- 1Chantal BIYA International Reference Center for Research on HIV/AIDS Prevention and Management (CIRCB), Yaoundé, Cameroon
- 2Department of Experimental Medicine, University of Rome “Tor Vergata”, Rome, Italy
- 3Graduate School of Medicine, University of Tohoku, Sendai, Japan
- 4UFR IM2AG, Université Grenoble Alpes, Grenoble, France
- 5Graduate School of Life Sciences, University of Tohoku, Sendai, Japan
- 6Laboratory for Industrial and Applied Mathematics (LIAM), Department of Mathematics and Statistics, York University, Toronto, ON, Canada
- 7Africa-Canada Artificial Intelligence and Data Innovation Consortium (ACADIC), Department of Mathematics and Statistics, York University, Toronto, ON, Canada
Background: More than 1 year after the beginning of the international spread of coronavirus 2019 (COVID-19), the reasons explaining its apparently lower reported burden in Africa are still to be fully elucidated. Few studies previously investigated the potential reasons explaining this epidemiological observation using data at the level of a few African countries. However, an updated analysis considering the various epidemiological waves and variables across an array of categories, with a focus on African countries might help to better understand the COVID-19 pandemic on the continent. Thus, we investigated the potential reasons for the persistently lower transmission and mortality rates of COVID-19 in Africa.
Methods: Data were collected from publicly available and well-known online sources. The cumulative numbers of COVID-19 cases and deaths per 1 million population reported by the African countries up to February 2021 were used to estimate the transmission and mortality rates of COVID-19, respectively. The covariates were collected across several data sources: clinical/diseases data, health system performance, demographic parameters, economic indicators, climatic, pollution, and radiation variables, and use of social media. The collinearities were corrected using variance inflation factor (VIF) and selected variables were fitted to a multiple regression model using the R statistical package.
Results: Our model (adjusted R-squared: 0.7) found that the number of COVID-19 tests per 1 million population, GINI index, global health security (GHS) index, and mean body mass index (BMI) were significantly associated (P < 0.05) with COVID-19 cases per 1 million population. No association was found between the median life expectancy, the proportion of the rural population, and Bacillus Calmette–Guérin (BCG) coverage rate. On the other hand, diabetes prevalence, number of nurses, and GHS index were found to be significantly associated with COVID-19 deaths per 1 million population (adjusted R-squared of 0.5). Moreover, the median life expectancy and lower respiratory infections rate showed a trend towards significance. No association was found with the BCG coverage or communicable disease burden.
Conclusions: Low health system capacity, together with some clinical and socio-economic factors were the predictors of the reported burden of COVID-19 in Africa. Our results emphasize the need for Africa to strengthen its overall health system capacity to efficiently detect and respond to public health crises.
Introduction
The global pandemic of “coronavirus disease 2019” (COVID-19), caused by the “Severe acute respiratory syndrome coronavirus-2” (SARS- CoV-2) first emerged in Wuhan City, Hubei Province of China (1–3). The SARS-CoV-2 infection rapidly spread in China and thereafter, was reported in the United States, Europe, and all the other continents (4–7). Following this international spread and the threat it represents for the world, the Director-General of the WHO declared COVID-19 a “global pandemic” on 11 March 2020.
Regarding the African continent, the first case, a contact of a person with a history of travel to China was notified by Egypt, an African country predicted to be among those with a high importation risk, on 14 February 2020 (7, 8). The epidemic evolved rapidly and as of 15 March 2020, a total of 25 countries reported COVID-19 cases in the WHO AFRO region (https://apps.who.int/iris/bitstream/handle/10665/331451/OEW11-0915032020.pdf). In this regard, the WHO has called Africa to “wake up” to the coronavirus threat and prepare for the worst scenario. There was an agreement among nearly all the predictive models that the new pandemic will be disastrous on the African countries, which have a weaker health system and are already facing huge public health challenges; this would be in terms of transmission, severity, and impact of COVID-19 (9–14).
Though the COVID-19 pandemic hit, directly and indirectly, the vital health services on the continent (15), unexpectedly, Africa has not yet experienced the predicted large burden of COVID-19 (16–18). As of 4 September 2021, the entire continent has reported a toll of approximately 8 million cases and 200,000 deaths, 3.6% and 4.4% of the global figures, respectively, despite the African population is about 16% of the worldwide population.
A number of hypotheses have been proposed to explain this unexpected observation. The early response and continent wide-response, under-reporting, younger age of the population, low international air traffic flows, the climate, cross-immunity, and the use of anti-parasitic drugs are among the commonest listed factors (7, 19–23). Additional factors, such as the effect of Bacillus Calmette–Guérin (BCG) vaccination, genetic background, population demography, climatic factors, and economic factors have been previously investigated to explore their association with COVID-19 burden (24–29).
So far, most of these studies analyzed only a single or few groups of possible covariates, but the factors determining the patterns of COVID-19 transmission, as it is the case of many other emerging/re-emerging infectious diseases, require a multi-factorial analysis. To the best of our knowledge, no study investigated the association of biological, clinical, organizational (health system capacity), economical-financial, climatic, environmental (including pollution, radiation, and air traffic) and behavioral (use of social media) parameters focusing only on the African countries, which, on the one hand, share among themselves similarities, but, on the other hand, are uniquely different from the Western countries, with potential country-specific confounders that might not be adjusted for in most models.
Moreover, an updated analysis taking into consideration the various epidemiological waves that occurred on the continent might provide public health decisions- and policymakers with useful information to properly deal with the still ongoing pandemic. To overcome some of the methodological gaps outlined above, in this analysis specifically focused on Africa, we investigated all the factors potentially related to COVID-19 burden, to obtain a comprehensive picture of the plausible reasons for the observed persistently lower transmission and mortality rates of COVID-19 on the African continent, more than a year after the first cases were reported on the continent.
Materials and Methods
Aim and Design of the Study
This study aimed to investigate the factors associated with the low burden of COVID-19 in Africa. More specifically, we investigated the factors potentially connected with the risk of COVID-19 transmission (number of COVID-19 cases per 1 million population) and the risk of COVID-19 fatality (number of COVID-19 deaths per 1 million population). To achieve this objective, data concerning different categories of covariates (epidemiological, socio-demographic, climatic, environmental, and economical-financial) from 54 African countries were collected and the multiple linear regression model was fitted to the data.
Data Collection
SARS-CoV-2 Transmission and Mortality
The data for SARS-CoV-2 transmission and mortality came from the coronavirus page of “Our World in Data” database (github.com/owid/covid-19-data/tree/master/public/data). The cumulative number of COVID-19 reported cases in the African countries from the beginning of the pandemic on February 14, 2020, to February 4, 2021, were extracted. Given that we aimed to investigate both COVID-19 transmission risk and fatality, we used two outcome variables separately to construct the models. To estimate COVID-19 transmission, we chose the total confirmed cases of COVID-19 per 1 million populations, over the total absolute number of cases because it is a better proxy to estimate the risk of being infected. Similarly, the total deaths attributed to COVID-19 per 1 million populations were used to estimate the risk of dying, which is a measure of disease severity.
Independent Variables
The covariates used in the analysis were collected across several fields of science, including clinical/diseases data, health system performance, demographic parameters, economic indicators, climatic variables, the levels of pollution and radiation, and the use of social media. The data were collected from publicly available and well-known, reliable sources, such as the World Bank Open Data, the Health Nutrition and Population Statistics, the WHO Global Health Observatory (GHO), the Institute of Health Metrics and Evaluation (IHME), the Global Health Security Index (GHS), and the Our World in Data databases among the others. The description of each covariate by category is reported in Table 1. These variables were included based on the list of variables previously hypothesized to be among the possible reasons for the low burden of COVID-19 in Africa. These variables have been reviewed in two scientific literature produced by our research group (one of them is currently under peer-review) (30).
Statistical Analysis
We summarized continuous variables as means and SDs, while the qualitative/categorical variables were expressed as proportions. Few missing values were found for 15 variables and imputed with median value since the median is robust to outliers. The “variance inflation factor” (VIF) was used to measure how much the variance of the estimated regression coefficient was increased due to collinearity and variables with a VIF greater than 10 were removed from the analysis. The numerical variables were scaled to ease downstream comparison of regression coefficients.
The selected covariates were used to analyze the relationship between the dependent and independent variables while controlling for other epidemiological, economical/financial, and organizational (health system) related indicators. To determine which covariates were significantly associated with COVID-19 transmission and death risk in Africa, the number of COVID-19 cases per 1 million population and the number of COVID-19 deaths per 1 million population were considered as our response variables, respectively. The sign and significance of the regression model coefficients were used to assess the effects of the independent variables on the outcome variable. After compiling the variables, they were then fitted to a multiple regression model using the “lm” function of the “stats” package of the R statistical environment, version 3.5 (R Core Team 2020, Austria).
The general formula of the multiple linear regression model is given as follows:
Where “y” is the dependent or outcome variable; v0, v1, v2, …, vn are the unknown parameters estimated by the regression model; and x1, x2, x3, …, xn are independent covariates variables. The P-values less than 0.05 were considered statistically significant.
Radar Plot
To visualize the scaled values of statistically significant covariates from the regression model, the African countries having a wide range of COVID-19 cases per 1 million populations and COVID-19 deaths per one million populations were represented on a radar chart, constructed using Microsoft Office Excel 2016 (Microsoft Corporation, WA, USA).
Results
Regression Analysis Results
The results obtained from fitting the variables in Table 1 to a multiple regression model are shown in Tables 2, 3, for the cases per 1 million populations and deaths per 1 million populations, respectively.
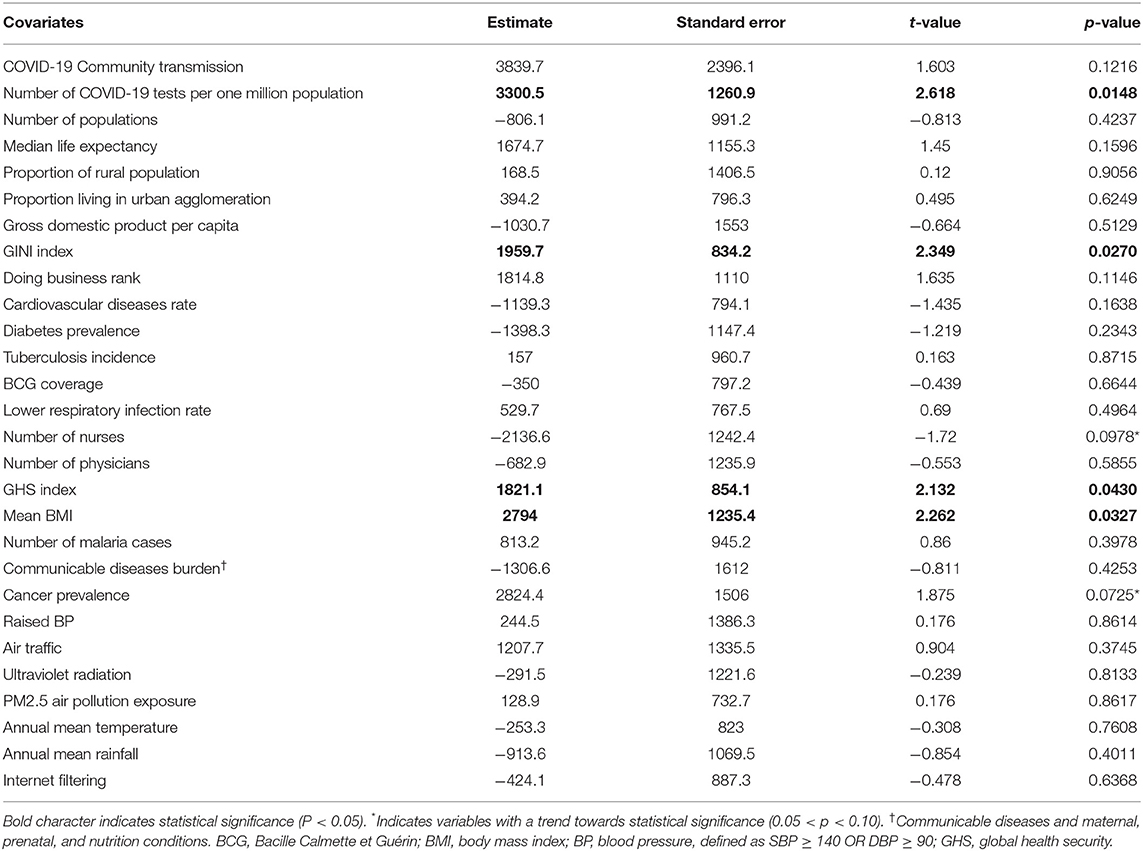
Table 2. Multiple regression analysis using COVID-19 cases per one million populations as the dependent variable.
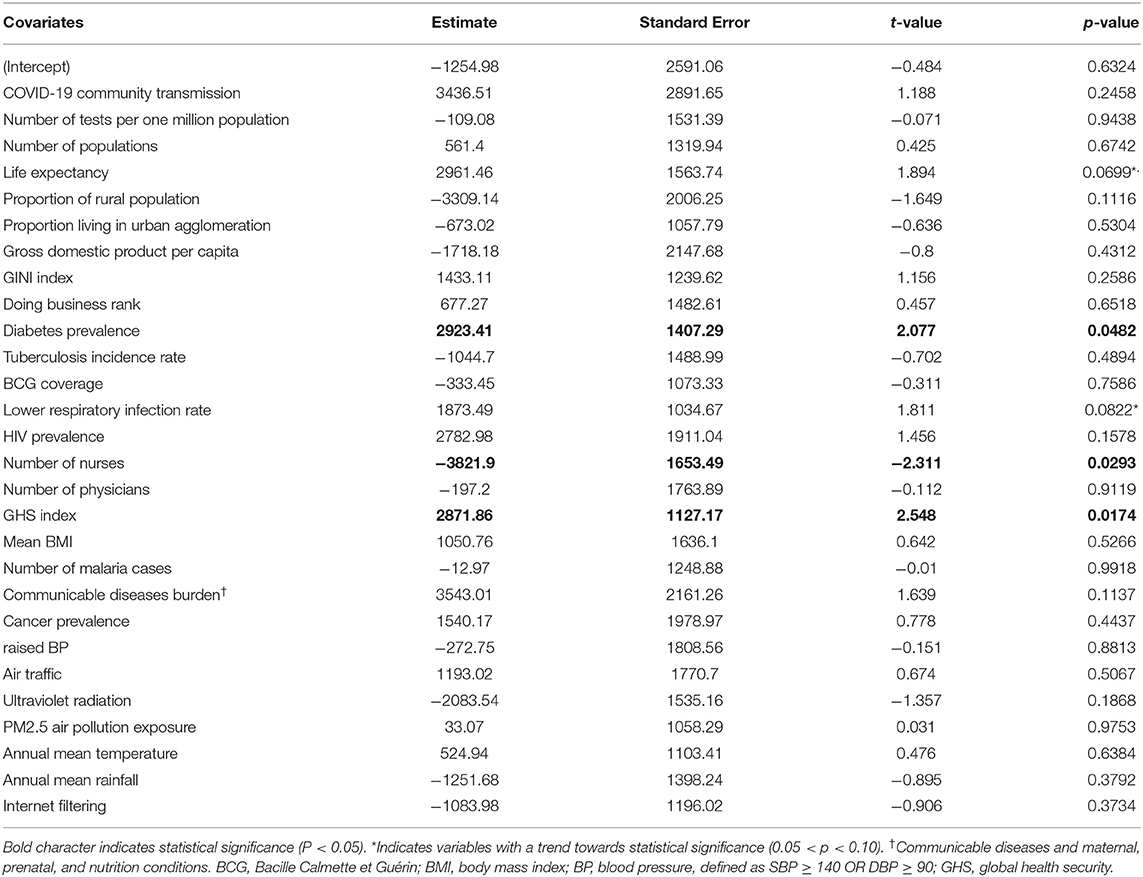
Table 3. The multiple regression analysis outputs using COVID-19 deaths per one million populations as a dependent variable.
Factors Associated With COVID-19 Cases per 1 Million Populations in Africa
The regression model constructed to determine the factors associated with COVID-19 cases per 1 million populations in Africa had an adjusted R-squared of 0.7 and the variables significantly associated (P < 0.05) with COVID-19 cases per 1 million populations on the African continent were the number of COVID-19 tests per 1 million populations, the GINI inequality index, the GHS detection index, and the mean body mass index (BMI). Table 2 shows, for example, that a unit increase in COVID-19 tests per 1 million populations, GINI index, the GHS, and BMI were significantly associated with nearly 3,300, 1,960, 1,821, and 2,794 units increase of the COVID-19 cases per 1 million population in Africa, respectively. Also, the number of nurses per 1,000 populations and cancer prevalence showed a negative and positive trend towards significance, respectively. Of note, no association was found with the median life expectancy, the proportion of the rural population, prevalence of diabetes, rate of cardiovascular diseases, BCG coverage, number of reported malaria cases, and climate variables.
Factors Associated With COVID-19 Deaths per 1 Million Population in Africa
The second regression model shown in Table 3, constructed to determine factors associated with COVID-19 deaths per 1 million populations in Africa had an adjusted R-squared of 0.5 and the variables significantly associated (P < 0.05) with COVID-19 deaths per 1 million populations on the African continent are the prevalence of diabetic patients, number of nurses per 1,000 populations, and the GHS detection index. This table shows that a unit increase in the prevalence of diabetic patients, number of nurses per 1,000 populations, and the GHS index was significantly associated, respectively with 2,923, 3,821, and 2,871 units increase in the COVID-19 deaths per 1 million populations in Africa. Therefore, the rate of diabetics and health system related indices (nurses, GHS) are important predictors of COVID-19 fatality in the people of Africa. Moreover, the median life expectancy and lower respiratory infections rate showed a trend towards significance. Of note, no associations between COVID-19 deaths per 1 million populations and BCG coverage, number of reported malaria cases, or communicable disease burden were found.
Graphical Representation of Significantly Associated Variables
Figure 1 shows how eight countries having a wide range of COVID-19 cases per 1 million populations, with scaled values representing the number of tests per 1 million populations are characterized by the GINI inequality index, GHS index, and BMI. This radar plot shows that the country ranking in covariate values mostly conforms to the statistical trends suggested by the regression model. For example, when compared with the countries having high COVID-19 cases per 1 million populations, e.g., South Africa, Tanzania, Burundi, Nigeria, and Chad, which have the lowest COVID-19 cases per 1 million populations display a lower number of tests per 1 million populations, a lesser BMI, lower GINI inequality index, and lesser GHS index. This indicates that most countries with a high number of COVID-19 cases per million populations tend to fit the statistically high COVID-19 cases profile suggested by the regression model. However, some exceptions are observed. This is the case of Tunisia which shows a relatively low number of tests per 1 million populations and a very low GINI index but is among the countries with the highest transmission rate.
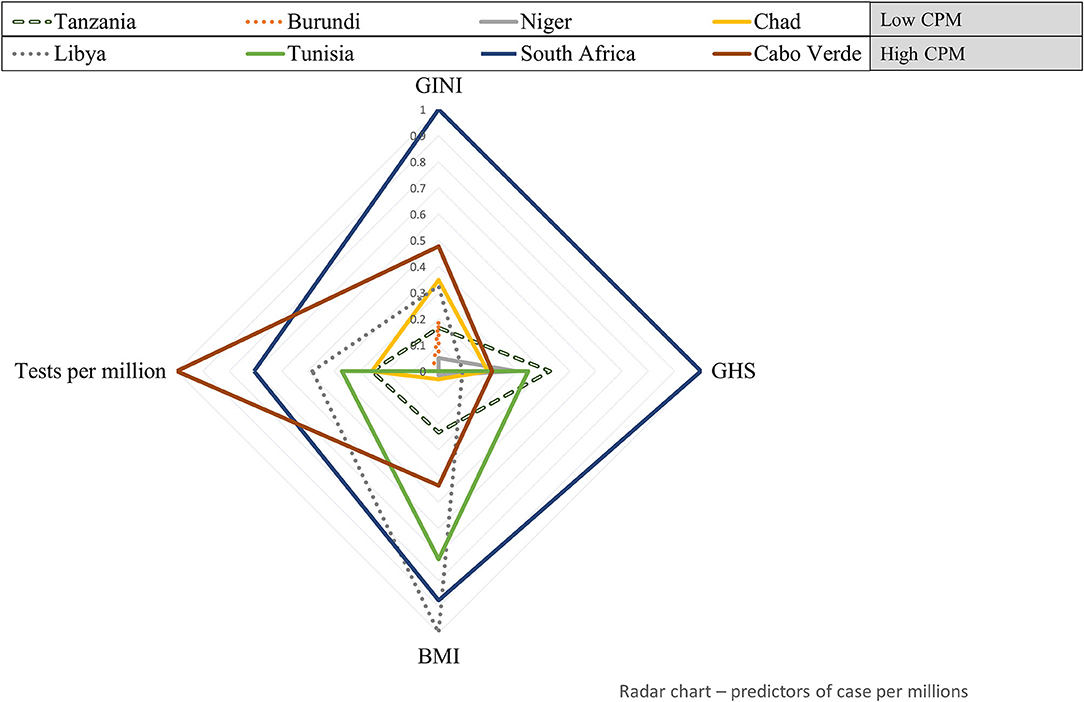
Figure 1. Health system, socio-economic, and clinical factors' profile of eight African countries with a wide range of COVID-19 cases per 1 million populations. Standardized values of variables significantly associated with COVID-19 cases per 1 million populations (test per millions, GINI, global health security [GHS], and body mass index [BMI]) are plotted for eight countries having the lowest (upper-left) to highest (lower-right) COVID-19 cases per million. CPM, Cases per 1 million population.
Figure 2 shows how eight countries covering a wide range of COVID-19 deaths per 1 million populations are characterized by the prevalence of diabetes, number of nurses, and the GHS index. This radar plot shows that the country ranking in the covariate values conforms in part with the findings from the regression model. In general, when compared with countries with the lowest number of COVID-19 deaths per 1 million populations, South Africa, Egypt, and Tunisia have a higher prevalence of diabetes and GHS index. An opposite trend was observed for the number of nurses per 1000 populations which had a negative association in the model. As was the case with transmission rate, exceptions exist; Mauritius, for example, has a low rate of COVID-19 deaths but recorded a high prevalence of diabetes.
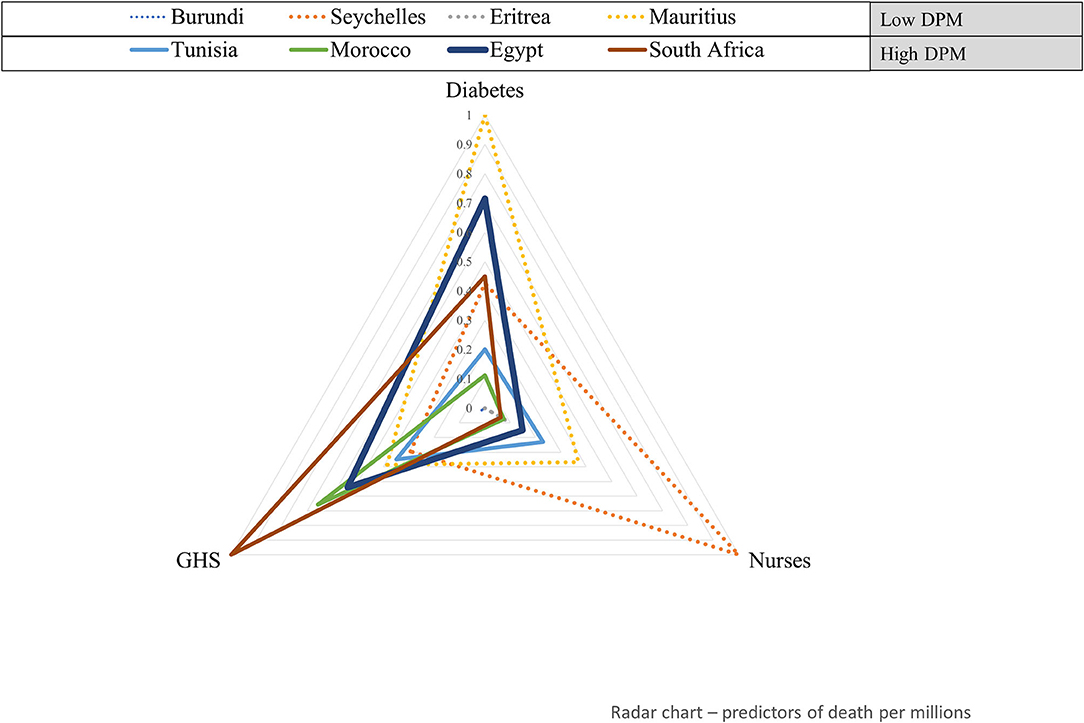
Figure 2. Health system and clinical factors' profile of eight African countries with a wide range of COVID-19 deaths per 1 million populations. Standardized values of variables significantly associated with COVID-19 deaths per 1 million populations (GHS index and diabetes) are plotted for eight countries having the lowest (upper-left) to highest (lower-right) COVID-19 DPM. DPM, Deaths per one million population.
Discussion
The COVID-19 pandemic has been a devastating crisis worldwide and more especially in Western countries. Despite the availability of several preventive measures, including the vaccine roll-out and the huge body of scientific knowledge generated to understand its pathophysiology and its transmission patterns, it still remains one of the major global health concerns. In this analysis with a focus on Africa, we investigated the factors with potential effects on the risk of COVID-19 infection and severity (mortality rate).
The variables that independently predicted the risk of acquiring COVID-19 infection were socio-economic factors, clinical factors, and health system capacity to detect and respond to epidemics. Some authors argue that the low burden of COVID-19 might be explained by the flaws in the detection and reporting system of most African countries (31). Our model constructed on data collected on African countries confirmed that the number of tests performed per million population was positively and significantly associated with the number of cases per million population (P = 0.0148). It is not excluded that this association can also be explained by the fact that the countries with high testing (likely due to high demand in testing) would be likely those with a high risk of infection transmission. However, this finding was further supported by the fact that the number of cases per million population was predicted by the GHS detection index (P = 0.0430), a measure of the overall health security and related capabilities (24). Though the COVID-19 pandemic has revealed the underestimation of the preparedness of some countries with lower scores on the GHS index (32), efforts are needed by African countries to improve their overall health system capacity, including GHS (33), which ranges from 55% (South Africa) to 16% (Equatorial Guinea) (Supplementary Table 1).
In the same line, we found that the higher the number of nurses and midwives per 1,000 population, the lower the risk of COVID-19 infection (even though only borderline statistically significant, P = 0.0978). On the other hand, the lower median age of the population in Africa (<20 years) has been most often found to be associated with the decreased severity of symptoms of COVID-19 infection on the continent. Several reports indicate that, in general, the adult population (>20 years) contributes disproportionately to the worldwide number of COVID-19 cases (34–36). However, our results suggest that life expectancy was not an independent predictor of the risk of COVID-19. This might imply that the detection of COVID-19 infection should be extended across all age groups for better control of the pandemics.
Another important finding was that the risk of COVID-19 infection increased with the inequalities index (GINI) within a country (P = 0.0270). This could be explained by the fact that high GINI values lead to the presence of more vulnerable people, generally working in the informal sector; and thus, to higher exposure to COVID-19. This is especially true for Africa where inequalities are fueled by conflicts and instabilities. Such situations might lead to limited access to healthcare facilities and to a higher number of infectious diseases, including the COVID-19 infection (24).
Interestingly, we found that the mean BMI in the adult population was positively associated with the risk of acquiring COVID-19 infection (P = 0.0327). The association of BMI with an increased risk of COVID-19 infection was previously documented in the literature (37, 38). Obesity and unhealthy lifestyle in general increase the risk of diseases, achieved through the impairment of immunity (39). Given that a study showed that an increased BMI was especially observed in people younger than 40 yr and of Black ethnicity (40), an appropriate policy to control the risk of infection in this group is needed. Our analysis did not confirm the increased risk with other underlying comorbidities, such as diabetes, cardiovascular diseases, and hypertension (41, 42). This analysis equally confirms that particular attention should be given to the patients with cancer, which, even though only with a trend, was associated with increased COVID-19 infection (P = 0.0725). Such association was previously documented and was especially attributed to lung cancer (43).
Of note, some clinical factors previously documented to be associated with the increased risk of COVID-19 infection, which was not confirmed in our analysis are as follows BCG vaccination coverage (44–47); the number of reported malaria cases (48–55), and deaths due to communicable diseases (56, 57). Lastly, regarding the environmental factors, such as rainfall, temperature, and exposure to UV radiation, contrary to some reports, we did not observe any association (23, 58).
Regarding the fatality of COVID-19 (deaths per 1 million population), our model indicates that about 50% of the risk of dying was explained by the variables we considered (Adjusted R-squared: 0.4976, 0.00468). Among the variables significantly associated are the clinical, health system related, and demographic factors.
Regarding the clinical factors, our data confirmed that the prevalence of diabetes independently predicted the risk of death. This observation has been widely documented also at the individual level (59, 60). This has been attributed to the fact that the patients with diabetes have delayed viral clearance, decreased immunity, and increased angiotensin-converting enzyme 2 (ACE2) expression, leading to an inappropriate response to COVID-19 infection and other secondary infections (41, 61, 62). Another consideration is that older age, which is a risk of COVID-19 severity is also associated with diabetes, with its prevalence and underlying complications increasing with older age (63, 64). Indeed, we found that life expectancy, which is a proxy of the proportion of the older age, slightly predicted the number of deaths per million. Another clinical factor found to be slightly associated with the COVID-19 deaths was the mortality rate due to the lower respiratory infections (P = 0.0822). This finding was also expected because a systematic review and meta-analysis reported that COVID-19 co-infections or superinfections with respiratory pathogens were associated with an increased odds of death (65). Of note, the BCG coverage, the number of malaria cases, and the burden of communicable diseases did not seem to have any protective effects on the number of deaths per million (P < 0.05 for all). Contrary to the risk of being infected, the GINI index was not associated with COVID-19 severity. This is probably because the population in Africa is generally very young (median age: 20 years), thus the severity will be less, likely even in the situations of promiscuity and precarity. This homogeneous population is also demonstrated by the fact that the rural population and those living in highly populated cities were not associated with COVID-19 death.
Concerning the health system related factors, the number of nurses (P = 0.0293) and the GHS index (P = 0.0174) positively predicted the COVID-19 fatality, as it was the case of the risk for acquiring the infection. The response capacity of a health system is indeed important to respond to COVID-19 infection, especially in elderly people and those with comorbidities, since their clinical management requires hospitalization. The insufficient number of nurses, in particular, would affect the access to healthcare facilities and the quality of healthcare provided.
Our findings have practical implications in terms of the achievement of herd immunity. Intriguingly, median life expectancy showed a trend towards statistical significance. COVID-19 spread among the youth may result in a higher number of asymptomatic/mild cases, with fewer cases, making populations not immunologically naïve to the virus anymore. This should be verified by seroepidemiologic surveys (66).
In conclusion, our model suggests that there are many explanatory variables that account for the risk of COVID-19 infection and death in Africa. These may span across the biological and epidemiological variables, including the growth rate and the basic reproduction number of the virus (67, 68), logistic-organizational (69, 70), and socio-cultural factors. The COVID-19 pandemic represents, both directly and indirectly, a threat for the continent, thus Africa should continue to strengthen its health system, also by building on its previous experience with infectious outbreaks (such as Ebola or Zika) to mitigate against the effects of the still ongoing pandemic.
However, our study has some limitations. First, as for all the ecological investigations, our study is subject to “ecological fallacy” and biases. For example, some of the data analyzed are not up-to-date, and therefore might not reflect the current situation. This is especially true for non-communicable diseases that rapidly rise in Africa. Second, there might also be some biases in the reporting of the number of cases and deaths, due to the different testing and reporting capacity across the African countries, and biases in the measurement and reporting of some covariates, such as the GINI index, which was expected to be high in some countries with instability and security issues, such as Chad. Third, the variables associated with COVID-19 cases and deaths reported in this study do not necessarily imply causality as this study design is not suitable for drawing any causal relationship. Another study limitation is the relatively moderate R-squared value (especially of the second model), which suggests that other variables not included in our regression model may explain from 35 to 40% of the variance in the number of COVID-19 cases and deaths per million population.
Conclusions
In the present study, we found that in Africa, the clinical conditions and health system capacity were predictors of both COVID-19 transmission and fatality, while socio-economic indices were associated only with the risk of COVID-19 transmission. This study complements and adds to the existing studies investigating the possible reasons for the low rates of COVID-19 cases and deaths in Africa and offering a broader picture at the continent level.
Our results emphasize the need for Africa to strengthen its overall health system capacity to efficiently detect and respond to COVID-19, as well as to potential future pandemics and outbreaks. Further studies based on the data collected at an individual level are warranted to confirm these observations. Moreover, for a better understanding of COVID-19 epidemiology across the continent, such studies should also consider the SARS-CoV-2 viral diversity.
Data Availability Statement
Publicly available datasets were analyzed in this study. This data can be found here: github.com/Jdkong/socioeconomicafrica.
Author Contributions
All the authors contributed to the conception and development of the study. YB, ET, MF, and GM collected and prepared the data. YB, NB, JK, ET, and MF analyzed the data. All the authors contributed significantly to the drafting of the manuscript and approved its final version. JK and NB revised the manuscript.
Funding
This research is funded by the International Development Research Center (IDRC), Canada (Grant No. 109559-001).
Conflict of Interest
The authors declare that the research was conducted in the absence of any commercial or financial relationships that could be construed as a potential conflict of interest.
Publisher's Note
All claims expressed in this article are solely those of the authors and do not necessarily represent those of their affiliated organizations, or those of the publisher, the editors and the reviewers. Any product that may be evaluated in this article, or claim that may be made by its manufacturer, is not guaranteed or endorsed by the publisher.
Supplementary Material
The Supplementary Material for this article can be found online at: https://www.frontiersin.org/articles/10.3389/fpubh.2021.751197/full#supplementary-material
Supplementary Table 1. Descriptive statistics of the main factors of interest.
References
1. World Health Organization. Novel Coronavirus (2019-nCoV). SITUATION REPORT - 1 as of January 21, 2020. World Heal Organ COVID-19 response. (2020). Available online at: https://www.who.int/docs/default-source/coronaviruse/situation-reports/20200121-sitrep-1-2019-ncov.pdf?sfvrsn=20a99c10_4 (accessed March 20, 2021)
2. Rich S, Poschman K, Hu H, Mavian C, Cook R, Salemi M, et al. Sociodemographic, ecological, and spatiotemporal factors associated with HIV drug resistance in Florida: a retrospective analysis. J Infect Dis. (2020) 223:866–75. doi: 10.1093/infdis/jiaa413
3. Zhu N, Zhang D, Wang W, Li X, Yang B, Song J, et al. A Novel coronavirus from patients with pneumonia in China, 2019. N Engl J Med. (2020) 382:727–33. doi: 10.1056/NEJMoa2001017
4. Guan W, Ni Z, Hu YY, Liang W, Ou C, He J, et al. Clinical characteristics of coronavirus disease 2019 in China. N Engl J Med. (2020) 382:1708–20. doi: 10.1056/NEJMoa2002032
5. Spiteri G, Fielding J, Diercke M, Campese C, Enouf V, Gaymard A, et al. First cases of coronavirus disease 2019 (COVID-19) in the WHO European Region, 24 January to 21 February 2020. Eurosurveillance. (2020) 25:1. doi: 10.2807/1560-7917.ES.2020.25.9.2000178
6. Worobey M, Pekar J, Larsen BB, Nelson MI, Hill V, Joy JB, et al. The emergence of SARS-CoV-2 in Europe and North America. Science. (2020) 370:564–70. doi: 10.1126/science.abc8169
7. Massinga Loembé M, Tshangela A, Salyer SJ, Varma JK, Ouma AEO, Nkengasong JN. COVID-19 in Africa: the spread and response. Nat Med. (2020) 26:999–1003. doi: 10.1038/s41591-020-0961-x
8. Gilbert M, Pullano G, Pinotti F, Valdano E, Poletto C, Boëlle PY, et al. Preparedness and vulnerability of African countries against importations of COVID-19: a modelling study. Lancet. (2020) 395:871–7. doi: 10.1016/S0140-6736(20)30411-6
9. Taboe HB, Salako K V, Tison JM, Ngonghala CN, Glèlè Kakaï R. Predicting COVID-19 spread in the face of control measures in West Africa. Math Biosci. (2020) 328:108431. doi: 10.1016/j.mbs.2020.108431
10. van Zandvoort K, Jarvis CI, Pearson CAB, Davies NG, Nightingale ES, Munday JD, et al. Response strategies for COVID-19 epidemics in African settings: a mathematical modelling study. BMC Med. (2020) 18:324. doi: 10.1186/s12916-020-01789-2
11. Cabore JW, Karamagi HC, Kipruto H, Asamani JA, Droti B, Seydi ABW, et al. The potential effects of widespread community transmission of SARS-CoV-2 infection in the World Health Organization African Region: a predictive model. BMJ Glob Heal. (2020) 5:2647. doi: 10.2139/ssrn.3569842
12. Evans M V, Garchitorena A, Rakotonanahary RJL, Drake JM, Andriamihaja B, Rajaonarifara E, et al. Reconciling model predictions with low reported cases of COVID-19 in Sub-Saharan Africa: insights from Madagascar. Glob Health Action. (2020) 13:1816044. doi: 10.1080/16549716.2020.1816044
13. Pearson CAB, van Schalkwyk C, Foss AM, O'Reilly KM, Pulliam JRC. Projected early spread of COVID-19 in Africa through 1 June 2020. Eurosurveillance. (2020) 25:2000543 doi: 10.2807/1560-7917.ES.2020.25.18.2000543
14. Clark A, Jit M, Warren-Gash C, Guthrie B, Wang HHX, Mercer SW, et al. Global, regional, and national estimates of the population at increased risk of severe COVID-19 due to underlying health conditions in 2020: a modelling study. Lancet Glob Heal. (2020) 8:e1003–17. doi: 10.1016/S2214-109X(20)30264-3
15. COVID-19 hits life-saving health services in Africa. Available online at: http://whotogo-whoafroccmaster.newsweaver.com/JournalEnglishNewsletter/1l8t7wec3zvy48iiujdam4?email=true&lang=en&a=11&p=58142773 (accessed April 8, 2021)
16. Musanabaganwa C, Cubaka V, Mpabuka E, Semakula M, Nahayo E, Hedt-Gauthier BL, et al. One hundred thirty-three observed COVID-19 deaths in 10 months: unpacking lower than predicted mortality in Rwanda. BMJ Glob Heal. (2021) 6:e004547. doi: 10.1136/bmjgh-2020-004547
17. Musa HH, Musa TH, Musa IHIH, Musa IHIH, Ranciaro A, Campbell MC. Addressing Africa's pandemic puzzle: perspectives on COVID-19 transmission and mortality in sub-Saharan Africa. Int J Infect Dis. (2021) 102:483–8. doi: 10.1016/j.ijid.2020.09.1456
18. Wells CR, Stearns JK, Lutumba P, Galvani AP. COVID-19 on the African continent. Lancet Infect Dis. (2020) 20:1368–70. doi: 10.1016/S1473-3099(20)30374-1
19. Lalaoui R, Bakour S, Raoult D, Verger P, Sokhna C, Devaux C, et al. What could explain the late emergence of COVID-19 in Africa? New Microbes New Infect. (2020) 38:100760. doi: 10.1016/j.nmni.2020.100760
20. El-Sadr WM, Justman J. Africa in the path of Covid-19. N Engl J Med. (2020) 383:e11. doi: 10.1056/NEJMp2008193
21. Kalk A, Schultz A. SARS-CoV-2 epidemic in African countries—are we losing perspective? Lancet Infect Dis. (2020) 20:1370. doi: 10.1016/S1473-3099(20)30563-6
22. Nkengasong JN, Mankoula W. Looming threat of COVID-19 infection in Africa: act collectively, and fast. Lancet. (2020) 395:841–2. doi: 10.1016/S0140-6736(20)30464-5
23. Nguimkeu P, Tadadjeu S. Why is the number of COVID-19 cases lower than expected in Sub-Saharan Africa? A cross-sectional analysis of the role of demographic and geographic factors. World Dev. (2021) 138:105251. doi: 10.1016/j.worlddev.2020.105251
24. Gesesew HA, Koye DN, Fetene DM, Woldegiorgis M, Kinfu Y, Geleto AB, et al. Risk factors for COVID-19 infection, disease severity and related deaths in Africa: a systematic review. BMJ Open. (2021) 11:e044618. doi: 10.1136/bmjopen-2020-044618
25. Meo SA, Abukhalaf AA, Alomar AA, Aljudi TW, Bajri HM, Sami W, et al. Impact of weather conditions on incidence and mortality of COVID-19 pandemic in Africa. Eur Rev Med Pharmacol Sci. (2020) 24:9753–9. doi: 10.26355/eurrev_202009_23069
26. Meyer A, Sadler R, Faverjon C, Cameron AR, Bannister-Tyrrell M. Evidence that higher temperatures are associated with a marginally lower incidence of COVID-19 cases. Front Public Heal. (2020) 8:367. doi: 10.3389/fpubh.2020.00367
27. Lin K, Fong DYT, Zhu B, Karlberg J. Environmental factors on the SARS epidemic: air temperature, passage of time and multiplicative effect of hospital infection. Epidemiol Infect. (2006) 134:223–30. doi: 10.1017/S0950268805005054
28. Ortiz-Fernández L, Sawalha AH. Genetic variability in the expression of the SARS-CoV-2 host cell entry factors across populations. Genes Immun. (2020) 21:269–72. doi: 10.1038/s41435-020-0107-7
29. Miyasaka M. Is BCG vaccination causally related to reduced COVID-19 mortality? EMBO Mol Med. (2020) 12:e12661 doi: 10.15252/emmm.202012661
30. Tsinda EK, Mmbando GS. Recent updates on the possible reasons for the low incidence and morbidity of COVID-19 cases in Africa. Bull Natl Res Cent. (2021) 45:133. doi: 10.1186/s42269-021-00589-9
31. Nordling L. Africa's pandemic puzzle: Why so few cases and deaths? Science. (2020) 369:756–7. doi: 10.1126/science.369.6505.756
32. Abbey EJ, Khalifa BAA, Oduwole MO, Ayeh SK, Nudotor RD, Salia EL, et al. The Global Health Security Index is not predictive of coronavirus pandemic responses among Organization for Economic Cooperation and Development countries. PLoS ONE. (2020) 15:e0239398 doi: 10.1371/journal.pone.0239398
33. Dzinamarira T, Dzobo M, Chitungo I. COVID-19: A perspective on Africa's capacity and response. J Med Virol. (2020) 92:2465–72. doi: 10.1002/jmv.26159
34. Monod M, Blenkinsop A, Xi X, Hebert D, Bershan S, Tietze S, et al. Age groups that sustain resurging COVID-19 epidemics in the United States. Science. (2021) 371:eabe8372. doi: 10.1126/science.abe8372
35. Bishnoi P, Kumar P, Singh A, Sahu M, Bansal V. A Study about the impact of weather, life expectancy and tourism on the transmission of COVID-19. Coronaviruses. (2020) 01:e280521186888. doi: 10.2174/2666796701999201014155715
36. Davies NG, Klepac P, Liu Y, Prem K, Jit M, Pearson CAB, et al. Age-dependent effects in the transmission and control of COVID-19 epidemics. Nat Med. (2020) 26:1205–11. doi: 10.1101/2020.03.24.20043018
37. Sattar N, Ho FK, Gill JM, Ghouri N, Gray SR, Celis-Morales CA, et al. BMI and future risk for COVID-19 infection and death across sex, age and ethnicity: preliminary findings from UK biobank. Diabetes Metab Syndr Clin Res Rev. (2020) 14:1149–51. doi: 10.1016/j.dsx.2020.06.060
38. Malik VS, Ravindra K, Attri SV, Bhadada SK, Singh M. Higher body mass index is an important risk factor in COVID-19 patients: a systematic review and meta-analysis. Environ Sci Pollut Res. (2020) 27:42115–23. doi: 10.1007/s11356-020-10132-4
39. de Frel DL, Atsma DE, Pijl H, Seidell JC, Leenen PJM, Dik WA, et al. The impact of obesity and lifestyle on the immune system and susceptibility to infections such as COVID-19. Front Nutr. (2020) 7:597600. doi: 10.3389/fnut.2020.597600
40. Gao M, Piernas C, Astbury NM, Hippisley-Cox J, O'Rahilly S, Aveyard P, et al. Associations between body-mass index and COVID-19 severity in 6·9 million people in England: a prospective, community-based, cohort study. Lancet Diabetes Endocrinol. (2021) 9:350–9. doi: 10.1016/S2213-8587(21)00089-9
41. Deravi N, Fathi M, Vakili K, Yaghoobpoor S, Pirzadeh M, Mokhtari M, et al. SARS-CoV-2 infection in patients with diabetes mellitus and hypertension: a systematic review. Rev Cardiovasc Med. (2020) 21:385–97. doi: 10.31083/j.rcm.2020.03.78
42. Jeong I-K, Yoon KH, Lee MK. Diabetes and COVID-19: global and regional perspectives. Diabetes Res Clin Pract. (2020) 166:108303. doi: 10.1016/j.diabres.2020.108303
43. Yang L, Chai P, Yu J, Fan X. Effects of cancer on patients with COVID-19: a systematic review and meta-analysis of 63,019 participants. Cancer Biol Med. (2021) 18:298–307. doi: 10.20892/j.issn.2095-3941.2020.0559
44. Arts RJW, Moorlag SJCFM, Novakovic B, Stunnenberg HG, Van Crevel R, Netea Correspondence MG, et al. Vaccination protects against experimental viral infection in humans through the induction of cytokines associated with trained immunity in brief. Cell Host Microbe. (2018) 23:89–100. doi: 10.1016/j.chom.2017.12.010
45. Saeed S, Quintin J, Kerstens HHD, Rao NA, Aghajanirefah A, Matarese F, et al. Epigenetic programming of monocyte-to-macrophage differentiation and trained innate immunity. Science. (2014) 345:1251086–1251086. doi: 10.1126/science.1251086
46. Netea MG, Joosten LABB, Latz E, Mills KHGG, Natoli G, Stunnenberg HG, et al. Trained immunity: A program of innate immune memory in health and disease. Science. (2016) 352:427. doi: 10.1126/science.aaf1098
47. Lau CM, Adams NM, Geary CD, Weizman O, El, Rapp M, Pritykin Y, et al. Epigenetic control of innate and adaptive immune memory. Nat Immunol. (2018) 19:963–72. doi: 10.1038/s41590-018-0176-1
48. Napoli PE, Nioi M. Global spread of coronavirus disease 2019 and malaria: an epidemiological paradox in the early stage of a pandemic. J Clin Med. (2020) 9:1138. doi: 10.3390/jcm9041138
49. Adams D, Alshaban F. COVID-19 cases are less prevalent in countries where malaria is endemic, suggesting a role for anti-malarial drugs as prophylaxis. Eye Reports. (2020) 6:S11–S116 doi: 10.16964/er.v6i1.100
50. Hajizadeh R, Behnemoon M. Is the New Coronavirus Disease (COVID-19) Pandemic Halted by Malaria Epidemics? Arch Bone Jt Surg. (2020) 8:319–20. doi: 10.22038/abjs.2020.47662.2336
51. Cheong DHJ, Tan DWS, Wong FWS, Tran T. Anti-malarial drug, artemisinin and its derivatives for the treatment of respiratory diseases. Pharmacol Res. (2020) 158:104901 doi: 10.1016/j.phrs.2020.104901
52. Savarino AA, Boelaert JRJR, Cassone A, Majori GG, Cauda R. Effects of chloroquine on viral infections: an old drug against today's diseases. Lancet Infect Dis. (2003) 3:722–7. doi: 10.1016/S1473-3099(03)00806-5
53. Plantone D, Koudriavtseva T. Current and future use of chloroquine and hydroxychloroquine in infectious, immune, neoplastic, and neurological diseases: a mini-review. Clin Drug Investig. (2018) 38:653–71. doi: 10.1007/s40261-018-0656-y
54. D'alessandro S, Scaccabarozzi D, Signorini L, Perego F, Ilboudo DP, Ferrante P, et al. The use of antimalarial drugs against viral infection. Microorganisms. (2020) 8:85 doi: 10.3390/microorganisms8010085
55. Heidary F, Gharebaghi R. Ivermectin: a systematic review from antiviral effects to COVID-19 complementary regimen. J Antibiot (Tokyo). (2020) 73:593–602. doi: 10.1038/s41429-020-0336-z
56. Gebrecherkos T, Gessesse Z, Kebede Y, Gebreegzabher A, Tassew G, Abdulkader M, et al. Effect of co-infection with parasites on severity of COVID-19. medRxiv. (2021) 267:1–15. doi: 10.1101/2021.02.02.21250995
57. McFarlane AJ, McSorley HJ, Davidson DJ, Fitch PM, Errington C, Mackenzie KJ, et al. Enteric helminth-induced type I interferon signaling protects against pulmonary virus infection through interaction with the microbiota. J Allergy Clin Immunol. (2017) 140:1068–78.e6. doi: 10.1016/j.jaci.2017.01.016
58. Leichtweis BG, de Faria Silva L, da Silva FL, Peternelli LA. How the global health security index and environment factor influence the spread of COVID-19: a country level analysis. One Heal. (2021) 12:100235. doi: 10.1016/j.onehlt.2021.100235
59. Nkhata SG, Ngoma TN, Chilenga PM. SARS-CoV 2 (Covid-19) heterogeneous mortality rates across countries may be partly explained by life expectancy, calorie intake, and prevalence of diabetes. Hum Ecol. (2020) 48:633–8. doi: 10.1007/s10745-020-00191-z
60. Wu Z, McGoogan JM. Characteristics of and important lessons from the coronavirus disease 2019 (COVID-19) outbreak in china: summary of a report of 72314 cases from the chinese center for disease control and prevention. JAMA - J Am Med Assoc. (2020) 323:1239–42. doi: 10.1001/jama.2020.2648
61. Fang L, Karakiulakis G, Roth M. Are patients with hypertension and diabetes mellitus at increased risk for COVID-19 infection? Lancet Respir Med. (2020) 8:e21. doi: 10.1016/S2213-2600(20)30116-8
62. Huang Y, Lu Y, Huang Y-M, Wang M, Ling W, Sui Y, et al. Obesity in patients with COVID-19: a systematic review and meta-analysis. Metabolism. (2020) 113:154378. doi: 10.1016/j.metabol.2020.154378
63. Apicella M, Campopiano MC, Mantuano M, Mazoni L, Coppelli A, Del Prato S. COVID-19 in people with diabetes: understanding the reasons for worse outcomes. Lancet Diabetes Endocrinol. (2020) 8:782–92. doi: 10.1016/S2213-8587(20)30238-2
64. Muniangi-Muhitu H, Akalestou E, Salem V, Misra S, Oliver NS, Rutter GA. Covid-19 and Diabetes: A Complex Bidirectional Relationship. Front Endocrinol. (2020) 11:758 doi: 10.3389/fendo.2020.582936
65. Musuuza JS, Watson L, Parmasad V, Putman-Buehler N, Christensen L, Safdar N. Prevalence and outcomes of co-infection and superinfection with SARS-CoV-2 and other pathogens: A systematic review and metaanalysis. PLoS ONE. (2021) 16:e0251170. doi: 10.1371/journal.pone.0251170
66. Mbow M, Lell B, Jochems SP, Cisse B, Mboup S, Dewals BG, et al. COVID-19 in Africa: Dampening the storm? Science. (2020) 369:624–6. doi: 10.1126/science.abd3902
67. Musa SS, Zhao S, Wang MH, Habib AG, Mustapha UT, He D. Estimation of exponential growth rate and basic reproduction number of the coronavirus disease 2019 (COVID-19) in Africa. Infect Dis Poverty. (2020) 9:96. doi: 10.1186/s40249-020-00718-y
68. Yu CJ, Wang ZX, Xu Y, Hu MX, Chen K, Qin G. Assessment of basic reproductive number for COVID-19 at global level: a meta-analysis. Medicine. (2021) 100:e25837. doi: 10.1097/MD.0000000000025837
69. Musa SS, Zhao S, Hussaini N, Zhuang Z, Wu Y, Abdulhamid A, et al. Estimation of COVID-19 under-ascertainment in Kano, Nigeria during the early phase of the epidemics. Alexandria Eng J. (2021) 60:4547–54. doi: 10.1016/j.aej.2021.03.003
Keywords: COVID-19, transmission, mortality, Africa, cross-country analysis
Citation: Bouba Y, Tsinda EK, Fonkou MDM, Mmbando GS, Bragazzi NL and Kong JD (2021) The Determinants of the Low COVID-19 Transmission and Mortality Rates in Africa: A Cross-Country Analysis. Front. Public Health 9:751197. doi: 10.3389/fpubh.2021.751197
Received: 31 July 2021; Accepted: 14 September 2021;
Published: 21 October 2021.
Edited by:
Daihai He, Hong Kong Polytechnic University, Hong Kong, SAR ChinaReviewed by:
Salihu Sabiu Musa, Hong Kong Polytechnic University, Hong Kong, SAR ChinaJosé Eduardo Levi, University of São Paulo, Brazil
Copyright © 2021 Bouba, Tsinda, Fonkou, Mmbando, Bragazzi and Kong. This is an open-access article distributed under the terms of the Creative Commons Attribution License (CC BY). The use, distribution or reproduction in other forums is permitted, provided the original author(s) and the copyright owner(s) are credited and that the original publication in this journal is cited, in accordance with accepted academic practice. No use, distribution or reproduction is permitted which does not comply with these terms.
*Correspondence: Jude Dzevela Kong, amRrb25nJiN4MDAwNDA7eW9ya3UuY2E=
†These authors have contributed equally to this work and share last authorship