagnostic test accuracy. Intensive Care Med. (2020) 46:383–400. doi: 10.1007/s00134-019-05872-y
34. Cecconi M, Evans L, Levy M, Rhodes A. Sepsis and septic shock. Lancet. (2018) 392:75–87. doi: 10.1016/S0140-6736(18)30696-2
35. Rowe TA, McKoy JM. Sepsis in older adults. Infect Dis Clin North Am. (2017) 31:731–42. doi: 10.1016/j.idc.2017.07.010
36. Glenn DA, Henderson CD, O'Shaughnessy M, Hu Y, Bomback A, Gibson K, et al. Infection-related acute care events among patients with glomerular disease. Clin J Am Soc Nephrol. (2020) 15:1749–61. doi: 10.2215/CJN.05900420
37. Cohen AJ, Packiam VT, Nottingham CU, Pariser JJ, Faris SF, Bales GT. Iatrogenic bladder injury: national analysis of 30-day outcomes. Urology. (2016) 97:250–6. doi: 10.1016/j.urology.2016.05.002
38. Duggan EW, Carlson K, Umpierrez GE. Perioperative hyperglycemia management: an update. Anesthesiology. (2017) 126:547–60. doi: 10.1097/ALN.0000000000001515
39. Gupta S, Koirala J, Khardori R, Khardori N. Infections in diabetes mellitus and hyperglycemia. Infect Dis Clin North Am. (2007) 21:617–38. doi: 10.1016/j.idc.2007.07.003
40. Hu ZD, Lippi G, Montagnana M. Diagnostic and prognostic value of red blood cell distribution width in sepsis: a narrative review. Clin Biochem. (2020) 77:1–6. doi: 10.1016/j.clinbiochem.2020.01.001
41. Ljungström L, Pernestig AK, Jacobsson G, Andersson R, Usener B, Tilevik D. Diagnostic accuracy of procalcitonin, neutrophil-lymphocyte count ratio, C-reactive protein, and lactate in patients with suspected bacterial sepsis. PLoS ONE. (2017) 12:e0181704. doi: 10.1371/journal.pone.0181704
42. Huang Z, Fu Z, Huang W, Huang K. Prognostic value of neutrophil-to-lymphocyte ratio in sepsis: a meta-analysis. Am J Emerg Med. (2020) 38:641–7. doi: 10.1016/j.ajem.2019.10.023
43. Chen C, Chen X, Chen J, Xing J, Hei Z, Zhang Q, et al. Association between preoperative hs-crp/albumin ratio and postoperative sirs in elderly patients: a retrospective observational cohort study. J Nutr Health Aging. (2022) 26:352–9. doi: 10.1007/s12603-022-1761-4
44. Eckart A, Struja T, Kutz A, Baumgartner A, Baumgartner T, Zurfluh S, et al. Relationship of nutritional status, inflammation, and serum albumin levels during acute illness: a prospective study. Am J Med. (2020) 133:713–22.e7. doi: 10.1016/j.amjmed.2019.10.031
Keywords: sepsis, risk factors, elderly, tree-based analysis, classification and regression tree
Citation: Peng X, Chen C, Chen J, Wang Y, Yang D, Ma C, Liu Z, Zhou S and Hei Z (2022) Tree-based, two-stage risk factor analysis for postoperative sepsis based on Sepsis-3 criteria in elderly patients: A retrospective cohort study. Front. Public Health 10:1006955. doi: 10.3389/fpubh.2022.1006955
Received: 29 July 2022; Accepted: 07 September 2022;
Published: 26 September 2022.
Edited by:
Jiayuan Wu, Affiliated Hospital of Guangdong Medical University, ChinaReviewed by:
Venkatesh Avula, Geisinger Health System, United StatesCopyright © 2022 Peng, Chen, Chen, Wang, Yang, Ma, Liu, Zhou and Hei. This is an open-access article distributed under the terms of the Creative Commons Attribution License (CC BY). The use, distribution or reproduction in other forums is permitted, provided the original author(s) and the copyright owner(s) are credited and that the original publication in this journal is cited, in accordance with accepted academic practice. No use, distribution or reproduction is permitted which does not comply with these terms.
*Correspondence: Ziqing Hei, aGVpemlxaW5nQHNpbmEuY29t; Shaoli Zhou, MTM2MTAyNzIzMDhAMTYzLmNvbQ==; Zifeng Liu, bGl1emZAbWFpbC5zeXN1LmVkdS5jbg==
†These authors have contributed equally to this work
Disclaimer: All claims expressed in this article are solely those of the authors and do not necessarily represent those of their affiliated organizations, or those of the publisher, the editors and the reviewers. Any product that may be evaluated in this article or claim that may be made by its manufacturer is not guaranteed or endorsed by the publisher.
Research integrity at Frontiers
Learn more about the work of our research integrity team to safeguard the quality of each article we publish.