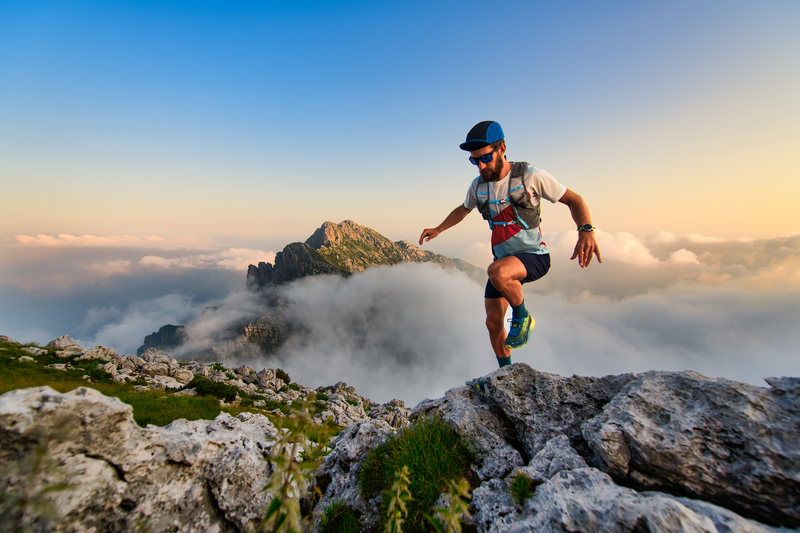
95% of researchers rate our articles as excellent or good
Learn more about the work of our research integrity team to safeguard the quality of each article we publish.
Find out more
ORIGINAL RESEARCH article
Front. Public Health , 24 December 2021
Sec. Digital Public Health
Volume 9 - 2021 | https://doi.org/10.3389/fpubh.2021.800549
This article is part of the Research Topic Machine Learning in Disease Screening, Diagnosis, and Surveillance View all 20 articles
Background: The etiology of fever of unknown origin (FUO) is complex and remains a major challenge for clinicians. This study aims to investigate the distribution of the etiology of classic FUO and the differences in clinical indicators in patients with different etiologies of classic FUO and to establish a machine learning (ML) model based on clinical data.
Methods: The clinical data and final diagnosis results of 527 patients with classic FUO admitted to 7 medical institutions in Chongqing from January 2012 to August 2021 and who met the classic FUO diagnostic criteria were collected. Three hundred seventy-three patients with final diagnosis were divided into 4 groups according to 4 different etiological types of classical FUO, and statistical analysis was carried out to screen out the indicators with statistical differences under different etiological types. On the basis of these indicators, five kinds of ML models, i.e., random forest (RF), support vector machine (SVM), Light Gradient Boosting Machine (LightGBM), artificial neural network (ANN), and naive Bayes (NB) models, were used to evaluate all datasets using 5-fold cross-validation, and the performance of the models were evaluated using micro-F1 scores.
Results: The 373 patients were divided into the infectious disease group (n = 277), non-infectious inflammatory disease group (n = 51), neoplastic disease group (n = 31), and other diseases group (n = 14) according to 4 different etiological types. Another 154 patients were classified as undetermined group because the cause of fever was still unclear at discharge. There were significant differences in gender, age, and 18 other indicators among the four groups of patients with classic FUO with different etiological types (P < 0.05). The micro-F1 score for LightGBM was 75.8%, which was higher than that for the other four ML models, and the LightGBM prediction model had the best performance.
Conclusions: Infectious diseases are still the main etiological type of classic FUO. Based on 18 statistically significant clinical indicators such as gender and age, we constructed and evaluated five ML models. LightGBM model has a good effect on predicting the etiological type of classic FUO, which will play a good auxiliary decision-making function.
Fever of unknown origin (FUO) is a difficult and active medical topic in the diagnosis and treatment of difficult and complicated diseases in internal medicine, and it is a challenging problem for physicians (1, 2). Currently, there are four categories of FUOs: classic FUO, FUO in hospitalized patients, FUO in patients with agranulocytosis, and FUO in patients with human immunodeficiency virus (HIV) infection (3, 4). Among them, classic FUO is the most common, which is defined as a disease that lasts for >3 weeks, has a body temperature of >38.3°C at least three times, and cannot be diagnosed after systematic and comprehensive examinations in the outpatient or inpatient department of the hospital for >1 week (5, 6). There are >200 kinds of causes of classic FUO (7). For clinicians, because of its complex etiology, lack of characteristic clinical signs, and inadequate laboratory tests, the diagnosis is very difficult (8). The etiological categories of classic FUO are infectious disease, non-infectious inflammatory disease (NIID), neoplastic disease, and others, and the treatment methods vary greatly, including anti-infective drugs, hormones, and chemotherapy (9–11). With the development of immunohistopathology and modern imaging (12, 13), the diagnosis of classic FUO has become easier, but the final diagnosis is often difficult and up to 50% of cases cannot be confirmed (8, 11, 14, 15).
The diagnostic process of a classic FUO includes four steps: to determine whether it belongs to classic FUO, a first stage of primary screening, a second stage of specific examination, and treatment (including symptomatic and diagnostic treatment) (4). Among them, the first stage (etiological screening) includes improving medical history collection, physical examination, and non-invasive laboratory and auxiliary examinations in line with local medical standards. After the first stage of screening, some patients are diagnosed and some patients offer no diagnostic clues and enter the second stage, which requires further specific examinations. The second phase of the process is more complex, partly invasive, and more expensive. Therefore, the first stage of etiology screening is very important. If the etiology of a FUO can be classified into one category, no matter the disease that caused the FUO, the direction of diagnosis can be determined, which is of great significance to physicians (16, 17). Previous studies of classic FUO have focused on the etiology, prognosis, or diagnosis of classic FUO (18, 19). So far, few researchers have studied the etiological causes of classic FUO from the perspective of clinical prediction models and machine learning (ML) (16). In recent years, ML has been widely used in the medical field and has achieved good results in disease diagnosis, risk assessment, and other factors (20–22).
In this study, the clinical data and etiological types of classic FUO patients were retrospectively analyzed, and a predictive model of FUO etiology was established to help clinicians make reasonable decisions in the diagnosis of classic FUO, improve diagnostic accuracy, and reduce the misdiagnosis rate.
The clinical data of 527 patients with classic FUO admitted to seven medical institutions in Chongqing from January 2012 to August 2021 were selected. The selected patients, whose ages ranged from 14 years old upwards, had each been hospitalized for more than a week with a fever higher than 38.3°C (101°F) that had occurred on several occasions and had persisted for at least 21 days (4, 8). Patients diagnosed with HIV infection before hospitalization, patients with immunodeficiency disorders, and pregnant women were screened out (4, 8). Of the 527 patients with classic FUO, 373 were finally diagnosed and 154 were not diagnosed at discharge. A total of 373 patients with classic FUO were divided into four groups according to their diagnosis and medical record information: infectious disease, NIID, neoplastic disease, and other diseases groups.
The index system of this study included general information (gender and age), past history (operation history and history of blood transfusion), accompanying symptoms (headache/consciousness disorders, nasal obstruction, sore throat, abdominal pain, arthralgia, muscle pain, and rash), physical (lymphadenopathy, hepatomegaly, and splenomegaly) and laboratory examinations [globulin, red blood cell (RBC), lactate dehydrogenase (LDH), C-reactive protein (CRP), procalcitonin (PCT), erythrocyte sedimentation rate (ESR), monocyte, basophils, eosinophils, lymphocyte, white blood cell (WBC), alkaline phosphatase (ALP), platelet (PLT), alanine aminotransferase (ALT), aspartate aminotransferase (AST), and gamma-glutamyltransferase (GGT)], and the final diagnosis of etiological types.
The research protocol was approved by the Medical Research Ethics Committee of Chongqing Medical University.
SPSS 25.0 statistical software was used for data processing. The continuity index was analyzed with a normality test, the median (M) and quartile (P25, P75) were used to express a non-normal distribution, the Kruskal–Wallis test was used to compare between groups. The normal distribution was expressed by x ± s. The analysis of variance was used to compare multiple groups, and the least significance difference (LSD) method was used for comparisons between two groups. The classification index was expressed by rate (%), and the comparison between groups was performed with a χ2-test. P < 0.05 was considered statistically significant. We used Python (version 3.7.3) for algorithm development.
This study was based on the aforementioned differences that were statistically significant indicators to build the model. In order to determine the best model for classifying etiological types in this study, we compared the performance of the following representative ML classification algorithms: RF, SVM, LightGBM, ANN, and NB. For each algorithm, we used the 5-fold cross-validation method to split the data, each time using the training set to train the model and verify the performance of the model on the test set data. Because the predicted etiological types of this study had four categories and the categories were imbalanced, we evaluated the performance of the model using micro-F1. micro-F1 is suitable for multi-classification problems and unbalanced data, and higher values represent better model performance. The calculation method for micro-F1 is as follows (taking four categories as an example):
a) Total
b) Total
c) Calculate
wherein TPi refers to a true positive of class i; FPi refers to a false positive of class i; TNi refers to a true negative of class i; and FNi refers to a false negative of class i.
A total of 527 patients with classic FUO were collected from seven medical institutions in Chongqing, including 303 men (57.5%) and 224 women (42.5%). Of the patients, 3.4% (n = 18), 19.2% (n = 101), 37.8% (n = 199), and 39.6% (n = 209) were <20, 20–39, 40–59, and ≥60 years, respectively. Table 1, Figure 1 show the distribution of classic FUO etiologies by age and gender, respectively.
Figure 1. The relationship between the distribution of etiology and gender in patients with classic FUO.
Infectious disease (n = 277; 52.5%) and NIID (n = 51; 9.7%) were the most common causes of classic FUO (Figure 2). Infectious diseases included bacterial (n = 193), tuberculosis (n = 46), and other bacterial infections (n = 2) and viral (n = 21), fungal (n = 12), parasitic (n = 1), and other pathogen infections (n = 2). The most common NIIDs were hemophagocytic syndrome (n = 12), anti-neutrophil cytoplasmic antibody (ANCA)-associated vasculitis (n = 9), systemic lupus erythematosus (n = 9), and Adult-onset Still's disease (n = 7). Thirty-one cases (5.9%) were diagnosed as neoplastic diseases, of which eight cases were lymphoma. Other causes, such as subacute thyroiditis (n = 9) and drug fever (n = 3), were diagnosed in 14 patients (2.7%). A total of 29.2% (n = 154) of the patients remained undiagnosed at discharge (Table 2).
There was a significant difference in the proportion of male and female patients with classic FUO among the four groups (χ2 = 8.24, P < 0.05). Male patients with FUO were common in the infectious and neoplastic disease groups, whereas female patients with FUO were common in the NIID and other diseases groups. There was a significant difference in age among the groups (H = 9.34, P < 0.05). The age of patients with tumor disease was the oldest [57.00 (43.50, 67.50)], whereas the age of patients with other diseases was the youngest [42.00 (32.50, 50.75)]. Regarding their past history, there was significant difference between patients with or without an history of blood transfusion and patients diagnosed with different types of causes (χ2 = 27.59, P < 0.001). There were significant differences in concomitant symptoms and physical examinations among the four groups (P < 0.05), except for nasal obstruction (χ2 = 2.66, P = 0.447), abdominal pain (χ2 = 5.79, P = 0.122), and splenomegaly (χ2 = 1.39, P = 0.708). In terms of laboratory tests, RBC (F = 6.97, P < 0.001), LDH (H = 12.37, P = 0.006), PCT (H = 15.69, P = 0.001), monocyte (H = 12.26, P = 0.007), lymphocyte (H = 8.51, P = 0.037), ALP (H = 9.83, P = 0.020), AST (H = 10.21, P = 0.017), and GGT (H = 8.70, P = 0.033) were performed. The results are shown in Tables 3, 4.
Table 3. Test of the difference of indexes (continuous indexes) in patients with classic FUO of different etiological types.
Table 4. Test of difference of indexes (classification indexes) in patients with classic FUO of different etiological types.
There were significant differences in the 18 characteristics including age and gender among the four different etiological types of patients with classic FUO. On the basis of the aforementioned indicators, five ML models were constructed and the whole dataset was included in the analyses. Table 5 shows the results of the five ML models. We mainly compared the sizes of the micro-F1 values. The micro-F1 value of each ML algorithm was the average of the five results in the 5-fold cross-validation. The micro-F1 of LightGBM was 75.8%, which was significantly higher than that of the other four ML algorithms (74.4, 73.4, 70.8, and 71.0%, respectively), and LightGBM has the best performance evaluation.
In order to better understand the contribution of each variable in our modeling results, we chose the LightGBM model with the best performance evaluation to present. Each variable was evaluated using Gini Importance, which is commonly used in ensembles of decision trees as a measure of a variable's impact in predicting a label that also takes into account the estimated error in randomly labeling an observation according to the known label distributions (23). Figure 3 shows the ranking of feature importance for all variables in the model. The results showed that age, PCT, ALP, AST, and GGT were the top five important features in the model, which made a great contribution to the prediction results.
Figure 3. Ranking of importance of characteristics of patients with classic FUO in predicting all types of causes.
For the LightGBM model defined as the final prediction model, the relationship between each variable and the prediction outcome for the model is illustrated in Figure 3. To determine the most salient features that drove the model predictions, we calculated the SHapley Additive exPlanation (SHAP) values of the best-performing models for different etiological types. Figures 4A–D shows the important characteristics of each etiological type. For infectious diseases, age, lymphocyte, and RBC increased and ALP and LDH decreased in favor of the classifier to predict infectious diseases. For NIID, higher LDH, monocyte, and AST; younger age; and a lower lymphocyte were helpful to the classifier to predict NIID. For neoplastic diseases, higher ALP, monocyte, and lymphocyte; older age; and previous history of blood transfusion were conducive to the classifier to predict the cause of neoplastic diseases. For other diseases, accompanied by headache or disturbance of consciousness and sore throat symptoms, younger age and lower PCT and GGT were conducive to the classifier to predict the cause of tumor diseases. Other important features of each etiological type are shown in Figure 4.
Figure 4. SHapley Additive exPlanations (SHAP) scores for identifying important features for the prediction of etiological types. (A) Infectious diseases; (B) NIID; (C) neoplastic diseases; and (D) other diseases. Colors indicate whether the value of the feature is high (red) or low (blue).
The etiological distribution of 527 patients with classic FUO was analyzed retrospectively, and the patients were divided into five groups according to the final diagnosis, including the group with unknown etiology of fever at discharge. Analysis showed that infectious diseases were the most common cause of classic FUO, followed by NIID. These results are consistent with most of the previous research results at home and abroad (8, 24–26), and the reasons for this phenomenon may be related to the non-standard use of antibiotics and drug resistance leading to disease persistence and changes in the rule of fever type. There were also different findings between this study and previous studies. First of all, the proportion of tuberculosis infection in infectious diseases was 16.6% (46/277), which was significantly lower than that of Li's study (30%) (27) but similar to that of Zhai's study (17.6%) (24). This change may be related to the strengthening of public awareness of tuberculosis and the improvement of medical conditions in recent years. Conversely, with the improvements in diagnosis and treatments, most tuberculosis infections can be diagnosed clearly in the early stage, thus reducing the proportion of patients with tuberculosis with FUO. Second, this study showed that the proportion of HIV infection was 3.6%, which is higher than the previous research results (1%) (8), which may be related to the increase in floating populations, sexual attitudes and sexual behavior, sexual orientation changes, and other factors that increase the risk of HIV infection. This study found that the proportion of NIIDs was 9.7%, which was significantly lower than the results of Naito's research (30.6%) in 2013 (28). It may be due to the early use of relevant immunological indicators, which enabled the early diagnosis of autoimmune diseases with more typical symptoms, and no longer classified as classic FUO. In this study, neoplastic diseases accounted for 5.9% of classic FUO, which was significantly lower than 15%, as reported in the literature (1), which may be due to PET-CT and serum tumor markers that have been widely used in recent years (29, 30). Many malignant tumors can be diagnosed early, and the widespread use of early biopsy is also a reason for the reduction of neoplastic diseases with classic FUO. Among other diseases, subacute thyroiditis accounts for a considerable proportion (64.3%), which is in line with the findings of Popovska-Jovicić (31). Subacute thyroiditis rarely has persistent fever as the only clinical manifestation, generally have some related clinical manifestations (32), such as upper respiratory tract infection symptoms, weight loss, neck pain, fatigue, and anorexia. Routine thyroid color ultrasound, thyroid antibody tests, and thyroid function tests are rarely performed; therefore, thyroiditis is easily misdiagnosed as an upper respiratory tract infection. The manifestations of elderly patients with subacute thyroiditis are often not obvious, and other underlying diseases may also have clinical signs of subacute thyroiditis, or patients cannot provide a good medical history. A total of 154 patients (29.2%) whose reason for fever was still not clear at the time of discharge from the hospital and who had early discharge due to economic or other personal reasons, had shorter hospitalization times, which led to inadequate examination and diagnostic treatment during hospitalization. A tentative diagnosis followed, and eventually, they were classified in the unclear group, which may be the reason for the high proportion of patients in this group.
In this study, 373 patients with classic FUO were divided into four groups and the groups were compared. The study found that male patients were more common and older in the tumor group than in the other three groups, whereas female patients were more common and younger in the other disease groups than in the other three groups. Regarding past history, accompanying symptoms and physical examination of patients with classic FUO had some special clinical signs that could provide clinical clues worthy of attention. Among them, arthralgia was very common. In this study, patients with classic FUO in all four groups with different etiological types had symptoms of arthralgia. The most common rheumatic diseases were heterogeneous diseases with joint, bone, and muscle pain as the main symptoms, which could have involved internal organs (33). Infectious arthritis in infectious diseases and some hematological tumors also have manifestations of arthralgia (34, 35). Rash is an important concomitant sign in patients with classic FUO, which may provide an important clue for the etiological diagnosis of classic FUO. In classic FUO, most diseases can be accompanied by clinical signs of skin rash (4), including (1) infectious diseases, such as Epstein–Barr virus infection, typhoid fever, and infective endocarditis; (2) NIID, such as systemic lupus erythematosus, dermatomyositis, and adult-onset Still's disease; (3) neoplastic diseases, such as lymphoma; and (4) other diseases, such as drug fever. In this study, all patients in the infectious disease, NIID, and neoplastic disease groups had symptoms of lymphadenopathy. Lymph node enlargement is either localized or generalized (36). Localized lymphadenopathy involves a draining region, often caused by a non-specific inflammatory response of the tissue or organ in the draining region or by lymphatic metastasis of malignant tumors corresponding to the draining region. Direct invasion of infectious pathogens or immune response caused by infection, allergic or autoimmune diseases, and invasion of neoplastic diseases can lead to systemic lymphadenopathy. In laboratory examinations, we found that the levels of LDH, PCT, monocyte, ALP, and GGT in the neoplastic disease group were significantly higher than those in the other three groups, whereas the level of AST in the NIID group was higher than that in the other three groups, and the levels of RBC and lymphocyte in the other disease groups were higher than those in the other three groups. Among them, the higher PCT levels in the neoplastic disease group was an interesting finding. In general, increase concentration of blood PCT is associated with severe bacterial infection. However, the clinical interpretation of elevated PCT concentration in blood represents a great challenge in cancer patients since its values might be influenced by several factors such as the presence of metastasis or neuroendocrine function of malignant tissue (37). In these cases, PCT concentrations can be elevated regardless of infections, manifesting a poor specificity for bacterial infection. Matzaraki et al. (38) indicated that patients with solid tumors, metastasis, and no evidence of infection had markedly elevated PCT levels, especially those with generalized metastatic disease. Similarly, Liu et al. (39) show that in the absence of bacterial infection, PCT levels are elevated in patients with certain inflammatory conditions, such as Kawasaki disease, Adult-onset Still's disease and some cancers like medullary carcinoma of the thyroid and small-cell lung carcinoma.
On the basis of the aforementioned discussion, this study screened 18 indicators, such as gender and age, and constructed a clinical prediction model of the etiological types of patients with classic FUO. The indicators included in the model are all from the indicators reported in the consensus on current management of fever of unknown origin, which adds reliability to the model we constructed. We compared five ML algorithms, all of which were tested using the 5-fold cross-validation method. These five ML algorithms are widely used in clinical prediction model construction. For example, SVM learning is widely used in cancer genomics (40). Compared to other ML algorithms, SVM is very powerful in identifying subtle patterns in complex data sets. However, there are also some shortcomings, such as slow training speed and difficult to understand the internal operation. Ivanović et al. (41) constructed an ANN model to predict the lymph node status of clinical lymph node-negative breast cancer. ANN have the ability to adapt to variable interaction and non-linear correlation, but also have the constraints of opaque underlying model and difficult to explain (42). Yang et al. (43) constructed a response prediction model of breast cancer neoadjuvant chemotherapy based on NB algorithm. In their study, the NB algorithm showed higher predictive values than other algorithms. Each ML algorithm has its own advantages and disadvantages, but in our research data, the micro-F1 value of the LightGBM model was 75.8%, which was significantly higher than that of the other four ML algorithms. It is suggested that the LightGBM model has better predictive performance for the classification of etiological types of patients with classic FUO. LightGBM is a distributed gradient lifting framework based on a decision tree algorithm, which has high efficiency and performance in dealing with binary classifications and multi-classification problems (44–46). LightGBM is an ensemble algorithm developed by Microsoft, which is superior to other machine learning methods for disease diagnosis in many cases (45). Fundamentally, this is achieved by combining multiple base classifiers into an ensemble model by learning the inherent statistics of the combined classifiers and, hence, outperforming the single classifiers. In addition, the RF model also achieved a high accuracy, micro-F1 was 74.4%, which was second only to LightGBM in the results of this study. RF is recognized as one type of ensemble learning method and are effective for the most classification and regression tasks (47), which further illustrates the advantages of ensemble learning methods. In this study, 18 indexes related to the etiological diagnosis of classic FUO were ranked in descending order of importance. Among them, the ranking of laboratory indicators can provide doctors with decision support for laboratory examination to a certain extent. We also calculated the SHAP value of the best performance model according to the cause category for explaining the model, and we could clearly see the influence of the characteristics of each cause type on the output of the model. In the following research, how to deal with the imbalanced data set and the small sample size problem is worth considering, because these problems affect the performance of the prediction model to some extent. Data imbalance is widespread in the real world, especially in medical big data, which affects the accuracy of medical diagnosis classification learning algorithm to a certain extent. In order to solve the problem of poor performance of medical diagnosis learning algorithms due to the serious shortage of minority samples, Han et al. (48) proposed a distribution-sensitive oversampling method for unbalanced large data, including the distribution-sensitive minority sample selection algorithm and the minority sample synthetic algorithm of weight adaptive adjustment, which improves the quality of newly generated minority samples. This may be a way to improve the accuracy of the model. In addition, few-shot learning is also a research direction that we should pay attention to. Few-shot learning is such a research topic that studies how to learn a new concept from few training data of this concept and has received significant attention from the machine learning community (49).
Our study has several limitations. First, this was a retrospective study, which had its own shortcomings, such as information bias. Second, the prediction model may have lacked generality because the 30 variables are still too few and many other variables were omitted because of the loss of too many values. Therefore, we hope to include more patients and variables in future studies. In addition, 154 cases with unknown etiology of fever were not included in the model, which do exist in the real world. Therefore, the accuracy in reality may be lower, and these situations should be taken into account in future studies.
In summary, this study retrospectively analyzed the clinical data of 527 patients with classic FUO from 7 medical institutions in Chongqing, discussed the differences of clinical indexes of 373 patients with classic FUO under 4 different etiological types, and introduced ML methods into the study of classic FUO to explore the application value of ML methods in the etiological diagnosis of classic FUO. The data of this study shows that infectious diseases are still the main etiological type of classic FUO. Based on 18 statistically significant clinical indicators such as gender and age, we constructed and compared 5 different ML algorithm models. The results show that compared with other algorithms, LightGBM is the best, and its micro-F1 value is 75.8%. We also use feature importance ranking and SHAP values to enhance the interpretability of the model. We believe that our model will provide clinicians with the most likely direction of etiological diagnosis in the diagnosis of classic FUO, assist clinicians to make reasonable decisions, improve the diagnostic accuracy of classic FUO, and reduce the misdiagnosis rate.
The original contributions presented in the study are included in the article/supplementary material, further inquiries can be directed to the corresponding author/s.
The studies involving human participants were reviewed and approved by Medical Research Ethics Committee of Chongqing Medical University. Written informed consent for participation was not required for this study in accordance with the national legislation and the institutional requirements.
YY, CC, and KP participated in the research design and coordination and helped in drafting the manuscript. KP contributed in data acquisition. YY, CC, YL, ZZ, and LX analyzed the data. All authors contributed to the article and approved the submitted version.
This study was supported by grants from the Natural Science Foundation of Chongqing (cstc2019jcyj-msxmX0027); the College of Medical Informatics, Chongqing Medical University, China, Student Research and Innovation Experiment Project (2019C011); the Philosophy and Social Sciences Innovation Team of Chongqing Medical University (ZX190101); and the Project of Innovative Research and Demonstration Base of Children's Medical Security in Children's Hospital Affiliated to Chongqing Medical University (NCRCCHD- 2019-HP-04).
The authors declare that the research was conducted in the absence of any commercial or financial relationships that could be construed as a potential conflict of interest.
All claims expressed in this article are solely those of the authors and do not necessarily represent those of their affiliated organizations, or those of the publisher, the editors and the reviewers. Any product that may be evaluated in this article, or claim that may be made by its manufacturer, is not guaranteed or endorsed by the publisher.
1. Kaya A, Ergul N, Kaya SY, Kilic F, Yilmaz MH, Besirli K, et al. The management and the diagnosis of fever of unknown origin. Expert Rev Anti Infect Ther. (2013) 11:805–15. doi: 10.1586/14787210.2013.814436
2. Li JJ, Huang WX, Shi ZY, Sun Q, Xin XJ, Zhao JQ, et al. Comparison of classical diagnostic criteria and Chinese revised diagnostic criteria for fever of unknown origin in Chinese patients. Ther Clin Risk Manag. (2016) 12:1545–51. doi: 10.2147/TCRM.S97863
3. Hayakawa K, Ramasamy B, Chandrasekar PH. Fever of unknown origin: an evidence-based review. Am J Med Sci. (2012) 344:307–16. doi: 10.1097/MAJ.0b013e31824ae504
4. Zhang WH, Li TS. Consensus on current management of fever of unknown origin. Shanghai Med J. (2018) 41:385–400.
5. Unger M, Karanikas G, Kerschbaumer A, Winkler S, Aletaha D. Fever of unknown origin (FUO) revised. Wien Klin Wochenschr. (2016) 128:796–801. doi: 10.1007/s00508-016-1083-9
6. Wright WF, Mulders-Manders CM, Auwaerter PG, Bleeker-Rovers CP. Fever of Unknown Origin (FUO) - a call for new research standards and updated clinical management. Am J Med. (2021). doi: 10.1016/j.amjmed.2021.07.038
7. Fusco FM, Pisapia R, Nardiello S, Cicala SD, Gaeta GB, Brancaccio G. Fever of unknown origin (FUO): which are the factors influencing the final diagnosis? A 2005-2015 systematic review. BMC Infect Dis. (2019) 19:653. doi: 10.1186/s12879-019-4285-8
8. Zhou G, Zhou Y, Zhong C, Ye H, Liu Z, Liu Y, et al. Retrospective analysis of 1,641 cases of classic fever of unknown origin. Ann Transl Med. (2020) 8:690. doi: 10.21037/atm-20-3875
9. Loizidou A, Aoun M, Klastersky J. Fever of unknown origin in cancer patients. Crit Rev Oncol Hematol. (2016) 101:125–30. doi: 10.1016/j.critrevonc.2016.02.015
10. Mulders-Manders CM, Engwerda C, Simon A, van der Meer JWM, Bleeker-Rovers CP. Long-term prognosis, treatment, and outcome of patients with fever of unknown origin in whom no diagnosis was made despite extensive investigation: a questionnaire based study. Medicine. (2018) 97:e11241. doi: 10.1097/MD.0000000000011241
11. Tan Y, Liu X, Shi X. Clinical features and outcomes of patients with fever of unknown origin: a retrospective study. BMC Infect Dis. (2019) 19:198. doi: 10.1186/s12879-019-3834-5
12. Besson FL, Chaumet-Riffaud P, Playe M, Noel N, Lambotte O, Goujard C, et al. Contribution of (18)F-FDG PET in the diagnostic assessment of fever of unknown origin (FUO): a stratification-based meta-analysis. Eur J Nucl Med Mol Imaging. (2016) 43:1887–95. doi: 10.1007/s00259-016-3377-6
13. Kouijzer IJE, Mulders-Manders CM, Bleeker-Rovers CP, Oyen WJG. Fever of unknown origin: the value of FDG-PET/CT. Semin Nucl Med. (2018) 48:100–7. doi: 10.1053/j.semnuclmed.2017.11.004
14. Kouijzer IJ, Bleeker-Rovers CP, Oyen WJ. FDG-PET in fever of unknown origin. Semin Nucl Med. (2013) 43:333–9. doi: 10.1053/j.semnuclmed.2013.04.005
15. Mulders-Manders CM, Simon A, Bleeker-Rovers CP. Rheumatologic diseases as the cause of fever of unknown origin. Best Pract Res Clin Rheumatol. (2016) 30:789–801. doi: 10.1016/j.berh.2016.10.005
16. Jiang H, Li Y, Zeng X, Xu N, Zhao C, Zhang J, et al. Exploring fever of unknown origin intelligent diagnosis based on clinical data: model development and validation. JMIR Med Inform. (2020) 8:e24375. doi: 10.2196/24375
17. Zhao MZ, Ruan QR, Xing MY, Wei S, Xu D, Wu ZH, et al. A diagnostic tool for identification of etiologies of fever of unknown origin in adult patients. Curr Med Sci. (2019) 39:589–96. doi: 10.1007/s11596-019-2078-3
18. Naito T, Tanei M, Ikeda N, Ishii T, Suzuki T, Morita H, et al. Key diagnostic characteristics of fever of unknown origin in Japanese patients: a prospective multicentre study. BMJ Open. (2019) 9:e032059. doi: 10.1136/bmjopen-2019-032059
19. Georga S, Exadaktylou P, Petrou I, Katsampoukas D, Mpalaris V, Moralidis EI, et al. Diagnostic value of (18)F-FDG-PET/CT in patients with FUO. J Clin Med. (2020) 9:2112. doi: 10.3390/jcm9072112
20. Abbasi B, Goldenholz DM. Machine learning applications in epilepsy. Epilepsia. (2019) 60:2037–47. doi: 10.1111/epi.16333
21. Allen A, Mataraso S, Siefkas A, Burdick H, Braden G, Dellinger RP, et al. A racially unbiased, machine learning approach to prediction of mortality: algorithm development study. JMIR Public Health Surv. (2020) 6:e22400. doi: 10.2196/22400
22. Jo YT, Joo SW, Shon SH, Kim H, Kim Y, Lee J. Diagnosing schizophrenia with network analysis and a machine learning method. Int J Methods Psychiatr Res. (2020) 29:e1818. doi: 10.1002/mpr.1818
23. Kim A, Miano T, Chew R, Eggers M, Nonnemaker J. Classification of twitter users who tweet about e-cigarettes. JMIR Public Health Surv. (2017) 3:e63. doi: 10.2196/publichealth.8060
24. Zhai YZ, Chen X, Liu X, Zhang ZQ, Xiao HJ, Liu G. Clinical analysis of 215 consecutive cases with fever of unknown origin: a cohort study. Medicine. (2018) 97:e10986. doi: 10.1097/MD.0000000000010986
25. Lv KL, Xin XJ, Li CH. The etiology analysis of 548 cases patients of fever with unknown origin. Electron J Emerg Infect Dis. (2020) 5:258–61. doi: 10.19871/j.cnki.xfcrbzz.2020.04.009
26. Tian D, Qi WJ, Wang C, Huang GW. Disease spectrum and etiology study on 347 patients with fever of unknown origin. Clin J Med Officers. (2020) 48:1433–6. doi: 10.16680/j.1671-3826.2020.12.14
27. Li JB, Zhang JP, Chen BY. Etiological factors for 541 patients with fever of unknown origin:a retrospective analysis. Chinese J Nosocomiol. (2011) 21:1587–9.
28. Naito T, Mizooka M, Mitsumoto F, Kanazawa K, Torikai K, Ohno S, et al. Diagnostic workup for fever of unknown origin: a multicenter collaborative retrospective study. BMJ Open. (2013) 3:e003971. doi: 10.1136/bmjopen-2013-003971
29. Wang W, Xu X, Tian B, Wang Y, Du L, Sun T, et al. The diagnostic value of serum tumor markers CEA, CA19-9, CA125, CA15-3, and TPS in metastatic breast cancer. Clin Chim Acta. (2017) 470:51–55. doi: 10.1016/j.cca.2017.04.023
30. Zaucha JM, Chauvie S, Zaucha R, Biggii A, Gallamini A. The role of PET/CT in the modern treatment of Hodgkin lymphoma. Cancer Treat Rev. (2019) 77:44–56. doi: 10.1016/j.ctrv.2019.06.002
31. Popovska-Jovicić B, Canović P, Gajović O, Raković I, Mijailović Z. Fever of unknown origin: most frequent causes in adults patients. Vojnosanitetski Pregled. (2016) 73:21–5. doi: 10.2298/VSP140820128P
32. Bahowairath FA, Woodhouse N, Hussain S, Busaidi MA. Lesson of the month 1: subacute thyroiditis: a rare cause of fever of unknown origin. Clin Med. (2017) 17:86–7. doi: 10.7861/clinmedicine.17-1-86
33. Kadavath S, Efthimiou P. Adult-onset Still's disease-pathogenesis, clinical manifestations, and new treatment options. Ann Med. (2015) 47:6–14. doi: 10.3109/07853890.2014.971052
34. Ross JJ. Septic arthritis of native joints. Infect Dis Clin North Am. (2017) 31:203–18. doi: 10.1016/j.idc.2017.01.001
35. Stephens DM, Byrd JC. How I manage ibrutinib intolerance and complications in patients with chronic lymphocytic leukemia. Blood. (2019) 133:1298–307. doi: 10.1182/blood-2018-11-846808
36. Gaddey HL, Riegel AM. Unexplained lymphadenopathy: evaluation and differential diagnosis. Am Fam Phys. (2016) 94:896–903.
37. Durnaś B, Watek M, Wollny T, Niemirowicz K, Marzec M, Bucki R, et al. Utility of blood procalcitonin concentration in the management of cancer patients with infections. Onco Targets Ther. (2016) 9:469–75. doi: 10.2147/OTT.S95600
38. Matzaraki V, Alexandraki KI, Venetsanou K, Piperi C, Myrianthefs P, Malamos N, et al. Evaluation of serum procalcitonin and interleukin-6 levels as markers of liver metastasis. Clin Biochem. (2007) 40:336–42. doi: 10.1016/j.clinbiochem.2006.10.027
39. Liu W, Sigdel KR, Wang Y, Su Q, Huang Y, Zhang YL, et al. High level serum procalcitonin associated gouty arthritis susceptibility: from a southern chinese han population. PLoS One. (2015) 10:e0132855. doi: 10.1371/journal.pone.0132855
40. Huang S, Cai N, Pacheco PP, Narrandes S, Wang Y, Xu W. Applications of Support Vector Machine (SVM) learning in cancer genomics. Cancer Genom Proteomics. (2018) 15:41–51. doi: 10.21873/cgp.20063
41. Ivanović D, Kupusinac A, Stokić E, Doroslovački R, Ivetić D. ANN prediction of metabolic syndrome: a complex puzzle that will be completed. J Med Syst. (2016) 40:264. doi: 10.1007/s10916-016-0601-7
42. Bi Q, Goodman KE, Kaminsky J, Lessler J. What is machine learning? A primer for the epidemiologist. Am J Epidemiol. (2019) 188:2222–39. doi: 10.1093/aje/kwz189
43. Yang L, Fu B, Li Y, Liu Y, Huang W, Feng S, et al. Prediction model of the response to neoadjuvant chemotherapy in breast cancers by a Naive Bayes algorithm. Comput Methods Programs Biomed. (2020) 192:105458. doi: 10.1016/j.cmpb.2020.105458
44. Kopitar L, Kocbek P, Cilar L, Sheikh A, Stiglic G. Early detection of type 2 diabetes mellitus using machine learning-based prediction models. Sci Rep. (2020) 10:11981. doi: 10.1038/s41598-020-68771-z
45. Rufo DD, Debelee TG, Ibenthal A, Negera WG. Diagnosis of diabetes mellitus using gradient boosting machine (LightGBM). Diagnostics. (2021) 11:1714. doi: 10.3390/diagnostics11091714
46. Zeng H, Yang C, Zhang H, Wu Z, Zhang J, Dai G, et al. A LightGBM-Based EEG analysis method for driver mental states classification. Comput Intell Neurosci. (2019) 2019:3761203. doi: 10.1155/2019/3761203
47. Wang Y, Xia ST, Tang Q, Wu J, Zhu X. A novel consistent random forest framework: bernoulli random forests. IEEE Trans Neural Networks Learn Syst. (2018) 29:3510–23. doi: 10.1109/TNNLS.2017.2729778
48. Han W, Huang Z, Li S, Jia Y. Distribution-sensitive unbalanced data oversampling method for medical diagnosis. J Med Syst. (2019) 43:39. doi: 10.1007/s10916-018-1154-8
Keywords: fever of unknown origin, machine learning, etiology, retrospective analysis, LightGBM algorithm
Citation: Yan Y, Chen C, Liu Y, Zhang Z, Xu L and Pu K (2021) Application of Machine Learning for the Prediction of Etiological Types of Classic Fever of Unknown Origin. Front. Public Health 9:800549. doi: 10.3389/fpubh.2021.800549
Received: 23 October 2021; Accepted: 08 December 2021;
Published: 24 December 2021.
Edited by:
Yi-Ju Tseng, National Central University, TaiwanReviewed by:
Chakrapani M, Kasturba Medical College, Mangalore, IndiaCopyright © 2021 Yan, Chen, Liu, Zhang, Xu and Pu. This is an open-access article distributed under the terms of the Creative Commons Attribution License (CC BY). The use, distribution or reproduction in other forums is permitted, provided the original author(s) and the copyright owner(s) are credited and that the original publication in this journal is cited, in accordance with accepted academic practice. No use, distribution or reproduction is permitted which does not comply with these terms.
*Correspondence: Kexue Pu, cHVrZXh1ZUBjcW11LmVkdS5jbg==
Disclaimer: All claims expressed in this article are solely those of the authors and do not necessarily represent those of their affiliated organizations, or those of the publisher, the editors and the reviewers. Any product that may be evaluated in this article or claim that may be made by its manufacturer is not guaranteed or endorsed by the publisher.
Research integrity at Frontiers
Learn more about the work of our research integrity team to safeguard the quality of each article we publish.