- 1Department of Oncology, The Second Affiliated Hospital of Xi'an Jiaotong University, Xi'an, China
- 2Department of Radiation Oncology, The Second Affiliated Hospital of Xi'an Jiaotong University, Xi'an, China
- 3Department of Otolaryngology, The Second Affiliated Hospital of Xi'an Jiaotong University, Xi'an, China
Background: Bone is the most common metastatic site of patients with advanced breast cancer and the survival time is their primary concern; however, we lack accurate predictive models in clinical practice. In addition to this, primary surgery for breast cancer patients with bone metastases is still controversial.
Method: The data used for analysis in this study were obtained from the SEER database (2010–2019). We made a COX regression analysis to identify prognostic factors of patients with bone metastatic breast cancer (BMBC). Through cross-validation, we constructed an XGBoost model to predicting survival in patients with BMBC. We also investigated the prognosis of patients treated with neoadjuvant chemotherapy plus surgical and chemotherapy alone using propensity score matching and K–M survival analysis.
Results: Our validation results showed that the model has high sensitivity, specificity, and correctness, and it is the most accurate one to predict the survival of patients with BMBC (1-year AUC = 0.818, 3-year AUC = 0.798, and 5-year survival AUC = 0.791). The sensitivity of the 1-year model was higher (0.79), while the specificity of the 5-year model was higher (0.86). Interestingly, we found that if the time from diagnosis to therapy was ≥1 month, patients with BMBC had even better survival than those who started treatment immediately (HR = 0.920, 95%CI 0.869–0.974, P < 0.01). The BMBC patients with an income of more than USD$70,000 had better OS (HR = 0.814, 95%CI 0.745–0.890, P < 0.001) and BCSS (HR = 0.808 95%CI 0.735–0.889, P < 0.001) than who with income of < USD$50,000. We also found that compared with chemotherapy alone, neoadjuvant chemotherapy plus surgical treatment significantly improved OS and BCSS in all molecular subtypes of patients with BMBC, while only the patients with bone metastases only, bone and liver metastases, bone and lung metastases could benefit from neoadjuvant chemotherapy plus surgical treatment.
Conclusion: We constructed an AI model to provide a quantitative method to predict the survival of patients with BMBC, and our validation results indicate that this model should be highly reproducible in a similar patient population. We also identified potential prognostic factors for patients with BMBC and suggested that primary surgery followed by neoadjuvant chemotherapy might increase survival in a selected subgroup of patients.
Introduction
Breast cancer (BC) now is the first most diagnosed cancer (11.7% of the new cancer cases) worldwide, accounts for a quarter of all female cancer cases and BC is also the leading cause of cancer death among female patients (1). With significant treatment advances, the survival of patients with BC was improved dramatically. However, distant metastases remain the leading cause of death in patients with BC (2) and a major challenge for clinicians.
Overall, the average proportion of all breast cancer patients with an initial diagnosis of bone metastases is about 5% (3), and bone metastases usually lead to skeletal-related events, namely, pain, pathological fractures, spinal cord compression, hypercalcemia, and other complications (4). Current treatments for bone metastases are limited and merely palliative; standard anti-osteoporotic agents, chemotherapy, and radiotherapy can delay or lessen skeletal-related events, but they cannot cure bone metastases (5), the 5-year overall survival rate is only 22.8% (6). Thus, the survival time is the most important concern for patients with bone metastatic breast cancer (BMBC) and clinicians. However, there is no accurate prediction model for them. The most used model for predicting the survival rate is nomogram, but its accuracy rate is only about 70% (7–12). As a result, a more accurate and powerful model is needed.
Nowadays, machine learning methods can create an artificial intelligence (AI) model to predict the survival of patients with cancer, which significantly increases the accuracy rate (13). However, machine learning algorithms also have drawbacks and need to be improved in practice. For example, a support-vector machine (SVM) is not good at handling large numbers of samples and variables, K-nearest neighbor (KNN) is not very interpretable, and decision trees are easy to train quickly, but not complex enough (14, 15). Whereas extreme gradient boosting (XGBoost) is created iteratively to minimize the loss function, which makes it perform well in many areas (16–18). But it is rarely applied in clinical patient prognosis prediction. Through inter-model comparison, we found that XGBoost also performed well on such prognostic problems.
This study examined the prognosis of patients with BMBC from the Surveillance Epidemiology and End Results (SEER) database. And we created a high-precision AI model to predict the 1-, 3-, and 5-year survival of patients with BMBC. This work provides insight into the factors that influence the prognosis of patients with BMBC and contributes to the development of a clinical model to improve the long-term follow-up of patients with BMBC.
Materials and methods
Data source and study design
The workflow of our study design and analyses is shown in Figure 1. As the information on distant metastases was included from 2010, the data used for analysis in this study were obtained from the Surveillance, Epidemiology, and End Results (SEER) database [SEER 17 Regs study data (changes 2010–2019); version 8.4.0]. Because the data are publicly available and do not include personally identifiable patient information, this retrospective cohort study was approved by the Institutional Review Board of the Second Affiliated Hospital of Xi'an Jiaotong University, which decided to waive informed consent. From this database, data were collected on women with BC. Inclusion criteria were as follows: (1) BC was only cancer diagnosed in the patient; (2) all patients with cancer had evidence of the International Classification of Cancer Diseases Edition III (ICD-O-3) morphological and histopathology diagnosis; (3) patient had bone metastases at initial diagnosis. Exclusion criteria was that patients were diagnosed with more than one primary cancer. In this study, patients were followed up until death, loss to follow-up or December 31, 2019.
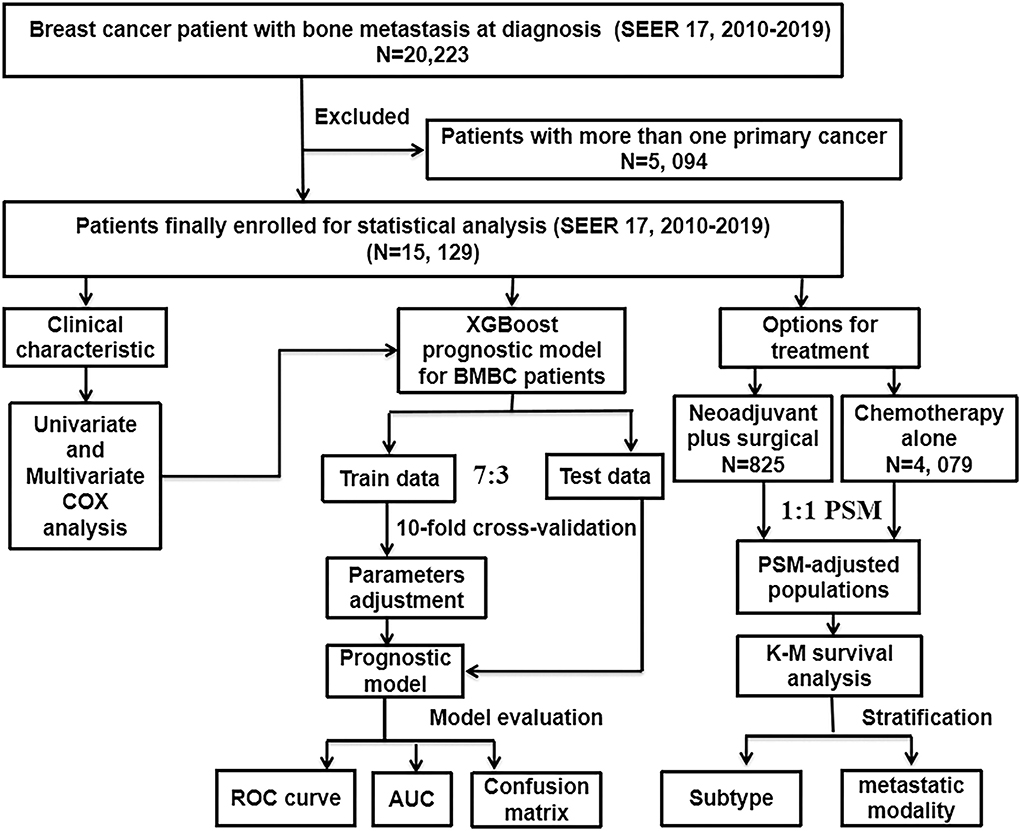
Figure 1. The flowchart described the process of conducting the study and statistical analysis. SEER, the Surveillance, Epidemiology, and End Results database; BMBC, bone metastatic breast cancer; PSM, propensity score matching; COX, concordance index; ROC curve, receiver operating characteristic curve; AUC, area under the curve; K–M, Kaplan–Meier; XGBoost, extreme gradient boosting.
Statistical analysis
We did univariate COX regression models to analyze the relationship between various clinical and pathological characteristics and patient survival. Further multifactorial COX analysis was done to compare the risk of death of patients and to identify independent factors of prognosis. To investigate the impact of neoadjuvant chemotherapy plus surgical treatment on the prognosis of patients with BMBC, patients receiving neoadjuvant chemotherapy plus surgical and chemotherapy alone, respectively, were matched on a 1:1 propensity score (PSM) based on statistically significant variables in multifactorial COX analysis. A Kaplan–Meier (K–M) survival analysis stratified by metastatic modality and molecular subtype was also performed on the PSM-adjusted population. All statistical analyses were performed using R software (version 4.0.2). A bilateral tail value of < 0.05 was considered statistically significant.
XGBoost model
XGBoost is a modification of the gradient boosting algorithm, using Newton's method when solving for the extreme values of the loss function, Taylor expansion of the loss function to the second order, and additionally a regularization term is added to the loss function. The objective function at training time consists of two parts, the first part being the gradient boosting algorithm loss and the second part being the regularization term. The principle of the XGBoost algorithm can be summarized as follows: feature vector with the corresponding (output) category yi:
Feature selection: univariate and multivariate COX analyses were performed on the clinical characteristics extracted from the SEER database, and statistically significant characteristics, namely, age at diagnosis, race, marital status, months from diagnosis to therapy, T stage, N stage, grade, breast subtype, median household income, distant sites of metastases, and treatment information were incorporated into the machine learning model to predict 1-, 3-, and 5-year overall survival for patients with BMBC. These analyses were performed before the exclusion of patients who were alive but survived less than 1, 3, or 5 years at the follow-up cut-off date. Before running the training program, a response variable was obtained for survival information, in which 1 = survival and 0 = death. Patients were randomly divided into train data and test data according to 7:3. And we compared the performance of SVM, decision tree (ID3), K-Nearest Neighbor (KNN), and extreme gradient boosting (XGBoost) on test data. Receiver operating characteristic (ROC) analysis, area under the ROC curve (AUC), and confusion matrix were used for the evaluation of the model. The main evaluation indicators in the confusion matrix include sensitivity, specificity, and correctness.
Results
Clinical characteristics of patients with BMBC
Ultimately, we extracted the information of 15,129 eligible patients with BMBC in the SEER database from 2010 to 2019. The clinic-pathological characteristics of BC patients with bone metastases are shown in Table 1 and summarized as follows. The mean age of patients was 61.39 years, with 1,113 (7.36%) patients younger than 40 and 1,752 (11.58%) patients older than 80. Five thousand six hundred nine (37.07%) patients received treatment immediately after diagnosis, while 7,271 (48.06%) patients received treatment more than 1 month after diagnosis. The molecular subtype of HR+/HER2– accounted for 57.19%, followed by HR+/HER2+ (14.22%) and HR–/HER2– (7.86%), and HR–/HER2+ only accounted for 6.05%. In terms of race, 76.70% of the patients were white. The most common histological type was infiltrating ductal carcinoma (IDC) (63.68%). In terms of marital status, 42.44% of the patients were married and 23.46% were single. The staging T1–T4 was 11.78, 28.72, 14.93, and 29.49%; and the percentages of N0–N3 were 21.13, 43.75, 9.72 and 20.26%, respectively. About 30.98% of the patients had a grade III or IV tumor grade pathology, while only 6.70% had a grade I. About 38.35% of the patients had a good family financial situation with an annual income of more than US$70,000. In terms of treatment, 20.37% of patients received surgical treatment, 34.15% received radiotherapy, 53.06% received chemotherapy, and 7% received neoadjuvant chemotherapy. Brain metastases, liver metastases, lung metastases, distant lymph nodes, and other distant organs metastases accounted for 7.31, 24.39, 26.75, 9.83, and 6.46% of patients, respectively.
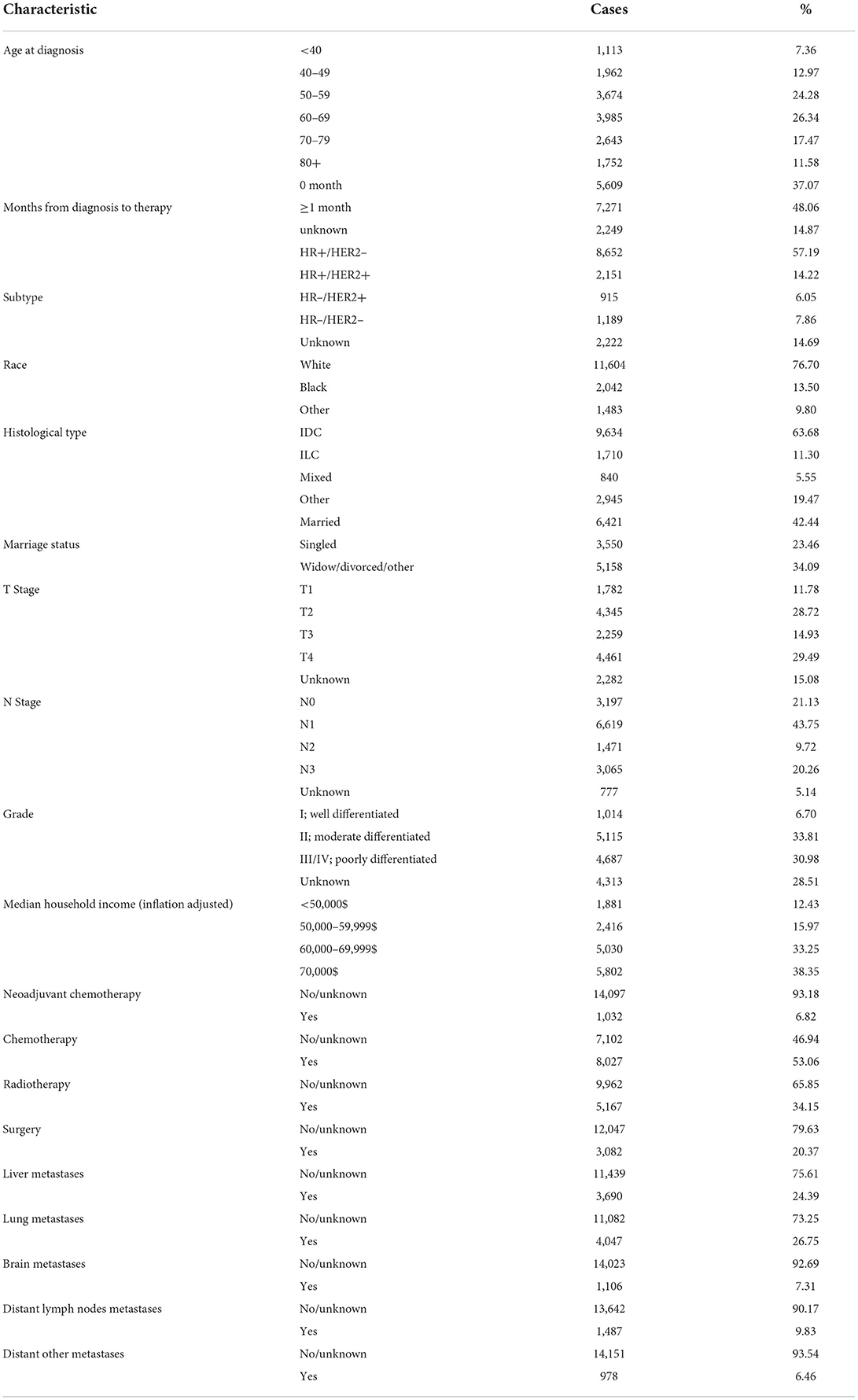
Table 1. Baseline characteristics of patients with bone metastatic breast cancer (BMBC) included from SEER data cohort.
Univariable and multivariable COX regression analyses
We performed univariate COX regression to identify significant variables affecting overall survival (OS) and breast cancer-specific survival (BCSS) in patients with BMBC, namely, age at diagnosis, months from diagnosis to therapy, molecular subtype, race, histological type, marital status, T stage, N stage, grade, household income (inflation-adjusted), treatment, and distant metastases information (Table 2).
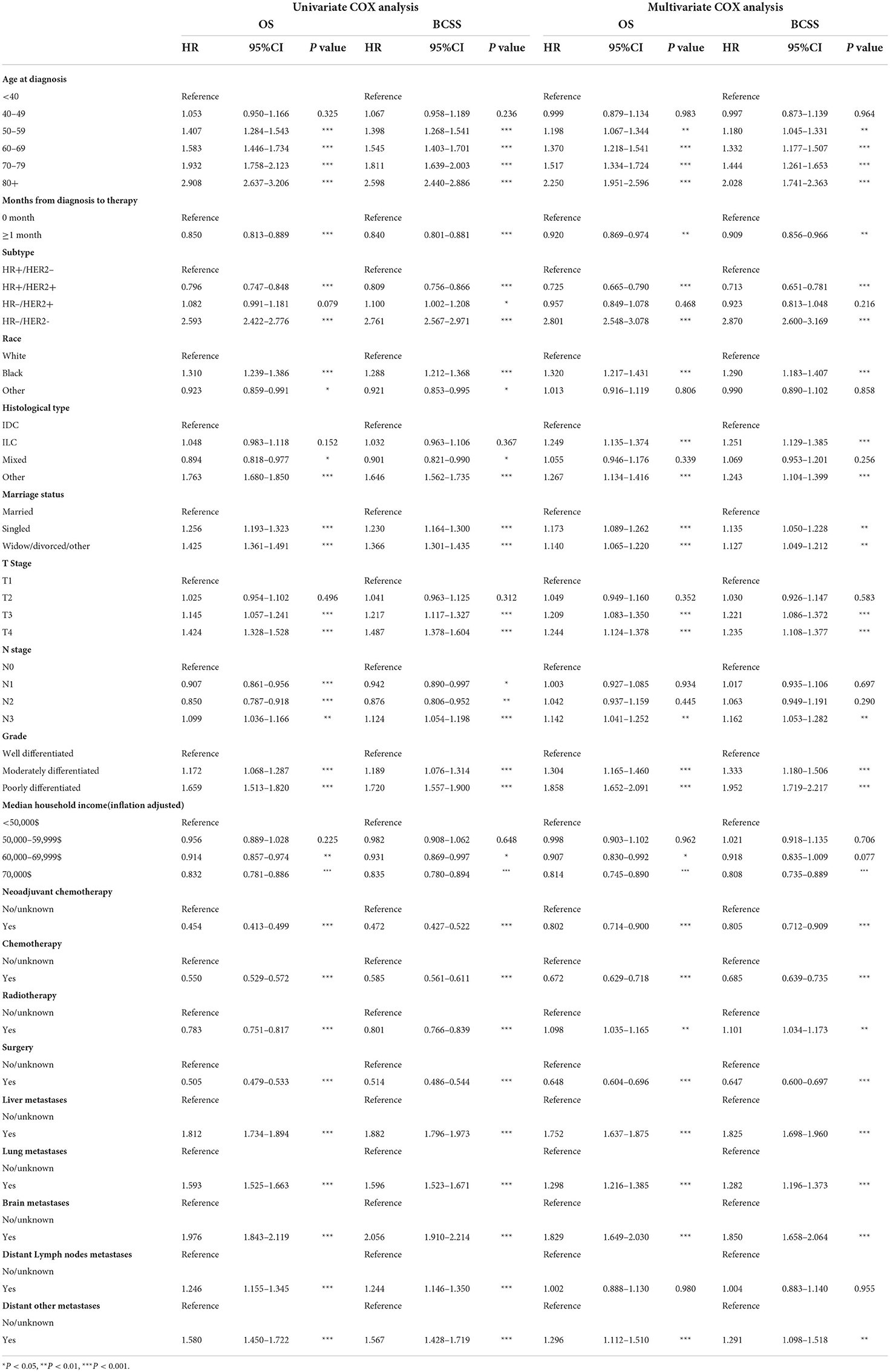
Table 2. Univariate and multivariate COX analyses of characteristics extracted from the SEER database.
To identify independent variables associated with OS and BCSS, we then conducted multivariable COX regression (Table 2). We found that patients older than 50 years old, < 1 month from diagnosis to therapy, black race, ILC, T Stage>T3, Stage N3, moderately or high Grade, and visceral metastases (brain, liver, lung, or other) were significantly related to worse OS and BCSS. Compared with patients with HR+/HER2–, the HR+/HER2+ subtype revealed improved OS and BCSS, while HR–/HER2– subtype showed the worst outcome, and there was no difference between HR+/HER2– and HR–/HER2+. For treatment, primary tumor surgery, chemotherapy, and neoadjuvant chemotherapy could prolong OS and BCSS; however, radiotherapy showed the opposite effect in multivariable COX regression analysis. Some social factors like marital status and income situation were also associated with survival, married status, and annual household income of more than USD$70,000 were significantly related to better survival.
Benefits of neoadjuvant chemotherapy plus surgical treatment in BMBC patients subdivided by molecular subtypes and metastatic sites
Neoadjuvant chemotherapy data were just opened by SEER; thus, we explored the role of this factor in the prognosis of patients with BMBC. We compared baseline characteristics between neoadjuvant chemotherapy plus surgical treatment and chemotherapy alone groups (Table 3). Patients in the neoadjuvant chemotherapy plus surgical group were younger, later T, N stages, and worse pathology grade, more likely to be married, hormone receptor negative and received surgery, and radiotherapy and chemotherapy. In addition, the neoadjuvant chemotherapy plus surgical group also included fewer liver, lung, brain and other distant metastases. Propensity score matching (PSM) was used to adjust for the observed imbalance. And no significant differences were seen in baseline characteristics after PSM adjustment (Table 3).
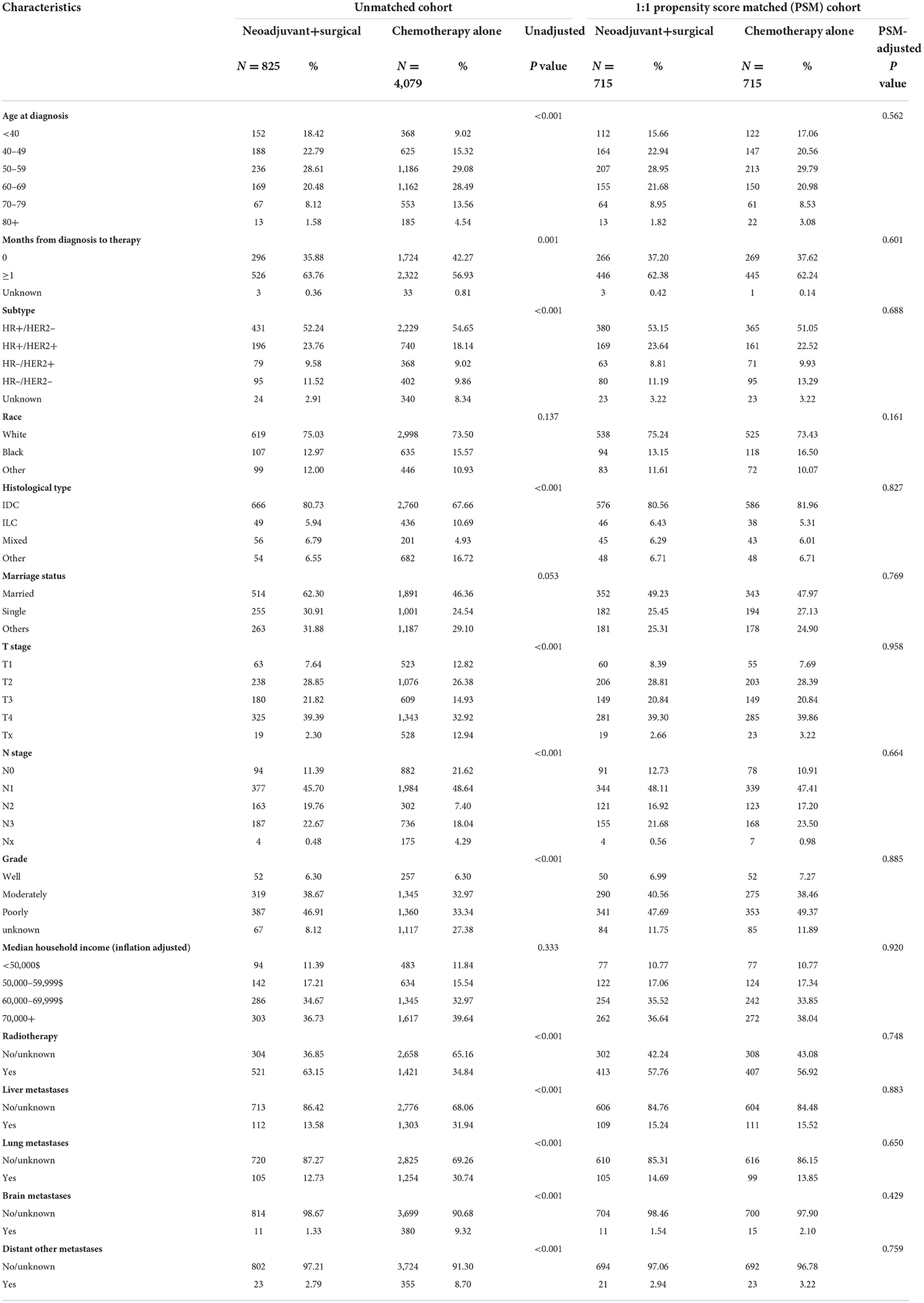
Table 3. Comparison of patient characteristics according to the use of neoadjuvant chemotherapy before and after propensity score matching (PSM).
The PSM-adjusted data showed about a 50% reduction in the overall risk of death in the neoadjuvant chemotherapy plus surgical group (p < 0.001, HR: 0.50; 95% CI: 0.43–0.59), which were similar to the results of multifactorial COX and allayed our concerns about selection bias in the PSM process (Figures 2A,B). Stratified K–M survival analysis showed that compared with chemotherapy alone, neoadjuvant chemotherapy plus surgical treatment significantly improved OS and BCSS in all molecular subtypes of patients with BMBC (Figures 3A–H; Supplementary Table 1). In addition to this, neoadjuvant chemotherapy plus surgical treatment significantly improved the OS and BCSS of patients with BMBC suffering from bone metastases only (Figures 4A,F), bone and liver metastases (Figures 4B,G), and bone and lung metastases (Figures 4C,H). In contrast, there was no significant difference in OS and BCSS of patients with BMBC who suffered from both liver and lung metastases (Figures 4D,I) or combined with brain metastases (Figures 4E,J; Supplementary Table 1).
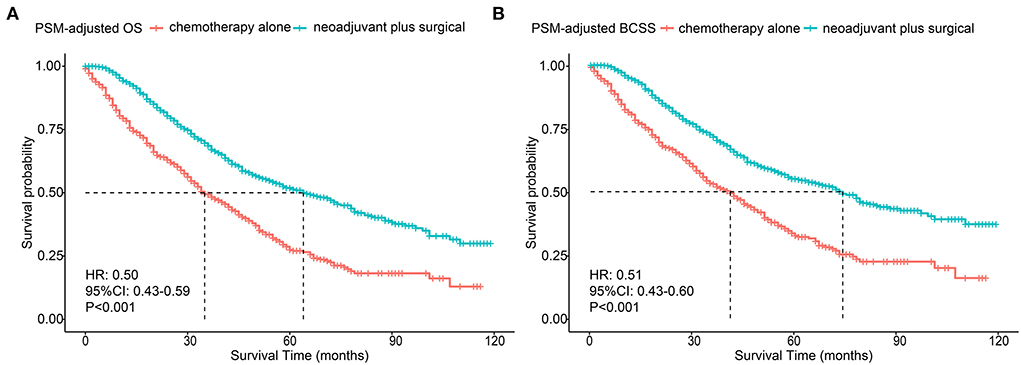
Figure 2. PSM-adjusted OS and BCSS of BMBC patients with neoadjuvant chemotherapy plus surgical treatment and chemotherapy alone. Kaplan–Meier (K–M) survival analysis: (A) OS of BMBC patients with neoadjuvant chemotherapy plus surgical treatment and chemotherapy alone; (B) BCSS of BMBC patients with neoadjuvant chemotherapy plus surgical treatment and chemotherapy alone. PSM, Propensity score matching OS, overall survival; BCSS, breast cancer-specific survival; BMBC, bone metastatic breast cancer; HR, hazard ratio; CI, confidence interval.
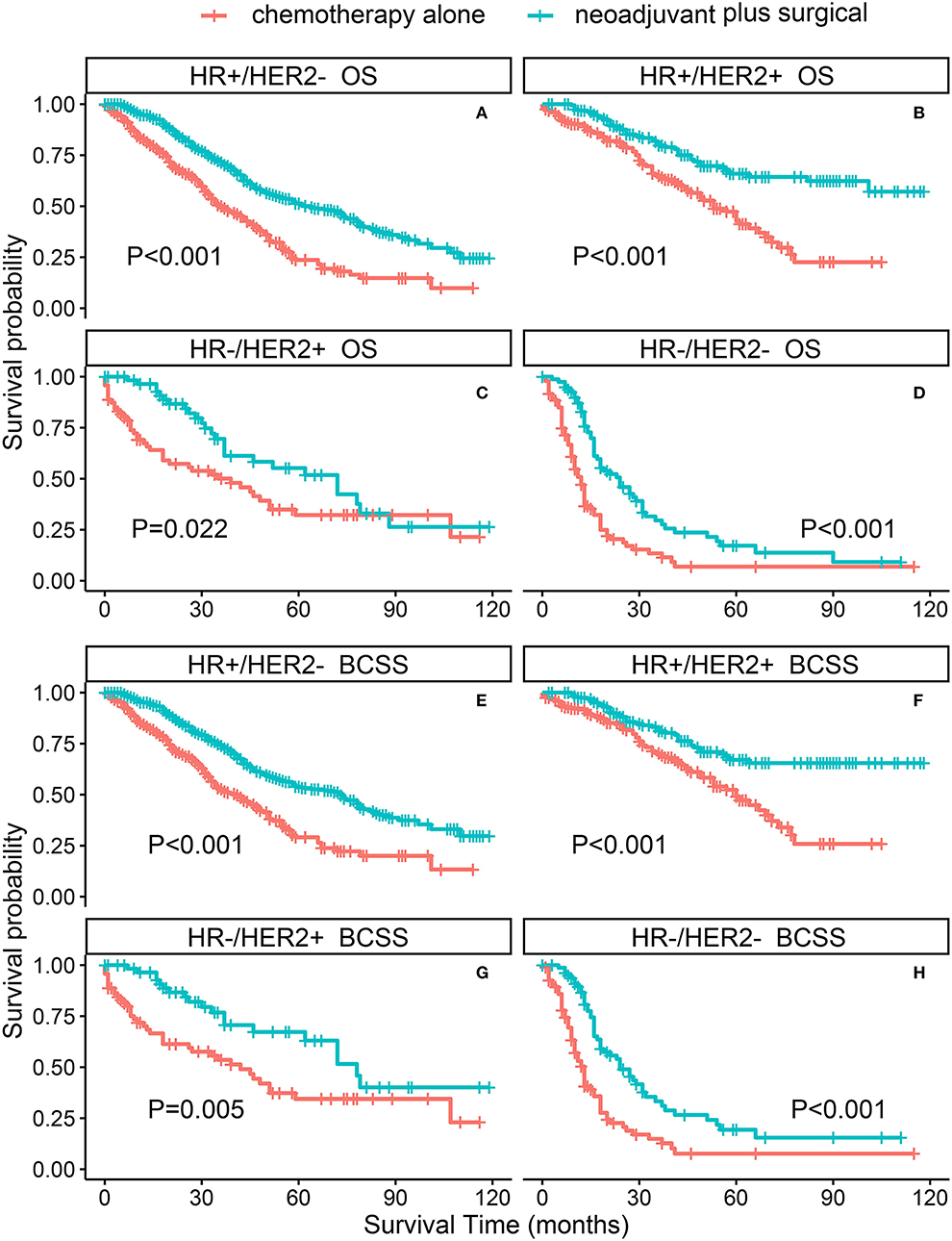
Figure 3. PSM-adjusted OS and BCSS of BMBC patients with neoadjuvant chemotherapy plus surgical treatment and chemotherapy alone (stratified by molecular subtype). Kaplan–Meier (K–M) survival analysis: (A) OS of BMBC patients with HR+/HER2- subtype; (B) OS of BMBC patients with HR+/HER2+ subtype; (C) OS of BMBC patients with HR–/HER2+ subtype; (D) OS of BMBC patients with HR–/HER2– subtype; (E) BCSS of BMBC patients with HR+/HER2– subtype; (F) BCSS of BMBC patients with HR+/HER2+ subtype; (G) BCSS of BMBC patients with HR–/HER2+ subtype; (H) BCSS of BMBC patients with HR-/HER2- subtype. OS, overall survival; BCSS, breast cancer-specific survival; BMBC, bone metastatic breast cancer; HR, hormone receptor; HER2, human epidermal growth factor receptor 2; PSM, propensity score matching.
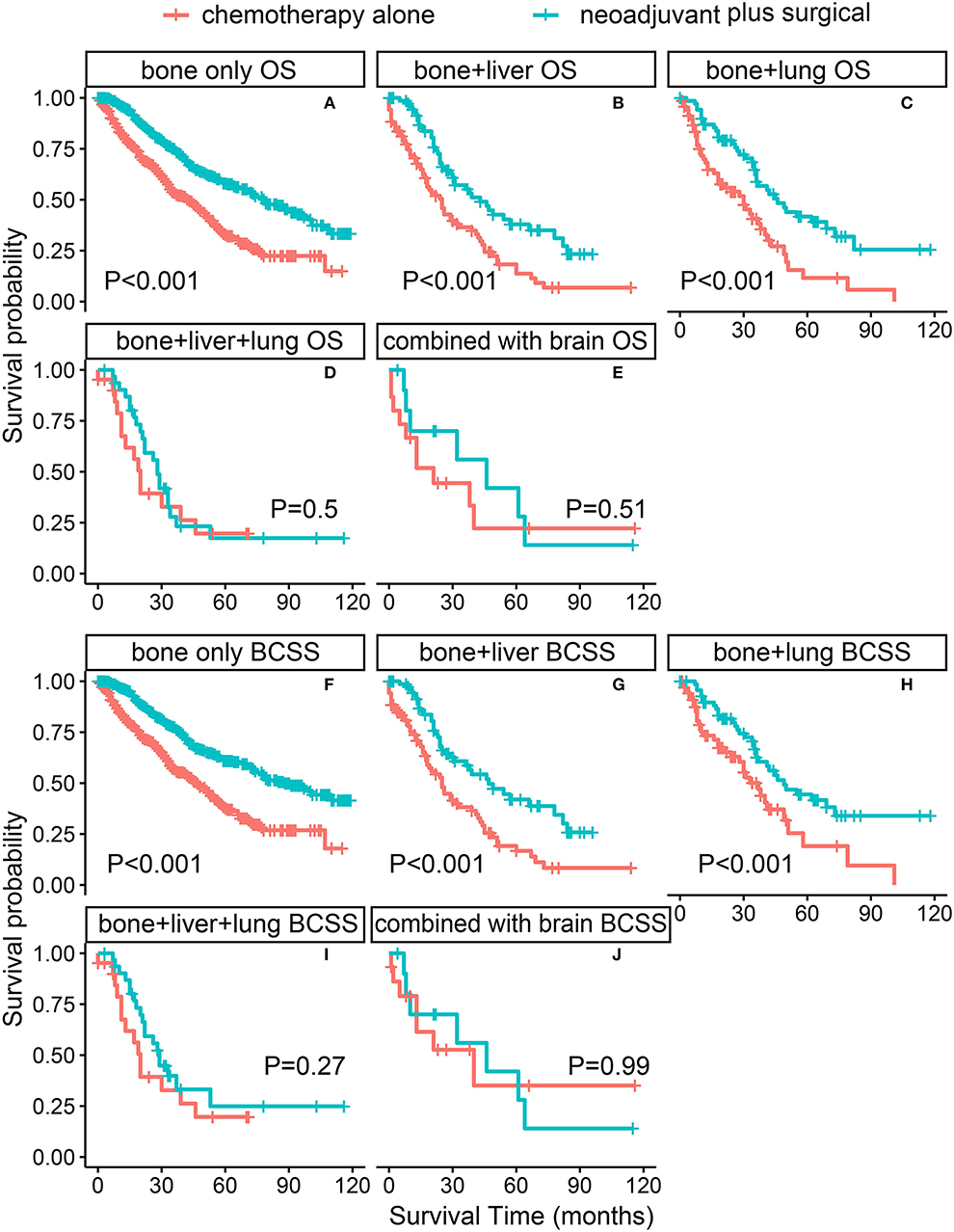
Figure 4. PSM-adjusted OS and BCSS of patients with BMBC in the neoadjuvant chemotherapy plus surgical treatment and chemotherapy alone groups (stratified by metastatic modality). Kaplan–Meier (K–M) survival analysis: (A) OS of patients with bone metastases only; (B) OS of patients with bone and liver metastases; (C) OS of patients with bone and lung metastases; (D) OS of patients with bone and liver and lung metastases; (E) OS of patients with BMBC combined with brain metastases; (F) BCSS of patients with bone metastases only; (G) BCSS of patients with bone and liver metastases; (H) BCSS of patients with bone and lung metastases; (I) BCSS of patients with bone and liver and lung metastases; (J) BCSS of patients with BMBC combined with brain metastases. OS, overall survival; BCSS, breast cancer-specific survival; PSM, Propensity score matching; BMBC, bone metastatic breast cancer.
Establishing and evaluating predictive models for estimating the prognosis of patients with BMBC
Given these results, we sought to build an XGBoost prediction model to estimate the OS of patients with BMBC at 1, 3, and 5 years. We divided the patients into train and test data according to 7:3, and to ensure the stability of the model, 10-fold cross-validation was used in the train set to assess the optimal number of subtrees. As shown in the figure, the logarithmic loss function was minimized at a number of 25 subtrees (Figure 5). From this, the “nrounds” parameter is determined and the model is then iteratively tested and adjusted to confirm other main hyperparameters to obtain the best model. We constructed predicted ROC curves for both the train and validation sets and calculated the corresponding AUCs. Our XGBoost model was highly effective in predicting the survival of patients with BMBC at 1 year (test set: AUC = 0.818; train set AUC = 0.845), 3 years (test set: AUC = 0.798; train set AUC = 0.839), and 5 years (test set: AUC = 0.791; train set AUC = 0.853) (Figure 6). Compared to traditional machine learning algorithms, SVM (1 year: AUC = 0.604; 3 years: AUC = 0.678; 5 years: AUC = 0.545), ID3 (1 year: AUC = 0.655; 3 years: AUC = 0.710; 5 years: AUC = 0.668) and KNN models (1 year: AUC = 0.607; 3 years: AUC = 0.664; 5 years: AUC = 0.596), XGBoost model performed significantly better (Table 4).
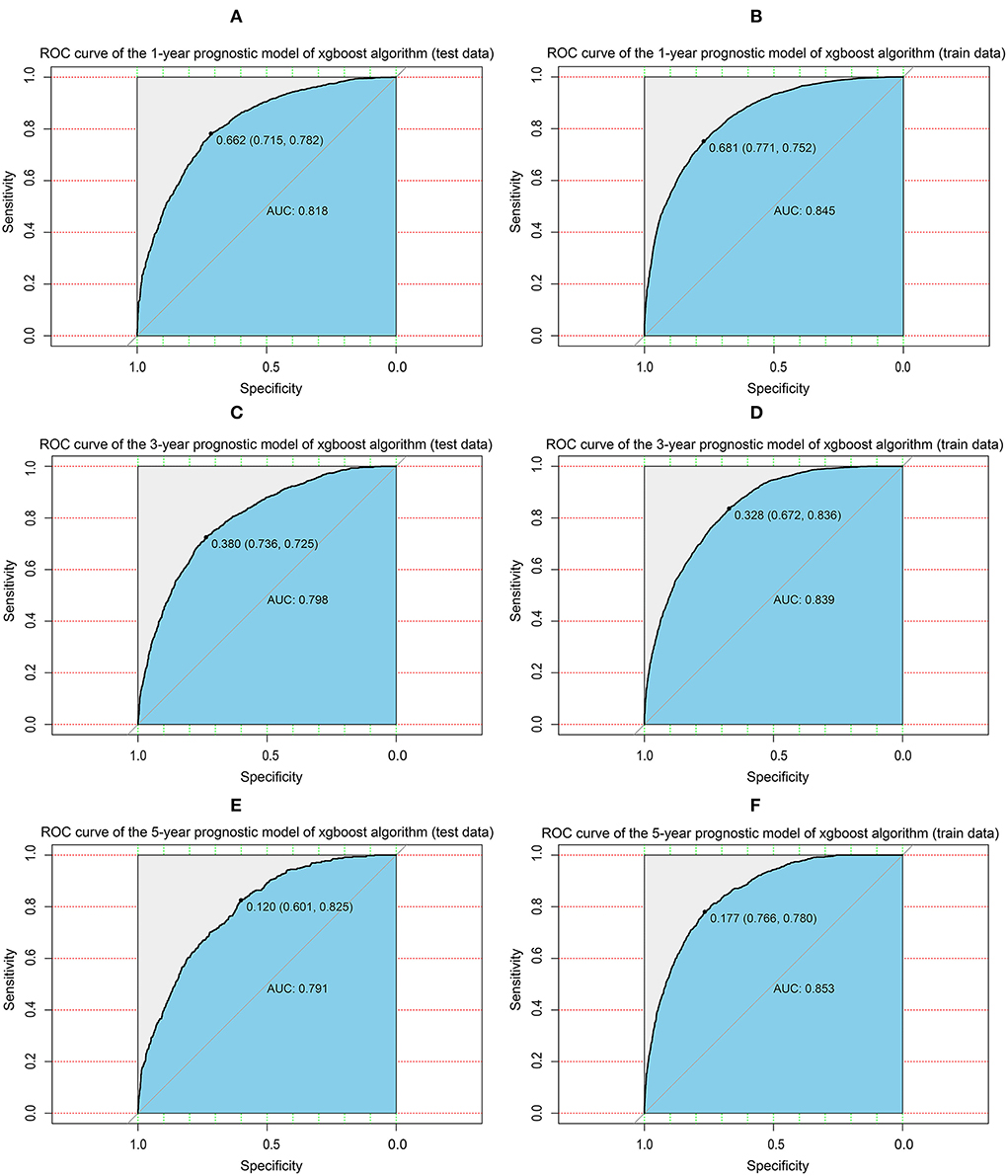
Figure 6. XGBoost model evaluation. (A) ROC curve for the 1-year prognostic model (test data); (B) ROC curve for the 1-year prognostic model (train data); (C) ROC curve for the 3-year prognostic model (test data); (D) ROC curve for the 3-year prognostic model (train data); (E) ROC curve for the 5-year prognostic model (test data); (F) ROC curve for the 5-year prognostic model (train data); ROC, receiver operating characteristic curve; AUC, area under the curve; XGBoost, extreme gradient boosting.
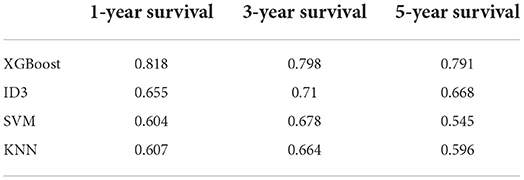
Table 4. Performance of prognostic models built by machine learning algorithms on the test data (area under the ROC curve).
Then, the accuracy of our XGBoost model was further evaluated by the confusion matrix. The 1-year survival prediction model was calculated to have a sensitivity of 0.79, a specificity of 0.72, and a correctness of 0.77 (Supplementary Figure 1A); the 3-year survival model had a sensitivity of 0.66, a specificity of 0.76, and a correctness of 0.74 (Supplementary Figure 1B); and the 5-year survival model had a sensitivity of 0.57, a specificity of 0.86, and a correctness of 0.85 (Supplementary Figure 1C). The 1-year model seemed more sensitive and the 3 and 5-year models were more specific. Overall, our models performed well.
We also assessed the ranking of clinical characteristics in terms of importance in the model. The results showed that molecular subtype, surgical treatment, age, liver metastases, and chemotherapy were the top five determinants of patient survival. Among them, the molecular subtype is the most important factor. In addition, chemotherapy was an important factor for short-term survival (1 year) (Supplementary Figure 2A), while surgery was more important for medium- to long-term patient survival (3 and 5 years) (Supplementary Figures 2B,C).
Discussion
Breast cancer exhibits metastatic properties, including bone, lung, liver, and brain, which leads to varied responses to treatment and patient prognosis (2). Bone metastases account for approximately 75% of metastatic cases (19), for these largest group patients of BMBC, who are considered incurable, the survival time is the most important concern. However, there is a lack of accurate prediction models in the clinic. Recently, some studies used nomograms to make several survival prediction models for patients with BMBC (7–12), but their accuracy rate is only about 70%. As a result, a more accurate and powerful model is needed. To our knowledge, the current study is the largest one to analyze the clinical characteristics and prognosis of patients with BMBC. The 1-, 3-, and 5-year OS of patients with BMBC is 66.39, 34.78 and 15.28%, respectively. Moreover, this study is the first to create an AI prognostic model for patients with BMBC and the model we made is the most accurate one to predict the survival of patients with BMBC.
In this analysis, several factors associated with improved outcomes were identified, including age < 50 years old, HR+/HER2+ subtype, white race, lower grade, lower T stage (T ≤ T2), no concurrent visceral metastases, ≥ 1 month from diagnosis to therapy, married, and income more than USD$70,000. Previous studies showed patients with BMBC of age < 40 years old were prone to better OS (6), while another one indicated the age < 60 years old was a protective factor (11), we analyzed more age groups, and found age < 50 years old was a feature for better OS and BCSS and the HR was increased with older age. The patients of the HR+/HER2+ subtype, rather than HR+/HER2– which usually present a preferred prognosis, showed the best survival among all subtypes in our analysis. This finding was similar to several previous studies (6, 11), and it might attribute to the progress of HER2 targeted therapy. Interestingly, we found if the time from diagnosis to treatment start was more than 1 month, the survival of patients with BMBC was even better than those with immediate treatment start. Of course, this does not mean the later treatment is better, if patients extended treatment delay indefinitely, they would die sooner. Treatment delays have a measurable impact on outcomes. Optimal times from diagnosis are < 90 days for surgery, < 120 days for chemotherapy, and < 365 days for radiotherapy (20). In large-scale hospitals, there can be more choices of systemic treatment, and the possible selection of optimal clinical trials can also have benefits for later-stage patients. Moreover, some previous studies have reported that shorter treatment delays are associated with poorer survival, because urgent treatment may be preferentially offered to patients who exhibit a higher symptom burden, which might lead to a worse prognosis (21–24). In addition, the treatment options also affect the time from diagnosis to treatment (25), for example, patients receiving chemotherapy alone or forgoing systemic therapy may have a shorter time from diagnosis to treatment. Our results imply that patients should not be worried about needing immediate treatment after diagnosis with BMBC, waiting for some relevant genetic or laboratory test to assess comprehensively their condition, and even searching for some appropriate clinical trials to enroll in could improve the therapeutic effect and prolong the survival time. It is reported that family income could affect the survival of patients with breast cancer (26); usually, patients with higher incomes have a better prognosis. We found that BMBC patients with an income of more than USD$70,000 had better OS and BCSS than those with income < USD$50,000, this income level dividing line was not reported before in this population, which could reflect their degree of cooperation with doctors in treatment.
For treatment, we found that primary tumor surgery, chemotherapy, and neoadjuvant chemotherapy could prolong OS and BCSS of patients with BMBC; however, radiotherapy showed an opposite effect in our analysis. Some studies showed that radiotherapy was not an independent prognostic factor of patients with BMBC (8, 10, 12), while other analyses reported that radiotherapy was associated with a significant survival advantage in patients with de novo Stage IV Breast Cancer (27, 28). These contradictory results may be due to different populations and clinical characteristics; we still need more detailed analysis to identify what kinds of patients would benefit from radiotherapy. Another controversial topic is whether surgical therapy for the primary site improves survival in patients presenting with de novo metastatic breast cancer. Many retrospective analyses of large cohort or mono-centric databases have shown a better prognosis of primary surgery in selected patients (11, 29–34); however, several randomized controlled trials indicated conflicting evidence (35–37), and a multicenter Turkish trial MF07-01 showed no difference in surgery arm of 3-year follow-up, but a statistically significant improvement in surgery arm of 4- to 10-year follow-up (38). We know that retrospective results are usually undermined for selection bias (women receiving surgery were younger and had biologically favorable tumors) (37), while prospective trials were also questioned for insufficient chemotherapy, deviation from contemporary practice, insufficient adapted p-value, and so on (11). Although each method has limitations and shows contradictory results, current studies imply that in well-selected patients, primary surgery might be a treatment option.
In this study, due to the SEER data of neoadjuvant chemotherapy therapy being first to open in April 2022, we are the first one to analyze the survival of patients with BMBC under surgery after neoadjuvant chemotherapy by SEER data, which could segment patients more precisely. We found that compared with chemotherapy alone, neoadjuvant chemotherapy plus surgical treatment significantly improved OS and BCSS in all molecular subtypes of patients with BMBC; however, this survival benefit depended on the metastatic burden. Only the patients with bone metastases only, bone and liver metastases, and bone and lung metastases could benefit from neoadjuvant chemotherapy plus surgical treatment, which indicated that the increase of metastatic burden, especially brain metastases reduced the effect of comprehensive treatment.
Despite the promising findings of the present study, there are some limitations of this research. First, although the SEER database covers about 30% of the USA population, clinical data on tumor subtypes and distant metastatic sites were collected only after 2010 in the SEER database and therefore limited the sample size of this study. Second, the SEER database offers a high representation of a general situation, but on the other side, not necessarily are suitable for applying to the Asian and Chinese populations on the basis of ethnic differences. Third, information about disease recurrence or subsequent sites of metastases was not collected in the SEER database. Thus, we could not investigate patients who developed bone metastases later in their remaining years, which may lead to bias in the results. Fourth, detailed treatment information for patients with bone metastases is not recorded in the SEER database, we cannot evaluate more on this. Even though the machine learning prognostic model achieved a higher accuracy rate, it lacked external validation to further enforce the reliability.
In summary, we constructed a machine learning prognostic model to provide a quantitative method to predict the survival of patients with BMBC, and our validation results indicate that this model should be highly reproducible in a similar patient population. We also identified potential prognostic factors for patients with BMBC and suggested that primary surgery followed by neoadjuvant chemotherapy therapy might increase survival in a selected subgroup of patients.
Data availability statement
The original contributions presented in the study are included in the article/Supplementary material, further inquiries can be directed to the corresponding authors.
Author contributions
Conceptualization: CL, JQ, and SZ. Methodology: CL and JQ. Formal analysis: CL. Data curation: ML. Writing—original draft preparation: JL, CF, and WW. Writing—review and editing: FW, XZ, CD, and YC. Supervision: YW and YZ. All authors have read and agreed to the published version of the manuscript.
Funding
This work was funded in part by the following: National Science Foundation of China (81903856 to XZ; 82174164 to SZ; 82103569 to JQ); Key Science and Technology Program of Shaanxi Province (2021KW-57 to XZ; 2021KW-60 to JQ). Scientific research fund of the Second Affiliated Hospital of Xi'an Jiaotong University [RC(XM)202004 to XZ]. Free exploring fund of Xi'an Jiaotong University (xzy012022096 to XZ; xzy012022097 to JQ). Medical basic - clinical integration and innovation project of Xi'an Jiaotong University (YXJLRH2022088 to JQ).
Acknowledgments
We thank all staff of the SEER database for their contribution to data collection, maintenance, distribution, and so on. Also, we would like to thank all the developers of the R programming package for selflessly sharing their code.
Conflict of interest
The authors declare that the research was conducted in the absence of any commercial or financial relationships that could be construed as a potential conflict of interest.
Publisher's note
All claims expressed in this article are solely those of the authors and do not necessarily represent those of their affiliated organizations, or those of the publisher, the editors and the reviewers. Any product that may be evaluated in this article, or claim that may be made by its manufacturer, is not guaranteed or endorsed by the publisher.
Supplementary material
The Supplementary Material for this article can be found online at: https://www.frontiersin.org/articles/10.3389/fpubh.2022.1003976/full#supplementary-material
Supplementary Figure 1. Confusion matrix of the XGBoost model's predicted results in the test set. (A) Confusion matrix in the 1-year prognostic model. (B) Confusion matrix in the 3-year prognostic model. (C) Confusion matrix in the 5-year prognostic model. TP, true positive; TN, true negative.
Supplementary Figure 2. The ranking of clinical characteristics in terms of importance in the XGBoost prognostic model. (A) The ranking of clinical characteristics in terms of importance in the 1-year prognostic model. (B) The ranking of clinical characteristics in terms of importance in the 3-year prognostic model. (C) The ranking of clinical characteristics in terms of importance in the 5-year prognostic model. XGBoost, extreme gradient boosting.
Supplementary Table 1. Results of the COX regression analysis [hazard ratio (HR), 95% CI, p-value].
References
1. Sung H, Ferlay J, Siegel RL, Laversanne M, Soerjomataram I, Jemal A, et al. Global cancer statistics 2020: globocan estimates of incidence and mortality worldwide for 36 cancers in 185 countries. CA Cancer J Clin. (2021) 71:209–49. doi: 10.3322/caac.21660
2. Liang Y, Zhang H, Song X, Yang Q. Metastatic heterogeneity of breast cancer: molecular mechanism and potential therapeutic targets. Semin Cancer Biol. (2020) 60:14–27. doi: 10.1016/j.semcancer.2019.08.012
3. Body JJ, Quinn G, Talbot S, Booth E, Demonty G, Taylor A, et al. Systematic review and meta-analysis on the proportion of patients with breast cancer who develop bone metastases. Crit Rev Oncol Hematol. (2017) 115:67–80. doi: 10.1016/j.critrevonc.2017.04.008
4. Fornetti J, Welm AL, Stewart SA. Understanding the bone in cancer metastasis. J Bone Mineral Res. (2018) 33:2099–113. doi: 10.1002/jbmr.3618
5. Satcher RL, Zhang XHF. Evolving Cancer–Niche interactions and therapeutic targets during bone metastasis. Nat Rev Cancer. (2021) 22:85–101. doi: 10.1038/s41568-021-00406-5
6. Xiong Z, Deng G, Huang X, Li X, Xie X, Wang J, et al. Bone metastasis pattern in initial metastatic breast cancer: a population-based study. Cancer Manag Res. (2018) 10:287–95. doi: 10.2147/CMAR.S155524
7. Wang Z, Shao H, Xu Q, Wang Y, Ma Y, Diaty DM, et al. Establishment and verification of prognostic nomograms for young women with breast cancer bone metastasis. Front Med. (2022) 9:840024. doi: 10.3389/fmed.2022.840024
8. Wang Z, Cheng Y, Chen S, Shao H, Chen X, Wang Z, et al. Novel prognostic nomograms for female patients with breast cancer and bone metastasis at presentation. Ann Transl Med. (2020) 8:197. doi: 10.21037/atm.2020.01.37
9. Tu Q, Hu C, Zhang H, Peng C, Kong M, Song M, et al. Establishment and validation of novel clinical prognosis nomograms for luminal a breast cancer patients with bone metastasis. Biomed Res Int. (2020) 2020:1972064. doi: 10.1155/2020/1972064
10. Huang Z, Hu C, Liu K, Yuan L, Li Y, Zhao C, et al. Risk factors, prognostic factors, and nomograms for bone metastasis in patients with newly diagnosed infiltrating duct carcinoma of the breast: a population-based study. BMC Cancer. (2020) 20:1145. doi: 10.1186/s12885-020-07635-1
11. Liu D, Wu J, Lin C, Andriani L, Ding S, Shen K, et al. Breast subtypes and prognosis of breast cancer patients with initial bone metastasis: a population-based study. Front Oncol. (2020) 10:580112. doi: 10.3389/fonc.2020.580112
12. Yao YB, Zheng XE, Luo XB, Wu AM. Incidence, prognosis and nomograms of breast cancer with bone metastases at initial diagnosis: a large population-based study. Am J Transl Res. (2021) 13:10248–61.
13. Tran KA, Kondrashova O, Bradley A, Williams ED, Pearson JV, Waddell N. Deep learning in cancer diagnosis, prognosis and treatment selection. Genome Med. (2021) 13:152. doi: 10.1186/s13073-021-00968-x
14. Khadse V, Mahalle PN, Biraris SV, IEEE editors. An empirical comparison of supervised machine learning algorithms for internet of things data. In: 4th International Conference on Computing Communication Control and Automation (ICCUBEA) (2018).
15. Khadse V, Mahalle PN, Biraris SV. IEEE: an empirical comparison of supervised machine learning algorithms for internet of things data. In: 4th International Conference on Computing Communication Control and Automation (ICCUBEA) (2018).
16. Yuan KC, Tsai LW, Lee KH, Cheng YW, Hsu SC, Lo YS, et al. The development an artificial intelligence algorithm for early sepsis diagnosis in the intensive care unit. Int J Med Inform. (2020) 141:104176. doi: 10.1016/j.ijmedinf.2020.104176
17. Yu Y, Tran H. An Xgboost-based fitted Q iteration for finding the optimal STI strategies for HIV patients. IEEE Trans Neural Netw Learn Syst. (2022) 2022:1–9. doi: 10.1109/TNNLS.2022.3176204
18. Ye Q, Chai X, Jiang D, Yang L, Shen C, Zhang X, et al. Identification of active molecules against Mycobacterium tuberculosis through machine learning. Brief Bioinform. (2021) 22:bbab068. doi: 10.1093/bib/bbab068
19. Tulotta C, Ottewell P. The role of Il-1b in breast cancer bone metastasis. Endocr Relat Cancer. (2018) 25:R421–r34. doi: 10.1530/erc-17-0309
20. Bleicher RJ. Timing and delays in breast cancer evaluation and treatment. Ann Surg Oncol. (2018) 25:2829–38. doi: 10.1245/s10434-018-6615-2
21. Diaconescu R, Lafond C, Whittom R. Treatment delays in non-small cell lung cancer and their prognostic implications. J Thoracic Oncol. (2011) 6:1254–9. doi: 10.1097/JTO.0b013e318217b623
22. González-Barcala FJ, García-Prim JM, Alvarez-Dobaño JM, Moldes-Rodríguez M, García-Sanz MT, Pose-Reino A, et al. Effect of delays on survival in patients with lung cancer. Clin Transl Oncol. (2010) 12:836–42. doi: 10.1007/s12094-010-0606-5
23. Gould MK, Ghaus SJ, Olsson JK, Schultz EM. Timeliness of care in veterans with non-small cell lung cancer. Chest. (2008) 133:1167–73. doi: 10.1378/chest.07-2654
24. Lopez-Cedrún JL, Varela-Centelles P, Otero-Rico A, Vázquez-Mahía I, Seoane J, Castelo-Baz P, et al. Overall time interval (“total diagnostic delay”) and mortality in symptomatic oral cancer: a u-shaped association. Oral Oncol. (2020) 104:104626. doi: 10.1016/j.oraloncology.2020.104626
25. Polesel J, Furlan C, Birri S, Giacomarra V, Vaccher E, Grando G, et al. The impact of time to treatment initiation on survival from head and neck cancer in north-eastern Italy. Oral Oncol. (2017) 67:175–82. doi: 10.1016/j.oraloncology.2017.02.009
26. Coughlin SS. Social determinants of breast cancer risk, stage, and survival. Breast Cancer Res Treat. (2019) 177:537–48. doi: 10.1007/s10549-019-05340-7
27. Zhang J, Luo S, Qiu Z, Lin Y, Song C. Impact of postoperative radiotherapy on survival of patients with de novo stage IV breast cancer: a population-based study from the seer database. Front Oncol. (2021) 11:625628. doi: 10.3389/fonc.2021.625628
28. Kim YJ, Kim Y-J, Kim YB, Lee IJ, Kwon J, Kim K, et al. Effect of postoperative radiotherapy after primary tumor resection in de novo stage iv breast cancer: a multicenter retrospective study (Krog 19-02). Cancer Res Treatment. (2022) 54:478–87. doi: 10.4143/crt.2021.632
29. Blanchard DK, Shetty PB, Hilsenbeck SG, Elledge RM. Association of surgery with improved survival in stage IV breast cancer patients. Ann Surg. (2008) 247:732–8. doi: 10.1097/SLA.0b013e3181656d32
30. Fields RC, Jeffe DB, Trinkaus K, Zhang Q, Arthur C, Aft R, et al. Surgical resection of the primary tumor is associated with increased long-term survival in patients with stage IV breast cancer after controlling for site of metastasis. Ann Surg Oncol. (2007) 14:3345–51. doi: 10.1245/s10434-007-9527-0
31. Gnerlich J, Jeffe DB, Deshpande AD, Beers C, Zander C, Margenthaler JA. Surgical removal of the primary tumor increases overall survival in patients with metastatic breast cancer: analysis of the 1988-2003 seer data. Ann Surg Oncol. (2007) 14:2187–94. doi: 10.1245/s10434-007-9438-0
32. Lang JE, Tereffe W, Mitchell MP, Rao R, Feng L, Meric-Bernstam F, et al. Primary tumor extirpation in breast cancer patients who present with stage IV disease is associated with improved survival. Ann Surg Oncol. (2013) 20:1893–9. doi: 10.1245/s10434-012-2844-y
33. Pons-Tostivint E, Kirova Y, Lusque A, Campone M, Geffrelot J, Mazouni C, et al. Survival impact of locoregional treatment of the primary tumor in de novo metastatic breast cancers in a large multicentric cohort study: a propensity score-matched analysis. Ann Surg Oncol. (2019) 26:356–65. doi: 10.1245/s10434-018-6831-9
34. Wang K, Shi Y, Li ZY, Xiao YL Li J, Zhang X, et al. Metastatic pattern discriminates survival benefit of primary surgery for de novo stage iv breast cancer: a real-world observational study. Eur J Surg Oncol. (2019) 45:1364–72. doi: 10.1016/j.ejso.2019.02.013
35. Badwe R, Hawaldar R, Nair N, Kaushik R, Parmar V, Siddique S, et al. Locoregional treatment versus no treatment of the primary tumour in metastatic breast cancer: an open-label randomised controlled trial. Lancet Oncol. (2015) 16:1380–8. doi: 10.1016/s1470-2045(15)00135-7
36. Bjelic-Radisic V, Fitzal F, Knauer M, Steger G, Egle D, Greil R, et al. Primary surgery versus no surgery in synchronous metastatic breast cancer: patient-reported quality-of-life outcomes of the prospective randomized multicenter ABCSG-28 posytive trial. BMC Cancer. (2020) 20:392. doi: 10.1186/s12885-020-06894-2
37. Khan SA, Zhao F, Goldstein LJ, Cella D, Basik M, Golshan M, et al. Early local therapy for the primary site in de novo stage IV breast cancer: results of a randomized clinical trial (Ea2108). J Clin Oncol. (2022) 40:978–87. doi: 10.1200/jco.21.02006
38. Soran A, Ozmen V, Ozbas S, Karanlik H, Muslumanoglu M, Igci A, et al. Primary surgery with systemic therapy in patients with de novo stage iv breast cancer: 10-year follow-up; Protocol Mf07-01 randomized clinical trial. J Am Coll Surg. (2021) 233:742–51.e5. doi: 10.1016/j.jamcollsurg.2021.08.686
Keywords: breast cancer, bone metastases, XGBoost algorithm, neoadjuvant chemotherapy, SEER
Citation: Li C, Liu M, Li J, Wang W, Feng C, Cai Y, Wu F, Zhao X, Du C, Zhang Y, Wang Y, Zhang S and Qu J (2022) Machine learning predicts the prognosis of breast cancer patients with initial bone metastases. Front. Public Health 10:1003976. doi: 10.3389/fpubh.2022.1003976
Received: 28 July 2022; Accepted: 05 September 2022;
Published: 26 September 2022.
Edited by:
Yu Jin Lim, Kyung Hee University, South KoreaReviewed by:
Jae Sik Kim, Soonchunhyang University Hospital Seoul, South KoreaYoungkyong Kim, Kyung Hee University Medical Center, South Korea
Copyright © 2022 Li, Liu, Li, Wang, Feng, Cai, Wu, Zhao, Du, Zhang, Wang, Zhang and Qu. This is an open-access article distributed under the terms of the Creative Commons Attribution License (CC BY). The use, distribution or reproduction in other forums is permitted, provided the original author(s) and the copyright owner(s) are credited and that the original publication in this journal is cited, in accordance with accepted academic practice. No use, distribution or reproduction is permitted which does not comply with these terms.
*Correspondence: Shuqun Zhang, c2h1cXVuX3poYW5nMTk3MSYjeDAwMDQwOzE2My5jb20=; Jingkun Qu, cXVqaW5na3VuJiN4MDAwNDA7eGp0dS5lZHUuY24=