- 1Department of Orthopedics, Second Hospital of Jilin University, Changchun, China
- 2Department of Endocrinology, Baoji City Hospital of Traditional Chinese Medicine, Baoji, China
Background: This study aimed to develop and validate a nomogram for predicting mortality in patients with thoracic fractures without neurological compromise and hospitalized in the intensive care unit.
Methods: A total of 298 patients from the Medical Information Mart for Intensive Care III (MIMIC-III) database were included in the study, and 35 clinical indicators were collected within 24 h of patient admission. Risk factors were identified using the least absolute shrinkage and selection operator (LASSO) regression. A multivariate logistic regression model was established, and a nomogram was constructed. Internal validation was performed by the 1,000 bootstrap samples; a receiver operating curve (ROC) was plotted, and the area under the curve (AUC), sensitivity, and specificity were calculated. In addition, the calibration of our model was evaluated by the calibration curve and Hosmer-Lemeshow goodness-of-fit test (HL test). A decision curve analysis (DCA) was performed, and the nomogram was compared with scoring systems commonly used during clinical practice to assess the net clinical benefit.
Results: Indicators included in the nomogram were age, OASIS score, SAPS II score, respiratory rate, partial thromboplastin time (PTT), cardiac arrhythmias, and fluid-electrolyte disorders. The results showed that our model yielded satisfied diagnostic performance with an AUC value of 0.902 and 0.883 using the training set and on internal validation. The calibration curve and the Hosmer-Lemeshow goodness-of-fit (HL). The HL tests exhibited satisfactory concordance between predicted and actual outcomes (P = 0.648). The DCA showed a superior net clinical benefit of our model over previously reported scoring systems.
Conclusion: In summary, we explored the incidence of mortality during the ICU stay of thoracic fracture patients without neurological compromise and developed a prediction model that facilitates clinical decision making. However, external validation will be needed in the future.
Background
A spinal fracture is a dislocation or fracture of the vertebrae with an annual incidence of 26 per 100 000 (1), accounting for ~23.3% of all trauma patients (2). The most prevalent spinal fractures occur at the level of the lumber spine, followed by the thoracic spine (3). Motor vehicle accidents (MVC) and falls from heights are the most common causes of spinal fracture; the annual incidence of spinal fracture has increased, with the reported increase in motor vehicle crashes (4). In China, the incidence rate of spinal fractures in 2007 was twice that in 2001 (3).
Numerous studies have investigated the long-term prognosis of spinal fractures using data from large patient populations in recent years. In patients with thoracolumbar fractures exhibiting mild symptoms, the visual analog scale (VAS) scores and the Roland Morris Disability Questionnaire demonstrated poor results and a poor outcome in 6% of patients 10 years after non-operative treatment (5). Furthermore, for patients exhibiting more severe symptoms requiring surgery, only 50% reportedly return to their original jobs postoperatively (6).
Meanwhile, long-term mortality associated with spinal fractures is significantly increased in post-traumatic patients compared to those with no history of trauma (7), especially in osteoporotic patients (8, 9). It has also been shown that old age and male gender increase the long-term mortality risk after thoracolumbar fracture (10). Interestingly, studies have shown that trauma patients in the ICU have high mortality rates, as high as 31% (11). Notwithstanding that substantial progress has been made in trauma advanced life support over the years, the mortality rates are still high (12). Patients with concomitant spinal cord injury are more likely than those without neurological damage to present with multiorgan injury and die from secondary infection (13). Most importantly, many patients experience death prior to hospitalization due to severe spinal cord injuries (14). To the best of our knowledge, the prognosis of acute severe spine fracture patients in ICU has been largely unexplored, and current research hot spots still focus on osteoporosis, bone cement, biomechanical analysis, and so on. Meanwhile, the interest of intensivists in trauma patients has mostly focused on rib fractures and pelvic fractures (15–17). Due to the lack of adequate attention to such patients, a large number of high-risk patients are not identified in time for admission, which often leads to poor prognosis and even higher mortality. Little is currently known about the risk factors of poor prognosis in ICU patients with thoracic spine fractures, which has resulted in high mortality rates. Accordingly, we selected this specific patient population for our study.
It is essential to develop a prediction model based on routine clinical and laboratory parameters to ensure that it can be easily implemented during clinical practice. It has been established that nomograms can provide evidence-based and personalized risk estimates and contribute to clinical management and prognosis evaluation (18–21). This study aimed to develop a prediction model to predict mortality during the ICU stay of thoracic fracture patients without neurological compromise based on the Medical Information Mart for Intensive Care III (MIMIC-III) (22) clinical database.
Materials and Methods
Data Source
Our data were derived from the MIMIC-III database, a database established and open source by the Massachusetts Institute of Technology (MA, USA) containing information on more than 58,000 patients that attended the Beth Israel Deaconess Medical Center. We completed a web course offered by the National Institutes of Health (NIH) and were granted access to the MIMIC-III database (certification number: 42442549). The data were extracted from the MIMIC-III database using structure query language (SQL) with pgAdmin4 PostgreSQL 9.6.
Study Population
Structured Query Language (SQL) with PostgreSQL (version 9.6 University of California, Berkeley) was used to extract information associated with each patient's unique HADM_ID from the MIMIC-III database. Through International Classification of Diseases 9th Edition (ICD-9) code = 8,052, we obtaining 381 patient. For patients with multiple admissions, we retained information only on the patient's first admission to the ICU. Patients with missing data >20% for laboratory tests (n = 5) and ital signs (n = 1) were excluded. Finally, 298 patients were included in the study (Figure 1).
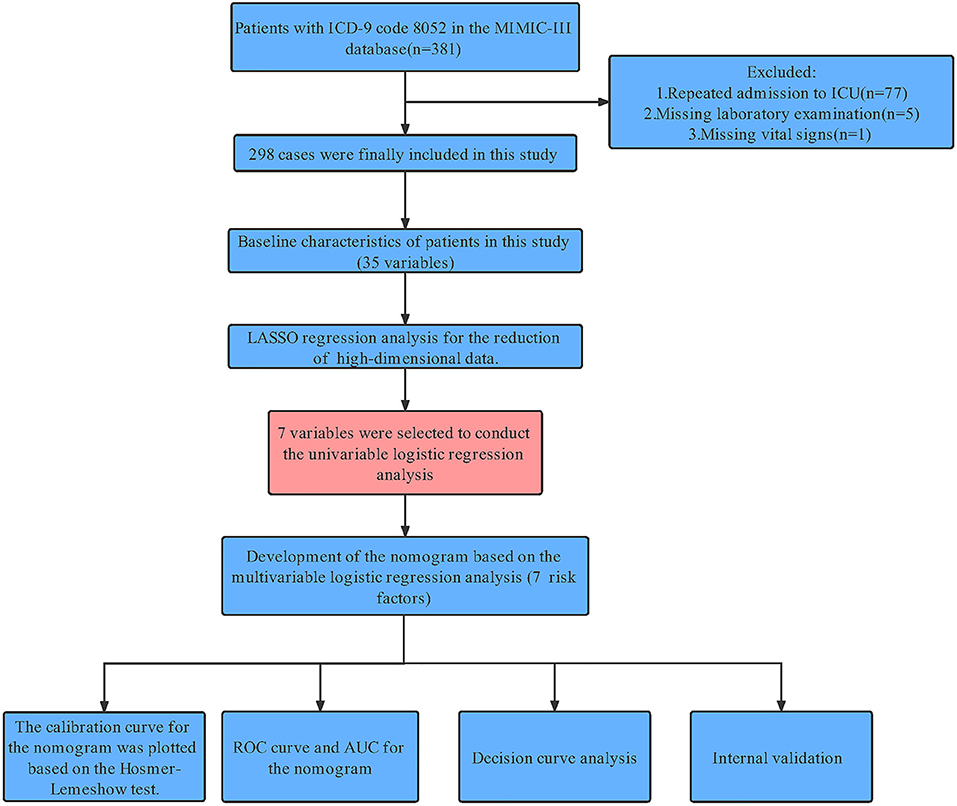
Figure 1. Workflow of the study. MIMIC-III, Medical Information Mart for Intensive Care III; ICU, intensive care unit; LASSO, least absolute shrinkage and selection operator; AUC, area under the curve; HL test, Hosmer-Lemeshow goodness-of-fit test; DCA, decision curve analysis.
Clinical Variables and Definition
Using the patient's HADM_ID and ICUSTAY_ID as described above, we extracted the following data: demographics, vital signs, laboratory tests, comorbidities, and scoring systems. Among these, demographics included age and gender; vital signs included heart rate (HR), systolic blood pressure (SBP), diastolic blood pressure (DBP), mean blood pressure (MBP), respiratory rate (RR), temperature, SpO2, bicarbonate; laboratory tests included blood urea nitrogen (BUN), chloride, creatinine, glucose, hemoglobin, potassium, platelet, partial thromboplastin time (PTT), prothrombin time (PT), sodium, white blood cell (WBC), red blood cell (RBC); comorbidities included congestive heart failure, cardiac arrhythmias, liver disease, coagulopathy, fluid-electrolyte disorders, hypertension, renal failure, obesity, chronic pulmonary; scoring systems include scoring systems included simplified acute physiology score II (SAPS II), sequential organ failure as severity (sofa) score, oxford acute severity of illness score (OASIS), and the Glasgow coma scale (GCS). Indicators with >20% missing data were removed, such as height, weight, calcium; A deletion was also made for some complications that were not present in all patients, such as peptic ulcer, lymphoma, metastatic cancer. All the variables mentioned above were collected within 24 h of patients' admission.
Statistical Analysis
We used the median and interquartile range to express continuous variables, while Wilcoxon's rank-sum test was selected for comparison between two groups; The categorical variables were expressed as frequency and proportion. Chi-square tests or Fisher's exact test was chosen for inter-group comparison according to the situation. We selected the bootstrap method for internal validation based on the number of patients, in accordance with the transparent reporting of a multivariable prediction model for individual prognosis or diagnosis (TRIPOD) guidelines (23). We used the neighbor interpolation method in the MICE R package (24) to fill in missing data. Then, the least absolute shrinkage and selection operator (LASSO) expression was used for screening predictors of mortality (25). For the cross-validation results, we selected lambda = min to determine the final candidate variables (26, 27). The multivariate logistic regression model was established using these variables, and multicollinearity was evaluated by variable inflation factors (VIF). The area under the receiver operating curve (AUC), sensitivity and specificity were used to evaluate the model's performance. The Youden index determined the best cutoff point. Finally, the nomogram was plotted using the R package “regplot.” The calibration C index (bootstrap resampling 1,000 times) (28), the calibration curve (relationship between observation probability and prediction probability), Hosmer-Lemeshow goodness of fit test (HL test), and brier score were used to evaluate the degree of consistency between observed and predicted outcomes. Decision curve analysis (DCA) was used to assess the net clinical benefit (29). All statistical analyses were completed using R language (version 3.6.3); a p < 0.05 was statistically significant.
Results
Patient Characteristics
Two hundred and ninety eight patients were finally included in the study, with an average age of 53.5 years. 34.6% (n = 103) were female, with a mortality rate of 13.6% while male patients accounted for 65.4% (n = 195), with a mortality rate of 7.2%. Table 1 compares the differences in characteristics between the in-hospital death group and the survival group. Compared with the survival group, patients in the death group were older, had lower body temperature and blood pressure, faster respiratory rate, and lower bicarbonate. Meanwhile, lower hemoglobin and platelet counts were observed in the death group, while PT and PTT values were relatively higher, indicating poor coagulation function. The OASIS, SOFA, and SAPS II scores were significantly higher, with a higher prevalence of cardiac arrhythmias, coagulopathy, and fluid-electrolyte disorders.
Characteristics Selection and Development of a Nomogram
Out of 35 variables, seven remained in the lasso logistic regression model based on the binomial deviance minimum criteria (ratio 5:1) (Figure 2). The final seven variables included in the multivariate logistic regression, were: age (OR: 1.02; 95% CI 0.99–1.06), OASIS score (OR: 1.08; 95%CI 1.00–1.17), SAPS II score (OR: 1.03; 95% CI 0.98–1.08), RR (OR: 1.07; 95% CI 0.94–1.20), PTT (OR: 1.08; 95%CI 1.03–1.13), cardiac arrhythmia (OR: 1.44; 95%CI 0.43–4.62), and fluid-electrolyte disorders (OR: 4.40; 95% CI 1.62–12.54) (Table 2). Based on this model, we constructed a nomogram to predict mortality in ICU patients with thoracic spine fractures without neurological injuries (Figure 3).
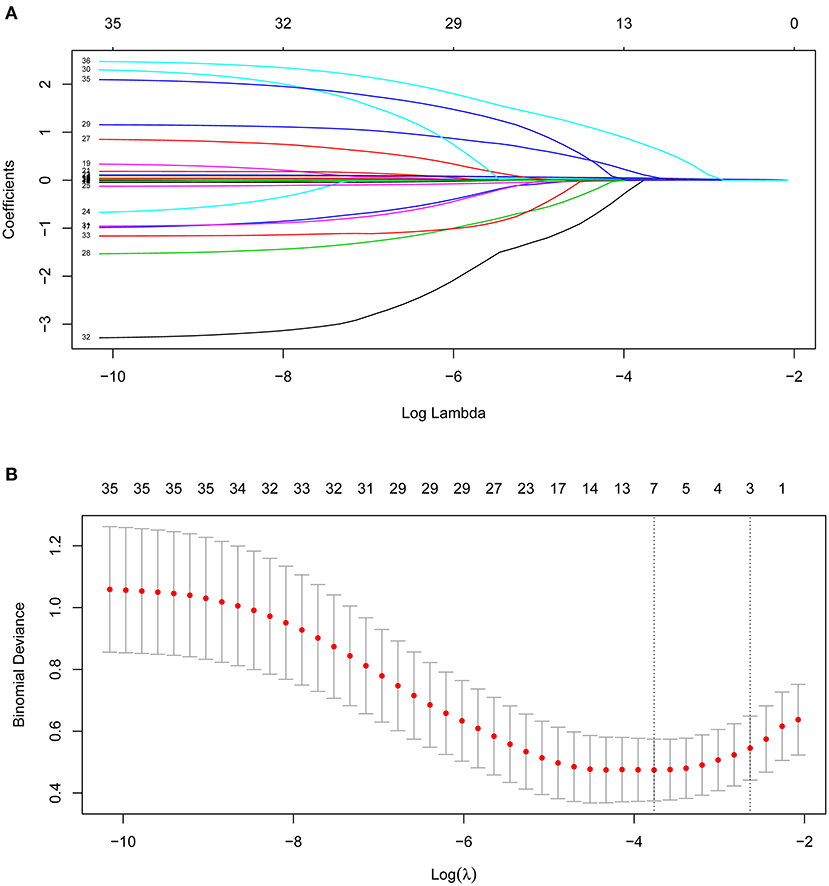
Figure 2. Clinical variables were selected using the lasso logistic regression model. (A) Tuning parameter (λ) selection using LASSO penalized logistic regression with 10-fold cross-validation. (B) LASSO coefficient profiles of the radiomic features.
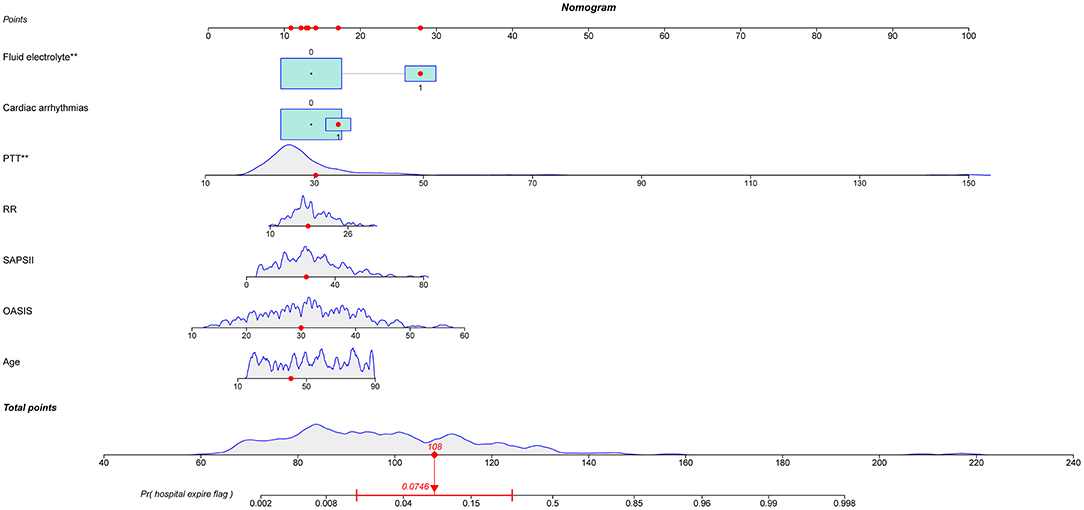
Figure 3. Nomogram to predict the risk of in-hospital mortality in patients with thoracic spine fractures without neurological injury in the ICU. PTT, partial thromboplastin time; RR, respiratory rate; SAPS II, simplified acute physiology score II; OASIS, oxford acute severity of illness score. ** means p < 0.01.
Apparent Performance of the Nomogram and Web Calculator
Our model yielded an AUC value of 0.902 (95% CI 0.849–0.959), with a C-index of 0.883 after 1,000 bootstrap resampling internal validations. According to the Youden index, the optimal cutoff value was 18.45%, with a sensitivity and specificity of 0.870 and 0.786, respectively (Figure 4). Meanwhile, the calibration curve showed a good fit during internal validation (Figure 5), while the HL test showed that our predicted and observed values were close (P = 0.648); The Brier score was 0.0543 and 0.0623 after bootstrap correction. The ROC values of the scoring systems SAPS II and OASIS incorporated into the regression were 0.856 and 0.837, respectively, suggesting that our model exhibited better predictive performance than scoring systems commonly used clinically. To facilitate clinical use, we constructed a web calculator (https://ouyyjlueducn.shinyapps.io/dynnomapp/) based on the model.
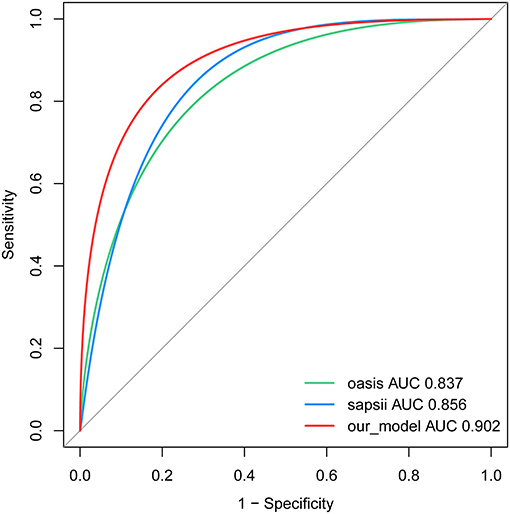
Figure 4. Receiver operating characteristic curve of the nomogram. AUC, area under curve; OASIS, oxford acute severity of illness score; SAPS II, simplified acute physiology score II.
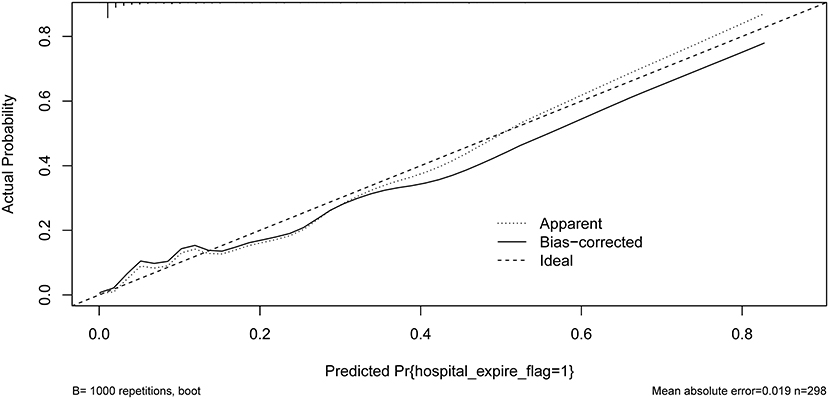
Figure 5. Calibration curves of the predicted nomogram. The dashed line represents the original performance, and the solid dashed line represents the performance during internal validation by bootstrapping (B = 1,000 repetitions). Results of the Hosmer-Lemeshow test demonstrate that the P-value was 0.648.
Clinical Practice
DCA of the nomogram was performed (Figure 6). The blue curve in the figure represents that all the patients received intervention, the straight purple line represents that none patients receive the intervention, and the red curve represents the clinical benefit of our model. For our model, when the predicted probability threshold was set to 0.065%, the net clinical benefit was 9.39%. Our results showed that our model had a superior net clinical benefit than the OASIS and SAPS II scoring systems.
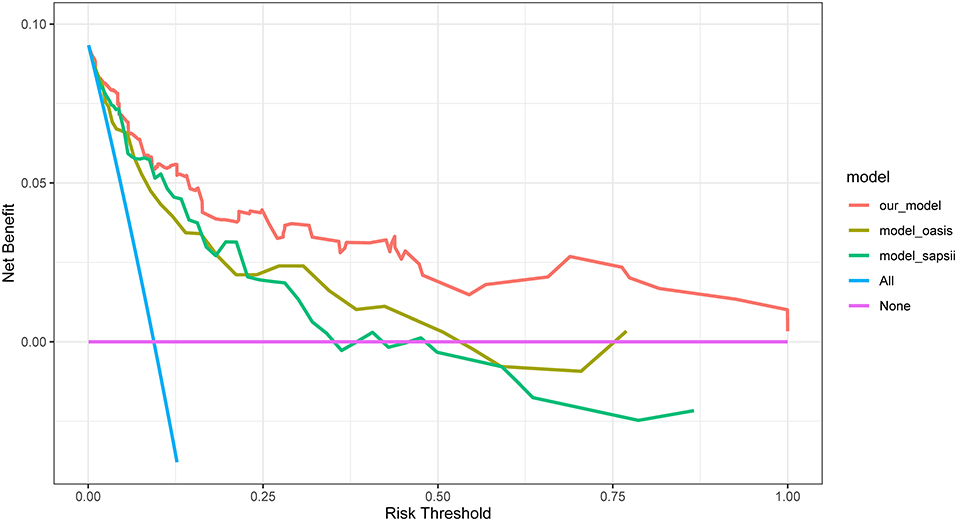
Figure 6. DCA for our model, OASIS and SAPS II. The y-axis measures the net benefit. DCA, decision curve analysis; OASIS, oxford acute severity of illness score; SAPS II, simplified acute physiology score II.
Discussion
Fractures have become a major public health problem in most countries (30), especially spine fractures, which account for approximately 46% of spinal injuries in severe trauma (31, 32). In this regard, patients that sustain spine fractures have the poorest functional prognosis and potential to return to work (33). Although many studies have discussed the long-term prognosis of spinal fractures, to the best of our knowledge, this is the first study to investigate the mortality risk of spinal fracture patients without neurological impairment. The injury severity score (ISS) has previously been used to guide the risk stratification of trauma patients; however, some studies have pointed out that this scoring system was not reliable (34) since it did not consider the characteristics of patients with spinal trauma. We summarized the related works in Table 3. Previous methods of classifying spinal trauma have been most relevant in guiding surgical treatment (35), and commonly include the Trauma and Injury Severity Score (ISS) and the New Trauma and Injury Severity Score (NISS). However, none of these assessment methods take into account the individual spinal trauma patient, and our work fills a gap in the risk stratification of patients with severe spinal trauma. After internal validation of the model, we found that the predictions of the model were in good agreement with the actual results. More interestingly, the constructed model had better discrimination and net clinical benefit compared to the previously used OASIS and SAPSII systems. Also, based on the reviewers' comments, we have done more work. To facilitate clinical use, we constructed a web calculator (https://ouyyjlueducn.shinyapps.io/dynnomapp/) based on the model. In summary, we provide an easy-to-use model for this group of patients, which can identify high-risk patients early, take appropriate interventions early, and reduce poor prognosis and in-hospital mortality.
With the rapid development of computer technology in recent years, the combination of machine learning technology and medical practice has become a major trend (19–21, 26, 27). Along with the continuous innovation of convolutional algorithms, from LeNet by Lecun et al. (36) to ResNet by He et al. (37), computer-aided decision making through imaging has become a hot topic in medical research, such as prediction of BMI by facial image features to predict BMI (38) and fundus images to predict diabetic retinopathy (39). In addition, the prevalence of electronic medical records and the establishment of large medical databases have also provided the basis for research on clinical problems, and the combination with machine learning has shown remarkable performance in predicting the occurrence and prognosis of diseases (40, 41). Unfortunately, however, there is still a lack of research in the current field for our patient population. It is therefore necessary to develop an easy-to-use and reliable tool to inform clinical practice. In the present study, we established a nomogram consisting of seven predictors: age, OASIS score, SAPS II score, RR, PTT, cardiac arrhythmias, fluid-electrolyte disorders. Multiple indicators used during the validation process, including AUC, calibration curve, HL test and DCA, indicated that our nomogram yielded satisfactory prediction results.
As seen in Table 1, significant differences in coagulation markers PT, PTT, and coagulopathy were found between the death and the survival groups, especially PTT, which was an important predictor of mortality. Trauma-induced coagulopathy (TIC) has been reported to play an important role in trauma healing (42), and an increasing body of evidence suggests that uncontrolled hemorrhage is a preventable cause of death in the early stages after injury (43–45), which has been reported to be as high as 40–80% (46). The incidence rate of TIC often correlates with the severity of tissue injury (47) since tissue injury releases a large number of damage-associated molecular patterns (DAMPs), which promote multiple inflammatory pathways and thereby affect coagulation (42). Endothelial dysfunction has been reported to participate in TIC (48), and plasma samples from severely injured patients on admission exhibited increased levels of syndecan-1, which correlated with increased activated protein C (APC), prolonged PTT, and elevated epinephrine levels (49). Furthermore, platelet defects and dysfunction are also important contributors to TIC (50). In our study, even though the platelet count in the deceased group was still within the normal range, a significant decrease was observed (Table 2), suggesting an association between a relatively low platelet count and increased mortality, consistent with previous studies (51, 52). Similarly, a prospective study demonstrated that 91% of patients with severe injuries had platelet dysfunction despite normal platelet counts (53).
The most common arrhythmia in patients with chest trauma or surgery is atrial fibrillation, often associated with longer ICU stay and higher mortality (54). On the one hand, most patients with pre-existing atrial fibrillation receive anticoagulant therapy. When combined with previously described coagulation dysfunction, fatal bleeding can result from small traumas (55). On the other hand, patients with new-onset atrial fibrillation have higher in-hospital mortality than patients with previous atrial fibrillation (56) reportedly. The mortality among patients with arrhythmia in our study group was 23.3% (n = 10/43), much higher than that of patients without arrhythmia. Moreover, a significant difference in the number of patients with arrhythmia was found between the survival and death groups (P < 0.01).
It is widely acknowledged that the onset of arrhythmias is highly correlated with electrolyte disturbances (54). Many studies have demonstrated that electrolyte disturbances and vertebral fractures are associated with higher mortality, while elderly patients with fractures are more likely to have hyponatremia (57–59). Interestingly, in our study, albeit patients in the deceased group were much older than those in the survival group, no significant difference in sodium levels was found between the two groups. However, our study also confirmed higher mortality in patients who develop fluid-electrolyte disorders, emphasizing the need for early and effective fluid management in this subset of patients (60).
An analysis of nationwide patients with vertebral fractures in Japan revealed that advanced age is a significant risk factor for complications (OR 1.38) (61). We consistently found that advanced age patients were more likely to experience in-hospital death, which may be associated with sarcopenia (62), poor nutritional status (63), and development of fluid-electrolyte disorders, as mentioned earlier. However, we found that gender was not a relevant factor for in-hospital death in our study, which was inconsistent with the literature. This discrepancy could be accounted for by the fact that the subjects of our research were ICU patients, which were critically ill, while gender had more influence in long-term prognosis (10).
Due to its simplicity and ease of observation, the respiratory rate is one of the indicators traditionally used for the early identification of high-risk patients after trauma (64). A respiratory rate >20 was an important indicator in new evaluation criteria for trauma patients (65). Consistently, we found that tachypneic patients with a respiratory rate within 24 h of admission are more likely to experience in-hospital mortality and require early intervention. Two severity scoring systems, SAPS II and OASIS were also included in the prediction model of this study. At present, much controversy surrounds the predictive accuracy of these two scoring systems in orthopedic trauma patients (66, 67). In our present study, both scoring systems were integrated into our nomogram. The ROC plots showed that our model outperformed these two scoring systems in discrimination (Figure 3). At the same time, DCA exhibited greater net clinical benefit than these two scoring systems (Figure 5).
There are still limitations that need to be considered. First, these data were from a public database spanning 2007–2014. Therefore, the model needs external validation from different medical Institutions. Second, because missing data is >20% in the dataset, there is a lack of some important clinical parameters. Finally, although nomogram is already widely used in clinical practice to aid medical decision making, we want to further simplify the work and expand the scenarios in which it can be used. Therefore, in the future we hope to package predictive tools into applications for mobile devices, wearables, or personal computers.
Conclusion
Our study found that age, OASIS score, SAPS II score, RR, PTT, cardiac arrhythmias, and fluid-electrolyte disorders are predictors of mortality during the ICU stay of thoracic fracture patients without neurological compromise. A multiple logistic regression model and a nomogram were developed and validated. During clinical practice, this nomogram could help physicians screen high-risk patients, make optimal use of resources, and decrease the occurrence of death in this patient population.
Data Availability Statement
The raw data supporting the conclusions of this article will be made available by the authors, without undue reservation.
Author Contributions
HW collected the data, analyzed the data, and drafted the manuscript. TF, YO, MK, JZ, and RD conceived of the study, participated in its design and coordination, and helped to draft the manuscript. YQ was responsible for the whole project, reviewed the manuscript, designed the study, and supervised the study. All authors contributed to the article and approved the submitted version.
Funding
This work was supported by Norman Bethune Program of Jilin University (JJKH20201101KJ).
Conflict of Interest
The authors declare that the research was conducted in the absence of any commercial or financial relationships that could be construed as a potential conflict of interest.
Publisher's Note
All claims expressed in this article are solely those of the authors and do not necessarily represent those of their affiliated organizations, or those of the publisher, the editors and the reviewers. Any product that may be evaluated in this article, or claim that may be made by its manufacturer, is not guaranteed or endorsed by the publisher.
Supplementary Material
The Supplementary Material for this article can be found online at: https://www.frontiersin.org/articles/10.3389/fpubh.2021.818439/full#supplementary-material
References
1. Niemi-Nikkola V, Saijets N, Ylipoussu H, Kinnunen P, Pesälä J, Mäkelä P, Alen M, Kallinen M, Vainionpää A. Traumatic spinal injuries in northern Finland. Spine. (2018) 43:e45–e51. doi: 10.1097/BRS.0000000000002214
2. Pirouzmand F. Epidemiological trends of spine and spinal cord injuries in the largest Canadian adult trauma center from 1986 to 2006. J Neurosurg Spine. (2010) 12:131–40. doi: 10.3171/2009.9.SPINE0943
3. Liu P, Yao Y, Liu MY, Fan WL, Chao R, Wang ZG, Liu YC, Zhou JH, Zhao JH. Spinal trauma in mainland China from 2001 to 2007: an epidemiological study based on a nationwide database. Spine. (2012) 37:1310–5. doi: 10.1097/BRS.0b013e3182474d8b
4. Doud AN, Weaver AA, Talton JW, Barnard RT, Meredith JW, Stitzel JD, et al. Has the incidence of thoracolumbar spine injuries increased in the United States from 1998 to 2011? Clin Orthop Relat Res. (2015) 473:297–304. doi: 10.1007/s11999-014-3870-9
5. Post RB, van der Sluis CK, Leferink VJ, Dijkstra PU, ten Duis HJ. Nonoperatively treated type A spinal fractures: mid-term versus long-term functional outcome. Int Orthop. (2009) 33:1055–60. doi: 10.1007/s00264-008-0593-0
6. Defino HL, Canto FR. Low thoracic and lumbar burst fractures: radiographic and functional outcomes. Eur Spine J. (2007) 16:1934–43. doi: 10.1007/s00586-007-0406-y
7. Davidson GH, Hamlat CA, Rivara FP, Koepsell TD, Jurkovich GJ, Arbabi S. Long-term survival of adult trauma patients. Jama. (2011) 305:1001–7. doi: 10.1001/jama.2011.259
8. Bliuc D, Nguyen ND, Milch VE, Nguyen TV, Eisman JA, Center JR. Mortality risk associated with low-trauma osteoporotic fracture and subsequent fracture in men and women. Jama. (2009) 301:513–21. doi: 10.1001/jama.2009.50
9. Center JR, Nguyen TV, Schneider D, Sambrook PN, Eisman JA. Mortality after all major types of osteoporotic fracture in men and women: an observational study. Lancet. (1999) 353:878–82. doi: 10.1016/S0140-6736(98)09075-8
10. Niemi-Nikkola V, Saijets N, Ylipoussu H, Kinnunen P, Pesala J, Makela P, et al. Long-term posttraumatic survival of spinal fracture patients in northern Finland. Spine. (2018) 43:1657–63. doi: 10.1097/BRS.0000000000002687
11. Darbandsar Mazandarani P, Heydari K, Hatamabadi H, Kashani P, Jamali Danesh Y. Acute physiology and chronic health evaluation (APACHE) III score compared to trauma-injury severity score (TRISS) in predicting mortality of trauma patients. Emerg. (2016) 4:88–91.
12. van Breugel JMM, Niemeyer MJS, Houwert RM, Groenwold RHH, Leenen LPH, van Wessem KJP. Global changes in mortality rates in polytrauma patients admitted to the ICU-a systematic review. World J Emerg Surg. (2020) 15:55. doi: 10.1186/s13017-020-00330-3
13. Lalwani S, Punia P, Mathur P, Trikha V, Satyarthee G, Misra MC. Hospital acquired infections: preventable cause of mortality in spinal cord injury patients. J Lab Physicians. (2014) 6:36–9. doi: 10.4103/0974-2727.129089
14. Krassioukov AV, Furlan JC, Fehlings MG. Medical co-morbidities, secondary complications, and mortality in elderly with acute spinal cord injury. J Neurotrauma. (2003) 20:391–9. doi: 10.1089/089771503765172345
15. Ju J, Zhang P, Wang Y, Kou Y, Fu Z, Jiang B, et al. A clinical nomogram predicting unplanned intensive care unit admission after hip fracture surgery. Surgery. (2021) 170:291–7. doi: 10.1016/j.surg.2021.01.009
16. O'Connell KM, Quistberg DA, Tessler R, Robinson BRH, Cuschieri J, Maier RV, et al. Decreased risk of delirium with use of regional analgesia in geriatric trauma patients with multiple rib fractures. Ann Surg. (2018) 268:534–40. doi: 10.1097/SLA.0000000000002929
17. Tignanelli CJ, Rix A, Napolitano LM, Hemmila MR, Ma S, Kummerfeld E. Association between adherence to evidence-based practices for treatment of patients with traumatic rib fractures and mortality rates among US trauma centers. JAMA Netw Open. (2020) 3:e201316. doi: 10.1001/jamanetworkopen.2020.1316
18. Fan T, Wang H, Wang J, Wang W, Guan H, Zhang C. Nomogram to predict the risk of acute kidney injury in patients with diabetic ketoacidosis: an analysis of the MIMIC-III database. BMC Endocr Disord. (2021) 21:37. doi: 10.1186/s12902-021-00696-8
19. Tang Z, Chen Y, Ye S, Hu R, Wang H, He J, et al. Fully memristive spiking-neuron learning framework and its applications on pattern recognition and edge detection. Neurocomputing. (2020) 403:80–7. doi: 10.1016/j.neucom.2020.04.012
20. Tang Z, Zhu R, Hu R, Chen Y, Wu EQ, Wang H, et al. A multilayer neural network merging image preprocessing and pattern recognition by integrating diffusion and drift memristors. IEEE Trans Cognit Dev Syst. (2020). 13:645–56. doi: 10.1109/TCDS.2020.3003377
21. Wu EQ, Hu D, Deng P-Y, Tang Z, Cao Y, Zhang W-M, et al. Nonparametric bayesian prior inducing deep network for automatic detection of cognitive status. IEEE Trans Cybernetics. (2020) 51:5483–96. doi: 10.1109/TCYB.2020.2977267
22. Johnson AE, Pollard TJ, Shen L, Lehman LW, Feng M, Ghassemi M, et al. MIMIC-III, a freely accessible critical care database. Sci Data. (2016) 3:160035. doi: 10.1038/sdata.2016.35
23. Collins GS, Reitsma JB, Altman DG, Moons KG. Transparent reporting of a multivariable prediction model for individual prognosis or diagnosis (TRIPOD): the TRIPOD statement. Bmj. (2015) 350:g7594. doi: 10.1136/bmj.g7594
24. Beretta L, Santaniello A. Nearest neighbor imputation algorithms: a critical evaluation. BMC Med Inform Decis Mak. (2016) 16(Suppl 3):74. doi: 10.1186/s12911-016-0318-z
25. Friedman J, Hastie T, Tibshirani R. Regularization paths for generalized linear models via coordinate descent. J Stat Softw. (2010) 33:1–22. doi: 10.18637/jss.v033.i01
26. Hu R, Zhou S, Liu Y, Tang Z. Margin-based Pareto ensemble pruning: An ensemble pruning algorithm that learns to search optimized ensembles. Comput Intel Neurosci. (2019) 2019:7560872. doi: 10.1155/2019/7560872
27. Tang Z, Chen Y, Wang Z, Hu R, Wu EQ. Non-spike timing-dependent plasticity learning mechanism for memristive neural networks. Appl Intelligence. (2021) 51:3684–95. doi: 10.1007/s10489-020-01985-w
28. Harrell FE Jr, Califf RM, Pryor DB, Lee KL, Rosati RA. Evaluating the yield of medical tests. JAMA. (1982) 247:2543–6. doi: 10.1001/jama.247.18.2543
29. Vickers AJ, Cronin AM, Elkin EB, Gonen M. Extensions to decision curve analysis, a novel method for evaluating diagnostic tests, prediction models and molecular markers. BMC Med Inform Decis Mak. (2008) 8:53. doi: 10.1186/1472-6947-8-53
30. Court-Brown CM, McQueen MM. Global Forum: fractures in the elderly. J Bone Joint Surg Am. (2016) 98:e36. doi: 10.2106/JBJS.15.00793
31. Heyde CE, Ertel W, Kayser R. Management of spine injuries in polytraumatized patients. Orthopade. (2005) 34:889–905. doi: 10.1007/s00132-005-0847-0
32. Woltmann A, Bühren V. Shock trauma room management of spinal injuries in the framework of multiple trauma. A systematic review of the literature. Unfallchirurg. (2004) 107:911–8. doi: 10.1007/s00113-004-0829-4
33. Johansson C, Mellström D, Rosengren K, Rundgren A. Prevalence of vertebral fractures in 85-year-olds. Radiographic examination of 462 subjects Acta Orthop Scand. (1993) 64:25–7. doi: 10.3109/17453679308994521
34. Ulvik A, Wentzel-Larsen T, Flaatten H. Trauma patients in the intensive care unit: short- and long-term survival and predictors of 30-day mortality. Acta Anaesthesiol Scand. (2007) 51:171–7. doi: 10.1111/j.1399-6576.2006.01207.x
35. Joaquim AF, Ghizoni E, Tedeschi H, Batista UC, Patel AA. Clinical results of patients with thoracolumbar spine trauma treated according to the Thoracolumbar Injury Classification and Severity Score. J Neurosurg Spine. (2014) 20:562–7. doi: 10.3171/2014.2.SPINE121114
36. Lecun Y, Bottou L, Bengio Y, Haffner P. Gradient-based learning applied to document recognition. Proc IEEE. (1998) 86:2278–324. doi: 10.1109/5.726791
37. He K, Zhang X, Ren S, Sun J. Deep residual learning for image recognition. In: Proceedings of the IEEE Conference on Computer Vision and Pattern Recognition, Vol. 2016. (2016). p. 770–8. doi: 10.1109/CVPR.2016.90
38. Mayer C, Windhager S, Schaefer K, Mitteroecker P. BMI and WHR are reflected in female facial shape and texture: a geometric morphometric image analysis. PLoS ONE. (2017) 12:e0169336. doi: 10.1371/journal.pone.0169336
39. Gulshan V, Peng L, Coram M, Stumpe MC, Wu D, Narayanaswamy A, et al. Development and validation of a deep learning algorithm for detection of diabetic retinopathy in retinal fundus photographs. JAMA. (2016) 316:2402–10. doi: 10.1001/jama.2016.17216
40. Khurshid S, Friedman S, Reeder C, Di Achille P, Diamant N, Singh P, et al. Electrocardiogram-based deep learning and clinical risk factors to predict atrial fibrillation. Circulation. (2021). doi: 10.1161/CIRCULATIONAHA.121.057480
41. Kim H, Lampertico P, Nam J, Lee H, Kim S, Sinn D, et al. An artificial intelligence model to predict hepatocellular carcinoma risk in Korean and Caucasian patients with chronic hepatitis B. J Hepatol. (2021). doi: 10.1016/j.jhep.2021.09.025
42. Moore EE, Moore HB, Kornblith LZ, Neal MD, Hoffman M, Mutch NJ, et al. Trauma-induced coagulopathy. Nat Rev Dis Primers. (2021) 7:30. doi: 10.1038/s41572-021-00264-3
43. Fox EE, Holcomb JB, Wade CE, Bulger EM, Tilley BC. Earlier endpoints are required for hemorrhagic shock trials among severely injured patients. Shock. (2017) 47:567–73. doi: 10.1097/SHK.0000000000000788
44. Kalkwarf KJ, Drake SA, Yang Y, Thetford C, Myers L, Brock M, et al. Bleeding to death in a big city: an analysis of all trauma deaths from hemorrhage in a metropolitan area during 1 year. J Trauma Acute Care Surg. (2020) 89:716–22. doi: 10.1097/TA.0000000000002833
45. Eastridge BJ, Mabry RL, Seguin P, Cantrell J, Tops T, Uribe P, et al. Death on the battlefield (2001-2011): implications for the future of combat casualty care. J Trauma Acute Care Surg. (2012) 73(6 Suppl 5):S431–7. doi: 10.1097/TA.0b013e3182755dcc
46. Teixeira PG, Inaba K, Hadjizacharia P, Brown C, Salim A, Rhee P, et al. Preventable or potentially preventable mortality at a mature trauma center. J Trauma. (2007) 63:1338–46; discussion 1346–37. doi: 10.1097/TA.0b013e31815078ae
47. Niles SE, McLaughlin DF, Perkins JG, Wade CE, Li Y, Spinella PC, et al. Increased mortality associated with the early coagulopathy of trauma in combat casualties. J Trauma. (2008) 64:1459–63; discussion 1463–75. doi: 10.1097/TA.0b013e318174e8bc
48. Johansson PI, Stensballe J, Rasmussen LS, Ostrowski SR. A high admission syndecan-1 level, a marker of endothelial glycocalyx degradation, is associated with inflammation, protein C depletion, fibrinolysis, and increased mortality in trauma patients. Ann Surg. (2011) 254:194–200. doi: 10.1097/SLA.0b013e318226113d
49. Cohen MJ, Christie SA. Coagulopathy of trauma. Crit Care Clin. (2017) 33:101–18. doi: 10.1016/j.ccc.2016.08.003
50. Davenport RA, Brohi K. Coagulopathy in trauma patients: importance of thrombocyte function? Curr Opin Anaesthesiol. (2009) 22:261–6. doi: 10.1097/ACO.0b013e328325a6d9
51. Stansbury LG, Hess AS, Thompson K, Kramer B, Scalea TM, Hess JR. The clinical significance of platelet counts in the first 24 hours after severe injury. Transfusion. (2013) 53:783–9. doi: 10.1111/j.1537-2995.2012.03828.x
52. Brown LM, Call MS, Margaret Knudson M, Cohen MJ, Holcomb JB, Wade CE, et al. A normal platelet count may not be enough: the impact of admission platelet count on mortality and transfusion in severely injured trauma patients. J Trauma. (2011) 71(2 Suppl 3):S337–42. doi: 10.1097/TA.0b013e318227f67c
53. Kutcher ME, Redick BJ, McCreery RC, Crane IM, Greenberg MD, Cachola LM, et al. Characterization of platelet dysfunction after trauma. J Trauma Acute Care Surg. (2012) 73:13–9. doi: 10.1097/TA.0b013e318256deab
54. Goodman S, Weiss Y, Weissman C. Update on cardiac arrhythmias in the ICU. Curr Opin Crit Care. (2008) 14:549–54. doi: 10.1097/MCC.0b013e32830a4c5d
55. Agnihotri K, Pothineni NV, Charilaou P, Vaidya VR, Thakkar B, Goyal V, et al. Impact of atrial fibrillation on outcomes with motor vehicle accidents. Int J Cardiol. (2018) 250:128–32. doi: 10.1016/j.ijcard.2017.10.002
56. Moss TJ, Calland JF, Enfield KB, Gomez-Manjarres DC, Ruminski C, DiMarco JP, et al. New-onset atrial fibrillation in the critically ill. Crit Care Med. (2017) 45:790–7. doi: 10.1097/CCM.0000000000002325
57. Murthy K, Ondrey GJ, Malkani N, Raman G, Hodge MB, Marcantonio AJ, et al. The effects of hyponatremia on bone density and fractures: a systematic review and meta-analysis. Endocr Pract. (2019) 25:366–78. doi: 10.4158/EP-2018-0499
58. Schiara LAM, Moirano G, Grosso E, Richiardi L, Tibaldi M, Spertino E, et al. Hyponatremia, hypokalemia, and fragility fractures in old patients: more than an association? Calcif Tissue Int. (2020) 106:599–607. doi: 10.1007/s00223-020-00675-6
59. Bhandari SK, Adams AL Li BH, Rhee CM, Sundar S, Krasa H, Danforth KN, et al. et al: Sub-acute hyponatraemia more than chronic hyponatraemia is associated with serious falls and hip fractures. Intern Med J. (2020) 50:1100–8. doi: 10.1111/imj.14684
60. Sedlacek M, Schoolwerth AC, Remillard BD. Electrolyte disturbances in the intensive care unit. Semin Dial. (2006) 19:496–501. doi: 10.1111/j.1525-139X.2006.00212.x
61. Lentsck MH, Oliveira RR, Corona LP, Mathias TAF. Risk factors for death of trauma patients admitted to an Intensive Care Unit. Rev Lat Am Enfermagem. (2020) 28:e3236. doi: 10.1590/1518-8345.3482.3236
62. Bokshan SL, Han AL, DePasse JM, Eltorai AE, Marcaccio SE, Palumbo MA, et al. Effect of sarcopenia on postoperative morbidity and mortality after thoracolumbar spine surgery. Orthopedics. (2016) 39:e1159–64. doi: 10.3928/01477447-20160811-02
63. Puvanesarajah V, Jain A, Kebaish K, Shaffrey CI, Sciubba DM, De la Garza-Ramos R, et al. Poor nutrition status and lumbar spine fusion surgery in the elderly: readmissions, complications, and mortality. Spine. (2017) 42:979–83. doi: 10.1097/BRS.0000000000001969
64. Bruijns SR, Guly HR, Bouamra O, Lecky F, Lee WA. The value of traditional vital signs, shock index, and age-based markers in predicting trauma mortality. J Trauma Acute Care Surg. (2013) 74:1432–7. doi: 10.1097/TA.0b013e31829246c7
65. Hardin KS, Leasia KN, Haenel J, Moore EE, Burlew CC, Pieracci FM. The sequential clinical assessment of respiratory function (SCARF) score: a dynamic pulmonary physiologic score that predicts adverse outcomes in critically ill rib fracture patients. J Trauma Acute Care Surg. (2019) 87:1260–8. doi: 10.1097/TA.0000000000002480
66. El-Manzalawy Y, Abbas M, Hoaglund I, Cerna AU, Morland TB, Haggerty CM, et al. OASIS +: leveraging machine learning to improve the prognostic accuracy of OASIS severity score for predicting in-hospital mortality. BMC Med Inform Decis Mak. (2021) 21:156. doi: 10.1186/s12911-021-01517-7
67. Fueglistaler P, Amsler F, Schuepp M, Fueglistaler-Montali I, Attenberger C, Pargger H, et al. Prognostic value of Sequential Organ Failure Assessment and Simplified Acute Physiology II Score compared with trauma scores in the outcome of multiple-trauma patients. Am J Surg. (2010) 200:204–14. doi: 10.1016/j.amjsurg.2009.08.035
Keywords: intensive care units, nomogram, spinal fractures, prediction model, mortality
Citation: Wang H, Ou Y, Fan T, Zhao J, Kang M, Dong R and Qu Y (2021) Development and Internal Validation of a Nomogram to Predict Mortality During the ICU Stay of Thoracic Fracture Patients Without Neurological Compromise: An Analysis of the MIMIC-III Clinical Database. Front. Public Health 9:818439. doi: 10.3389/fpubh.2021.818439
Received: 19 November 2021; Accepted: 03 December 2021;
Published: 22 December 2021.
Edited by:
Thippa Reddy Gadekallu, VIT University, IndiaCopyright © 2021 Wang, Ou, Fan, Zhao, Kang, Dong and Qu. This is an open-access article distributed under the terms of the Creative Commons Attribution License (CC BY). The use, distribution or reproduction in other forums is permitted, provided the original author(s) and the copyright owner(s) are credited and that the original publication in this journal is cited, in accordance with accepted academic practice. No use, distribution or reproduction is permitted which does not comply with these terms.
*Correspondence: Yang Qu, cXV5QGpsdS5lZHUuY24=
†These authors have contributed equally to this work and share first authorship