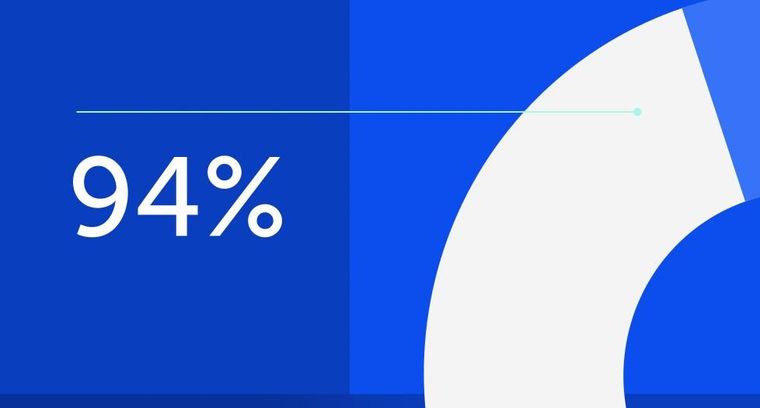
94% of researchers rate our articles as excellent or good
Learn more about the work of our research integrity team to safeguard the quality of each article we publish.
Find out more
SYSTEMATIC REVIEW article
Front. Public Health, 29 October 2021
Sec. Life-Course Epidemiology and Social Inequalities in Health
Volume 9 - 2021 | https://doi.org/10.3389/fpubh.2021.741334
This article is part of the Research TopicMeasuring and Analysing Social Determinants of Health in the Era of Big DataView all 12 articles
The five-year survival rate of childhood cancer has increased substantially over the past 50 yr; however, racial/ethnic disparities in health outcomes of survival have not been systematically reviewed. This scoping review summarized health disparities between racial/ethnic minorities (specifically non-Hispanic Black and Hispanic) and non-Hispanic White childhood cancer survivors, and elucidated factors that may explain disparities in health outcomes. We used the terms “race”, “ethnicity”, “childhood cancer”, “pediatric cancer”, and “survivor” to search the title and abstract for the articles published in PubMed and Scopus from inception to February 2021. After removing duplicates, 189 articles were screened, and 23 empirical articles were included in this review study. All study populations were from North America, and the mean distribution of race/ethnicity was 6.9% for non-Hispanic Black and 4.5% for Hispanic. Health outcomes were categorized as healthcare utilization, patient-reported outcomes, chronic health conditions, and survival status. We found robust evidence of racial/ethnic disparities over four domains of health outcomes. However, health disparities were explained by clinical factors (e.g., diagnosis, treatment), demographic (e.g., age, sex), individual-level socioeconomic status (SES; e.g., educational attainment, personal income, health insurance coverage), family-level SES (e.g., family income, parent educational attainment), neighborhood-level SES (e.g., geographic location), and lifestyle health risk (e.g., cardiovascular risk) in some but not all articles. We discuss the importance of collecting comprehensive social determinants of racial/ethnic disparities inclusive of individual-level, family-level, and neighborhood-level SES. We suggest integrating these variables into healthcare systems (e.g., electronic health records), and utilizing information technology and analytics to better understand the disparity gap for racial/ethnic minorities of childhood cancer survivors. Furthermore, we suggest national and local efforts to close the gap through improving health insurance access, education and transportation aid, racial-culture-specific social learning interventions, and diversity informed training.
The shifting racial/ethnic makeup of the population indicates that in as early as 2045 the United States (US) will become a “minority majority population” country (1). By 2060, non-White individuals will make up more than 60% of the population (2). Racial/ethnic disparities in health are the race- and ethnicity-specific illnesses, injuries, or mortality (3) that disproportionately impact the marginalized groups. The growing diversity of the US population will be accompanied by growing health disparities.
Health equity is considered one of the four basic human rights (3), yet determinants of the inequity and effective implementation strategies to improve racial/ethnic disparity in health outcomes are still limited. Although life expectancy of the US general population has steadily increased since the 1950s in the US, non-Hispanic Black individuals have a 40% higher overall mortality rates (4) and non-Hispanic Black and Hispanic populations have a higher burden of chronic health conditions (e.g., cancer, heart disease, diabetes) (5) compared to non-Hispanic White individuals. In addition, minority populations often have lower healthcare utilization and access to quality care (6). In the context of oncology, health disparities in the US are significantly different in the rates of cancer screening, incidence, survival, treatment-related complications, and quality-of-life (7). Although the 5-yr relative cancer survival rates of childhood cancer have reached 94% among the child and 85% among adolescent survivors (8), there is evidence of lower patient-reported outcomes and survival rates (9–11) in minority vs. non-Hispanic White survivors. Furthermore, the mechanisms behind these disparities are understudied.
While scholars have yet to agree on specifics, it is clear that health disparities are influenced by multiple factors. Some argue that socioeconomic status (SES) is a stronger determinant of health outcomes than race per se (12). Others suggest that cultural (13) or population-level factors (14) contribute to health disparities in childhood cancer survivors. Neighborhoods with a higher proportion of Black or Hispanic residents are associated with higher poverty due to a lack in community investment and built environments (i.e., fast food restaurants, liquor stores, lack of green space) which decrease the opportunities for healthy eating and exercises (15). Disadvantaged neighborhood conditions (e.g., high crime rate, poor community support, collective efficacy or social capital) have shown elevated mortality through the mechanisms of practicing health behaviors (16).
The main objective of this study was to summarize the evidence of racial/ethnic disparities in health outcomes for survivors of childhood cancer based on a scoping review of previously published literature. We focused on race/ethnicity as the primary variable determining disparities in health outcomes, and viewed SES factors as confounding or mediating variables that explain the associations between race/ethnicity and health outcomes. This is because the vast majority of the studies selected are based on the cross-sectional design, and the true effect of SES factors on health outcomes cannot be determined (e.g., survivors having lower incomes may develop worse chronic health conditions, and worse health conditions may further lower survivors' incomes). Specifically, we aimed to elucidate the role that personal/family/community-level SES factors alongside other demographic and clinical factors might play to explain the associations between race/ethnicity and health outcomes. Based on these findings, we made recommendations toward improving health disparities for minority childhood cancer survivors, especially by identifying modifiable social determinants of health using information technology, integrating social determinant information into healthcare systems, and suggesting potential interventions for health outcomes improvement.
In line with our aims, a scoping review was performed to aggregate evidence from empirical studies. Scoping reviews are particularly useful for complex/diverse issues (17) such as race/ethnicity, and generally precede systematic and meta-analyses (18).
We performed a literature search process according to the Preferred Reporting Items for Systematic Reviews and Meta-Analyses Extension for Scoping Reviews (PRISMA-ScR) (19). Two independent investigators researched the title and abstract for articles published between inception and February 2021 in the PubMed and Scopus using the terms “race”, “ethnicity”, “childhood cancer”, “pediatric cancer”, and “survivor”. In addition, the search was limited to articles published in the English language and available in full-text. The initial search yielded 26 articles from PubMed and 173 articles from Scopus. After removing duplicates, a combined total of 189 articles were prepared for screening.
Two independent investigators screened the articles for inclusion if these articles included the following criteria: health disparity (i.e. difference in outcome based on race/ethnicity), health outcomes/late effects, and any age range of the survivorship stage. We excluded articles if they met the following criteria: not reporting health outcomes/late effects (90 articles), race/ethnicity listed but only in descriptive statistics (64 articles), no full-text available (4 articles), and absence of IRB approval or otherwise proof of rigor (e.g., qualitative, opinion, review, briefs, and meta-analysis; 6 articles). In addition, two Swiss articles were removed from subsequent review because they did not include non-Hispanic Black or Hispanic survivors. We included two articles that use the term “non-White” (i.e., a combined concept for non-Hispanic Black and Hispanic) into our review.
We extracted data from each article according to the study design, race/ethnicity, health outcomes, and risk modulators of racial/ethnic disparity in health outcomes. We focused on marginalized/minoritized (20). US categories of non-Hispanic Black and Hispanic, and reported its association with health disparity.
We classified health outcomes of childhood cancer survivors by four distinct categories: (1) healthcare utilization, (2) patient-reported outcomes, (3) chronic health conditions, and (4) survival rates. For counting health outcomes of interest, if studies reported outcomes in more than one category, these studies were listed in different, separate outcome categories. Healthcare utilization outcomes included the concept of healthcare self-efficacy, initial and follow-up care visits, contact with general or cancer-specific healthcare providers, and use of hospital services. Patient-reported outcomes included the concept of health-related quality of life, symptom presence or severity, adaptive functioning, and post-traumatic stress. Chronic health conditions represented individual health conditions (e.g., diabetes) or organ system-based condition groups (e.g., endocrine). Survival outcomes were categorized as all-cause or condition-specific survival rates. In addition, we reported the factors used to explain racial/ethnic disparities in health outcomes per the rationale of the articles or statistical modeling process (e.g., the mediating effects from the path analysis and covariate-adjustment or interaction effects from the standard regression models).
Figure 1 presents the PRISMA flow diagram for the process of article selection. Of 185 full-text articles initially identified, 23 articles which met the inclusion/exclusion criteria were selected into full review and data extraction. Table 1 summarizes the characteristics of the 23 selected articles, published between 2002 and 2020. All study populations included in the 23 articles were from North America. A majority (52%) of the selected articles were based on the US National Cancer Institute-funded Childhood Cancer Survivorship Study or the US Surveillance, Epidemiology, and End Results program (SEER). The size of the samples ranged from under 100 (5 articles) to over 10,000 (6 articles). The age range of survivors included in the 23 articles varied from adolescents (7 articles) and young adults (5 articles) to adults (5 articles) or all ages (6 articles). The distribution of race/ethnicity was calculated and presented as percentage with non-Hispanic White as the reference group (see Table 1). The average percentages were 6.9% for non-Hispanic Black and 4.5% for Hispanic, which were smaller compared to 13.4% for non-Hispanic Black and 18.5% for Hispanic in the general US population (45). Data abstracted from the selected studies were all cross-sectional in nature. A variety of statistical techniques were used to test the statistical difference and suggest the influential factors. The most used methods included multivariate modeling (13 articles) and ratio-based models [e.g., odds ratio (3 articles), proportional hazards (2 articles), or standardized ration (3 articles)]; other methods included analysis of covariance and flexible parameters model.
Figure 1. PRISMA diagram of study selection. Adapted from (21).
Five articles reported racial/ethnic disparities in healthcare utilization (Table 2). The type of healthcare utilization disparities reported for non-Hispanic Black survivors included general medical contact (26) and an initial survivorship visit (28). The type of healthcare utilization disparities reported for Hispanic survivors included general medical contact (26), a cancer center visit (26), the use of follow-up care (35), health-care self-efficacy, defined as perceived control and confidence in managing healthcare (36), and seeking information from a hospital (37) or from family members (37). Across the articles, risk modulators included in analytic models for healthcare utilization disparities included clinical factors (diagnosis, treatment), individual characteristics (age, sex, depressive symptoms, post-traumatic growth, self-efficacy), individual-/family-level SES (educational attainment, household income, health insurance coverage), and social/contextual factors (support, family influence).
Table 2. Factors influencing disparities in healthcare utilization for childhood cancer survivors by race/ethnicity.
In two articles, inclusion of individual characteristics and individual-/family-level SES in multivariable modeling attenuated the statistical significance for racial/ethnic disparities in healthcare utilization. Specifically, in an odds-ratio model, inclusion of individual-level and family-level SES and individual characteristics (cancer diagnosis and age at the time of study) removed statistical significance of the disparity in general medical contact among non-Hispanic Black male survivors (26). Similarly, inclusion of age, sex, and individual-level SES (i.e., health insurance) removed the statistical significance for receiving less cancer-related information from family members among Hispanic survivors (37). However, two articles found significant racial/ethnic disparity after adjusting for individual characteristics and social contextual factors in the multivariable analyses. Specifically, inclusion of cancer treatment, age, sex, social support, family influence, post-traumatic growth, depressive symptoms, and self-efficacy factors revealed significantly fewer previous receipt of follow-up care among Hispanic survivors compared to non-Hispanic White survivors (35). In addition, inclusion of cancer treatment, age, sex, social support, family influence, post-traumatic growth, and depressive symptoms factors revealed significantly lower healthcare self-efficacy for Hispanic survivors compared to non-Hispanic White survivors (36).
Nine articles have reported racial/ethnic disparities in patient-reported outcomes (Table 3). The types of patient-reported outcomes assessed for non-Hispanic Black survivors included quality-of-life (24), adverse mental health (26), functional impairment (26), pain or abnormal sensations (32), migraines (32), frequent headaches (32) and parental uncertainty about the child's health (41). The types of patient-reported outcomes assessed for Hispanic survivors included post-traumatic growth (23, 42), psychosocial health (33), quality-of-life (33), school functioning (33), emotional functioning (33), parental post-traumatic stress (34), depression (34), and conceptual, social and practical adaptive functioning (39), pain or abnormal sensations (32), and frequent headaches (32). In addition, the types of patient-report outcomes assessed for non-White survivors included psychosocial functioning (33), quality-of-life (33), school functioning (33), and positive impact of cancer (44). Across the articles, risk modulators included in multivariable modeling comprised clinical factors (diagnosis, treatment), individual characteristics (age, sex, depressive symptoms, post-traumatic stress, optimism, fatigue), individual-/family-level SES (educational attainment, health insurance, household income, parent educational attainment), and social/contextual factors (birthplace, language spoken at home).
Table 3. Factors influencing disparities in patient-reported outcomes for childhood cancer survivors by race/ethnicity.
In two articles, inclusion of individual characteristics and SES in multivariable modeling attenuated the significance for racial/ethnic disparities in patient-reported outcomes. Specifically, inclusion of cancer diagnosis, individual-level SES and age at study participation removed the significance for adverse mental health outcomes among non-Hispanic Black females (26). Similarly, inclusion of family-level SES removed the significance for poor global, conceptual, social, and practical adaptive functioning in Hispanic survivors (39). However, one article found that after adjusting for cancer diagnosis and fatigue symptoms, poorer psychosocial functioning and quality-of-life in minority (both non-Hispanic Black and Hispanic) survivors vs. non-Hispanic White survivors remained statistically significant (33).
Five articles reported racial/ethnic disparities in chronic health conditions (Table 4). The type of chronic health condition disparities assessed for non-Hispanic Black survivors included vitamin-D deficiency (27), subsequent neoplasms (31), cardiovascular disorders (31), cardiovascular risks (38), and serious/life-threatening health conditions (43). The type of chronic health condition disparities assessed for Hispanic survivors included vitamin-D deficiency (27), obesity (29), subsequent neoplasm (31), endocrine condition (31). Across the articles, risk modulators included in multivariable modeling included clinical factors (diagnosis, treatment), individual characteristics (age, sex, pubertal status), individual-level SES (educational attainment, income, health insurance), family-level SES (parent educational attainment, household income), and lifestyle health risk for chronic health conditions (BMI and cardiovascular risk factors including obesity, diabetes, hypertension and dyslipidemia).
Table 4. Factors influencing disparities in chronic health conditions for childhood cancer survivors by race/ethnicity.
In two articles, inclusion of clinical factors, individual characteristics, and SES in the modeling attenuated the significance for racial/ethnic disparities in chronic health condition. Specifically, one article found that inclusion of clinical factors, individual characteristics, and SES factors removed the significance of disparity in subsequent neoplasms for non-Hispanic Black survivors (31). Another article suggested that inclusion of clinical and demographic factors removed the significance of the disparity in serious/life-threatening health conditions for non-Hispanic Black survivors (43). However, one article found that disparities in subsequent neoplasms and cardiovascular disorders remained significant for non-Hispanic Black survivors and disparities in subsequent neoplasms and endocrine disorders remained significant for Hispanic survivors after adjusting for clinical, cardiovascular risk, and/or individual SES factors in the modeling (31).
Five articles reported racial/ethnic disparities in survival rates (Table 5). Type of survival outcomes assessed for non-Hispanic Black survivors included all-cause mortality (22), all-cause mortality including relative and standardized rates (31), subsequent malignancy mortality (22), risk of cardiovascular-specific death (25), and risk of any death (25). Types of survival metrics assessed for Hispanic survivors included all-cause standardized mortality rates (31). Type of survival metrics assessed for non-White survivors included hazard of death for survivors diagnosed with acute myeloid leukemia, astrocytoma, and non-astrocytoma CNS tumors (30) and mortality to incidence ratios (40). Across the articles, modulators included in multivariable modeling included clinical factors (time since cancer diagnosis, age at cancer diagnosis, cancer type), individual characteristics (age, sex), SES (educational attainment, income, health insurance), lifestyle health risk (cardiovascular risk factors such as obesity, diabetes, hypertension, dyslipidemia), neighborhood factors (census-track SES Index), and US national mortality rate (for the purpose of mortality standardization).
Table 5. Factors influencing disparities in survival for childhood cancer survivors by race/ethnicity.
In two articles, inclusion of clinical and SES factors attenuated the significance for racial/ethnic disparities in survival outcomes. Specifically, one article found that inclusion of census-tract (i.e., neighborhood-level) SES removed the significance of death hazard for non-Hispanic Black survivors diagnosed with astrocytoma and non-astrocytoma CNS tumor, but not acute myeloid leukemia (30). Another article suggests that the adjustment individual and clinical factors removed the significance of cardiovascular-specific death for non-Hispanic Black survivors (25). However, another article found that disparities in all-cause relative mortality rates remained statistically significant for non-Hispanic Black and Hispanic survivors after adjusting for clinical factors, individual demographic and SES factors, and cardiovascular risk in the modeling (31). In addition, based on a path analysis focusing neighborhood socioeconomic determinants as the mediator, one article found significantly higher death hazard among non-White survivors of acute myeloid leukemia compared to non-Hispanic White survivors (30).
Compared to non-Hispanic White, non-Hispanic Black and Hispanic childhood cancer survivors suffer more from poorer health outcomes including healthcare utilization, patient-reported outcomes, chronic health conditions and survival rate. While there is an effect of race/ethnicity on health outcomes for childhood cancer survivors; there is not yet enough evidence to determine the true effect of SES across all outcomes given the cross-sectional design of previous studies. The current findings do show that embedded in race and ethnicity are a multitude of factors at the clinical (e.g., disease, treatment), individual (e.g., demographic, SES), and neighborhood (e.g., community SES) levels that may explain some of the disparities and poor health outcomes. However, the magnitude of racial/ethnic disparities changed in some but not all studies after adjusting for these risk modulators. As such, we see a complex interplay among these risk factors for health disparities. Future research is warranted to elucidate the complex associations between racial/ethnic and SES factors and health outcomes for childhood cancer survivors.
Potential risk modulators that explained the associations between race/ethnicity and health outcomes attempted in all articles were reviewed. Risk modulators commonly reported for healthcare utilization disparity included individual-level SES (26, 28). Social support and religious importance (35–37) also explained aspects of the racial/ethnic disparities. In patient-reported outcomes, risk modulators for racial/ethnic disparities included family-level SES (23, 24, 26, 39), family dynamics (34, 42), and treatment factors (33). Particularly for Hispanic survivors, family dynamics (e.g., language spoken at home) should be further investigated as they are potentially protective factors for poor patient-reported outcomes. In chronic health conditions, most articles found that racial/ethnic disparities remained statistically significant after risk modulators (e.g., clinical factors, individual demographic and SES factors, and cardiovascular risk factors) were included in the multivariable modeling (27, 29, 31, 38, 43). It is possible that underlying biological mechanisms (e.g., inherited genetic predisposition to disease risks and epigenetic modifications due to life experiences or environmental exposures) and disadvantaged neighborhood environments may elevate disparities in chronic health conditions beyond the influence of individual SES and clinical risk. In survival rate, individual-level and neighborhood-level SES (22, 30, 31), together with age at cancer diagnosis (25, 39) and years since diagnosis, played an important role for risk facilitation (26).
Bhatia et al. argues that the “burden of morbidity and mortality [between races] is comparable because mortality is mitigated by SES” (12, 46); however, our findings suggest that the effect of SES on disparities is less straightforward. In fact, individual-level, family-level, and neighborhood-level variables may have distinct impacts on health disparities. We found that adjustment for SES increased the magnitude of disparities in some patient-reported outcomes (i.e. adverse mental health for Black females, post-traumatic stress for Hispanic parents) rather than mitigated them (23, 26). Furthermore, various sources and datasets used to quantify SES risk modulators in the analysis across studies may complicate the interpretations of findings. While the majority of the articles in our review used individual-level SES or family-level SES), one article used a validated composite SES index with seven specific indicators (proportion employed in working class occupations, proportion over 16 employed, education index, median household income, proportion below 200% poverty level, median rent and median house value) (30, 47) to capture the complex influences of different levels of SES. However, very few selected articles included neighborhood contextual factors in the analysis. In fact, neighborhood-level factors such as the built environment (e.g., green space) (48), accessibility to healthy food (49), and healthcare services (50) are increasingly considered key determinants of health outcomes for adult-onset cancer but not for pediatric cancer research. In addition, race/ethnicity-sensitive indices warrant consideration including crime-rate, incarceration, and residential segregation. The use of geospatial neighborhood metrics may provide useful information for understanding disparities in health outcomes thereby offering a more complete depiction of health disparities for childhood cancer survivors.
In addition to improving SES measurement for childhood cancer research, it is important to use a holistic and life-course approach to investigating risk of health disparities. Williams (4, 51) suggests that race is an antecedent for SES instead of a variable inside, and embedded in race and ethnicity are layered factors that may be inextricably linked. Geronimus et al., suggest the burden of physiological stress (i.e. allostatic load) of race, ethnicity, and low SES can accumulate over time (52), which in turn may link to health disparities in underserved minority breast cancer (53, 54) and general (54) populations. Cultural and familial factors can influence the impact of allostatic load (54, 55) which may explain the risk for poor patient-reported outcomes in minority survivors. Krieger (56) suggested a federal mandate to include and categorize individual-level data pertinent to racialized societal inequities and explicit justification of metrics used to categorize racial groups. Therefore, in addition to standard SES variables, the design and collection of standardized race/ethnic-specific risk modulators for childhood cancer survivors are needed.
Risk modulators that substantially impact health outcomes of individual childhood cancer survivors were SES, healthcare accessibility, and health insurance. Several studies suggested that neighborhood-level SES (30), individual-level SES (26, 31) and/or family-level SES (26, 39) plays a more significant role as compared to health insurance in explaining the effects of race/ethnicity on poor health outcomes in childhood cancer survivors. In fact, a population-based study found that improving health insurance coverage alone may disproportionately benefit non-Hispanic White with lower SES rather than racial/ethnic minorities (57, 58). A more inclusive, need-based financial assistance program for individual survivors should be considered for minority survivors to reduce the risk of health disparities. In addition, the first two to three years from cancer diagnosis (25, 38, 43) and primary caregiver education background and proximity/access to care (39) were associated with elevated risk of health disparities in minority childhood cancer survivors. Therefore, healthcare systems should assess the disparity status for minority childhood cancer survivors immediately following completion of therapy and provide social support or resources to address these issues (e.g., coordinating transportation aids for minority families) toward improving follow-up care and reducing disease burden.
Our findings highlight that individual-level factors, such as culture (28, 33, 34, 37) and sex (26, 28) may contribute to racial/ethnic disparities in health outcomes. Cultural beliefs (i.e. fatalism) and gender beliefs seem relevant to health disparities in non-Hispanic Black survivors (26), while family dynamics, such as foreign-born parents experiencing greater amounts of post-traumatic stress, may impact Hispanic survivors (34, 59). In addition, minority childhood cancer survivors who had better social skills (27) and post-traumatic growth (31) were associated with better health outcomes. Therefore, it is critical to provide culture-/race-/ethnicity-/gender-specific social and emotional learning (i.e. stress prevention) interventions and diversity informed training for healthcare navigators (i.e. social workers, hospital staff, researchers, etc.). Social and emotional learning interventions that acknowledge established race-/gender-related stigma are avenues to augment resilience and provide social support and belonging.
There is an opportunity to leverage health information technology to promote health equity for minority and underserved populations (60, 61). Emerging evidence has found that the use of eHealth and mHealth platforms can improve physiological and psychological well-being, health knowledge, and self-management skill in racial/ethnic minorities and underserved populations (62). Given the importance of visiting oncologists/primary physicians for follow-up care and maintaining healthy lifestyle among childhood cancer survivors, mHealth and eHealth technology represent the methods that may improve access to medical care (e.g., telemedicine consultation and remote lifestyle and psychological interventions), communication with healthcare providers (e.g., digital therapy and education, tailored supportive resources), and symptom monitoring and management (e.g., real-time symptom monitoring for identifying early signs of late effects) (62). However, the vast majority of current eHealth and mHealth applications are designed in the English language. Future efforts are warranted to ensure the provision of technology platforms that are multilingual and culturally and literately appropriate.
Improving medical informatics infrastructure within healthcare systems can facilitate the collection and assessment of social determinants of health data for cancer survivors on a regular-basis and integrate social determinant information into clinical decision-making process. Incorporating neighborhood-/community-level social determinant data into electronic health records (EHRs) will allow clinicians to provide tailored interventions that are clinically actionable based on the survivors' need and contextual influence. Given the big data stored in EHRs, the use of artificial intelligence analytics (e.g., machine learning and natural processing techniques) can help identify complex social determinants for individual minority survivors. Recent evidence suggests that implementation of machine learning approaches helps identify the patterns of social determinants for impaired health outcomes with superior performance compared to the use of traditional analytics (63).
While this scoping review provides useful information for racial/ethnic disparities in health outcomes among childhood cancer survivors, the findings should be carefully interpreted. First, race/ethnicity data from all articles were self-reported. Self-reported race/ethnicity information is often arbitrary and poorly defined (16). Furthermore, based on the available data included in the articles, we only focused on two traditionally minoritized/marginalized groups and excluded other non-White minority groups from our review. For example, American Indian childhood cancer survivors with acute lymphoblastic leukemia have lower survival rates compared to other races/ethnicities (46). Second, characteristics and patients of the survivor populations included in our review were generally homogenous. As the majority of selected studies were derived from the US-based Childhood Cancer Survivorship Study or the Surveillance Epidemiology and End Results registry, health outcome data are likely to overlap in time, collection, and patients. As mentioned in the beginning of the Results section, the percentage of minority survivors in the selected study was far lower than the percentage of the US general population. Finally, this scoping review focused on non-Hispanic Black and Hispanic health disparities, which is an emerging topic supported by current research on minoritized populations (20). In fact, the studies selected into our review did not breakdown race/ethnicity into categories beyond the three minoritized categories reported. Some articles just reported White and Other. It is critical to evaluate health disparities across more categories and intersections of races and ethnicities in the future research. It is also important to use a community-based, culture-specific participatory research design to recruit and engage racial/ethnic minorities to in childhood cancer survivorship research for better understanding the gap while also elucidating clinical interventions (64).
The raw data supporting the conclusions of this article will be made available by the authors, without undue reservation.
JB and I-CH: concept and design. MH and LR: administrative support. TR and TM: collection and assembly of data. TR, TM, and I-CH: data analysis and interpretation. TR and I-CH: manuscript writing. All authors editing and final approval of manuscript. All authors contributed to the article and approved the submitted version.
The research reported in this manuscript was supported by the National Cancer Institute under award number R01CA238368 (MPIs: I-CH, JB) and V-Foundation Robin Roberts Cancer Thrivership Fund under award number DT2020-014 (MPIs: ZW, I-CH). The content is solely the responsibility of the authors and does not necessarily represent the official views of the funding agencies.
The authors declare that the research was conducted in the absence of any commercial or financial relationships that could be construed as a potential conflict of interest.
All claims expressed in this article are solely those of the authors and do not necessarily represent those of their affiliated organizations, or those of the publisher, the editors and the reviewers. Any product that may be evaluated in this article, or claim that may be made by its manufacturer, is not guaranteed or endorsed by the publisher.
1. US Census Bureau. Methodology, Assumptions, and Inputs for the 2017 National Population Projections. Washington (2018). Available online at: https://www2.census.gov/programs-surveys/popproj/technical-documentation/methodology/methodstatement17.pdf (accessed September 30, 2021).
2. US Census Bureau. American Community Survey demographic and housing estimates. Washington (2020). Available online at: https://www.census.gov/programs-surveys/acs/data.html (accessed September 30, 2021).
3. Ford CL, Airhihenbuwa CO. The public health critical race methodology: praxis for antiracism research. Soc Sci Med. (2010) 71:1390–8. doi: 10.1016/j.socscimed.2010.07.030
4. Williams DR. Race, socioeconomic status, and health. The added effects of racism and discrimination. Ann N Y Acad Sci. (1999) 896:173–88. doi: 10.1111/j.1749-6632.1999.tb08114.x
5. American Association for Cancer Research. Cancer Progress Report. Philidelphia (2020). Available online at: https://cancerprogressreport.aacr.org/progress/ (accessed September 30, 2021).
6. Heron M. Deaths: leading causes for 2017. Natl Vital Stat Rep. (2019) 68:1–77. Available online at: https://www.cdc.gov/nchs/data/nvsr/nvsr68/nvsr68_06-508.pdf
7. Viale PH. The American cancer society's facts & figures: 2020 edition. J Adv Pract Oncol. (2020) 11:135–6. doi: 10.6004/jadpro.2020.11.2.1
8. Huang IC, Ehrhardt MJ LI C, Mulrooney DA, Chamaitilly W, Srivastava D, et al. Longitudinal assessment of patient-reported cumulative symptom burden as an indicator of chronic health conditions in adult survivors of childhood cancer: a joint report of the St. Jude Lifetime Cohort (SJLIFE) and the Childhood Cancer Survivor Study (CCSS). J Clin Oncol. (2018) 36:10571. doi: 10.1200/JCO.2018.36.15_suppl.10571
9. Dixon SB Li N, Yasui Y, Bhatia S, Casillas JN, Gibson TM, et al. Racial and ethnic disparities in neurocognitive, emotional, and quality-of-life outcomes in survivors of childhood cancer: a report from the Childhood Cancer Survivor Study. Cancer. (2019) 125:3666–77. doi: 10.1002/cncr.32370
10. Robison LL, Hudson MM. Survivors of childhood and adolescent cancer: life-long risks and responsibilities. Nat Rev Cancer. (2014) 14:61–70. doi: 10.1038/nrc3634
11. Nolan VG, Krull KR, Gurney JG, Leisenring W, Robison LL, Ness KK. Predictors of future health-related quality of life in survivors of adolescent cancer. Pediatr Blood Cancer. (2014) 61:1891–4. doi: 10.1002/pbc.25037
12. Bhatia S, Gibson TM, Ness KK, Liu Q, Oeffinger KC, Krull KR, et al. Childhood cancer survivorship research in minority populations: a position paper from the Childhood Cancer Survivor Study. Cancer. (2016) 122:2426–39. doi: 10.1002/cncr.30072
13. Egede LE. Race, ethnicity, culture, and disparities in health care. J Gen Intern Med. (2006) 21:667–9. doi: 10.1111/j.1525-1497.2006.0512.x
14. Caplin DA, Smith KR, Ness KK, Hanson HA, Smith SM, Nathan PC, et al. Effect of population socioeconomic and health system factors on medical care of childhood cancer survivors: a report from the childhood cancer survivor study. J Adolesc Young Adult Oncol. (2017) 6:74–82. doi: 10.1089/jayao.2016.0016
15. Williams DR, Sternthal M. Understanding racial-ethnic disparities in health: sociological contributions. J Health Soc Behav. (2010) 51:S15–27. doi: 10.1177/0022146510383838
16. Gomez SL, Shariff-Marco S, DeRouen M, Keegan TH, Yen IH, Mujahid M, et al. The impact of neighborhood social and built environment factors across the cancer continuum: current research, methodological considerations, and future directions. Cancer. (2015) 121:2314–30. doi: 10.1002/cncr.29345
17. Peters MD, Godfrey CM, Khalil H, McInerney P, Parker D, Soares CB. Guidance for conducting systematic scoping reviews. Int J Evid Based Healthc. (2015) 13:141–6. doi: 10.1097/XEB.0000000000000050
18. Sucharew H, Macaluso M. Progress notes: methods for research evidence synthesis: the scoping review approach. J Hosp Med. (2019) 14:416–8. doi: 10.12788/jhm.3248
19. Page MJ, McKenzie JE, Bossuyt PM, Boutron I, Hoffmann TC, Mulrow CD, et al. The PRISMA 2020 statement: an updated guideline for reporting systematic reviews. BMJ. (2021) 372:n71. doi: 10.1136/bmj.n71
20. Brown LA, Strega S. Research as Resistance, 2e: Revisiting Critical, Indigenous, and Anti-Oppressive Approaches. Canadian Scholars' Press (2015).
21. Moher D, Liberati A, Tetzlaff J, Altman DG, The PRISMA Group. Preferred reporting items for systematic reviews and meta-analyses: the PRISMA statement. PLoS Med. (2009) 6:e1000097.
22. Armstrong GT, Pan Z, Ness KK, Srivastava D, Robison LL. Temporal trends in cause-specific late mortality among 5-year survivors of childhood cancer. J Clin Oncol. (2010) 28:1224–31. doi: 10.1200/JCO.2009.24.4608
23. Arpawong TE, Oland A, Milam JE, Ruccione K, Meeske KA. Post-traumatic growth among an ethnically diverse sample of adolescent and young adult cancer survivors. Psychooncology. (2013) 22:2235–44. doi: 10.1002/pon.3286
24. Barrera M, Atenafu EG, Schulte F, Bartels U, Sung L, Janzen L, et al. Determinants of quality of life outcomes for survivors of pediatric brain tumors. Pediatr Blood Cancer. (2017) 64:e26481. doi: 10.1002/pbc.26481
25. Berkman AM, Brewster AM, Jones LW Yu J, Lee JJ, Peng SA, et al. Racial differences in 20-year cardiovascular mortality risk among childhood and young adult cancer survivors. J Adolesc Young Adult Oncol. (2017) 6:414–21. doi: 10.1089/jayao.2017.0024
26. Castellino SM, Casillas J, Hudson MM, Mertens AC, Whitton J, Brooks SL, et al. Minority adult survivors of childhood cancer: a comparison of long-term outcomes, health care utilization, and health-related behaviors from the childhood cancer survivor study. J Clin Oncol. (2005) 23:6499–507. doi: 10.1200/JCO.2005.11.098
27. Choudhary A, Chou J, Heller G, Sklar C. Prevalence of vitamin D insufficiency in survivors of childhood cancer. Pediatr Blood Cancer. (2013) 60:1237–9. doi: 10.1002/pbc.24403
28. Daly A, Lewis RW, Vangile K, Masker KW, Effinger KE, Meacham LR, et al. Survivor clinic attendance among pediatric- and adolescent-aged survivors of childhood cancer. J Cancer Surviv. (2019) 13:56–65. doi: 10.1007/s11764-018-0727-3
29. Gance-Cleveland B, Linton A, Arbet J, Stiller D, Sylvain G. Predictors of overweight and obesity in childhood cancer survivors. J Pediatr Oncol Nurs. (2020) 37:154–62. doi: 10.1177/1043454219897102
30. Kehm RD, Spector LG, Poynter JN, Vock DM, Altekruse SF, Osypuk TL. Does socioeconomic status account for racial and ethnic disparities in childhood cancer survival? Cancer. (2018) 124:4090–7. doi: 10.1002/cncr.31560
31. Liu Q, Leisenring WM, Ness KK, Robison LL, Armstrong GT, Yasui Y, et al. Racial/ethnic differences in adverse outcomes among childhood cancer survivors: the childhood cancer survivor study. J Clin Oncol. (2016) 34:1634–43. doi: 10.1200/JCO.2015.66.3567
32. Lu Q, Krull KR, Leisenring W, Owen JE, Kawashima T, Tsao JCI, et al. Pain in long-term adult survivors of childhood cancers and their siblings: a report from the Childhood Cancer Survivor Study. Pain. (2011) 152:2616–24. doi: 10.1016/j.pain.2011.08.006
33. Meeske KA, Patel SK, Palmer SN, Nelson MB, Parow AM. Factors associated with health-related quality of life in pediatric cancer survivors. Pediatr Blood Cancer. (2007) 49:298–305. doi: 10.1002/pbc.20923
34. Meeske KA, Sherman-Bien S, Hamilton AS, Olson AR, Slaughter R, Kuperberg A, et al. Mental health disparities between Hispanic and non-Hispanic parents of childhood cancer survivors. Pediatr Blood Cancer. (2013) 60:1470–7. doi: 10.1002/pbc.24527
35. Milam JE, Meeske K, Slaughter RI, Sherman-Bien S, Ritt-Olson A, Kuperberg A, et al. Cancer-related follow-up care among Hispanic and non-Hispanic childhood cancer survivors: the Project Forward study. Cancer. (2015) 121:605–13. doi: 10.1002/cncr.29105
36. Miller KA, Wojcik KY, Ramirez CN, Ritt-Olson A, Freyer DR, Hamilton AS, et al. Supporting long-term follow-up of young adult survivors of childhood cancer: correlates of healthcare self-efficacy. Pediatr Blood Cancer. (2017) 64:358–63. doi: 10.1002/pbc.26209
37. Miller KA, Ramirez CN, Wojcik KY, Ritt-Olson A, Baezconde-Garbanati L, Thomas SM, et al. Prevalence and correlates of health information-seeking among Hispanic and non-Hispanic childhood cancer survivors. Support Care Cancer. (2018) 26:1305–13. doi: 10.1007/s00520-017-3956-5
38. Oikonomou EK, Athanasopoulou SG, Kampaktsis PN, Kokkinidis DG, Papanastasiou CA, Feher A, et al. Development and validation of a clinical score for cardiovascular risk stratification of long-term childhood cancer survivors. Oncologist. (2018) 23:965–73. doi: 10.1634/theoncologist.2017-0502
39. Raghubar KP, Orobio J, Ris MD, Heitzer AM, Roth A, Brown AL, et al. Adaptive functioning in pediatric brain tumor survivors: An examination of ethnicity and socioeconomic status. Pediatr Blood Cancer. (2019) 66:e27800. doi: 10.1002/pbc.27800
40. Samaan MC, Akhtar-Danesh N. The impact of age and race on longevity in pediatric astrocytic tumors: a population-based study. Pediatr Blood Cancer. (2015) 62:1567–71. doi: 10.1002/pbc.25522
41. Santacroce S. Uncertainty, anxiety, and symptoms of posttraumatic stress in parents of children recently diagnosed with cancer. J Pediatr Oncol Nurs. (2002) 19:104–11. doi: 10.1177/104345420201900305
42. Tobin J, Allem JP, Slaughter R, Unger JB, Hamilton AS, Milam JE. Posttraumatic growth among childhood cancer survivors: associations with ethnicity, acculturation, and religious service attendance. J Psychosoc Oncol. (2018) 36:175–88. doi: 10.1080/07347332.2017.1365799
43. Wasilewski-Masker K, Mertens AC, Patterson B, Meacham LR. Severity of health conditions identified in a pediatric cancer survivor program. Pediatr Blood Cancer. (2010) 54:976–82. doi: 10.1002/pbc.22431
44. Zebrack BJ, Stuber ML, Meeske KA, Phipps S, Krull KR, Liu Q, et al. Perceived positive impact of cancer among long-term survivors of childhood cancer: a report from the childhood cancer survivor study. Psychooncology. (2012) 21:630–9. doi: 10.1002/pon.1959
45. US Census Bureau. Quick Facts: Race and Hispanic Origin. Washington (2020). Available online at: https://www.census.gov/quickfacts/fact/table/US/PST045219 (accessed September 30, 2021).
46. Bhatia S. Disparities in cancer outcomes: lessons learned from children with cancer. Pediatr Blood Cancer. (2011) 56:994–1002. doi: 10.1002/pbc.23078
47. Yu M, Tatalovich Z, Gibson JT, Cronin KA. Using a composite index of socioeconomic status to investigate health disparities while protecting the confidentiality of cancer registry data. Cancer Causes Control. (2014) 25:81–92. doi: 10.1007/s10552-013-0310-1
48. Kish JK Yu M, Percy-Laurry A, Altekruse SF. Racial and ethnic disparities in cancer survival by neighborhood socioeconomic status in Surveillance, Epidemiology, and End Results (SEER) Registries. J Natl Cancer Inst Monogr. (2014) 2014:236–43. doi: 10.1093/jncimonographs/lgu020
49. Richardson LD, Norris M. Access to health and health care: how race and ethnicity matter. Mt Sinai J Med. (2010) 77:166–77. doi: 10.1002/msj.20174
50. Syed ST, Gerber BS, Sharp LK. Traveling towards disease: transportation barriers to health care access. J Community Health. (2013) 38:976–93. doi: 10.1007/s10900-013-9681-1
51. Williams DR. Race/ethnicity and socioeconomic status: measurement and methodological issues. Int J Health Serv. (1996) 26:483–505. doi: 10.2190/U9QT-7B7Y-HQ15-JT14
52. Geronimus AT, Hicken M, Keene D, Bound J. “Weathering” and age patterns of allostatic load scores among blacks and whites in the United States. Am J Public Health. (2006) 96:826–33. doi: 10.2105/AJPH.2004.060749
53. Linnenbringer E, Gehlert S, Geronimus AT. Black-white disparities in breast cancer subtype: the intersection of socially patterned stress and genetic expression. AIMS Public Health. (2017) 4:526–56. doi: 10.3934/publichealth.2017.5.526
54. Peek MK, Cutchin MP, Salinas JJ, Sheffield KM, Eschbach K, Stowe RP, et al. Allostatic load among non-Hispanic Whites, non-Hispanic Blacks, and people of Mexican origin: effects of ethnicity, nativity, and acculturation. Am J Public Health. (2010) 100:940–6. doi: 10.2105/AJPH.2007.129312
55. Maguire-Jack K, Lanier P, Lombardi B. Investigating racial differences in clusters of adverse childhood experiences. Amn J Orthopsychiatry. (2020) 90:106–14. doi: 10.1037/ort0000405
56. Krieger N. Structural racism, health inequities, and the two-edged sword of data: structural problems require structural solutions. Front Public Health. (2021) 9:301. doi: 10.3389/fpubh.2021.655447
57. Manuel JI. Racial/ethnic and gender disparities in health care use and access. Health Serv Res. (2018) 53:1407–29. doi: 10.1111/1475-6773.12705
58. Callison K, Nguyen BT. The effect of medicaid physician fee increases on health care access, utilization, and expenditures. Health Serv Res. (2018) 53:690–710. doi: 10.1111/1475-6773.12698
59. Tobin J, Miller KA, Baezconde-Garbanati L, Unger JB, Hamilton AS, Milam JE. Acculturation, mental health, and quality of life among hispanic childhood cancer survivors: a latent class analysis. Ethn Dis. (2018) 28:55–60. doi: 10.18865/ed.28.1.55
60. Kruse CS, Beane A. Health information technology continues to show positive effect on medical outcomes: systematic review. J Med Internet Res. (2018) 20:e41. doi: 10.2196/jmir.8793
61. Clauser SB, Wagner EH, Aiello Bowles EJ, Tuzzio L, Greene SM. Improving modern cancer care through information technology. Am J Prev Med. (2011) 40:S198–207. doi: 10.1016/j.amepre.2011.01.014
62. Armaou M, Araviaki E, Musikanski L. eHealth and mHealth interventions for ethnic minority and historically underserved populations in developed countries: an umbrella review. Int J Community Well-Being. (2020) 3:193–221. doi: 10.1007/s42413-019-00055-5
63. Seligman B, Tuljapurkar S, Rehkopf D. Machine learning approaches to the social determinants of health in the health and retirement study. SSM Popul Health. (2018) 4:95–9. doi: 10.1016/j.ssmph.2017.11.008
64. Elk R, Emanuel L, Hauser J, Bakitas M, Levkoff S. Developing and testing the feasibility of a culturally based tele-palliative care consult based on the cultural values and preferences of southern, rural african american and white community members: a program by and for the community. Health Equity. (2020) 4:52–83. doi: 10.1089/heq.2019.0120
Keywords: childhood cancer survivor, ethnicity, health disparities, health outcomes, race
Citation: Reeves TJ, Mathis TJ, Bauer HE, Hudson MM, Robison LL, Wang Z, Baker JN and Huang I-C (2021) Racial and Ethnic Disparities in Health Outcomes Among Long-Term Survivors of Childhood Cancer: A Scoping Review. Front. Public Health 9:741334. doi: 10.3389/fpubh.2021.741334
Received: 15 July 2021; Accepted: 30 September 2021;
Published: 29 October 2021.
Edited by:
Yi Guo, University of Florida, United StatesReviewed by:
Xue Han, Bristol Myers Squibb, United StatesCopyright © 2021 Reeves, Mathis, Bauer, Hudson, Robison, Wang, Baker and Huang. This is an open-access article distributed under the terms of the Creative Commons Attribution License (CC BY). The use, distribution or reproduction in other forums is permitted, provided the original author(s) and the copyright owner(s) are credited and that the original publication in this journal is cited, in accordance with accepted academic practice. No use, distribution or reproduction is permitted which does not comply with these terms.
*Correspondence: I-Chan Huang, aS1jaGFuLmh1YW5nQHN0anVkZS5vcmc=
Disclaimer: All claims expressed in this article are solely those of the authors and do not necessarily represent those of their affiliated organizations, or those of the publisher, the editors and the reviewers. Any product that may be evaluated in this article or claim that may be made by its manufacturer is not guaranteed or endorsed by the publisher.
Research integrity at Frontiers
Learn more about the work of our research integrity team to safeguard the quality of each article we publish.