- Department of Health Behavioral and Social Medicine, West China School of Public Health and West China Fourth Hospital, Sichuan University, Chengdu, China
Background: Rural communities worldwide are experiencing the most significant levels of aging. Most rural elderly have no stable pension, and leading family income plays an indispensable role in the life security of rural elderly. This study aims to investigate whether the association between annual family income per capita and body mass index (BMI) and self-rated health (SRH) in rural elderly is moderated by education during fast economic development.
Methods: We chose the fixed-effects model to analyze the impact of the annual family income per capita change on BMI and SRH based on a large, nationally representative longitudinal dataset of rural respondents aged above 60 of the China Family Panel Studies (CFPS) from 2010 to 2018.
Results: Six hundred and fifty-eight were eligible for inclusion in our analysis in CFPS. The median age of participants was 65 years in 2010, and 379 (57.60%) participants were male. Self-rated health increased with higher the logarithmized family income per capita among the rural illiterate elderly (β = 0.0770; 95% CI = 0.0065–0.1473). Body mass index increased with higher the logarithmized family income per capita among the rural elderly (β = 0.1614, 95% CI: 0.0325–0.2903), and it was more evident among the illiterate elderly (β = 0.2462, 95% CI: 0.05519–0.4372).
Conclusion: Family income has an impact on BMI and SRH moderated by education level among rural elderly in China. These results contribute to developing more targeted strategies in the context of a developing country. In addition, it also reminds us to consider the differences in the educational level of the elderly in rural areas when examining the relationship between family income and health.
Introduction
With the decline of fertility and mortality, the number of elders continues to increase rapidly. It is estimated that between 2017 and 2050, the proportion of the world's population aged 60 and above will nearly double from 926 million to 2.1 billion (1). The problem of aging is particularly evident in Asia. From 2015 to 2050, the total number of people aged 80 and above in 23 Asian countries is expected to quadruple (2).
Rural communities worldwide are experiencing the most significant levels of aging in place, including many developed countries and developing countries, such as China, India, Japan, Indonesia, Australia, the United States (3–7). Compared with the urban elderly, the rural elderly have limited economic and material resources (8, 9). Under the background of economic and social transformation and rural structural adjustment, the rural economy of many developing countries has developed rapidly (10–13). Some developing countries, such as Brazil, South Africa, Mexico, and China, have launched government transfer or social pension programs for elderly who have not previously covered pensions (14). Nevertheless, the elderly in rural areas, especially in less developed regions, is expected to receive less pension than those in urban counterparts (15). Therefore, family income has played an indispensable role in life support for the rural elderly.
Previous studies confirmed a relationship between family income and health (16, 17). The reason may be that income affects health through quality housing, social networks and relationships, health-related knowledge, diet choices, and sports activities in safe communities (18). Most previous studies of the association between family income and health have used regression modeling applied to cross-sectional data (19–22). We cannot be confident that the associations uncovered in these studies are not spurious by this methodological approach. In addition to methods, the choice of outcome indicators will also affect the results. Veenstra and Vanzella-Yang found that the change of family average income impacted health (18), but it only takes self-rated health (SRH) as an outcome indicator and lacks the objective outcome indicator to evaluate health.
The outcome indicators used in this study were body mass index (BMI) and SRH. Body mass index was regarded as an objective indicator of health outcomes. Moreover, the health burden of obesity has been widely recognized, and it is the leading risk factor for many adverse health consequences, such as cardiovascular disease, diabetes, and depression. Therefore, it has been the focus of many researchers in social science and public health to clarify the reasons for weight gain (23, 24). Self-rated health was used as an outcome indicator to evaluate the subjective health of the elderly. It is also a concise and straightforward public health indicator to evaluate the overall health status in epidemiological studies (20), and it is also can be used as a predictor of subsequent mortality and health issues (25, 26). This study aims to find the relationship between the growth of family income and the health changes of the elderly in rural areas based on a large, nationally representative longitudinal dataset of rural respondents aged above 60 from three waves of the China Family Panel Studies (CFPS) from 2010 to 2018. Because the education level of the elderly did not change, this study hopes to focus on whether annual family income per capita has different effects on BMI and SRH of the elderly with different educational levels.
Methods
Sample and Data Collection
The data were derived from the CFPS. China Family Panel Studies is a biennial longitudinal survey conducted by the Institution of Social Science Survey at Peking University. This investigation launched in 2010 with five waves of publicly released datasets. The samples covered 25 provinces, accounting for 95% of the total population of China. The contents of CFPS are rather typical, covering the demographics, socioeconomic condition, education, and health of respondents.
The Biomedical Ethics Review Committee of Peking University approved CFPS, and all participants were required to provide written informed consent. The ethical approval number was IRB00001052-14010.
Since the fixed-effect regression studied the relationship between the change in dependent variables and those independent variables across each wave, we selected 658 participants according to the following criteria: (1) aged 60 and above in 2010, (2) rural elderly (respondents were categorized into urban and rural residents according to their household living regions defined by National Bureau of Statistics of the People's Republic of China), and (3) had not missing values of dependent or independent variables in all three waves (2010, 2014, and 2018).
Variables
Dependent Variable
The health outcomes selected in this paper are SRH and BMI, considered from subjective and objective aspects.
The dependent variable was SRH. Respondents were asked, “in general, would you say your health is excellent, very good, good, fair, or poor?” We coded this variable as 5 = excellent, 4 = very good, 3 = good, 2 = fair, and 1 = poor.
Body mass index, a continuous measure of body weight relative to height, was calculated as body weight in kilograms divided by squared height in meters (kg/m2).
Independent Variable
Family income was measured by annual family income per capita (taking the natural log and deflating based on the 2010 CPI). Family income of CFPS included wage income, business income, transfer income, property income, and other income. Then, we divided the family income by the family size to get the family per capita income.
Control Variable
Socio-demographics included sex, age, marital status, and the number of years of education. Age and the number of years of schooling were continuous variables. Marital status and chronic diseases weredefined as binary variables. Marital status was divided into married and divorced/widowed/unmarried, and chronic disease was divided into with and without.
Illiterate refers to the older adults who have not received a formal education, that is, the number of years of education was 0. Non-illiterate refers to the older adults who have received a formal education, that is, the number of years of education was more than 0.
Analysis Strategy
Fixed effect model and random effect model can explain the estimation error caused by concrete variables. The main difference between the two models is that the fixed effect model treats the unobserved differences between individuals as fixed parameters. In contrast, the random effect model treats the missing variables as random variables with a special probability distribution and assumes they are unrelated to the observed variables. This assumption of the stochastic model is generally challenging because the missing variables are usually associated with other explanatory variables in the model. The Hausman test testifies the null hypothesis that the random effects coefficients are identical to the fixed effects coefficients. The test produced P < 0.001 for both SRH/BMI, indicating that fixed effect models were appropriate.
To address the potential endogeneity, one of the best methods for addressing confounding in observational research are fixed effect models applied to longitudinal data containing repeated measures of both income and health (27). In this study, a longitudinal linear fixed-effects regression model was employed to estimate the association between changes in annual family income per capita and changes in SRH/BMI during three waves in older adults. The fixed effect model could effectively eliminate the influence of missing variables on dependent variables and the interference between independent and dependent variables. Meanwhile, the model also allows us to control time-variant factors that do not vary across individuals.
Specification of our model was as follows:
SRHit = β0 + β1 Annual family income per capitait + β2 Control Ait + β3 Control Bi + εit
BMIit = β0 + β1 Annual family per capitait + β2 ControlAit + β3Control Bi + εit
SRHit refers to SRH for individual i at time t, and BMIit refers to BMI for individual i at time t. Similarly, Annual family income per capitait denotes the explanatory variable, the annual family income per capita for individual i at time t. Control Ait represents the time-varying control variable, including age, marital status, and chronic disease. Control Bi represents the non-time-varying control variable, including gender and the number of years of education. εit is the error term.
Results
Basic Characteristics of the Respondents
Table 1 shows descriptive statistics separated by sample wave. Overall, the SRH increased significantly from 2010 to 2014, and the average value in 2018 was the same as that in 2014. For BMI, the rural elderly had a slight increase with age.
The annual family income per capita we used was comparable with that in 2010. The revenue increased significantly from 2010 to 2014 and decreased slightly in 2018.
Regression Results of the Effect of Annual Family Income per Capita on SRH and BMI
Model 1 (Table 2) summarized the modeling results of SRH changes in 2010, 2014, and 2018 and changes in equivalized annual family income per capita in 2010, 2014, and 2018, controlling for survey year, age, and marital status. The annual family income per capita was not significantly associated with SRH in model 1. Model 2 and 3 summarized the changes in SRH and equivalized per capita family income between illiterate and non-illiterate in 2010, 2014, and 2018, controlling for age, marital status, chronic diseases, and survey year. Interestingly, with the increase of annual family income per capita of the rural illiterate elderly, their SRH level improved significantly (β = 0.0770; 95% CI = 0.0065–0.1473), but for the non-illiterate elderly, there was no significant correlation between their SRH and annual family income per capita.
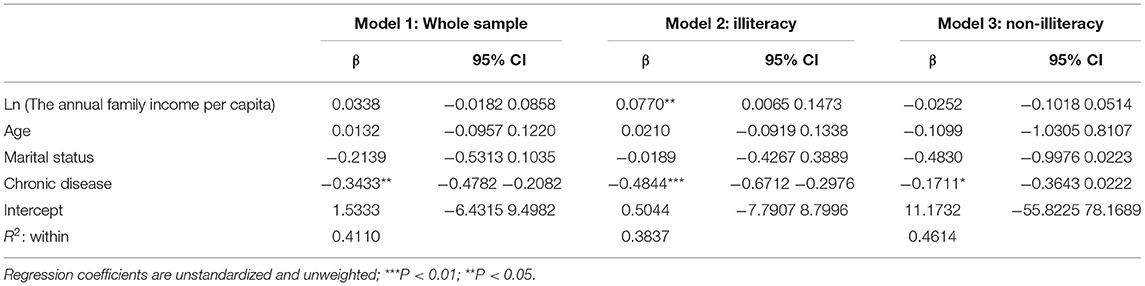
Table 2. Fixed effect modeling of changes in SRH and the annual family income per capita between 2010, 2014, and 2018.
Model 4 (Table 3) summarized the modeling results of BMI health changes in three waves and changes in equivalized annual family income per capita in three waves. The time-varying factors of survey year, age, and marital status were controlled. The annual family income per capita was significantly associated with BMI in model 4. Income increased corresponded to increases in BMI (β = 0.1614; 95% CI = 0.0325–0.2903).
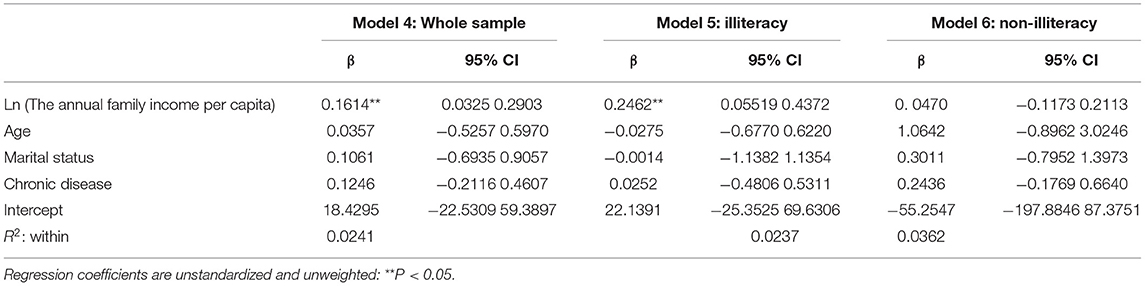
Table 3. Fixed effect modeling of changes in BMI and the annual family income per capita between 2010, 2014, and 2018.
Model 5 and 6 (Table 3) summarized BMI changes and equivalent annual family income per capita between illiteracy and non-illiteracy in three waves. Compared with the overall rural elderly, the increase in BMI of the elderly in illiterate rural areas was more prominent (β = 0.2462; 95% CI = 0.05519–0.4372), and there was not significantly associated with BMI for non-illiteracy.
Discussion
This study used a nationally representative dataset to investigate annual family income per capita on BMI and SRH among rural older adults in China. We found that, for SRH, with the increase of the annual family income per capita, the better results of SRH only existed in the illiterate elderly. However, for BMI, annual family income per capita had a positive correlation with the BMI of the rural elderly, that is, with the increase of income, the BMI of the rural elderly also increased. Meanwhile, in the illiterate elderly, with a rise in income, BMI was more prominent. Our application of dynamic panel models with fixed effects on the Chinese rural old adult population provided a unique chance to uncover the association between annual family income per capita and SRH and BMI in a society undergoing the rapid development of the rural economy.
In previous studies, the relationship between income and SRH was not consistent. Our research found that the increase of income among the rural elderly did not cause significant changes in SRH, and these changes only existed in the illiterate elderly after the stratification of education level. Gunasekara et al. analyzed 13 studies and five longitudinal surveys from four different countries. They came to a general conclusion: in most cases, there was a slight positive correlation between the increase of income and SRH, but these four countries were developed countries and did not consider developing countries (27). By analyzing the panel data of Canada, Veenstra et al. found a weak negative correlation between family income and self-health (18). However, the difference between our study results and the above is probably due to the different study objects. The population of our study is the elderly in rural areas. We can use resource substitution (28) to explain our results. When resources replace each other, the existence of one resource will reduce the harm caused by the lack of another resource. On the contrary, the less one resource, the more influential the other. Income and education are two kinds of resources. Using the resource substitution perspective, we can argue that more substantial health-protective effects of income among disadvantaged populations (rural illiterate elderly) because they have fewer alternative health-promoting resources, especially in rural areas. The increase of family income can increase their economic resources, better access to medical care, and more extensive social networks that promote healthier lifestyles that helps rural illiterate elderly to avoid poor health. Chronic diseases pose a great health threat to the rural elderly, reducing SRH, especially for the illiterate rural elderly. It can be explained that chronic diseases can lead to the decline of physical function of the elderly, affect their mental health, and reduce their quality of life (29).
The BMI of rural elderly increased significantly with the increase of income, but there is no such relationship among the non-illiteracy elderly. Our study differs from the conclusion of Deuchert et al., who found that obesity was a problem that focuses on the social and economic elites in developing countries, such as high-income groups (30). The previous explanation was that the relationship between income and BMI is related to nutrition change. The shift in nutrition refers to the change in eating habits with the development of the economy (31). Through the China Health and Nutrition Survey (CHNS) panel data, Ren found that rising income increased the adults' BMI and the propensity to be overweight. From the perspective of nutrition, there are five potential channels: nutritional intakes, dietary diversity, dietary knowledge, food preference, and dining out, among which dietary diversity plays the most significant role in explaining the income impact (32). It is also helpful to explain the relationship between family income and BMI in rural elders by nutritional change. Because for the rural elderly, higher family income means more food choices and dining out, increasing their extra energy intake. However, we were surprised to find that education seems to be alleviating this relationship, and the educated rural elderly do not increase BMI due to the increase of income. This result can explain the associations between levels of education and nutrition knowledge and concern with weight control (33).
Three limitations of this study must be noted. Firstly, because our BMI and SRH are self-reported—the prevalence of obesity would be overestimated when using self-reported height and weight (34), recall bias may exist in this study, affecting the accuracy of the estimation. Future research should consider other indicators, such as body fat percentage and waist-hip ratio, to better measure body fat and subjective health. At the same time, more perfect scales can be used to measure health status. Secondly, fixed effects analysis controls time-invariant confounding factors at the individual level. There may be unmeasurable or unmeasurable time-varying variables in this study. Third, our sample is restricted to better observe the changing process of the health impact of family income among the rural elderly in China. A threat to representativeness pertains to the high attrition rate experienced by the CFPS from wave one to wave three, although we control for marital status, gender, and age in our models. The existence of missing independent and dependent variables of the CFPS compromises the generalizability of our results, given that rural older are not well-represented by our study. Overall, our study has selection bias.
Conclusion
Few studies measured the association between annual family income per capita between BMI and SRH in rural elderly using fixed effects analysis that rules out the potential endogeneity to the best of our knowledge. At the same time, this study considers the gap between illiteracy and non-illiteracy to adapt to the high illiteracy rate in rural areas. In this study, the illiteracy rate is as high as 55.47%.
Self-rated health increased with higher the logarithmized family income per capita among the rural illiterate elderly. Moreover, BMI increased with higher the logarithmized family income per capita among the rural elderly, especially the illiterate elderly.
In rural economic development, the impact of family income on the rural elderly is undeniable. It is worth noting that the health status of the rural illiterate elderly needs particular attention, because first of all, they have lower education levels and lack health knowledge. Second, most of them have lower insurance levels and lower ability to resist disease risk. At the same time, the average BMI of the rural non-illiterate elderly was 22.56 ± 3.67, while the average BMI of the rural illiterate elderly was 21. 75 ± 3.85. The family income of the illiterate elderly in rural areas also needs to be paid attention to because the increase of average family income can improve their BMI, which is likely to be reasonable. Theoretically and methodologically, we need more research to understand the relationship between socioeconomic status and the health of the elderly and its internal mechanism. It also reminds us to consider the educational differences in rural areas when investigating causal relationships further, which may help develop more targeted strategies in a developing country.
Data Availability Statement
The original contributions presented in the study are included in the article/supplementary material, further inquiries can be directed to the corresponding author.
Author Contributions
YX: methodology, software, and writing—original draft. XR: writing—review and editing. All authors have read and agreed to the published version of the manuscript.
Conflict of Interest
The authors declare that the research was conducted in the absence of any commercial or financial relationships that could be construed as a potential conflict of interest.
Publisher's Note
All claims expressed in this article are solely those of the authors and do not necessarily represent those of their affiliated organizations, or those of the publisher, the editors and the reviewers. Any product that may be evaluated in this article, or claim that may be made by its manufacturer, is not guaranteed or endorsed by the publisher.
References
1. UN. World Population Ageing 2015. Statistical Papers - United Nations (Ser. A), Population and Vital Statistics Report. New York: UN (2017).
2. He W, Goodkind D, Kowal P. An Aging World: 2015. International Population Reports 2016 P95/16-1. Washington, DC: U.S. Census Bureau (2016).
3. Golant SM. Explaining the ageing in place realities of older adults. In Skinner M, Andrews G, Cutchin M, editors. Geographical Gerontology: Concepts and Approaches. London: Routledge (2018). p. 189–202. doi: 10.4324/9781315281216-15
4. Burholt V, Dobbs C. Research on rural ageing: where have we got to and where are we going in Europe? J Rural Stud. (2012) 28:432–46. doi: 10.1016/j.jrurstud.2012.01.009
5. Liao L, Long H, Gao X. Effects of land use transitions and rural aging on agricultural production in China's farming area: a perspective from changing labor employing quantity in the planting industry. Land Use Policy. (2019) 88:104152. doi: 10.1016/j.landusepol.2019.104152
6. Kumar K, Shukla A, Singh A, Ram F, Kowal P. Association between wealth and health among older adults in rural China and India. J Econ Ageing. (2016) 7:43–52. doi: 10.1016/j.jeoa.2016.02.002
7. Brown DL, Glasgow N, Kulcsar LJ, Sanders S, Thiede BC. The multi-scalar organization of aging-related services in US rural places. J Rural Stud. (2019) 68:219–29. doi: 10.1016/j.jrurstud.2018.09.010
8. Glasgow N, Berry EH, editors. Rural Aging in 21st Century America. Dordrecht: Springer (2013). doi: 10.1007/978-94-007-5567-3
9. Keating N. Rural Ageing: A Good Place to Grow Old? Bristol, UK: Policy Press (2008). doi: 10.2307/j.ctt9qgmzc
10. Kanagawa M, Nakata T. Analysis of the energy access improvement and its socioeconomic impacts in rural areas of developing countries. Ecol Econ. (2007) 62:319–29. doi: 10.1016/j.ecolecon.2006.06.005
11. Qu Y, Jiang G, Li Z, Tian Y, Wei S. Understanding rural land use transition and regional consolidation implications in China. Land Use Policy. (2019) 82:742–53. doi: 10.1016/j.landusepol.2018.11.014
12. Smith JP, Strauss John, Zhao Y. Healthy aging in China. J Econ Ageing. (2014) 4:37–43. doi: 10.1016/j.jeoa.2014.08.006
13. Tu S, Long H. Rural restructuring in China: theory, approaches and research prospect. J Geograph Sci. (2017) 27:1169–84. doi: 10.1007/s11442-017-1429-x
14. Jung J, Tran C. The extension of social security coverage in developing countries. J Dev Econ. (2012) 99:439–58. doi: 10.1016/j.jdeveco.2012.05.007
15. Hanewald K, Jia R, Liu Z. Why is inequality higher among the old? Evidence from China. China Econ Rev. (2021) 66:101592. doi: 10.1016/j.chieco.2021.101592
16. Chakraborty R, Bose K, Bisai S. Relationship of family income and house type to body mass index and chronic energy deficiency among urban Bengalee male slum dwellers of Kolkata, India. HOMO. (2009) 60:45–57. doi: 10.1016/j.jchb.2008.06.007
17. Kim DH. Myung-Yong U. The relationships among family income, parental depression, and adolescent aggression in South Korea: a latent growth mediation model. Child Youth Serv Rev. (2018) 84:168–75. doi: 10.1016/j.childyouth.2017.11.025
18. Veenstra G, Vanzella-Yang A. Family income and self-rated health in Canada: using fixed effects models to control for unobserved confounders and investigate causal temporality. Soc Sci Med. (2020) 250:112884. doi: 10.1016/j.socscimed.2020.112884
19. Newton S, Braithwaite D, Akinyemiju TF. Socioeconomic status over the life course and obesity: systematic review and meta-analysis. PLoS ONE. (2017) 12:e0177151. doi: 10.1371/journal.pone.0177151
20. Abdollahpour I, Mooijaart S, Aguilar-Palacio I, Salimi Y, Nedjat S, Mansournia MA, et al. Socioeconomic status as the strongest predictor of self-rated health in Iranian population; a population-based cross-sectional study. J Psychosom Res. (2019) 124:109775. doi: 10.1016/j.jpsychores.2019.109775
21. Prus S. Comparing social determinants of self-rated health across the United States and Canada. Soc Sci Med. (2011) 73:50–9. doi: 10.1016/j.socscimed.2011.04.010
22. Lin H, Jin M, Liu Q, Du Y, Fu J, Sun C, et al. Gender-specific prevalence and influencing factors of depression in elderly in rural China: a cross-sectional study. J Affect Disord. (2021) 288:99–106. doi: 10.1016/j.jad.2021.03.078
23. Bhaskaran K. Isabel dos-Santos-Silva, Leon DA, Douglas IJ, Smeeth L. Association of BMI with overall and cause-specific mortality: a population-based cohort study of 36 million adults in the UK. Lancet Diabet Endocrinol. (2018) 6:944–53. doi: 10.1016/S2213-8587(18)30288-2
24. Luppino FS, de Wit LM, Bouvy PF, Stijnen T, Cuijpers P, Penninx BWJH, et al. Overweight, obesity, and depression: a systematic review and meta-analysis of longitudinal studies. Arch Gen Psychiatry. (2010) 67:220–9. doi: 10.1001/archgenpsychiatry.2010.2
25. Mossey JM, Shapiro E. Self-rated health: a predictor of mortality among the elderly. Am J Public Health. (1982) 72:800–8. doi: 10.2105/AJPH.72.8.800
26. Weinberger M, Darnell JC, Tierney WM, Martz BL, Hiner SL, Barker J, et al. Self-rated health as a predictor of hospital admission and nursing home placement in elderly public housing tenants. Am J Public Health. (1986) 76:457–9. doi: 10.2105/AJPH.76.4.457
27. Gunasekara FI, Carter K, Blakely T. Change in income and change in self-rated health: Systematic review of studies using repeated measures to control for confounding bias. Soc Sci Med. (2011) 72:193–201. doi: 10.1016/j.socscimed.2010.10.029
28. Mirowsky J, Ross CE, Reynolds J. Social status and health status. In: Bird CE, Conrad P, Fremont AM, editors. Handbook of Medical Sociology. Upper Saddle River, NJ: Prentice Hall (2000). p. 47–67.
29. Lisko I, Törmäkangas T, Jylhä M. Structure of self-rated health among the oldest old: analyses in the total population and those living with dementia. SSM Popul Health. (2020) 11:100567–100567. doi: 10.1016/j.ssmph.2020.100567
30. Deuchert E, Cabus S, Tafreschi D. A short note on economic development and socioeconomic inequality in female body weight. Health Econ. (2014) 23:861–9. doi: 10.1002/hec.2968
31. Barlow P, McKee M, Basu S, Stuckler D. The health impact of trade and investment agreements: a quantitative systematic review and network co-citation analysis. Global Health. (2017) 13:1. doi: 10.1186/s12992-017-0240-x
32. Ren Y, Li H, Wang X. Family income and nutrition-related health: evidence from food consumption in China. Soc Sci Med. (2019) 232:58–76. doi: 10.1016/j.socscimed.2019.04.016
33. Monteiro CA, Conde WL, Popkin BM. Independent effects of income and education on the risk of obesity in the Brazilian adult population. J Nutr. (2001) 131:881–6. doi: 10.1093/jn/131.3.881S
Keywords: rural elderly, family income, body mass index, self-rated health, fixed effects
Citation: Xin Y and Ren X (2021) The Impact of Family Income on Body Mass Index and Self-Rated Health of Illiterate and Non-illiterate Rural Elderly in China: Evidence From a Fixed Effect Approach. Front. Public Health 9:722629. doi: 10.3389/fpubh.2021.722629
Received: 09 June 2021; Accepted: 23 August 2021;
Published: 17 September 2021.
Edited by:
Demetris Lamnisos, European University Cyprus, CyprusReviewed by:
Mariela Deliverska, Medical University Sofia, BulgariaPetros Galanis, National and Kapodistrian University of Athens, Greece
Copyright © 2021 Xin and Ren. This is an open-access article distributed under the terms of the Creative Commons Attribution License (CC BY). The use, distribution or reproduction in other forums is permitted, provided the original author(s) and the copyright owner(s) are credited and that the original publication in this journal is cited, in accordance with accepted academic practice. No use, distribution or reproduction is permitted which does not comply with these terms.
*Correspondence: Xiaohui Ren, cmVueGlhb2h1aSYjeDAwMDQwO3NjdS5lZHUuY24=