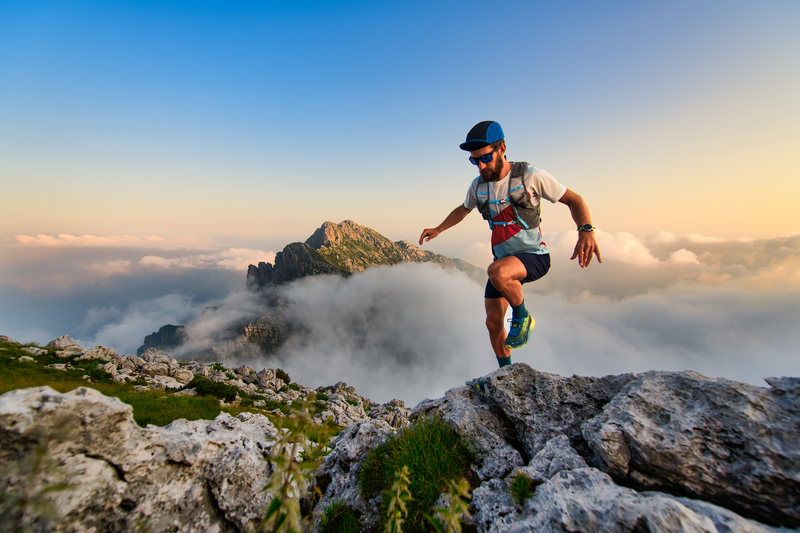
95% of researchers rate our articles as excellent or good
Learn more about the work of our research integrity team to safeguard the quality of each article we publish.
Find out more
ORIGINAL RESEARCH article
Front. Public Health , 23 December 2021
Sec. Life-Course Epidemiology and Social Inequalities in Health
Volume 9 - 2021 | https://doi.org/10.3389/fpubh.2021.731962
Background: Prior observational studies indicated that lower educational attainment (EA) is associated with higher COVID-19 risk, while these findings were vulnerable to bias from confounding factors. We aimed to clarify the causal effect of EA on COVID-19 susceptibility, hospitalization, and severity using Mendelian randomization (MR).
Methods: We identified genetic instruments for EA from a large genome-wide association study (GWAS) (n = 1,131,881). Summary statistics for COVID-19 susceptibility (112,612 cases and 2,474,079 controls), hospitalization (24,274 cases and 2,061,529 controls), and severity (8,779 cases and 1,001,875 controls) were obtained from the COVID-19 Host Genetics Initiative. We used the single-variable MR (SVMR) and the multivariable MR (MVMR) controlling intelligence, income, body mass index, vigorous physical activity, sedentary behavior, smoking, and alcohol consumption to estimate the total and direct effects of EA on COVID-19 outcomes. Inverse variance weighted was the primary analysis method. All the statistical analyses were performed using R software.
Results: Results from the SVMR showed that genetically predicted higher EA was correlated with a lower risk of COVID-19 susceptibility [odds ratio (OR) 0.86, 95% CI 0.84–0.89], hospitalization (OR 0.67, 95% CI 0.62–0.73), and severity (OR 0.67, 95% CI 0.58–0.79). EA still maintained its effects in most of the MVMR.
Conclusion: Educational attainment is a predictor for susceptibility, hospitalization, and severity of COVID-19 disease. Population with lower EA should be provided with a higher prioritization to public health resources to decrease the morbidity and mortality of COVID-19.
COVID-19 first emerged in December 2019 and has become a worldwide pandemic currently (1). At the time of April 18, 2021, there have been 140,821,384 confirmed cases and 3,013,042 deaths globally (2). In this condition, it is essential to identify high-risk groups that need special attention (3). Particularly, with the available but limited supply of COVID-19 vaccines, a crucial challenge is prioritizing groups to receive vaccines (4–6). There have been discussions about vaccine prioritization for racial minorities (4) and diabetes groups (7), since prior studies report these groups are more vulnerable to COVID-19 disease. In contrast, more evidence for other potential high-risk groups such as the population with low educational attainment (EA) is needed.
Educational attainment is a well-established social determinant of health (8) and correlates with many diseases (9–11). Prior observational studies indicated that the same might have happened during the current COVID-19 pandemic and a population with lower EA was found at a higher risk of susceptibility, hospitalization, and mortality of COVID-19 (12–14). However, conventional observational studies lacking randomization designs are generally prone to confounding factors (15). Randomized controlled trials, on the other hand, cannot be conducted.
Mendelian randomization (MR) is a method that uses genetic variants correlated with an exposure (such as EA) to evaluate whether it has a causal effect on the disease outcome (such as COVID-19 susceptibility) (16), which is less likely to be influenced by unmeasured confounding than observational studies (17). MR is especially useful for exploring causal pathways when the risk factors are difficult to randomize (18). There have been several MR studies exploring the risk factors for COVID-19 (18–20).
An extension of the single-variable MR (SVMR) is the multivariable MR (MVMR), which can incorporate genetic variants associated with several exposures into the same model (21). Since EA was identified to correlate with intelligence, income, body mass index (BMI), vigorous physical activity, sedentary behavior, smoking, and alcohol consumption in a prior study (22), we would also perform the paired MVMR to investigate the direct effects of EA when controlling these exposures separately and explore whether the effects of EA on COVID-19 were independent of them.
Therefore, in this study, we aimed to evaluate the total and direct effects of EA on the susceptibility, hospitalization, and severity of COVID-19 using the SVMR and the MVMR separately, trying to provide evidence for public health resources allocation and targeting prevention planning.
We extracted single-nucleotide polymorphisms (SNPs) correlated with EA from a published GWAS meta-analysis, which included 71 studies with 1,131,881 European-descent individuals in total (23) and this is the largest GWAS of EA to date. We used SNPs at the genome-wide significance of p < 5 × 10−8 and excluded those in potential linkage disequilibrium (r2 > 0.01), being palindromic with intermediate allele frequencies or not reported in COVID-19 outcome GWAS datasets. SNP coefficients were expressed in SD units (SD = 4.2 years). We used the F statistics to evaluate the strength of genetic variants. One prior study using the similar SNPs with ours reported a median F statistics of 45 (24), indicating that the validity of genetic variants was generally reliable. Proportion of variance explained by included SNPs was calculated according to one prior study (25). We presented a detailed description of EA in Supplementary Table 1. Due to privacy policy, we only used summary statistics excluding 23andMe in our MVMR.
Summary statistics for intelligence (26), income, BMI (27), vigorous physical activity (28), sedentary behavior, smoking (29), and alcohol consumption (29) were obtained. We placed the detailed information about these exposures in Supplementary Table 1.
We obtained GWAS data for COVID-19 outcomes from the 6th round of the COVID-19 Host Genetics Initiative (COVID-19 HGI), which was conducted on mixed ancestry and released at June 15, 2021. Detailed information about the COVID-19 HGI has been described elsewhere (30). Three different phenotypes, including susceptibility (112,612 cases and 2,474,079 controls), hospitalization (24,274 cases and 2,061,529 controls), and severity (8,779 cases and 1,001,875 controls), were analyzed in our MR analyses. We placed the definition and sample size of each phenotype in Supplementary Table 2.
Additionally, we would also use the 5th round summary data for European ancestry only to conducted sensitivity analyses.
Genetic instrumental SNPs explained 3.4% of the variance for EA (Supplementary Table 3). We used an online tool to calculate the power to detect the casual estimates (31) https://sb452.shinyapps.io/power/. With a type I error of 5%, we have sufficient statistical power to detect the difference in the risk of susceptibility, hospitalization, and severity of COVID-19 using all the cohorts. When using the instrumental SNPs after excluding those from 23andMe, similar results were observed. We presented detailed information in Supplementary Table 3.
In the SVMR, we used the random-effects inverse-variance weighted (IVW) method to estimate the total effects of EA on COVID-19 susceptibility, hospitalization, and severity separately. To validate the results of this study, several sensitivity analyses, including MR-Egger, weighted median, and weighted mode, were conducted additionally. MR-Egger is a method, which can detect and adjust for directional pleiotropy (32). Weighted median method allows up to half of the genetic variants to be invalid (33). As for the weighted mode, it is robust to horizontal pleiotropy (34). If we obtained similar results from all these four MR models, our findings would be more robust. We also used the Cochran's Q test to detect possible heterogeneity across individual SNPs and intercept from MR-Egger regression to detect directional pleiotropy.
Next, we used overlapping SNPs as instruments. We applied the random-effects IVW framework to estimate the direct effects of EA in the MVMR analyses after controlling intelligence, income, BMI, vigorous physical activity, sedentary behavior, smoking, and alcohol consumption separately. Although there was partial overlap among GWAS data and we did not have individual-level data, we still calculated the conditional F-statistic approximately for reference. The calculated results were presented in Supplementary Table 4.
Genome-wide association study data could be accessed through the MR-Base platform (35, 36). All the statistical analyses were conducted using R software.
After selection, we used 751 SNPs for our SVMR analysis investigating the total effect of EA on COVID-19 susceptibility (Supplementary Figure 1). Primary analysis using IVW indicated that a 1-SD increase in EA was related to a lower risk of COVID-19 susceptibility [odds ratio (OR) 0.86, 95% CI 0.84–0.89]. This effect was consistent across MR-Egger and weighted median, while no significant relationship was observed in weighted mode (Figure 1A). No directional pleiotropy was found (p = 0.485), while potential heterogeneity was detected. When using 462 SNPs after excluding those from 23andMe (Supplementary Figure 2), we observed similar results (Figure 1B). Detailed information was presented in Supplementary Table 5. Results from sensitivity analyses also supported our findings (Supplementary Figure 3).
Figure 1. The SVMR forest plot of EA on COVID-19 susceptibility using SNPs from (A) all the cohorts and (B) all the cohorts excluding 23andMe. COVID-19, coronavirus disease 2019; MR, Mendelian randomization; OR, odds ratio; SNPs, single-nucleotide polymorphisms; IVW, inverse-variance weighted; SVMR, single-variable MR; EA, educational attainment.
In the MVMR analysis, EA retained its association with susceptibility of COVID-19 after controlling all other exposures except for intelligence (Figure 2).
Figure 2. The MVMR forest plot of EA on COVID-19 susceptibility controlling intelligence, income, body mass index, vigorous physical activity, sedentary behavior, smoking, and alcohol consumption separately. COVID-19, coronavirus disease 2019; OR, odds ratio; MVMR, multivariable Mendelian randomization; EA, educational attainment.
We used 751 SNPs in our SVMR analysis investigating the total effect of EA on COVID-19 hospitalization (Supplementary Figure 1). Primary analysis using IVW indicated that a 1-SD increase in EA was correlated with a lower risk of COVID-19 hospitalization (OR 0.67, 95% CI 0.62–0.73). This association was consistent with weighted median, while no causal relationship was observed in MR-Egger and weighted mode (Figure 3A). Results from the MR-Egger intercept and heterogeneity test were presented in Supplementary Table 5. When using 462 SNPs after excluding those from 23andMe (Supplementary Figure 2), we observed similar results (Figure 3B). Detailed results were presented in Supplementary Table 5. Results from sensitivity analyses also supported our findings (Supplementary Figure 3).
Figure 3. The SVMR forest plot of EA on COVID-19 hospitalization using SNPs from (A) all the cohorts and (B) all the cohorts excluding 23andMe. COVID-19, coronavirus disease 2019; MR, Mendelian randomization; OR, odds ratio; SNPs, single-nucleotide polymorphisms; IVW, inverse-variance weighted; SVMR, single-variable MR; EA, educational attainment.
In the MVMR analysis, EA maintained its association with susceptibility of COVID-19 after accounting for all other exposures (Figure 4).
Figure 4. The MVMR forest plot of EA on COVID-19 hospitalization controlling intelligence, income, body mass index, vigorous physical activity, sedentary behavior, smoking, and alcohol consumption separately. COVID-19, coronavirus disease 2019; OR, odds ratio; MVMR, multivariable Mendelian randomization; EA, educational attainment.
After selection, we used 744 SNPs in our SVMR analysis investigating the total effect of EA on COVID-19 severity (Supplementary Figure 1). Primary analysis using IVW indicated that a 1-SD increase in EA was correlated with a lower COVID-19 severity (OR 0.67, 95% CI 0.58–0.79). This association was consistent with weighted median, while no causal relationship was observed in MR-Egger and weighted mode (Figure 5A). Results from the MR-Egger intercept and heterogeneity test were presented in Supplementary Table 5. When using 455 SNPs after excluding those from 23andMe (Supplementary Figure 2), the effect of EA on COVID-19 severity was observed among all the methods, except weighted mode (Figure 5B). Results from sensitivity analyses also supported our findings (Supplementary Figure 3).
Figure 5. The SVMR forest plot of EA on COVID-19 severity using SNPs from (A) all the cohorts and (B) all the cohorts excluding 23andMe. COVID-19, coronavirus disease 2019; MR, Mendelian randomization; OR, odds ratio; SNPs, single-nucleotide polymorphisms; IVW, inverse-variance weighted; SVMR, single-variable MR; EA, educational attainment.
In the MVMR analysis, EA maintained a direct effect on the severity of COVID-19 after controlling all other related exposures, except income and sedentary behavior (Figure 6).
Figure 6. The MVMR forest plot of EA on COVID-19 severity controlling intelligence, income, body mass index, vigorous physical activity, sedentary behavior, smoking, and alcohol consumption separately. COVID-19, coronavirus disease 2019; OR, odds ratio; MVMR, multivariable Mendelian randomization; EA, educational attainment.
In this study, using both the SVMR and MVMR, we found genetically higher EA was related to a lower risk of susceptibility, hospitalization, and severity of COVID-19.
Using data from UK Biobank, a prior study reported that lower EA was correlated with a higher COVID-19 infection risk [relative risk (RR) 2.00, 95% CI 1.66–2.42] (13), indicating EA might be a possible predictor for COVID-19 infection. However, the sample size of this study was relatively small, with only 948 cases enrolled, making their results less reliable. While in this study, using the largest GWAS of EA and latest summary statistics for COVID-19, we have sufficient statistical power and found that EA is correlated with COVID-19 susceptibility. The underlying mechanism between EA and COVID-19 susceptibility could be partly explained by frontline jobs usually without a requirement of an advanced degree (37), thus individuals with lower EA being more likely to be infected. Second, people with lower EA tend to have a lower socioeconomic status. As a result, they are more likely to live with multiple close generations and, therefore, at greater risk of contracting COVID-19 (4).
Hospitalization is another important COVID-19 outcome and the population with lower EA was reported at a higher rate of hospitalization in a prior observational study (38). However, a major disadvantage of this study is that they only used data from 5 New York City boroughs and their sample might not be representative enough. While in this study, this shortcoming was overcome and we found that EA was a predictor for COVID-19 hospitalization in both the SVMR and MVMR. In addition to COVID-19, EA has been identified as a risk factor for hospitalization among many infectious diseases such as pneumonia and bacteremia (39, 40). Data from a Danish population-based case–control study indicated that the population with a short duration of education had a substantially higher risk of bacteremia than those with long duration (39). The underlying mechanism between lower EA and higher risk of hospitalization might be mediated by overcrowding, poor housing conditions, and hygienic practices.
In addition, this study also found that EA was a predictor for COVID-19 severity, which was in accordance with one prior MR study from Japan (41). However, comparing with the study from Japan, we applied the latest 6th round of COVID-19 summary statistics in this study with a larger sample. Several hypotheses are elucidating the mechanism between EA and COVID-19 severity. First, individuals with lower EA and socioeconomic positions are more likely to be affected by job stress such as unemployment, which might lead to a higher risk of immune system disruption and comorbidities (42). So far, both the weak immunity and the presence of comorbidities are recognized risk factors of COVID-19 severity (43, 44). Besides, population with lower EA is more likely to have unhealthy behaviors such as smoking and an unbalanced diet (45), while these unhealthy behaviors have been recognized as risk factors of COVID-19 mortality or severity (46, 47). Additionally, lower EA generally correlates with lower income and socioeconomic status, which means that they have limited access to healthcare (48) and once they are infected, they might not get treatment in time and turn to be critically ill or even dead.
As for the MVMR, we found that EA still maintained its effects on COVID-19 outcomes under almost all the conditions. This showed that the effects of EA were generally independent of these exposures. One limitation of our MVMR was that part of the conditional F-statistic was relatively low. However, since there was partial overlap, the conditional F-statistic might not be accurate enough and should be interpreted cautiously (21).
This study has several strengths. First, using the largest GWAS of EA with 1.1 million participants and the latest summary statistics for COVID-19 from the 6th round of the COVID-19 HGI, we have enough statistical power. Second, although MR study is still prone to potential sources of bias, it is generally less vulnerable to confounding factors than observational studies. Third, in addition to the SVMR, we also conducted the MVMR analyses and results showed that the effects of EA on COVID-19 outcomes were generally independent of some other exposures. However, this study could not be devoid of limitations. First, since individuals with no or mild symptoms are less likely to test for COVID-19 and there might be potential selection bias in this study. Second, COVID-19 outcome ascertainment methods were not exactly the same among all the cohorts in the COVID-19 HGI, leading to the potential heterogeneity. However, we were unable to estimate how these differences might influence our findings since we only had access to the overall summary statistics. Third, in order to increase the sample size and statistical power, we used the overall COVID-19 HGI GWAS in our primary analyses, which was conducted on mixed ancestry and might lead to some potential population stratification biases. However, we have conducted sensitivity analyses using the summary statistics for European only. Fourth, one prior study reported that using GWAS of education-related traits might be biased from population stratification (49). Another limitation of our MR is the partial overlap of participants (e.g., UKB) in the exposure and outcome datasets, which might lead to possible bias (50). However, bias caused by sample overlap would likely to be minimal for both the continuous and binary outcomes (50) and it has also been shown that 2-sample MR methods may be safely used in single sample provided the data are derived from large biobanks (51), as is the case in our analysis. Last, EA is a complex phenotype and might correlate with some cofounders. Although we observed no directional pleiotropy and the MVMR analyses also showed that the effects of EA on COVID-19 outcomes were generally independent of some other exposures, it is still possible that confounding and pleiotropy may be present.
Our MR analyses indicated that EA is a predictor for susceptibility, hospitalization, and severity of COVID-19 disease. Population with lower EA should be provided with a higher prioritization of public health resources to decrease the morbidity and mortality of COVID-19.
Publicly available datasets were analyzed in this study and can be accessed via the references we used.
Ethical review and approval was not required for the study on human participants in accordance with the local legislation and institutional requirements. Written informed consent for participation was not required for this study in accordance with the national legislation and the institutional requirements.
ZJ, MW, XJ, and XW: designing the study. ZJ and MW: carrying out the study, analyzing the data, and writing the article. XJ and XW: revising the article. All authors read and approved the final manuscript.
This study was funded by Post-Doctor Research Project, West China Hospital, Sichuan University (2020HXBH016).
The authors declare that the research was conducted in the absence of any commercial or financial relationships that could be construed as a potential conflict of interest.
All claims expressed in this article are solely those of the authors and do not necessarily represent those of their affiliated organizations, or those of the publisher, the editors and the reviewers. Any product that may be evaluated in this article, or claim that may be made by its manufacturer, is not guaranteed or endorsed by the publisher.
We thank the COVID-19 HGI, Social Science Genetic Association Consortium (SSGAC), Complex Trait Genetics Lab (CTGlab), Medical Research Center-Integrative Epidemiology Unit (MRC-IEU) (UK Bristol), Genetic Investigation of ANthropometric Traits (GIANT) consortium, and GWAS and Sequencing Consortium of Alcohol and Nicotine use (GSCAN) for providing publicly available summary statistics. We would also like to thank Miao Wang for helping us revising our manuscript.
The Supplementary Material for this article can be found online at: https://www.frontiersin.org/articles/10.3389/fpubh.2021.731962/full#supplementary-material
1. Polack FP, Thomas SJ, Kitchin N, Absalon J, Gurtman A, Lockhart S, et al. Group, safety and efficacy of the BNT162b2 mRNA Covid-19 vaccine. N Engl J Med. (2020) 383:2603–15. doi: 10.1056/NEJMoa2034577
2. Dong E, Du H, Gardner L. An interactive web-based dashboard to track COVID-19 in real time. Lancet Infect Dis. (2020) 20:533–4. doi: 10.1016/S1473-3099(20)30120-1
3. Chen J, Qi T, Liu L, Ling Y, Qian Z, Li T, et al. Clinical progression of patients with COVID-19 in Shanghai, China. J Infect. (2020) 80:e1–6. doi: 10.1016/j.jinf.2020.03.004
4. Schmidt H, Gostin LO, Williams MA. Is it lawful and ethical to prioritize racial minorities for COVID-19 vaccines? JAMA. (2020) 324:2023–4. doi: 10.1001/jama.2020.20571
5. Zhang Y, Rogers A, Nadeau K, Gu J, Yang S. A perspective on the role of point-of-care “immuno-triaging” to optimize COVID-19 vaccination distribution in a time of scarcity. Front Public Health. (2021) 9:638316. doi: 10.3389/fpubh.2021.638316
6. Zimmerman RK, South-Paul JE, Poland GA. Rationing of civilian coronavirus disease 2019 vaccines while supplies are limited. J Infect Dis. (2020) 222:1776–9. doi: 10.1093/infdis/jiaa569
7. Powers AC, Aronoff DM, Eckel RH. COVID-19 vaccine prioritisation for type 1 and type 2 diabetes. Lancet Diabetes Endocrinol. (2021) 9:140–1. doi: 10.1016/S2213-8587(21)00017-6
8. Cohen AK, Syme SL. Education: a missed opportunity for public health intervention. Am J Public Health. (2013) 103:997–1001. doi: 10.2105/AJPH.2012.300993
9. Chan EH, Scales DA, Brewer TF, Madoff LC, Pollack MP, Hoen AG, et al. Forecasting high-priority infectious disease surveillance regions: a socioeconomic model. Clin Infect Dis. (2013) 56:517–24. doi: 10.1093/cid/cis932
10. Mountjoy E, Davies NM, Plotnikov D, Smith GD, Rodriguez S, Williams CE, et al. Education and myopia: assessing the direction of causality by mendelian randomisation. BMJ. (2018) 361:k2022. doi: 10.1136/bmj.k2022
11. Tillmann T, Vaucher J, Okbay A, Pikhart H, Peasey A, Kubinova R, et al. Education and coronary heart disease: mendelian randomisation study. BMJ. (2017) 358:j3542. doi: 10.1136/bmj.j3542
12. Chadeau-Hyam M, Bodinier B, Elliott J, Whitaker MD, Tzoulaki I, Vermeulen R, et al. Risk factors for positive and negative COVID-19 tests: a cautious and in-depth analysis of UK biobank data. Int J Epidemiol. (2020) 49:1454–67. doi: 10.1093/ije/dyaa134
13. Niedzwiedz CL, O'Donnell CA, Jani BD, Demou E, Ho FK., Celis-Morales C, et al. Ethnic and socioeconomic differences in SARS-CoV-2 infection: prospective cohort study using UK Biobank. BMC Med. (2020) 18:160. doi: 10.1186/s12916-020-01640-8
14. Ribeiro KB, Ribeiro AF, de Sousa Mascena Veras MA, de Castro MC. Social inequalities and COVID-19 mortality in the city of São Paulo, Brazil. Int J Epidemiol. (2021) 50:732–42. doi: 10.1093/ije/dyab022
15. C. Telomeres Mendelian Randomization,, Haycock PC, Burgess S, Nounu A, Zheng J, Okoli GN, et al. Association between telomere length and risk of cancer and non-neoplastic diseases: a mendelian randomization study. JAMA Oncol. (2017) 3:636-651. doi: 10.1001/jamaoncol.2016.5945
16. Rees JMB, Foley CN, Burgess S. Factorial mendelian randomization: using genetic variants to assess interactions. Int J Epidemiol. (2020) 49:1147–58. doi: 10.1093/ije/dyz161
17. Davies NM, Holmes MV, Davey Smith G. Reading mendelian randomisation studies: a guide, glossary, and checklist for clinicians. BMJ. (2018) 362:k601. doi: 10.1136/bmj.k601
18. Leong A, Cole JB, Brenner LN, Meigs JB, Florez JC, Mercader JM. Cardiometabolic risk factors for COVID-19 susceptibility and severity: a mendelian randomization analysis. PLoS Med. (2021) 18:e1003553. doi: 10.1371/journal.pmed.1003553
19. Ponsford MJ, Gkatzionis A, Walker VM, Grant AJ, Wootton RE, Moore LSP, et al. Cardiometabolic traits, sepsis, and severe COVID-19: a mendelian randomization investigation. Circulation. (2020) 142:1791–3. doi: 10.1161/CIRCULATIONAHA.120.050753
20. Butler-Laporte G, Nakanishi T, Mooser V, Renieri A, Amitrano S, Zhou S, et al. The effect of angiotensin-converting enzyme levels on COVID-19 susceptibility and severity: a Mendelian randomization study. Int J Epidemiol. (2021) 50:75–86. doi: 10.1093/ije/dyaa229
21. Rosoff DB, Davey Smith G, Mehta N, Clarke TK, Lohoff FW. Evaluating the relationship between alcohol consumption, tobacco use, and cardiovascular disease: a multivariable mendelian randomization study. PLoS Med. (2020) 17:e1003410. doi: 10.1371/journal.pmed.1003410
22. Davies NM, Hill WD, Anderson EL, Sanderson E, Deary IJ Davey Smith G. Multivariable two-sample Mendelian randomization estimates of the effects of intelligence and education on health. Elif e. (2019) 8:e43990. doi: 10.7554/eLife.43990
23. Lee JJ, Wedow R, Okbay A, Kong E, Maghzian O, Zacher M. et al. Gene discovery and polygenic prediction from a genome-wide association study of educational attainment in 11 million individuals. Nat Genet. (2018) 50:1112–21. doi: 10.1038/s41588-018-0147-3
24. Gill D, Karhunen V, Malik R, Dichgans M, Sofat N. Cardiometabolic traits mediating the effect of education on osteoarthritis risk: a Mendelian randomization study. Osteoarthritis Cartilage. (2021) 29:365–71. doi: 10.1016/j.joca.2020.12.015
25. Locke AE, Kahali B, Berndt SI, Justice AE, Pers TH, Day FR, et al. Genetic studies of body mass index yield new insights for obesity biology. Nature. (2015) 518:197–206. doi: 10.1038/nature14177
26. Savage JE, Jansen PR, Stringer S, Watanabe K, Bryois J, De Leeuw CA, et al. Genome-wide association meta-analysis in 269,867 individuals identifies new genetic and functional links to intelligence. Nat Genet. (2018) 50:912–9. doi: 10.1038/s41588-018-0152-6
27. Yengo L, Sidorenko J, Kemper KE, Zheng Z, Wood AR, Weedon MN, et al. Consortium, Meta-analysis of genome-wide association studies for height and body mass index in approximately 700000 individuals of European ancestry. Hum Mol Genet. (2018) 27:3641–9. doi: 10.1093/hmg/ddy271
28. Klimentidis YC, Raichlen DA, Bea J, Garcia DO, Wineinger NE, Mandarino LJ, et al. Genome-wide association study of habitual physical activity in over 377,000 UK Biobank participants identifies multiple variants including CADM2 and APOE. Int J Obes (Lond). (2018) 42:1161–76. doi: 10.1038/s41366-018-0120-3
29. Liu M, Jiang Y, Wedow R, Li Y, Brazel DM, Chen F., et al. Association studies of up to 12 million individuals yield new insights into the genetic etiology of tobacco and alcohol use. Nat Genet. (2019) 51:237–44. doi: 10.1038/s41588-018-0307-5
30. COVID-19 Host Genetics Initiative. The COVID-19 host genetics initiative, a global initiative to elucidate the role of host genetic factors in susceptibility and severity of the SARS-CoV-2 virus pandemic. Eur J Hum Genet. (2020) 28:715–8. doi: 10.1038/s41431-020-0636-6
31. Burgess S. Sample size and power calculations in mendelian randomization with a single instrumental variable and a binary outcome. Int J Epidemiol. (2014) 43:922–9. doi: 10.1093/ije/dyu005
32. Burgess S, Thompson SG. Interpreting findings from mendelian randomization using the MR-Egger method. Eur J Epidemiol. (2017) 32:377–89. doi: 10.1007/s10654-017-0255-x
33. Bowden J, Davey Smith G, Haycock PC, Burgess S. Consistent estimation in mendelian randomization with some invalid instruments using a weighted median estimator. Genet Epidemiol. (2016) 40:304–14. doi: 10.1002/gepi.21965
34. Hartwig FP, Davey Smith G, Bowden J. Robust inference in summary data Mendelian randomization via the zero modal pleiotropy assumption. Int J Epidemiol. (2017) 46:1985–98. doi: 10.1093/ije/dyx102
35. Hemani G, Zheng J, Elsworth B, Wade KH, Haberland V, Baird D, et al. The MR-base platform supports systematic causal inference across the human phenome. Elife. (2018) 7:e34408. doi: 10.7554/eLife.34408
36. Elsworth BL, Lyon MS, Alexander T, Liu Y, Matthews P, Hallett J, et al. The MRC IEU OpenGWAS data infrastructure. bioRxiv. doi: 10.1101/2020.08.10.244293
37. Hawkins RB, Charles EJ, Mehaffey JH. Socio-economic status and COVID-19-related cases and fatalities. Public Health. (2020) 189:129–34. doi: 10.1016/j.puhe.2020.09.016
38. Wadhera RK, Wadhera P, Gaba P, Figueroa JF, Maddox KE, Yeh RW, et al. Variation in COVID-19 hospitalizations and deaths across new york city boroughs JAMA. (2020) 323:2192–5. doi: 10.1001/jama.2020.7197
39. Koch K, Søgaard M, Nørgaard M, Thomsen RW, Schønheyder HC, Danish Collaborative Bacteremia Network. Socioeconomic inequalities in risk of hospitalization for community-acquired bacteremia: a danish population-based case-control study. Am J Epidemiol. (2014) 179:1096–106. doi: 10.1093/aje/kwu032
40. Flory JH, Joffe M, Fishman NO, Edelstein PH, Metlay JP. Socioeconomic risk factors for bacteraemic pneumococcal pneumonia in adults. Epidemiol Infect. (2009) 137:717–26. doi: 10.1017/S0950268808001489
41. Yoshikawa M, Asaba K. Educational attainment decreases the risk of COVID-19 severity in the european population: a two-sample mendelian randomization study. Front Public Health. (2021) 9:673451. doi: 10.3389/fpubh.2021.673451
42. Bermudi PMM, Lorenz C, Aguiar BS, Failla MA, Barrozo LV, Chiaravalloti-Neto F. Spatiotemporal ecological study of COVID-19 mortality in the city of São Paulo, Brazil: Shifting of the high mortality risk from areas with the best to those with the worst socio-economic conditions. Travel Med Infect Dis. (2021) 39:101945. doi: 10.1016/j.tmaid.2020.101945
43. Jordan RE, Adab P, Cheng KK. Covid-19: risk factors for severe disease and death. BMJ. (2020) 368:m1198. doi: 10.1136/bmj.m1198
44. Khalatbari-Soltani S, Cumming RC, Delpierre C, Kelly-Irving M. Importance of collecting data on socioeconomic determinants from the early stage of the COVID-19 outbreak onwards. J Epidemiol Community Health. (2020) 74:620–3. doi: 10.1136/jech-2020-214297
45. Lawrence EM. Why do college graduates behave more healthfully than those who are less educated? J Health Soc Behav. (2017) 58:291–306. doi: 10.1177/0022146517715671
46. Elliott J, Bodinier B, Whitaker M, Delpierre C, Vermeulen R, Tzoulaki I. COVID-19 mortality in the UK Biobank cohort: revisiting and evaluating risk factors. Eur J Epidemiol. (2021) 36:299–309. doi: 10.1007/s10654-021-00722-y
47. Gao YD, Ding M, Dong X, Zhang JJ, Kursat Azkur A, Azkur D, et al. Risk factors for severe and critically ill COVID-19 patients: a review allergy. (2021) 76:428–55. doi: 10.1111/all.14657
48. Zajacova A, Lawrence EM. The relationship between education and health: reducing disparities through a contextual approach. Annu Rev Public Health. (2018) 39:273–89. doi: 10.1146/annurev-publhealth-031816-044628
49. Sanderson E, Richardson TG, Hemani G, Davey Smith G. The use of negative control outcomes in mendelian randomization to detect potential population stratification. Int J Epidemiol. (2021) 50:1350–61. doi: 10.1093/ije/dyaa288
50. Burgess S, Davies NM, Thompson SG. Bias due to participant overlap in two-sample mendelian randomization. Genet Epidemiol. (2016) 40:597–608. doi: 10.1002/gepi.21998
Keywords: COVID-19, Mendelian randomization, susceptibility, severity, educational attainment
Citation: Jian Z, Wang M, Jin X and Wei X (2021) Genetically Predicted Higher Educational Attainment Decreases the Risk of COVID-19 Susceptibility and Severity: A Mendelian Randomization Study. Front. Public Health 9:731962. doi: 10.3389/fpubh.2021.731962
Received: 30 June 2021; Accepted: 12 November 2021;
Published: 23 December 2021.
Edited by:
Angelo D'Errico, Azienda Sanitaria Locale TO3, ItalyReviewed by:
Masahiro Yoshikawa, Nihon University School of Medicine, JapanCopyright © 2021 Jian, Wang, Jin and Wei. This is an open-access article distributed under the terms of the Creative Commons Attribution License (CC BY). The use, distribution or reproduction in other forums is permitted, provided the original author(s) and the copyright owner(s) are credited and that the original publication in this journal is cited, in accordance with accepted academic practice. No use, distribution or reproduction is permitted which does not comply with these terms.
*Correspondence: Xin Wei, d2VpeGluc2N1QHNjdS5lZHUuY24=; Xi Jin, SmFuY3lfa2luZ0Bob3RtYWlsLmNvbQ==
†These authors have contributed equally to this work and share first authorship
Disclaimer: All claims expressed in this article are solely those of the authors and do not necessarily represent those of their affiliated organizations, or those of the publisher, the editors and the reviewers. Any product that may be evaluated in this article or claim that may be made by its manufacturer is not guaranteed or endorsed by the publisher.
Research integrity at Frontiers
Learn more about the work of our research integrity team to safeguard the quality of each article we publish.