- 1College of Environment, Liaoning University, Shenyang, Liaoning, China
- 2Liaoning Provincial Ecological and Environmental Affairs Service Center, Shenyang, Liaoning, China
- 3National Center for Human Genetic Resources, Beijing, China
- 4Department of Environmental Health, School of Public Health, Key Laboratory of Environmental Health Damage Research and Assessment, China Medical University, Shenyang, Liaoning, China
Background: Epidemiological surveys have found that particulate matter 2.5 (PM2.5) plays an important role in hypothyroidism. However, due to the methodological limitations of traditional observational studies, it is difficult to make causal inferences. In the present study, we assessed the causal association between PM2.5 concentrations and risk of hypothyroidism using two-sample Mendelian randomization (TSMR).
Methods: We performed TSMR by using aggregated data from genome-wide association studies (GWAS) on the IEU Open GWAS database. We identified seven single nucleotide polymorphisms (SNPs) associated with PM2.5 concentrations as instrumental variables (IVs). We used inverse-variance weighting (IVW) as the main analytical method, and we selected MR-Egger, weighted median, simple model, and weighted model methods for quality control.
Results: MR analysis showed that PM2.5 has a positive effect on the risk of hypothyroidism: An increase of 1 standard deviation (SD) in PM2.5 concentrations increases the risk of hypothyroidism by ~10.0% (odds ratio 1.10, 95% confidence interval 1.06–1.13, P = 2.93E-08, by IVW analysis); there was no heterogeneity or pleiotropy in the results.
Conclusion: In conclusion, increased PM2.5 concentrations are associated with an increased risk of hypothyroidism. This study provides evidence of a causal relationship between PM2.5 and the risk of hypothyroidism, so air pollution control may have important implications for the prevention of hypothyroidism.
Introduction
Hypothyroidism refers to thyroid hormone deficiency. The diagnosis is mainly based on serum thyroid-stimulating hormone (TSH) and free thyroxine (FT4) levels (1). As a common condition, the prevalence of clinical hypothyroidism is ~0.2–5.3% in the general European population and 0.3–3.7% in the United States (2). Hypothyroidism can lead to an increased risk of hyperlipidemia and the development of cardiovascular disease and even heart failure, somatic and neuromuscular symptoms, reproductive disorders, and other adverse outcomes (3). Due to the widespread use of thyroid function tests (4), researchers have focused on exploring the factors that contribute to hypothyroidism, and there is growing evidence of the detrimental effects of exposure to environmental factors on thyroid function (5–8).
Epidemiological studies have shown that exposure to nitrogen dioxide (NO2) and carbon monoxide (CO) is associated with increased TSH and decreased FT4 (9). Particulate matter (PM) significantly affects the binding of thyroxine to transthyretin and reduces thyroxine levels (10). In 2015, a survey of about 15.1 million neonates in China showed that maternal exposure to air pollution during pregnancy may affect fetal thyroid development (8). Moreover, the survey revealed that PM2.5 exposure levels are positively associated with the risk of congenital hypothyroidism in offspring (8). To date, evidence on whether air pollution exposure impairs thyroid function remains limited, and current observational studies cannot confirm a causal relationship between air pollution and hypothyroidism.
When assessing the health risks of environmental pollutant exposure, consideration of genetic polymorphisms may provide better insights into individual environmental health risks. Previous studies have shown that adverse health outcomes due to environmental exposures are influenced by changes in gene expression (11, 12). Women carrying the GPX4-rs376102 AC/CC genotype are more sensitive to air pollutants and more likely to have preterm births (13). At high exposure levels of PM10, ozone (O3) and mean pollution standard index (PSI), children carrying the thrombomodulin-33G/A polymorphism (GA + AA genotype) are at higher risk of atherosclerosis (14). However, no studies have examined the combined effect of genetic polymorphisms and PM2.5 on the risk of hypothyroidism. With the advent of the post-genome-wide association study (GWAS) era, many efforts have been made to move beyond genetic associations to causality and mechanistic examination. Mendelian randomization (MR) uses single nucleotide polymorphism (SNP) as an instrumental variable (IV) and integrating existing GWAS summary statistics for causal inference. Supported by the fact that parental alleles are randomly assigned at the time of conception, the MR design is similar to a randomized controlled trial, that can effectively avoid the influence of confounding factors and reverse causality (15). A large number of GWAS provide an abundant data resource for MR studies (16). Many scholars have used MR studies to explore the causal relationship between hypothyroidism and systemic lupus erythematosus, hepatocellular carcinoma, and type 1 diabetes (17–19). However, the causal relationship between PM2.5 and hypothyroidism remains unclear.
Taken together, we have raised the hypotheses that these PM2.5 exposure may causally contribute to the development of hypothyroidism. In order to address the important gap in literature regarding this research question, we performed a two-sample Mendelian randomization (TSMR) study using the GWAS dataset publicly available on the IEU Open GWAS database to evaluate the causal relationship between PM2.5 concentrations and the risk of hypothyroidism.
Materials and methods
Study design and data sources
We conducted a TSMR analysis base on the summary-level data from the IEU Open GWAS database (https://gwas.mrcieu.ac.uk/datasets). The exposure data were PM2.5 GWAS summary dataset. The outcome data were GWAS summary dataset. The personal data of the subjects in this study was obtained from the UK Biobank, a large prospective study with over 500,000 UK participants (20). The detailed procedures for phenotyping, genetic detail, genome-wide genotyping, imputation and quality control of UK Biobank participants have been described elsewhere (21, 22). All participants had given informed consent in the corresponding original studies.
The PM2.5 GWAS summary dataset (GWAS ID:ukb-b-10817) included 423,796 participants of European ancestry. PM2.5 concentrations at participants' home addresses were estimated using a Land Use Regression (LUR) model (23).
The hypothyroidism GWAS summary dataset (GWAS ID:ukb-b-19732) contained 462,933 individuals of European descent, including 22,687 cases and 440,246 controls. Hypothyroidism cases were defined on the basis of clinical diagnosis and self-reported. Supplementary Table 1 presented the demographics of the patients included in GWAS summary dataset.
Selection of instrumental variables
As shown in Figure 1, to construct valid IVs, genetic variation must satisfy the three assumptions of MR. (1) Genetic IVs of PM2.5 are significantly associated with PM2.5 exposure levels. (2) The association between genetic IVs of PM2.5 and hypothyroidism is independent of confounding factors. (3) Genetic IVs of PM2.5 can only affect hypothyroidism risk through PM2.5 exposure. The study followed the Strengthening the Reporting of Observational Studies in Epidemiology using Mendelian Randomization (STROBE-MR) guideline (24), and the STORBE-MR checklist is provided in the Supplementary Table 2.
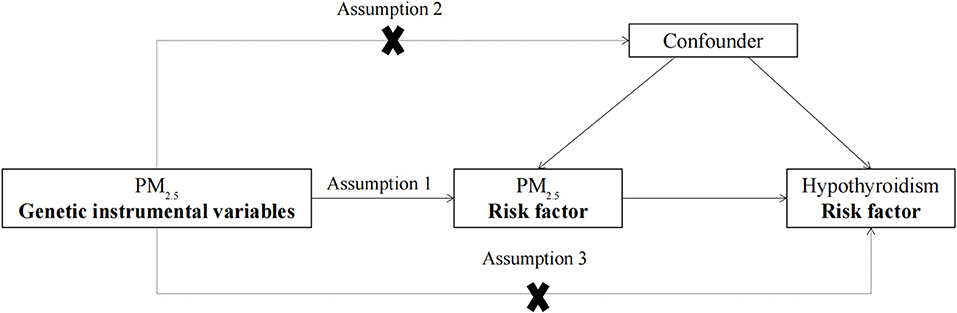
Figure 1. The design flow chart for the MR study. MR assumptions: assumption 1, 2, and 3. The solid line represents direct putative causal effects that PM2.5 genetic instrumental variants are reliably associated with I PM2.5 levels and influence the risk of hypothyroidism through the PM2.5 in assumption 1. The dotted line represents that PM2.5 genetic instrumental variants are not associated with any measured and unmeasured confounders and do not influence the risk of hypothyroidism through other pathways in assumptions 2 and 3, respectively. MR, Mendelian randomization; PM2.5, particulate matter 2.5.
To meet assumption 1, we selected the corresponding single nucleotide polymorphisms (SNPs) for PM2.5 exposure at the threshold of genome-wide significance (P < 5 × 10−8). Linkage disequilibrium (LD) was estimated between SNPs to select independent genetic variants using clump parameter in R version 4.1.3 software (distance window 5,000 kb, linkage disequilibrium coefficient r2 <0.01 using the R packages “TwoSampleMR”) (25). We found eight SNPs that are significantly associated (P < 5 × 10−8) with PM2.5 exposure levels without LD, as shown in Supplementary Table 3.
In our MR analysis, pleiotropy testing was required to ensure that IVs did not influence hypothyroidism risk through other confounders or other biological pathways independent of PM2.5 exposure. The MR-Egger regression effects model can provide pleiotropy-corrected causal estimates in MR, assessing instrument strength independently of the null causality hypothesis under the direct effect assumption (26). Moreover, the method can work even if all selected SNPs are not unbiased estimates (26). By judging whether there is statistical significance between the intercept and 0, it indicates whether there is horizontal pleiotropy in IVs. MR-PRESSO enables a systematic assessment of the role of pleiotropy in MR (27). It includes three components: MR-PRESSO global testing, MR-PRESSO outlier testing, and MR-PRESSO distortion testing. The statistical threshold for IVs that may have horizontal pleiotropy is P < 0.05.
We used Cochran's Q test to evaluate heterogeneity in the estimates of heterogeneity calculated by the inverse-variance weighting (IVW) and MR-Egger models (28, 29). P > 0.05 indicated no significant heterogeneity in the screened IVs.
TSMR analysis
In this TSMR study, we used IVW, MR-Egger, weighted median model, simple model, and weighted model methods to evaluate the causal relationship between PM2.5 exposure and hypothyroidism risk (30–32). The basic idea of IVW is to use the Wald ratio to obtain estimates of causal effects based on a single genetic IV, and then select a fixed-effects model to meta-aggregate multiple estimates of causal effects based on a single genetic IV. The IVW estimate is the combined causal effect estimate (33). IVW can provide reliable causal estimates without directed pleiotropy and is widely used in MR studies (34). We used MR-Egger, weighted median model, simple model, and weighted model methods to verify the causal association between exposure factors and outcomes. This endeavor has improved the accuracy of the findings (30).
Sensitivity analysis
We used the leave-one-out method to analyze the influence of a single SNP on the results of TSMR analysis (35). By removing SNPs one by one and performing a meta-analysis on the remaining SNPs, we estimated the MR result including all remaining SNPs and compared it to the result with all SNPs. If the MR result changes significantly after excluding one SNP, then this SNP may be directly related to the results, violating assumption 3 (36).
Statistical analysis
We conducted statistical analysis using R version 4.1.3 (R Foundation for Statistical Computing, Vienna, Austria) using the packages “TwoSampleMR” (25) and “MR-PRESSO” (27). The threshold of statistical significance for evidence of pleiotropy is P < 0.05.
Results
Extraction of genetic IVs of PM2.5 from the hypothyroidism GWAS dataset
We extracted corresponding information for seven genetic variants associated with PM2.5 concentrations in the hypothyroid GWAS dataset. The details of these seven IVs were presented in Table 1.
Pleiotropy and heterogeneity analysis
Both MR-Egger intercept and the MR-PRESSO test showed no significant pleiotropy (P > 0.05, Table 2). This indicated that the seven SNPs do not affect hypothyroidism through biological pathways independent of PM2.5 exposure. In addition, both MR-Egger and IVW showed P > 0.05 in Cochran's Q-test (Table 2), indicating that the seven genetic variants of PM2.5 did not have significant heterogeneity in the hypothyroidism GWAS dataset. Therefore, we could use the seven selected genetic variants of PM2.5 exposure as effective IVs for TSMR analysis.

Table 2. Pleiotropy and heterogeneity test of PM2.5 genetic instrumental variables in GWAS for hypothyroidism.
TSMR analysis of PM2.5 level and hypothyroidism
Our TSMR analysis revealed the following odds ratios (ORs) and 95% confidence intervals (CIs): (1) in the MR-egger model, OR = 1.12, 95% CI = 1.00–1.25, P = 0.119; (2) in the weighted median model, OR = 1.10, 95% CI = 1.06–1.14, P < 0.001; (3) in the IVW model, OR = 1.10, 95% CI 1.06–1.13, P < 0.001; (4) in the simple model, OR = 1.11, 95% CI 1.04–1.19, P < 0.05; and (5) in the weighted model, OR = 1.12, 95% CI = 1.05–1.19, P < 0.05. Although the MR-Egger model results were not significant, the IVW and median weighted model results were significant, and the ORs of the five models are all positive. This finding indicated that each standard error increase in the level of PM2.5 exposure was significantly associated with increased risk of hypothyroidism (Figure 2, Table 3). As shown in Figure 3, the regression lines obtained by these five methods were in the same direction, and the promoting effect of a single SNP on hypothyroidism increased as the effect of a single SNP on the PM2.5 exposure level increases.
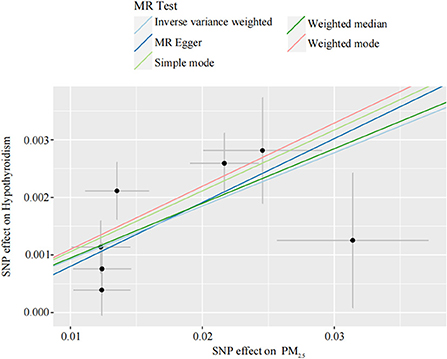
Figure 2. Individual estimates about the putative causal effect of PM2.5 on hypothyroidism. The x-axis shows the SNP effect and SE on each PM2.5. The y-axis shows the SNP effect and SE on hypothyroidism. The regression line for MR Egger, weighted median, inverse variance weighted, simple mode, and weighted mode is shown. PM2.5, particulate matter 2.5; SNP, single nucleotide polymorphism; SE, standard error.
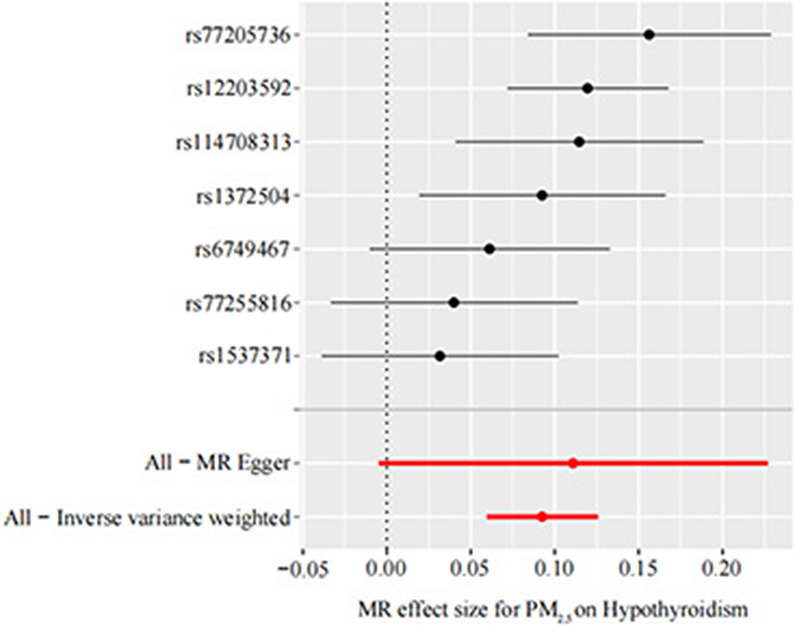
Figure 3. Forest plot of PM2.5 associated with risk of hypothyroidism. The x-axis shows the MR effect size for PM2.5 on hypothyroidism. The y-axis shows the analysis for each of the SNPs. MR, Mendelian randomization; PM2.5, particulate matter 2.5; SNP, single nucleotide polymorphism.
Sensitivity analysis
We performed sensitivity analysis of the TSMR results by using the leave-one-out method to determine whether the MR results were sensitive to an IV. Each black dot in the forest plot represents a TSMR analysis (using the IVW method), excluding that specific SNP; an overall analysis including all SNPs is shown for comparison (Figure 4). The lines of all IVs are on the right side of 0. Moreover, removing each SNP does not have a fundamental impact on the results. The TSMR results in this study are relatively robust, suggesting that PM2.5 is a risk factor for hypothyroidism.
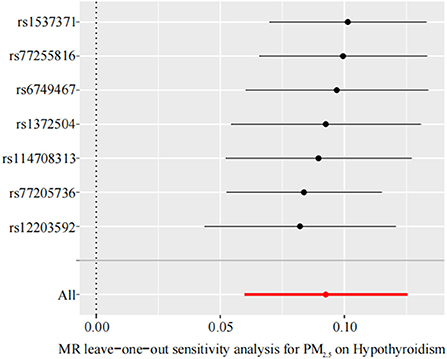
Figure 4. Leave-one-out sensitivity analysis for the effect of PM2.5 on hypothyroidism. The x-axis shows MR leave-one-out sensitivity analysis for PM2.5 on hypothyroidism. The y-axis shows the analysis for the effect of leave-one-out of SNPs on hypothyroidism. MR, Mendelian randomization; PM2.5, particulate matter 2.5; SNP, single nucleotide polymorphism.
Discussion
To date, a number of epidemiological studies have found that specific pollutants in the air are risk factors for hypothyroidism (37–40), but due to the methodological limitations of traditional observational studies, it is difficult to determine the causal relationship between the two. MR is based on the premise that human genetic variants are randomly distributed in the population and that these genetic variants are largely independent of confounders, and can be used as IVs to assess the causal association between exposure and outcome (41). In the present study, we assessed the causal association between PM2.5 concentrations and the risk of hypothyroidism using TSMR analysis based on a large-scale GWAS dataset. We found that in the European population, increased PM2.5 concentrations are associated with an increased risk of hypothyroidism. Our findings indicate a strong causal relationship between PM2.5 concentrations and hypothyroidism.
A large epidemiological survey of five cohorts from Europe and the United States found that higher PM2.5 concentrations are associated with higher odds of hypothyroidism in pregnant women (OR 1.21 per 5 μg/m3 change; 95% CI 1.00–1.47) (42). Other studies have reported that PM2.5 exposure affects thyroid function and thyroid hormone secretion. Wang et al. found that PM2.5 exposure during pregnancy is significantly negatively correlated with maternal serum FT4 levels (43), and there have been similar results in pregnant women in other regions (44, 45). In traditional observational epidemiological studies, confounding factors often interfere with the results, making the interpretation of etiology unreliable. This study is the first to investigate the causal relationship between PM2.5 concentrations and hypothyroidism using TSMR. Our findings are similar to those of traditional observational studies, showing that elevated PM2.5 concentrations are significantly associated with an increased risk of hypothyroidism (OR 1.10, 95% CI 1.06–1.13, P = 2.93E-08). These findings show that the causal association between genetic variation in PM2.5 and increased hypothyroidism risk is robust. Therefore, improving air quality and reducing PM2.5 concentrations can effectively reduce the risk of hypothyroidism.
The mechanism by which PM2.5 increases the risk of hypothyroidism remains unclear. Compared with PM10, PM2.5 has a smaller particle size and can reach the distal lung segments including the alveoli, enter the blood, and penetrate the blood barriers of multiple organs such as the brain, liver, and kidney, posing a greater threat to health (46, 47). Studies have found that PM2.5 can inhibit the gene expression and activity of endogenous antioxidant enzymes (48), activate the body's oxidative stress response, and promote dysfunction in multiple organs and systems (49, 50). Previous studies have reported that increased traffic-related PM2.5 concentrations are associated with altered responses to inflammatory markers (51). Animal experiments have found that artificial PM2.5 exposure can induce increased levels of interleukin (IL)-1, IL-6 and tumor necrosis factor α (TNFα) in rats, thereby increasing the risk of nasal lesions (52). Hypothyroidism is associated with disturbed cytokine concentrations, an abundance of reactive oxygen species (ROS), and altered signal transduction in most parts of the brain (53). In addition, chronic inflammation plays an important role in the pathogenesis of many diseases, including hypothyroidism (54). A recent experimental study in female rats found that PM2.5 exposure reduces circulating thyroid hormone levels by interrupting thyroid hormone biosynthesis, biotransformation, and transport; by inducing oxidative stress and inflammatory responses; and ultimately by activating the hypothalamic–pituitary–thyroid axis and inducing the production of hepatic transthyretin (55). Epidemiological studies found that a 10 μg/m3 increase in PM2.5 is associated with a 0.12 μmol/L decrease in FT4 and a 0.07 μmol/L increase in FT3, and the FT4/FT3 ratio is negatively correlated with PM2.5 (56). Based on the above findings, we hypothesize that high PM2.5 concentrations induce oxidative stress and inflammatory responses in the body, which in turn deregulate thyroid hormone secretion, decrease serum FT4 levels, and increase the incidence of hypothyroidism.
Our MR study has several advantages. First, we used TSMR to analyze the causal relationship between PM2.5 concentrations and hypothyroidism, making up for the insufficiency of traditional observational studies and adding new evidence for assessing the health risks of environmental pollutants. Second, this study benefits from large-scale PM2.5 GWAS (n = 423,796 individuals from Europe) and hypothyroidism GWAS (n = 462,933 individuals from Europe) datasets. Moreover, because the individuals are all of European descent, the impact of potential associations caused by population stratification have likely been reduced. Third, we used multiple independent genetic variants as a tool to reduce the impact of linkage disequilibrium on potential associations. Fourth, we selected multiple approaches for MR analysis and performed a comprehensive pleiotropy analysis to assess these. The potential association between genetic variation in PM2.5 levels with known risk of hypothyroidism warrants robust results.
This study also has some limitations. First, the TSMR analysis is based on European ancestry, and this relationship may change in individuals of other ancestries. Hence, TSMR analysis should also be performed in individuals of at least one other ancestry. Second, we only used summary statistics for MR analysis, and can only make a preliminary judgment on the causal relationship between PM2.5 and hypothyroidism. The specific mechanism of how PM2.5 increases the risk of hypothyroidism still needs further research.
Conclusion
We have provided genetic evidence that high PM2.5 concentrations can increase the risk of hypothyroidism. Our findings may have public health implications to raise awareness of the extent to which air quality is associated with the risk of hypothyroidism. This may provide guidance for the prevention and treatment of hypothyroidism.
Data availability statement
Publicly available datasets were analyzed in this study. This data can be found here: https://gwas.mrcieu.ac.uk/datasets/.
Ethics statement
This article contains human participants collected by several studies to report the large-scale GWAS for PM2.5 and for hypothyroidism. All participants gave informed consent in all the corresponding original studies, as described in the Materials and methods.
Author contributions
YZ and YueW: designing the study. SL and YueW: carrying out the study, analyzing the data, and writing the article. YunW: revising the article. All authors read and approved the final manuscript.
Acknowledgments
We thank the IEU Open GWAS project (https://gwas.mrcieu.ac.uk/datasets/) for providing summary results data for the analyses.
Conflict of interest
The authors declare that the research was conducted in the absence of any commercial or financial relationships that could be construed as a potential conflict of interest.
Publisher's note
All claims expressed in this article are solely those of the authors and do not necessarily represent those of their affiliated organizations, or those of the publisher, the editors and the reviewers. Any product that may be evaluated in this article, or claim that may be made by its manufacturer, is not guaranteed or endorsed by the publisher.
Supplementary material
The Supplementary Material for this article can be found online at: https://www.frontiersin.org/articles/10.3389/fpubh.2022.1000103/full#supplementary-material
References
1. Taylor PN, Albrecht D, Scholz A, Gutierrez-Buey G, Lazarus JH, Dayan CM, et al. Global epidemiology of hyperthyroidism and hypothyroidism. Nat Rev Endocrinol. (2018) 14:301–16. doi: 10.1038/nrendo.2018.18
2. Chaker L, Bianco AC, Jonklaas J, Peeters RP. Hypothyroidism. Lancet. (2017) 390:1550–62. doi: 10.1016/S0140-6736(17)30703-1
3. Kostoglou-Athanassiou I, Ntalles K. Hypothyroidism - new aspects of an old disease. Hippokratia. (2010) 14:82–7.
4. Asvold BO, Vatten LJ, Bjøro T. Changes in the prevalence of hypothyroidism: the HUNT Study in Norway. Eur J Endocrinol. (2013) 169:613–20. doi: 10.1530/EJE-13-0459
5. Hallgren S, Sinjari T, Håkansson H, Darnerud PO. Effects of polybrominated diphenyl ethers (PBDEs) and polychlorinated biphenyls (PCBs) on thyroid hormone and vitamin A levels in rats and mice. Arch Toxicol. (2001) 75:200–8. doi: 10.1007/s002040000208
6. Howe CG, Eckel SP, Habre R, Girguis MS, Gao L, Lurmann FW, et al. Association of prenatal exposure to ambient and traffic-related air pollution with newborn thyroid function: findings from the children's health study. JAMA Netw Open. (2018) 1:e182172. doi: 10.1001/jamanetworkopen.2018.2172
7. Richardson VM, Staskal DF, Ross DG, Diliberto JJ, DeVito MJ, Birnbaum LS. Possible mechanisms of thyroid hormone disruption in mice by BDE 47, a major polybrominated diphenyl ether congener. Toxicol Appl Pharmacol. (2008) 226:244–50. doi: 10.1016/j.taap.2007.09.015
8. Shang L, Huang L, Yang W, Qi C, Yang L, Xin J, et al. Maternal exposure to PM(25) may increase the risk of congenital hypothyroidism in the offspring: a national database based study in China. BMC Public Health. (2019) 19:1412. doi: 10.1186/s12889-019-7790-1
9. Kim HJ, Kwon H, Yun JM, Cho B, Park JH. Association between exposure to ambient air pollution and thyroid function in Korean adults. J Clin Endocrinol Metab. (2020) 105:e2912–20. doi: 10.1210/clinem/dgaa338
10. Alink GM, Brouwer A, Heussen GA. Effects of outdoor and indoor airborne particulate matter on thyroid hormone and vitamin A metabolism. Toxicol Lett. (1994) 72:73–81. doi: 10.1016/0378-4274(94)90012-4
11. Dutta SK, Mitra PS, Ghosh S, Zang S, Sonneborn D, Hertz-Picciotto I, et al. Differential gene expression and a functional analysis of PCB-exposed children: understanding disease and disorder development. Environ Int. (2012) 40:143–54. doi: 10.1016/j.envint.2011.07.008
12. McHale CM, Zhang L, Lan, Vermeulen R, Li G, Hubbard AE, et al. Global gene expression profiling of a population exposed to a range of benzene levels. Environ Health Perspect. (2011) 119:628–34. doi: 10.1289/ehp.1002546
13. Zhao N, Wu W, Feng Y, Yang F, Han T, Guo M, et al. Polymorphisms in oxidative stress, metabolic detoxification, and immune function genes, maternal exposure to ambient air pollution, and risk of preterm birth in Taiyuan, China. Environ Res. (2021) 194:110659. doi: 10.1016/j.envres.2020.110659
14. Poursafa P, Kelishadi R, Haghjooy-Javanmard S, Rafiei L, Keramatian K. Synergistic effects of genetic polymorphism and air pollution on markers of endothelial dysfunction in children. J Res Med Sci Official J Isfahan Univ Med Sci. (2012) 17:718–23. doi: 10.1186/1471-2458-11-115
15. Emdin CA, Khera AV, Kathiresan S. Mendelian randomization. JAMA. (2017) 318:1925–6. doi: 10.1001/jama.2017.17219
16. Reyna BA, Pickler RH. Patterns of genetic inheritance. Neonatal Netw. (1999) 18:7–10. doi: 10.1891/0730-0832.18.1.7
17. Liu X, Yuan J, Zhou H, Wang Y, Tian G, Liu X, et al. Association between systemic lupus erythematosus and primary hypothyroidism: evidence from complementary genetic methods. J Clin Endocrinol Metab. (2022). doi: 10.1210/clinem/dgac614
18. Lu L, Wan B, Li L, Sun M. Hypothyroidism has a protective causal association with hepatocellular carcinoma: a two-sample Mendelian randomization study. Front Endocrinol. 13:987401. doi: 10.3389/fendo.2022.987401
19. Zhao Y, Si S, Zhang K, Yuan J, Li J, Xue F. Causal relationship between type 1 diabetes and hypothyroidism: a Mendelian randomization study. Clin Endocrinol. (2022) 97:740–46. doi: 10.1111/cen.14801
20. Sudlow C, Gallacher J, Allen N, Beral V, Burton P, Danesh J, et al. UK biobank: an open access resource for identifying the causes of a wide range of complex diseases of middle and old age. PLoS Med. (2015) 12:e1001779. doi: 10.1371/journal.pmed.1001779
21. Bycroft C, Freeman C, Petkova D, Band G, Elliott LT, Sharp K, et al. The UK Biobank resource with deep phenotyping and genomic data. Nature. (2018) 562:203–9. doi: 10.1038/s41586-018-0579-z
22. Mitchell R, Hemani G, Dudding T, Corbin L, Harrison S, Paternoster L. UK Biobank Genetic Data: MRC-IEU Quality Control, version 2 - Datasets - data.bris. databris. (2018). doi: 10.5523/bris.1ovaau5sxunp2cv8rcy88688v
23. Eeftens M, Beelen R, de Hoogh K, Bellander T, Cesaroni G, Cirach M, et al. Development of Land Use Regression models for PM(25), PM(25) absorbance, PM(10) and PM(coarse) in 20 European study areas; results of the ESCAPE project. Environ Sci Technol. (2012) 46:11195–205. doi: 10.1021/es301948k
24. Skrivankova VW, Richmond RC, Woolf BAR, Davies NM, Swanson SA, VanderWeele TJ, et al. Strengthening the reporting of observational studies in epidemiology using Mendelian randomisation (STROBE-MR): explanation and elaboration. BMJ. (2021) 375:n2233. doi: 10.1136/bmj.n2233
25. Hemani G, Zheng J, Elsworth B, Wade KH, Haberland V, Baird D, et al. The MR-base platform supports systematic causal inference across the human phenome. Elife. (2018) 7:e34408. doi: 10.7554/eLife.34408
26. Bowden J, Davey Smith G, Burgess S. Mendelian randomization with invalid instruments: effect estimation and bias detection through Egger regression. Int J Epidemiol. (2015) 44:512–25. doi: 10.1093/ije/dyv080
27. Verbanck M, Chen CY, Neale B, Do R. Detection of widespread horizontal pleiotropy in causal relationships inferred from Mendelian randomization between complex traits and diseases. Nat Genet. (2018) 50:693–8. doi: 10.1038/s41588-018-0099-7
28. Greco MF, Minelli C, Sheehan NA, Thompson JR. Detecting pleiotropy in Mendelian randomisation studies with summary data and a continuous outcome. Stat Med. (2015) 34:2926–40. doi: 10.1002/sim.6522
29. Wang J, Zhuge J, Feng D, Zhang B, Xu J, Zhao D, et al. Mendelian randomization study of circulating lipids and biliary tract cancer among East Asians. BMC Cancer. (2022) 22:273. doi: 10.1186/s12885-022-09382-x
30. Bowden J, Del Greco MF, Minelli C, Davey Smith G, Sheehan N, Thompson J, et al. A framework for the investigation of pleiotropy in two-sample summary data Mendelian randomization. Stat Med. (2017) 36:1783–802. doi: 10.1002/sim.7221
31. Lee YH. Gout and the risk of Alzheimer's disease: a Mendelian randomization study. Int J Rheum Dis. (2019) 22:1046–51. doi: 10.1111/1756-185X.13548
32. Ren Z, Simons P, Wesselius A, Stehouwer CDA, Brouwers M. Relationship between non-alcoholic fatty liver disease and coronary artery disease: a Mendelian randomization study. Hepatology. (2022). doi: 10.1002/hep.32534. [Epub ahead of print].
33. Burgess S, Butterworth A, Thompson SG. Mendelian randomization analysis with multiple genetic variants using summarized data. Genet Epidemiol. (2013) 37:658–65. doi: 10.1002/gepi.21758
34. Li C, Ou R, Shang H. Rheumatoid arthritis decreases risk for Parkinson's disease: a Mendelian randomization study. NPJ Parkinsons Dis. (2021) 7:17. doi: 10.1038/s41531-021-00166-x
35. Lee YH, Song GG. Uric acid level, gout and bone mineral density: a Mendelian randomization study. Eur J Clin Invest. (2019) 49:e13156. doi: 10.1111/eci.13156
36. Li K, Feng T, Wang L, Chen Y, Zheng P, Pan P, et al. Causal associations of waist circumference and waist-to-hip ratio with type II diabetes mellitus: new evidence from Mendelian randomization. Mol Genet Genom. (2021) 296:605–13. doi: 10.1007/s00438-020-01752-z
37. Harari-Kremer R, Calderon-Margalit R, Korevaar TIM, Nevo D, Broday D, Kloog I, et al. Associations between prenatal exposure to air pollution and congenital hypothyroidism. Am J Epidemiol. (2021) 190:2630–8. doi: 10.1093/aje/kwab187
38. Ilias I, Kakoulidis I, Togias S, Stergiotis S, Michou A, Lekkou A, et al. Atmospheric pollution and thyroid function of pregnant women in Athens, Greece: a pilot study. Med Sci. (2020) 8:19. doi: 10.3390/medsci8020019
39. idism in Qingdao C. 2014-2017: a quantitative analysis. Ther Adv Endocrinol Metab. (2019) 10:2042018819892151.
40. Qi C, Shang L, Yang W, Huang L, Yang L, Xin J, et al. Maternal exposure to O and NO(2) may increase the risk of newborn congenital hypothyroidism: a national data-based analysis in China. Environ Sci Pollut Res Int. (2021) 28:34621–9. doi: 10.1007/s11356-021-13083-6
41. Emdin CA, Khera AV, Natarajan P, Klarin D, Zekavat SM, Hsiao AJ, et al. Genetic association of waist-to-hip ratio with cardiometabolic traits, type 2 diabetes, and coronary heart disease. JAMA. (2017) 317:626–34. doi: 10.1001/jama.2016.21042
42. Ghassabian A, Pierotti L, Basterrechea M, Chatzi L, Estarlich M, Fernández-Somoano A, et al. Association of exposure to ambient air pollution with thyroid function during pregnancy. JAMA Netw Open. (2019) 2:e1912902. doi: 10.1001/jamanetworkopen.2019.12902
43. Wang X, Liu C, Zhang M, Han Y, Aase H, Villanger GD, et al. Evaluation of maternal exposure to PM(25) and its components on maternal and neonatal thyroid function and birth weight: a cohort study. Thyroid. (2019) 29:1147–57. doi: 10.1089/thy.2018.0780
44. Li J, Liao J, Hu C, Bao S, Mahai G, Cao Z, et al. Preconceptional and the first trimester exposure to PM(25) and offspring neurodevelopment at 24 months of age: Examining mediation by maternal thyroid hormones in a birth cohort study. Environ Pollut. (2021) 284:117133. doi: 10.1016/j.envpol.2021.117133
45. Zhao Y, Cao Z, Li H, Su X, Yang Y, Liu C, et al. Air pollution exposure in association with maternal thyroid function during early pregnancy. J Hazard Mater. (2019) 367:188–93. doi: 10.1016/j.jhazmat.2018.12.078
46. Chan YL, Wang B, Chen H, Ho KF, Cao J, Hai G, et al. Pulmonary inflammation induced by low-dose particulate matter exposure in mice. Am J Physiol Lung Cell Mol Physiol. (2019) 317:L424–l430. doi: 10.1152/ajplung.00232.2019
47. Xu MX, Ge CX, Qin YT, Gu TT, Lou DS Li Q, Hu LF, et al. Prolonged PM25 exposure elevates risk of oxidative stress-driven non-alcoholic fatty liver disease by triggering increase of dyslipidemia. Free Rad Biol Med. (2019) 130:542–56. doi: 10.1016/j.freeradbiomed.2018.11.016
48. Wang Y, Xiong L, Tang M. Toxicity of inhaled particulate matter on the central nervous system: neuroinflammation, neuropsychological effects and neurodegenerative disease. J Appl Toxicol JAT. (2017) 37:644–67. doi: 10.1002/jat.3451
49. Gangwar RS, Bevan GH, Palanivel R, Das L, Rajagopalan S. Oxidative stress pathways of air pollution mediated toxicity: recent insights. Redox Biol. (2020) 34:101545. doi: 10.1016/j.redox.2020.101545
50. Reyes-Caballero H, Rao X, Sun Q, Warmoes MO, Lin P, Sussan TE, et al. Author correction: air pollution-derived particulate matter dysregulates hepatic Krebs cycle, glucose and lipid metabolism in mice. Sci Rep. (2020) 10:5082. doi: 10.1038/s41598-020-60083-6
51. Gogna P, King WD, Villeneuve PJ, Kumarathasan P, Johnson M, Lanphear B, et al. Ambient air pollution and inflammatory effects in a Canadian pregnancy cohort. Environ Epidemiol. (2021) 5:e168. doi: 10.1097/EE9.0000000000000168
52. Hong Z, Zeng P, Zhuang G, Guo Q, Cai C. Toxicological effects of artificial fine particulate matter in rats through induction of oxidative stress and inflammation. Tohoku J Exp Med. (2021) 255:19–25. doi: 10.1620/tjem.255.19
53. Das D, Banerjee A, Jena AB, Duttaroy AK, Pathak S. Essentiality, relevance, and efficacy of adjuvant/combinational therapy in the management of thyroid dysfunctions. Biomed Pharmacother. (2022) 146:112613. doi: 10.1016/j.biopha.2022.112613
54. Lacka K, Maciejewski A. Rare thyroid non-neoplastic diseases. Thyroid Res. (2015) 8:5. doi: 10.1186/s13044-015-0017-3
55. Dong X, Wu W, Yao S, Li H, Li Z, Zhang L, et al. PM(25) disrupts thyroid hormone homeostasis through activation of the hypothalamic-pituitary-thyroid (HPT) axis and induction of hepatic transthyretin in female rats 25. Ecotoxicol Environ Saf. (2021) 208:111720. doi: 10.1016/j.ecoenv.2020.111720
Keywords: PM2.5, hypothyroidism, Mendelian randomization, air pollution, GWAS
Citation: Zhang Y, Liu S, Wang Y and Wang Y (2022) Causal relationship between particulate matter 2.5 and hypothyroidism: A two-sample Mendelian randomization study. Front. Public Health 10:1000103. doi: 10.3389/fpubh.2022.1000103
Received: 06 September 2022; Accepted: 14 November 2022;
Published: 25 November 2022.
Edited by:
Tongjian Cai, Army Medical University, ChinaReviewed by:
Zhijing Lin, Anhui Medical University, ChinaDirga Kumar Lamichhane, Inha University, South Korea
Copyright © 2022 Zhang, Liu, Wang and Wang. This is an open-access article distributed under the terms of the Creative Commons Attribution License (CC BY). The use, distribution or reproduction in other forums is permitted, provided the original author(s) and the copyright owner(s) are credited and that the original publication in this journal is cited, in accordance with accepted academic practice. No use, distribution or reproduction is permitted which does not comply with these terms.
*Correspondence: Yue Wang, eXVld2FuZ0BjbXUuZWR1LmNu