- 1Department of Respiratory and Critical Care Medicine, Shanghai General Hospital, Shanghai Jiao Tong University School of Medicine, Shanghai, China
- 2Department of Gastroenterology, Shanghai General Hospital, Shanghai Jiao Tong University School of Medicine, Shanghai, China
- 3School of Mathematical Sciences, Shanghai Jiao Tong University, Shanghai, China
- 4Department of Infectious Disease, Shanghai General Hospital, Shanghai Jiao Tong University School of Medicine, Shanghai, China
Fever is one of the typical symptoms of coronavirus disease (COVID-19). We aimed to investigate the association between early fever (EF) and clinical outcomes in COVID-19 patients. A total of 1,014 COVID-19 patients at the Leishenshan Hospital were enrolled and classified into the EF and non-EF groups based on whether they had fever within 5 days of symptom onset. Risk factors for clinical outcomes in patients with different levels of disease severity were analyzed using multivariable analyses. Time from symptom onset to symptom alleviation, CT image improvement, and discharge were longer for patients with moderate and severe disease in the EF group than in the non-EF group. Multivariable analysis showed that sex, EF, eosinophil number, C-reactive protein, and IL-6 levels were positively correlated with the time from symptom onset to hospital discharge in moderate cases. The EF patients showed no significant differences in the development of acute respiratory distress syndrome, compared with the non-EF patients. The Kaplan–Meier curve showed no obvious differences in survival between the EF and non-EF patients. However, EF patients with increased temperature showed markedly lower survival than the non-EF patients with increased temperature. EF had no significant impact on the survival of critically ill patients, while an increase in temperature was identified as an independent risk factor. EF appears to be a predictor of longer recovery time in moderate/severe COVID-19 infections. However, its value in predicting mortality needs to be considered for critically ill patients with EF showing increasing temperature.
Introduction
Coronavirus disease (COVID-19) is a serious respiratory disorder that was initially discovered in Wuhan, China. COVID-19 has been shown to be caused by infection with severe acute respiratory syndrome coronavirus (SARS-CoV)-2, a novel coronavirus from the same family as SARS-CoV (1). The CoVs are RNA viruses broadly distributed among mammals and birds; they cause respiratory and intestinal infections in animals and humans (2). Both the severe acute respiratory syndrome (SARS) epidemic in the Guangdong province, China in 2002 and the Middle East respiratory syndrome (MERS) pandemic in the Middle-Eastern countries in 2012 were caused by CoVs (3, 4). SARS-CoV, MERS-CoV, and SARS-CoV-2, which are all β-CoVs, can cause severe respiratory disease in humans (5).
COVID-19 has spread across China and worldwide; the World Health Organization declared it a pandemic within a few months of its first report (6). The patients presented clinical symptoms of dry cough, fever, dyspnea, bilateral lung infiltrates on imaging, and in some cases, gastrointestinal infection symptoms (7). Mild cases typically recover within a week, while patients with more severe forms of the disease show respiratory failure due to alveolar damage and may eventually die (8). There are several factors influencing the outcomes of COVID-19. Non-communicable conditions such as high blood pressure, cardiovascular disease, and diabetes have been reported as risk factors for patients with new coronary disease (9, 10). Patients with hematological malignancies have also been shown to have a higher risk of respiratory infections and serious complications (11).
Fever is an important part of the host defense against infection, playing a key role in increasing the clearance of microorganisms, modulating the cellular immune responses, and inducing heat shock responses (12). During a viral infection, the host mounts an immune response addressed to contain the infection, and fever is regarded as a cornerstone diagnostic sign for screening patients that are potentially infected with COVID-19 (13, 14). Most influenza A strains that infect humans are sensitive to temperature and their replication is inhibited under the body temperature range of 38–41°C. Furthermore, during viral infection, the fever response determines the survival advantage (15). Based on a meta-analysis, Hu et al. reported that fever is the most common symptom in 85.6% of COVID-19 cases during the course of the disease (16). Another study showed that patients with no fever on admission had worse outcomes in the critical/mortality group (17). However, to our knowledge, thus far, no study has focused on the association between early fever (EF) and clinical outcomes in a heterogeneous population of COVID-19 patients with different levels of case severity.
In this study, we compared the clinical outcomes between EF and non-EF patients suffering from moderate, severe, and critical COVID-19 and investigated the value of EF in predicting clinical outcomes in patients with different disease severities.
Materials and Methods
Ethical Approval
The ethics committee of Leishenshan Hospital approved this study (Approval No. 2020-LS04). Informed consent was obtained from all patients prior to being enrolled in the study.
Patients
A total of 1,172 patients diagnosed with COVID-19 and hospitalized at Leishenshan Hospital, a COVID-19-designated hospital in Wuhan, between February 16 and April 14, 2020, were retrospectively reviewed. All cases were diagnosed and confirmed based on the interim guidance issued by the World Health Organization (18). Only adult patients with positive nucleic acid tests for respiratory specimens (nasopharyngeal or oropharyngeal swab samples) or sputum specimens prior to admission were included. Patients with no pneumonia or patients who were transferred to other hospitals were excluded from our study. Finally, we enrolled 1,014 patients after screening using the pre-determined selection criteria (Figure 1).
Leishenshan Hospital is no longer existing. It was officially closed on April 15, 2020 when the epidemic was got controlled in Wuhan. Indeed, the hospital was a temporary one, which was especially built and used for COVID-19 treatment in Wuhan since the local hospital resources were not enough at that time, and the clinicians were from different hospitals across the country. Some authors of our manuscript (Fengming Ding, Yun Feng, Yan Zhou, Yong Ji, Peng-yu Zhang) were members of Shanghai medical supporting team working in Leishenshan. The medical data in our manuscript were collected and used by our team members. We have no issue of data transferring.
Study Design
All patients included in the study were classified into EF or non-EF groups based on whether they had fever within 5 days of symptom onset. The symptoms of COVID-19 included cough, dyspnea, sore throat, thoracic tightness or pain, myalgia, headache, diarrhea, nausea, and consciousness disorder. Fever was defined as a maximum body temperature (Tmax) of more than 37.3°C, measured using an ear thermometer. Based on the Clinical Classification in the Novel Coronavirus Pneumonia Diagnosis and Treatment Guidance from the National Health Commission of China (19), the severity of disease was assessed at the first clinic visit. In brief, moderately ill patients were diagnosed based on fever and respiratory symptoms, as well as radiologic manifestations of pneumonia. Severely ill patients were diagnosed using any of these criteria: (1) difficulty breathing, with more than 30 breaths per minute, (2) oxygen saturation levels <93% at rest, and (3) partial arterial oxygen pressure (PaO2)/fraction of inspired oxygen (FiO2) <300 mmHg (l mmHg = 0.133 kPa). Patients whose chest imaging showed apparent lesion progression of more than 50% within 24–48 h were classified as severe cases. Patients with respiratory failure requiring mechanical ventilation, or shock or organ failure requiring ICU care were classified as critical cases.
Data Collection
Two researchers independently collected the demographic and clinical information by reviewing electronic medical records, including laboratory data, radiographic characteristics, disease severity, treatment protocols, length of hospital stay, disease progression, and clinical outcomes. Symptom data were also estimated and confirmed by direct communication with patients or their families.
The primary outcome for patients with moderate/severe infection was the time from symptom onset to discharge from hospital. Patients who met the following criteria were discharged from the hospital: significant improvement in clinical symptoms and chest CT imaging, at least two consecutive negative nucleic acid tests from respiratory specimens (nasopharyngeal or oropharyngeal swab samples), and sputum specimens with an interval of more than 24 h. Other clinical outcomes included time from symptom onset to symptom alleviation and the recovery of lymphocyte and eosinophil numbers.
The primary outcome for critically ill patients was the time from symptom onset to death. Other clinical outcomes included time from symptom onset to incidence of acute respiratory distress syndrome (ARDS) and complications (i.e., shock, disseminated intravascular coagulation, and multiple organ failure).
Statistical Analysis
Data were analyzed using SAS software (version 8.0; SAS Institute, Cary, NC, USA). GraphPad Prism (version 5; GraphPad Software, San Diego, CA, USA) and R software version 4.0.2 (http://CRAN.R-project.org, R Foundation, Vienna, Austria) were used to generate graphs for visualizing the results. The Kolmogorov-Smirnov test was used to determine the distribution of each variable. Normally distributed quantitative variables were compared using independent t-test, while non-normal distributed variables were compared using Mann-Whitney-test. Qualitative variables were analyzed using chi-squared test. Differences between end-hospitalization and the first clinic visit for each index are shown using Δindex(a) [index(a) at end-hospitalization subtracting index(a) at the first clinic visit]. ΔTmax is the Tmax in the last 3 days before end-hospitalization minus the Tmax from symptom onset to the first clinic visit. Data on demographic, clinical and laboratory tests, and treatments were included in a generalized linear model to estimate correlations between EF and time from symptom onset to hospital discharge in patients with moderate/severe infections. The outcomes of ARDS, complications, and survival in critically ill patients were analyzed using the Kaplan-Meier method followed by the log-rank test to calculate differences between the two groups. A Cox proportional-hazards regression model with stepwise selection was used to identify risk factors for mortality in critical patients. Pairwise Spearman correlations (r) were calculated between indices at two time points (the first clinic visit and end-hospitalization) in EF and non-EF patients. Results with absolute correlations |r| > 0.2 were utilized, and the network was visualized using line widths matched to the strength of correlation. We selected a p-value < 0.05 to denote statistically significant differences.
Results
Patient Characteristics
Baseline characteristics (at the first clinic visit) of the 1,014 patients are shown in Table 1. Patients with EF accounted for 66.6% (n = 675) of the study participants, while non-EF patients made up 33.4% (n = 339) of the participants. EF patients had a mean age of 56.6 ± 15.0 years, and 48.7% were female; non-EF patients were aged 58.8 ± 14.3 years, and 56.3% were female. EF patients tended to be more prone to cough symptoms (66.5 vs. 57.9%, χ2 = 7.38, P = 0.0066), myalgia (24.3 vs. 11.8%, χ2 = 21.93, P < 0.0001), and diarrhea (9.2 vs. 4.4%, χ2 = 7.29, P = 0.0069) compared with non-EF patients. A higher proportion of non-EF patients had comorbidities such as chronic lung diseases (6.2 vs. 2.1%, χ2 = 11.50, P = 0.0007), cardiovascular disease (12.7 vs. 8.4%, χ2 = 4.56, P = 0.0327), and chronic kidney failure (8.3 vs. 4.4%, χ2 = 6.09, P = 0.0136) relative to patients with EF. In addition, EF patients had higher levels of C-reactive protein (CRP, t = 6.34, P < 0.0001), and IL-6 (t = 7.51, P < 0.0001) at the first clinic visit compared with non-EF patients (P < 0.05).
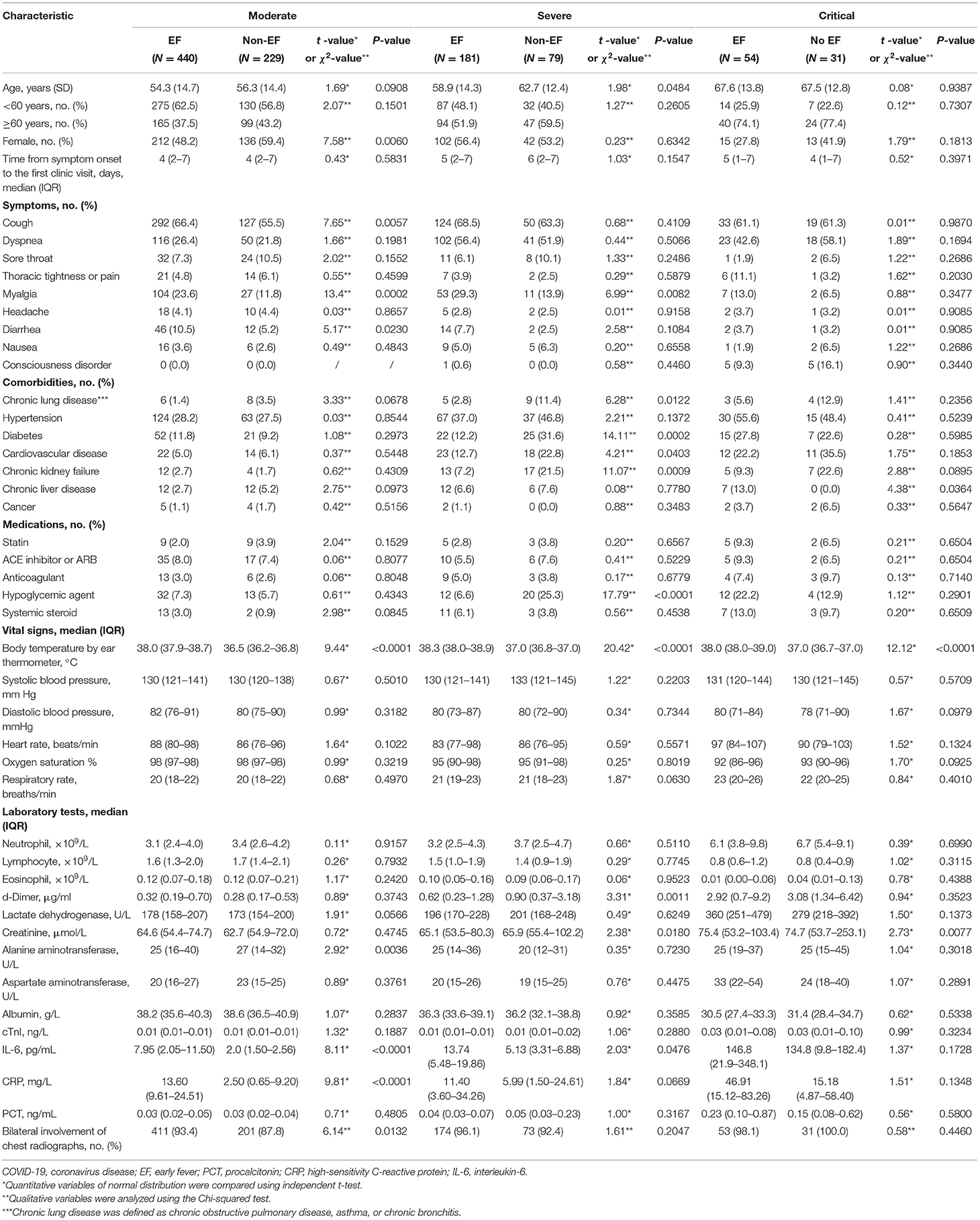
Table 1. Demographics and baseline characteristics of COVID-19 patients with or without early fever.
Based on the clinical classification in the Seventh Version of the Novel Coronavirus Pneumonia Diagnosis and Treatment Guidance from the National Health Commission of China, the 1,014 patients included 669 moderate cases, 260 severe cases, and 85 critical cases. Table 1 shows that the mean age of critical cases was significantly higher than that of severe (t = 2.99, P = 0.0030) or moderate cases (t = 2.68, P = 0.0075). That is, disease severity increased with the age of the patient. EF patients in moderate and severe condition had higher IL-6 level than their non-EF counterparts at the first clinic visit (t = 8.11, P < 0.0001; t = 2.03, P = 0.0476; respectively), but no significant difference of IL-6 was found between EF and non-EF patients in critical condition (t = 1.37, P = 0.1728). The CRP level also showed significant difference between EF and non-EF patients in moderate condition (t = 9.81, P < 0.0001), but no significant difference was found between the two groups in severe and critical condition (t = 1.84, P = 0.0669; t = 1.51, P = 0.1348). By the end of the study, 974 patients had been discharged from the hospital, while 40 had died (one severely ill patient and 39 critically ill patients).
Changes in Body Temperature Following Hospitalization
After admission to the hospital, antiviral therapy was administered to 405 (60.0%) EF patients and 188 (55.5%) non-EF patients (χ2 = 2.05, P = 0.1526). Antibiotic therapy was administered to 298 (44.1%) EF patients and 144 (42.5%) non-EF patients (χ2 = 0.26, P = 0.6129). Systemic glucocorticoid treatment was administered to 45 (6.7%) EF patients and 13 (3.8%) non-EF patients (χ2 = 3.36, P = 0.0670). Among patients who received systemic glucocorticoids, 21 (46.7%) EF patients and 8 (61.5%) non-EF patients were in critical condition (χ2 = 0.89, P = 0.3449) and of these, 11 (52.4%) EF patients and 4 (50%) non-EF patients presented with increased body temperatures at end-hospitalization.
The number of patients with fever in the EF group was significantly reduced after treatment. The number of patients with fever among the severe and moderate cases declined faster than among the critical cases. In the non-EF group, patients developed fever during hospitalization, and most of these were critical cases (Figure 2A). There were no obvious differences in Tmax between EF and non-EF patients after 4 weeks of hospitalization (Figure 2B).
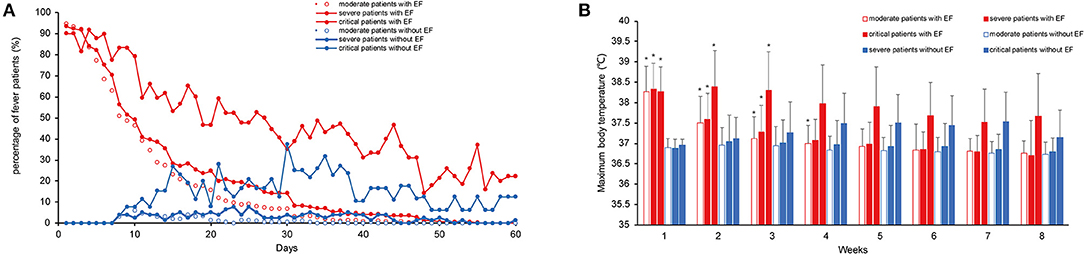
Figure 2. Characteristics of fever in moderate, severe, and critically ill COVID-19 patients with and without EF. Daily percentage of patients with fever in each group (A). Maximum weekly body temperature (B). COVID-19, coronavirus disease; EF, early fever, defined as the incidence of fever (Tmax ≥ 37.3°C, measured using an ear thermometer) within 5 days of symptom onset. Data are presented as the means ± standard deviations in (B). *P < 0.05 for the EF group vs. the non-EF group in the corresponding severity conditions.
Clinical Outcomes in Severe and Moderate Cases
Among the moderately ill cases, patients with EF showed longer time from symptom onset to symptom alleviation, CT image improvement, lymphocyte and eosinophil recovery, nucleic acid tests turning negative, and discharge from hospital compared with non-EF patients (all P < 0.05). Among the severe cases, patients with EF showed longer time periods from symptom onset to symptom alleviation, CT image improvement, and discharge from hospital compared with non-EF patients (all P < 0.05). No obvious differences were detected in the time from symptom onset to nucleic acid tests turning negative, or to lymphocyte or eosinophil recovery (Figure 3).
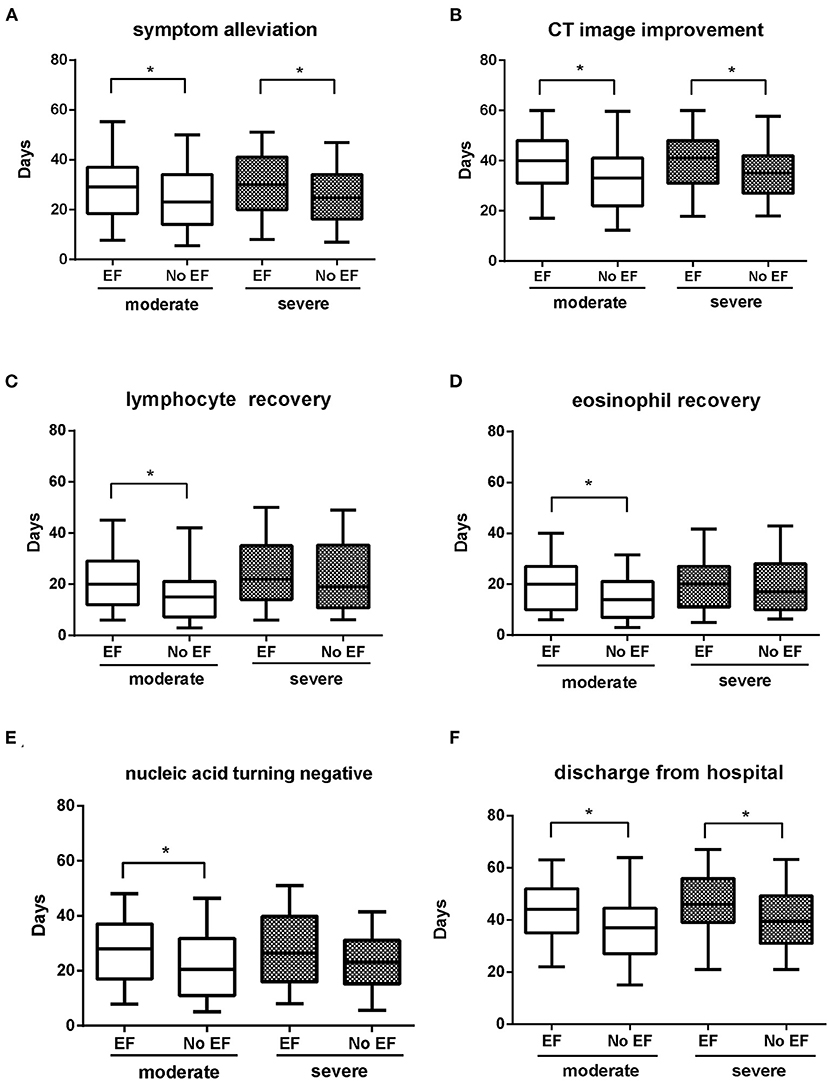
Figure 3. Comparison of clinical outcomes in EF and non-EF COVID-19 patients with moderate/severe infection. Time from symptom onset to symptom alleviation (A); CT image improvement (B); lymphocyte recovery (C); eosinophil recovery (D); nucleic acid tests turning negative (E); time of discharge from hospital (F). Whiskers of the boxplot mark the 5th and 95th percentiles, while the box contains 25th percentile, median, and 75th percentiles. COVID-19, coronavirus disease; EF, early fever, defined as the incidence of fever (Tmax ≥ 37.3°C, measured using an ear thermometer) within 5 days of symptom onset. *P < 0.05 for EF patients vs. non-EF patients in corresponding severity conditions.
Risk Factors for Time From Symptom Onset to Discharge in Moderately or Severely Ill Patients
The primary outcome for moderately or severely ill patients was the time from symptom onset to discharge from hospital. Therefore, we investigated the factors influencing time from symptom onset to hospital discharge in these cases. Demographic, clinical and laboratory tests, and treatments were included in the generalized linear model (Table 2). Multivariable analysis showed that sex, EF, eosinophil number, CRP, and IL-6 levels were correlated with time from symptom onset to hospital discharge among the moderate cases. CRP levels at first clinic visit showed the highest positive correlation with time from symptom onset to hospital discharge (t = 4.26, P < 0.0001), followed by EF (t = 3.01, P = 0.0028) and IL-6 (t = 3.00, P = 0.0028). Among the severe cases, EF showed the most significant correlation with time from symptom onset to hospital discharge (t = 2.42, P = 0.0164), followed by IL-6 (t = 2.07, P = 0.0398). When we compared the results of multivariable analysis between moderately/severely ill patients, the difference in EF coefficient did not reach statistical significance (t = 1.12, P = 0.2647).
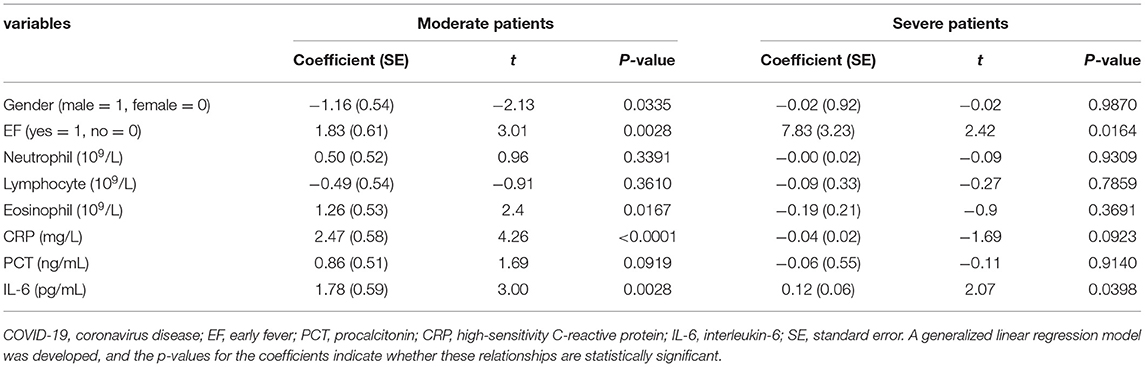
Table 2. Multivariable analysis of time from symptom onset to hospital discharge in COVID-19 patients with moderate and severe infections.
Clinical Outcomes in Critical Cases
Clinical outcomes for critically ill patients included time from symptom onset to death, time from symptom onset to incidence of ARDS, and complications. ARDS developed in 74 patients, including 47 (87%) EF patients and 27 (87.1%) non-EF patients. Three patients with acute exacerbation died without ventilator treatment. Among these, 17 EF patients and 12 non-EF patients received non-invasive respiratory support, including high-flow nasal cannula oxygen therapy (4 EF patients and 8 non-EF patients) and non-invasive mechanical ventilation (13 EF patients and 4 non-EF patients). In addition, 29 EF patients and 13 non-EF patients had invasive respiratory support, including intubation and invasive mechanical ventilation (23 EF patients and 12 non-EF patients) and membrane oxygenation (6 EF patients and 1 non-EF patient). EF patients showed no significant differences in the development of ARDS compared with non-EF patients (HR = 1.13, 95% CI 0.71–1.81, P = 0.6031, Figure 4A).
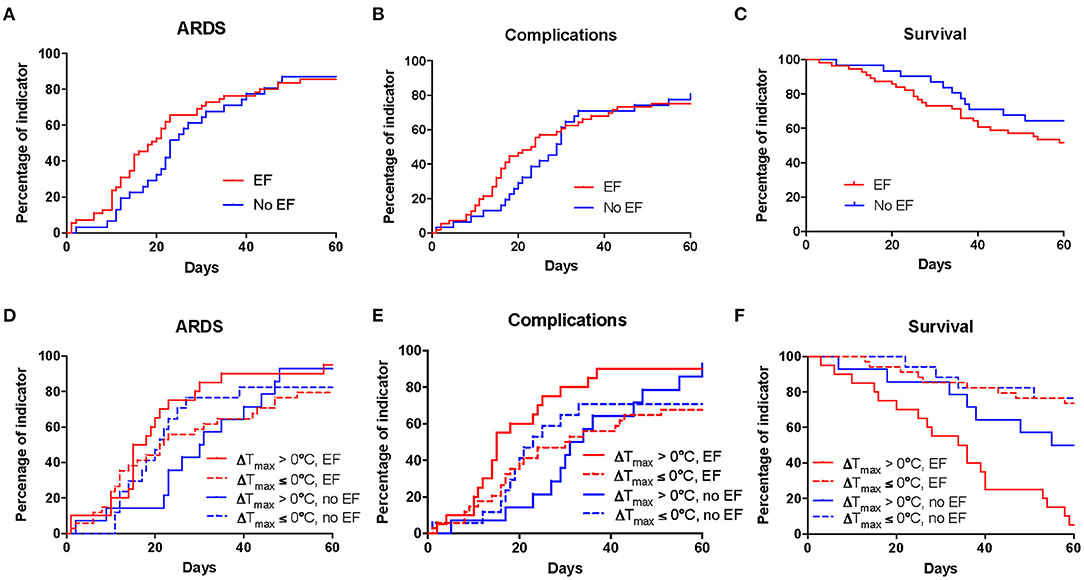
Figure 4. Kaplan-Meier analysis of clinical outcomes in critical COVID-19 patients. Kaplan–Meier curves between EF and non-EF patients for ARDS (A), complications (B), and survival (C) were analyzed. The Kaplan-Meier curves of EF and non-EF patients for ARDS (D), complications (E), and survival (F) after further dividing patients based on ΔTmax. Tmax, maximum body temperature; ΔTmax, the difference between Tmax in the last 3 days before end-hospitalization and Tmax from symptom onset to the first clinic visit; COVID-19, coronavirus disease; EF, early fever, defined as the incidence of fever (Tmax ≥ 37.3°C, measured using an ear thermometer) within 5 days of symptom onset. Complications included shock, disseminated intravascular coagulation, and multiple organ failure.
A total of 63 patients developed complications, including 40 (74.1%) EF patients and 23 (74.2%) non-EF patients, with no significant differences in the development of complications between EF and non-EF patients (HR = 1.14, 95% CI 0.70–1.86, P = 0.6002, Figure 4B). Complications included shock (24 EF patients and 8 non-EF patients), disseminated intravascular coagulation (10 EF patients and 10 non-EF patients), and multiple organ failure (33 EF patients and 23 non-EF patients).
Thirty-nine of the 85 critically ill patients died during the observation period. EF patients accounted for 51.9% (n = 28) of these, while 35.5% (n = 11) were non-EF patients. The KM curve showed no obvious differences in survival between EF and non-EF patients (HR 1.7, 95% CI 0.85–3.1, P = 0.1480, Figure 4C).
EF and non-EF patients were further categorized into the ΔTmax > 0°C and ΔTmax ≤ 0°C groups. The results showed that EF patients with temperature increase (EF patients with ΔTmax > 0°C) had significantly reduced survival compared with non-EF patients with ΔTmax > 0°C (HR 3.10, 95% CI 1.42–6.64, P = 0.0055), while no obvious difference in survival was detected between EF patients with ΔTmax ≤ 0°C and non-EF patients with ΔTmax ≤ 0°C (HR 1.17, 95% CI 0.37–3.66, P = 0.7956). This suggests that an increase in temperature was a risk factor for mortality in patients with EF. There were no statistically significant differences in complications (HR 1.89, 95% CI 0.99–4.03, P = 0.0606) or ARDS (HR 1.77, 95% CI 0.94–3.79, P = 0.0895) between EF patients with temperature increase and non-EF patients with temperature increase (Figures 4D–F).
Risk Factors for Mortality in Critically Ill Patients
Using the Cox proportional-hazards regression model with stepwise selection, risk factors for mortality were identified in critically ill patients. Results showed that EF had no significant impact on the survival of critically ill patients, while an increase in temperature (ΔTmax > 0°C) was identified as an independent risk factor (HR 4.51, 95% CI 1.31–15.54, P = 0.016). Therefore, EF combined with ΔTmax > 0°C was included in the regression model. As shown in Figure 5, EF and ΔTmax > 0°C had a HR of 2.45 (95% CI 1.01–5.92, P = 0.0004), indicating that EF patients with ΔTmax > 0°C were at greater risk of mortality than others. In addition, sex (HR 2.63, 95% CI 1.15–6.04, P = 0.0197), ΔIL-6 > 10 pg/mL (HR 6.58, 95% CI 2.17–19.93, P = 0.0049), ΔCRP > 35 mg/L (HR 11.24, 95% CI 3.91–32.28, P < 0.0001), systemic steroid treatment (HR 8.50, 95% CI 3.59–20.18, P = 0.0320), and a history of chronic lung disease (HR 4.29, 95% CI 1.24–14.80, P = 0.0004) were risk factors for mortality among critical cases. Of these risk factors, ΔCRP > 35 mg/L had the highest hazard ratio.
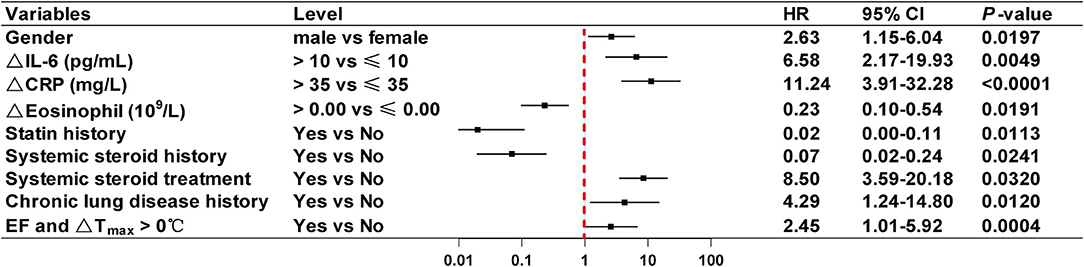
Figure 5. Risk factors for fatal COVID-19 outcome in the multivariate Cox proportional-hazards regression model. Shown in the figure are the hazard ratio (HR) and 95% confidence interval (95% CI) associated with the endpoint. Δ index(a), index(a) end-hospitalization - index(a) at the first clinic visit; Tmax, maximum body temperature; ΔTmax, the difference between Tmax in the last 3 days before end-hospitalization and Tmax from symptom onset to the first clinic visit; COVID-19, coronavirus disease; EF, early fever, defined as the incidence of fever (Tmax ≥ 37.3°C, measured using an ear thermometer) within 5 days of symptom onset. Chronic lung disease was defined as chronic obstructive pulmonary disease, asthma, or chronic bronchitis.
Correlation Network Analysis
The correlation networks between Tmax, the number of immunologic cells, and level of inflammatory biomarkers in EF and non-EF patients are shown in Figure 6. Among moderately/severely ill patients (non-critical), Tmax showed a moderate correlation with IL-6 level (r = 0.48) and a weak correlation with CRP level (r = 0.26) in patients with EF at first clinic visit. No correlations were found between Tmax and both IL-6 and CRP levels in non-EF patients. In addition, there were correlations between CRP, IL-6, and procalcitonin (PCT) levels and neutrophil counts in non-EF patients. At end-hospitalization, Tmax showed no correlation with immunologic cell numbers or inflammatory biomarker levels in either EF or non-EF patients.
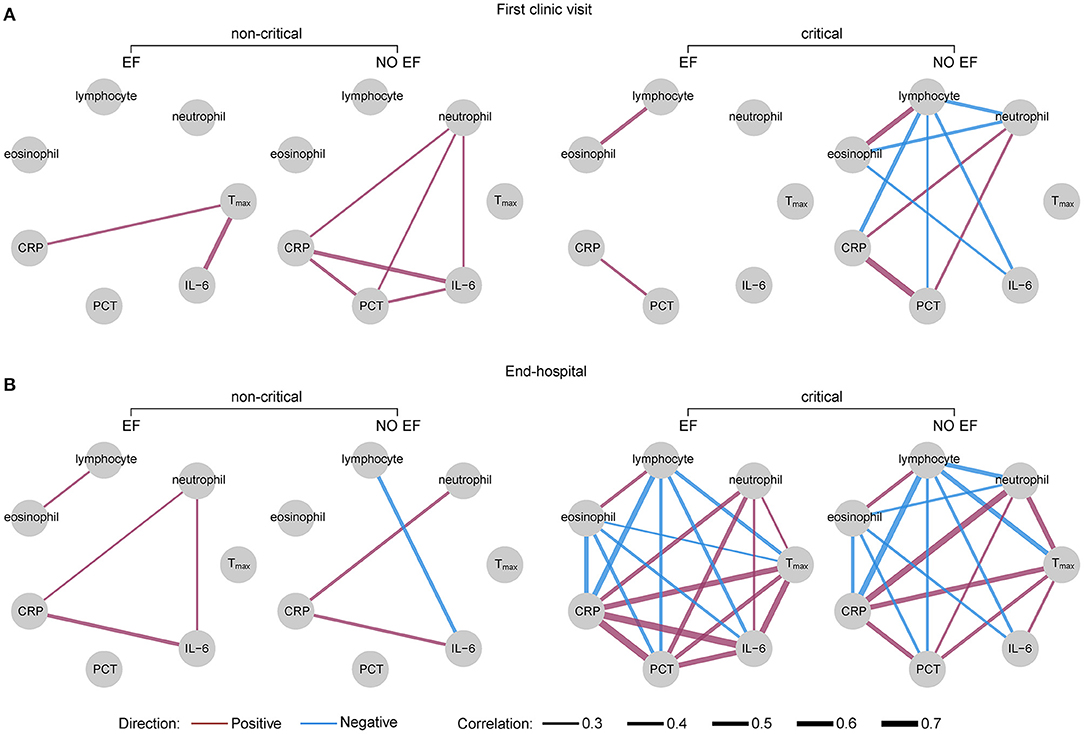
Figure 6. Correlation networks of Tmax, immunologic cells, and biomarkers in EF and non-EF COVID-19 patients. Networks showed different profiles of correlations between EF and non-EF patients in critical and non-critical conditions at the first clinic visit (A) and end-hospitalization (B). The width of the edge showing stronger or weaker interactions is proportional to the absolute value of correlation (|r|). Edges were observed only when |r| > 0.2. A purple edge indicates a positive correlation, and a blue edge indicates a negative correlation. COVID-19, coronavirus disease; EF, early fever, defined as the incidence of fever (Tmax ≥ 37.3°C, measured using an ear thermometer) within 5 days of symptom onset. Tmax, maximum body temperature from symptom onset to the first clinic visit (A), and Tmax in the last 3 days before end-hospitalization (B). PCT, procalcitonin; CRP, high-sensitivity C-reactive protein; IL-6, interleukin-6.
However, the reverse effects were observed in critically ill patients; at the first clinic visit, Tmax showed no correlation with immunologic cell numbers or inflammatory biomarker levels in both EF and non-EF patients. At end-hospitalization, Tmax was positively correlated with neutrophil numbers and CRP, IL-6, and PCT levels, but negatively correlated with lymphocyte numbers in both EF and non-EF patients. The correlation between Tmax and IL-6 levels in EF patients was significantly weaker than in non-EF patients (0.65 vs. 0.27, z = −2.12, P = 0.0170). In addition, Tmax was negatively correlated with eosinophil counts (r = −0.24) in patients with EF, while no correlation was found between Tmax and eosinophils in non-EF patients.
Discussion
Fever is one of the earliest symptoms that COVID-19 patients display when visiting the clinic for treatment, and it is easily measured in these patients (20, 21). In this investigation of clinical outcome in a large sample of COVID-19 patients, we found that EF patients with moderate/severe infection exhibited a longer time period between symptom onset and symptom alleviation, CT image improvement, and discharge from hospital compared with non-EF patients. We also found that EF was significantly positively correlated with time from symptom onset to hospital discharge in patients with moderate/severe infection. In addition, critically ill EF patients whose temperature increased as the disease progressed had worse survival outcomes compared with critically ill, non-EF patients. EF combined with ΔTmax > 0°C was an independent risk factor for mortality in critically ill patients.
Considering that mortality rates were quite different between critically and non-critically ill patients (22), the time to hospital discharge or death were selected as the primary outcomes for moderately/severely ill patients and critically ill patients, respectively. Our data showed that 39 (45.9%) of the 85 critically ill patients died during the observation period, while only one severely ill patient died during the observation period. Factors other than EF that were significantly associated with discharge time in patients with moderate/severe disease included sex, levels of IL-6 and CRP, and eosinophil numbers. All these factors, apart from EF, have been reported in previous studies (23–25). Apart from EF combined with temperature increase, risk factors for mortality in critically ill patients included sex (HR 2.63, 95% CI 1.15–6.04, P = 0.0197), ΔIL-6 > 10 pg/mL (HR 6.58, 95% CI 2.17–19.93, P = 0.0049), ΔCRP > 35 mg/L (HR 11.24, 95% CI 3.91–32.28, P < 0.0001), systemic steroid treatment (HR 8.50, 95% CI 3.59–20.18, P = 0.0320), and a history of chronic lung disease (HR 4.29, 95% CI 1.24–14.80, P = 0.0004). Most of these factors have also been reported in previous studies (26–29). However, to our knowledge, the positive correlations between increased temperature and mortality risk in patients with EF have not been reported.
SARS-CoV-2 infection can stimulate leukocytes to release cytokines, and in critically ill patients, an aberrant uncontrolled response known as “cytokine storm” can be triggered, contributing to lymphopenia, lung injury, and multi-organ failure (30, 31). Of these cytokines, IL-6 is a major pyrogenic cytokine that elevates the core body temperature via thermoregulatory autonomic mechanisms and correlates directly with disease severity (30, 32–34). In this study, we found that EF patients with moderate/severe infection displayed higher IL-6 levels than their non-EF counterparts at first clinic visit, and that there were positive associations between Tmax and IL-6 levels in patients with EF. However, no obvious differences in IL-6 levels were observed at first clinic visit between EF and non-EF patients in critical condition and no correlations between Tmax and IL-6 levels were found in either EF or non-EF patients at the first clinic visit. This phenomenon could be associated with the high proportion of elderly patients who were identified as being in critical condition in this study. It has been reported that elderly patients with COVID-19 have much higher mortality than younger patients and elderly patients often remain sedated or are slow to respond to infection (35–37). Therefore, the temperatures of critically ill patients may not reflect the acute inflammatory responses in early-stage disease, which is consistent with our finding that EF was not an independent risk factor for mortality in critically ill patients. However, our data showed that at end-hospitalization, Tmax of critically ill patients in both EF and non-EF groups were positively correlated with IL-6 levels, and the correlation was significantly stronger in EF patients than in non-EF patients. This suggests that at the late stage, higher temperatures in critically ill, EF patients could reflect higher IL-6 levels, contributing to formation of cytokine storm and increasing the mortality risk.
The use of systemic glucocorticoids in COVID-19 patients remains debatable (38). Based on a meta-analysis, Lu et al. reported that glucocorticoids can reduce the duration of fever in COVID-19 patients; however, no benefits were found in terms of mortality (39). In our study, systemic glucocorticoids were administered to ~30% of critically ill patients, and no significant differences in glucocorticoid treatment outcomes were observed between EF and non-EF patients. Although the use of systemic glucocorticoids may decrease body temperature in patients with fever (40), our data showed that about half of critically ill patients treated with systemic glucocorticoid still presented with elevated temperature at end-hospitalization. In the multivariable analysis, both systemic glucocorticoid treatment and the combination of EF and increase in temperature were independent risk factors for mortality, suggesting that the impact of glucocorticoids on body temperature did not interfere with the predictive value of EF in critically ill patients when their body temperature increased.
Our study had some limitations. First, this was a single-center study; thus, our results should be confirmed using data from multi-center or prospective studies. In addition, the application of NSAIDs and physical cooling in patients with fever may have affected Tmax in the correlation network analysis. However, the impact on the results of the correlation analysis was limited because the NSAIDs and physical cooling were only applied when patients had high fever (>39°C) during hospitalization.
Conclusions
In conclusion, our findings suggest that EF may be a predictor for a long recovery time in moderately/severely ill patients. In critically ill patients, its value in predicting death needs to be considered in cases of elevated temperature. The positive correlation between Tmax and IL-6 levels may play a role in the capacity for EF to predict clinical outcomes.
Data Availability Statement
The raw data supporting the conclusions of this article will be made available by the authors, without undue reservation.
Ethics Statement
The studies involving human participants were reviewed and approved by the Ethics Committee of Leishenshan Hospital approved this study (Approval No. 2020-LS04). The patients/participants provided their written informed consent to participate in this study.
Author Contributions
MZ, P-yZ, F-mD, YF, and LH: conceptualization. F-mD, YF, LH, YZ, YJ, H-jH, Y-sX, D-nY, Z-cX, SL, P-yZ, and MZ: data curation. YZ, YJ, H-jH, Y-sX, D-nY, Z-cX, and SL: formal analysis. MZ: funding acquisition. F-mD, YF, and LH: writing-original draft. MZ and P-yZ: writing-review and editing. All authors contributed to the article and approved the submitted version.
Funding
This work was supported by the Shanghai Jiao Tong University Scientific Research Fund for COVID-19 Prevention and Control (Grant Number YG2020YQ22), the Zhejiang University Special Scientific Research Fund for COVID-19 Prevention and Control (Grant Number 2020XGZX009), the National Natural Science Foundation of China (Grant Numbers 81970006 and 81873402), project of Science and Technology Commission of Shanghai Municipality (Grant Number 20ZR1444300), and First People's Hospital of Shanghai Research Project No. 3 Sub-center Study on Early Identification and Severe Early Warning of Acute Respiratory Infectious Diseases (Grant Number 20Z11900903). The funders had no role in the study design, data collection, analysis, and interpretation, and writing of the report or the decision to submit the paper for publication.
Conflict of Interest
The authors declare that the research was conducted in the absence of any commercial or financial relationships that could be construed as a potential conflict of interest.
The reviewer ZX declared a shared affiliation with the authors to the handling editor at the time of the review.
Publisher's Note
All claims expressed in this article are solely those of the authors and do not necessarily represent those of their affiliated organizations, or those of the publisher, the editors and the reviewers. Any product that may be evaluated in this article, or claim that may be made by its manufacturer, is not guaranteed or endorsed by the publisher.
References
1. Ahn DG, Shin HJ, Kim MH, Lee S, Kim HS, Myoung J, et al. Current status of epidemiology, diagnosis, therapeutics, and vaccines for novel coronavirus disease 2019 (COVID-19). J Microbiol Biotechnol. (2020) 30:313–24. doi: 10.4014/jmb.2003.03011
2. Cheng VC, Lau SK, Woo PC, Yuen KY. Severe acute respiratory syndrome coronavirus as an agent of emerging and reemerging infection. Clin Microbiol Rev. (2007) 20:660–94. doi: 10.1128/CMR.00023-07
3. Drosten C, Günther S, Preiser W, van der Werf S, Brodt HR, Becker S, et al. Identification of a novel coronavirus in patients with severe acute respiratory syndrome. N Engl J Med. (2003) 348:1967–76. doi: 10.1056/NEJMoa030747
4. Zaki AM, van Boheemen S, Bestebroer TM, Osterhaus AD, Fouchier RA. Isolation of a novel coronavirus from a man with pneumonia in Saudi Arabia. N Engl J Med. (2012) 367:1814–20. doi: 10.1056/NEJMoa1211721
5. Cui J, Li F, Shi ZL. Origin and evolution of pathogenic coronaviruses. Nat Rev Microbiol. (2019) 17:181–92. doi: 10.1038/s41579-018-0118-9
6. Kannan S, Shaik Syed Ali P, Sheeza A, Hemalatha K. COVID-19 (Novel Coronavirus 2019) - recent trends. Eur Rev Med Pharmacol Sci. (2020) 24:2006–11. doi: 10.26355/eurrev_202002_20378
7. Pascarella G, Strumia A. COVID-19 diagnosis and management: a comprehensive review. J Intern Med. (2020) 288:192–206. doi: 10.1111/joim.13091
8. Adhikari SP, Meng S, Wu YJ, Mao YP, Ye RX, Wang QZ, et al. Epidemiology, causes, clinical manifestation and diagnosis, prevention and control of coronavirus disease (COVID-19) during the early outbreak period: a scoping review. Infect Dis Poverty. (2020) 9:29. doi: 10.1186/s40249-020-00646-x
9. Li B, Yang J, Zhao F, Zhi L, Wang X, Liu L, et al. Prevalence and impact of cardiovascular metabolic diseases on COVID-19 in China. Clin Res Cardiol. (2020) 109:531–8. doi: 10.1007/s00392-020-01626-9
10. Muniyappa R, Gubbi S. COVID-19 pandemic, coronaviruses, and diabetes mellitus. Am J Physiol Endocrinol Metab. (2020) 318:E736–41. doi: 10.1152/ajpendo.00124.2020
11. Borah P, Mirgh S, Sharma SK, Bansal S, Dixit A, Dolai TK, et al. Effect of age, comorbidity and remission status on outcome of COVID-19 in patients with hematological malignancies. Blood Cells Mol Dis. (2021) 87:102525. doi: 10.1016/j.bcmd.2020.102525
12. Launey Y, Nesseler N, Mallédant Y, Seguin P. Clinical review: fever in septic ICU patients–friend or foe? Crit Care (London, England). (2011) 15:222. doi: 10.1186/cc10097
13. Mao B, Liu Y, Chai YH, Jin XY, Lu HW, Yang JW, et al. Assessing risk factors for SARS-CoV-2 infection in patients presenting with symptoms in Shanghai, China: a multicentre, observational cohort study. Lancet Digital Health. (2020) 2:e323–30. doi: 10.1016/S2589-7500(20)30109-6
14. Huang T, Guo Y. Application and effects of fever screening system in the prevention of nosocomial infection in the only designated hospital of coronavirus disease 2019 (COVID-19) in Shenzhen, China. Infect Control Hosp Epidemiol. (2020) 41:978–81. doi: 10.1017/ice.2020.119
15. Young PJ, Saxena M. Fever management in intensive care patients with infections. Crit Care (London, England). (2014) 18:206. doi: 10.1186/cc13773
16. Hu Y, Sun J, Dai Z, Deng H, Li X, Huang Q, et al. Prevalence and severity of corona virus disease 2019 (COVID-19): a systematic review and meta-analysis. J Clin Virol. (2020) 127:104371. doi: 10.1016/j.jcv.2020.104371
17. Mo P, Xing Y, Xiao Y, Deng L, Zhao Q, Wang H, et al. Clinical characteristics of refractory COVID-19 pneumonia in Wuhan, China. Clin Infect Dis. (2020) ciaa270. doi: 10.1093/cid/ciaa270. [Epub ahead of print].
18. World Health Organization. Coronavirus disease 2019 (COVID-19) Situation Report-92. World Health Organization (2020). Available online at: https://www.who.int/docs/default-source/coronaviruse/situation-reports/202004 25921-sitrep-92-covid-19.pdf?sfvrsn=38e6b06d_4
19. Wei PF. Diagnosis and treatment protocol for novel coronavirus pneumonia (trial version 7). Chin Med J. (2020) 133:1087–95. doi: 10.1097/CM9.0000000000000819
20. Huang C, Wang Y, Li X, Ren L, Zhao J, Hu Y, et al. Clinical features of patients infected with 2019 novel coronavirus in Wuhan, China. Lancet (London, England). (2020) 395:497–506. doi: 10.1016/S0140-6736(20)30183-5
21. Xu XW, Wu XX, Jiang XG, Xu KJ, Ying LJ, Ma CL, et al. Clinical findings in a group of patients infected with the 2019 novel coronavirus (SARS-Cov-2) outside of Wuhan, China: retrospective case series. BMJ. (2020) 368:m606. doi: 10.1136/bmj.m606
22. Wiersinga WJ, Rhodes A, Cheng AC, Peacock SJ, Prescott HC. Pathophysiology, transmission, diagnosis, and treatment of coronavirus disease 2019 (COVID-19): a review. JAMA. (2020) 324:782–93. doi: 10.1001/jama.2020.12839
23. Wang F, Qu M, Zhou X, Zhao K, Lai C, Tang Q, et al. The timeline and risk factors of clinical progression of COVID-19 in Shenzhen, China. J Transl Med. (2020) 18:270. doi: 10.1186/s12967-020-02423-8
24. Cheng B, Hu J, Zuo X, Chen J, Li X, Chen Y, et al. Predictors of progression from moderate to severe coronavirus disease 2019: a retrospective cohort. Clin Microbiol Infect. (2020) 26:1400–5. doi: 10.1016/j.cmi.2020.06.033
25. Xie G, Ding F, Han L, Yin D, Lu H, Zhang M. The role of peripheral blood eosinophil counts in COVID-19 patients. Allergy. (2021) 76:471–82. doi: 10.1111/all.14465
26. Chen R, Sang L, Jiang M, Yang Z, Jia N, Fu W, et al. Longitudinal hematologic and immunologic variations associated with the progression of COVID-19 patients in China. J Allergy Clin Immunol. (2020) 146:89–100. doi: 10.1016/j.jaci.2020.05.003
27. Zheng Z, Peng F, Xu B, Zhao J, Liu H, Peng J, et al. Risk factors of critical & mortal COVID-19 cases: a systematic literature review and meta-analysis. J Infect. (2020) 81:e16–25. doi: 10.1016/j.jinf.2020.04.021
28. Zhang JJ, Cao YY, Tan G. Clinical, radiological, and laboratory characteristics and risk factors for severity and mortality of 289 hospitalized COVID-19 patients. Allergy. (2021) 76:533–50. doi: 10.1111/all.14496
29. Luo X, Zhou W, Yan X, Guo T, Wang B, Xia H, et al. Prognostic value of C-reactive protein in patients with COVID-19. Clin Infect Dis. (2020) 71:2174–9. doi: 10.1101/2020.03.21.20040360
30. Gao YM, Xu G, Wang B. Cytokine storm syndrome in coronavirus disease 2019: A narrative review. J Intern Med. (2020) 289:147–61. doi: 10.1111/joim.13144
31. Abdin SM, Elgendy SM, Alyammahi SK, Alhamad DW, Omar HA. Tackling the cytokine storm in COVID-19, challenges and hopes. Life Sci. (2020) 257:118054. doi: 10.1016/j.lfs.2020.118054
32. Netea MG, Kullberg BJ, Van der Meer JW. Circulating cytokines as mediators of fever. Clin Infect Dis. (2000) 31(Suppl. 5):S178–84. doi: 10.1086/317513
33. Nishimoto N, Kishimoto T. Interleukin 6: from bench to bedside. Nat Clin Pract Rheumatol. (2006) 2:619–26. doi: 10.1038/ncprheum0338
34. Abbasifard M, Khorramdelazad H. The bio-mission of interleukin-6 in the pathogenesis of COVID-19: a brief look at potential therapeutic tactics. Life Sci. (2020) 257:118097. doi: 10.1016/j.lfs.2020.118097
35. Li T, Lu L, Zhang W, Tao Y, Wang L, Bao J, et al. Clinical characteristics of 312 hospitalized older patients with COVID-19 in Wuhan, China. Arch Gerontol Geriatr. (2020) 91:104185. doi: 10.1016/j.archger.2020.104185
36. Wester AL, Dunlop O, Melby KK, Dahle UR, Wyller TB. Age-related differences in symptoms, diagnosis and prognosis of bacteremia. BMC Infect Dis. (2013) 13:346. doi: 10.1186/1471-2334-13-346
37. Hernández C, Fehér C, Soriano A, Marco F, Almela M, Cobos-Trigueros N, et al. Clinical characteristics and outcome of elderly patients with community-onset bacteremia. J Infect. (2015) 70:135–43. doi: 10.1016/j.jinf.2014.09.002
38. Yang JW, Yang L, Luo RG, Xu JF. Corticosteroid administration for viral pneumonia: COVID-19 and beyond. Clin Microbiol Infect. (2020) 26:1171–7. doi: 10.1016/j.cmi.2020.06.020
39. Lu S, Zhou Q, Huang L, Shi Q, Zhao S, Wang Z, et al. Effectiveness and safety of glucocorticoids to treat COVID-19: a rapid review and meta-analysis. Ann Transl Med. (2020) 8:627. doi: 10.21037/atm-20-3307
Keywords: early fever, clinical outcomes, COVID-19, severity, interleukin-6
Citation: Ding F-m, Feng Y, Han L, Zhou Y, Ji Y, Hao H-j, Xue Y-s, Yin D-n, Xu Z-c, Luo S, Zhang P-y and Zhang M (2021) Early Fever Is Associated With Clinical Outcomes in Patients With Coronavirus Disease. Front. Public Health 9:712190. doi: 10.3389/fpubh.2021.712190
Received: 20 May 2021; Accepted: 02 August 2021;
Published: 26 August 2021.
Edited by:
Shisan Bao, The University of Sydney, AustraliaReviewed by:
Jinpeng Li, Wuhan University, ChinaZhongqing Xu, Shanghai Jiao Tong University School of Medicine, China
Copyright © 2021 Ding, Feng, Han, Zhou, Ji, Hao, Xue, Yin, Xu, Luo, Zhang and Zhang. This is an open-access article distributed under the terms of the Creative Commons Attribution License (CC BY). The use, distribution or reproduction in other forums is permitted, provided the original author(s) and the copyright owner(s) are credited and that the original publication in this journal is cited, in accordance with accepted academic practice. No use, distribution or reproduction is permitted which does not comply with these terms.
*Correspondence: Min Zhang, bXpoYW5nNzRAMTYzLmNvbQ==; Peng-yu Zhang, emhhbmdwZW5neXUxOTczQGhvdG1haWwuY29t
†These authors have contributed equally to this work