- 1Business School, Shandong University, Weihai, China
- 2Department of Logistics, Service & Operations Management, Korea University Business School, Seoul, South Korea
- 3Department of International Logistics, Chung-Ang University, Seoul, South Korea
This study aims to understand the influence of COVID-19 on consumers' fears and self-protection motivations. Furthermore, the study seeks to understand the effects of these fears and motivations on consumers' intentions to use omnichannel retailing. A modified theoretical model is proposed by integrating protection motivation theory (PMT) and extending the extended parallel process model (E-EPPM). A total of 398 valid questionnaires are collected and used for further structural equation modeling analysis. The results suggest that the perceived severity, perceived vulnerability, and health anxiety positively impact perceived fears surrounding COVID-19. Furthermore, it is found that perceived fear, self-efficacy, and response efficacy will affect the protection motivation of consumers and ultimately contribute to their behavioral intention to use omnichannel retailing. The findings theoretically enrich the research on COVID-19, PMT, and E-EPPM and empirically provide managerial implications for omnichannel retail service providers.
Introduction
In recent years, retail service providers have been witnessed to actively integrate and align physical and digital resources, supply chains, and logistics networks in pursuit of a new business model, namely, omnichannel retailing (e.g., Apple, Suning, and Starbucks) (1–3). It is considered an innovative practice to provide consumers with more reliable and seamless channel-agnostic services (4–7). Admittedly, the adoption of multichannel retail services that do business in both online and offline channels has catalyzed better firm performance over the past decades (8). However, the channels are relatively parallel in such settings, which means channel migration in end-to-end shopping activities (from pre-shopping to post-shopping) often encounters barriers. For example, a product bought from the online channel cannot be returned through the offline channel. With the advent of omnichannel retail services, such boundaries can be erased by enabling consumers to choose their preferred end-to-end shopping method. The tenet is that, in any event, no channel barrier would be anticipated by consumers at all stages of the shopping journey (i.e., the interaction between consumer and service provider), which includes, but is not limited to, the process of selection, evaluation, procurement, and after-sale services. In an omnichannel setting, channels are integrated and can be freely chosen by consumers. For instance, one can place an order on the website or by visiting a physical store. After the order is submitted, the buyer can choose home delivery or to collect the product by visiting a service point, and the buyer can also request a pick-up service or visit a service point to complete the return personally if he/she need to return the product (9–11).
Scholars have revealed that omnichannel retailing can benefit stakeholders in various ways. From the service provider's perspective, the operations of omnichannel retailing can translate to increased sales, enhanced revenue, and profitability (4, 12–14). For example, the transformation to omnichannel creates brand equity, such as positive word-of-mouth (15), which potentially attracts more engaged customers. Also, relationships with the existing customer base may deepen due to improved shopping experiences, such as reduced uncertainty and greater agility, leading to better customer retention (16–18). From the customer's perspective, omnichannel retailing can contribute to consumers' opportunity cost savings. For example, omnichannel retailing allows consumers to browse, feel and assess products at their convenience through physical or virtual showrooms (13). Also, consumers' hedonic benefits can be realized through engagement in omnichannel shopping. For example, they may consider arranging purchases and pick-ups according to their preferences a pleasurable activity. Research on omnichannels has been considerably surging because it is especially relevant (8, 19, 20). However, consistent with the review of Mishra et al. (21), it should be noted that few studies have focused on the cognitive dimensions of consumer decision-making in adopting omnichannel retailing. In addition, as they claimed that “we could not find a single review paper linking consumer decision making with behavioural theories,” the theoretical foundation related to the consumer perspective is rather underdeveloped.
Moreover, since the massive shock of the ongoing pandemic has changed the business environment and disrupted the retail industry (22–26), we argue that it is necessary to consider the role of COVID-19 in our research model. Indeed, literature on changes in consumer behavior is surging (10, 11, 27–29). Some scholars have reported that COVID-19 has led consumers to panic buy, and they investigated the drivers of this phenomenon (27, 30). Some have argued that consumers' safety and health concerns have catalyzed their dependence on contactless shopping technologies, such as using augmented reality technologies in the information acquisition process (31), live-stream shopping in purchasing (32), and robot or drone deliveries in last-mile logistics (9). Others focused on how the social properties of shopping have been affected by the pandemic. For instance, Wang et al. (28) predicted that COVID-19 would result in the emergence of a massive community of “lonely” consumers who were deeply enveloped in social isolation. Wang et al. (29) analyzed consumer privacy protection in the face of rapidly emerging online shopping. However, to date, knowledge on channel switching behavior under COVID-19 is rather underdeveloped. Specifically, most of the current literature is based on single-channel or multichannel settings, e.g., from brick-and-mortar to online shopping (33), and these arguments may be ill-suited in an omnichannel context.
We aim to fill the knowledge gap by developing a theoretically driven model to identify the antecedents of consumers' adopting omnichannel retailing during the COVID-19 pandemic. In this study, protection motivation theory (PMT) (34) and the extension of the extended parallel process model (E-EPPM) (35) are introduced as theoretical lenses to build the conceptual framework. PMT and E-EPPM are chosen because they consider not only the evaluation of the technology, such as self-efficacy and response efficacy, but also how the external environment creates changes in their intentions to use the technology. The theories are proposed because of their appropriateness for studying the adoption behavior of technologies and COVID-19 research (36–38). This study makes the following academic contributions. First, our research empirically enriches the omnichannel literature by focusing on a consumer behavioral perspective, which is different from past studies mainly located at the organizational level (39, 40). Second, we also contribute to the literature by extending the understanding of the determinants of consumer decision-making in the omnichannel context. By conceptualizing the PMT and E-EPPM model, we explore how consumers' intentions to use omnichannel shopping can be motivated by irrational factors (e.g., fear of COVID-19 and health concerns). This approach is distinct from previous research that has primarily investigated the determinants of consumers' behavioral intentions to use omnichannel retailing through rational-based decisions (5, 41).
The remainder of this study proceeds as follows. The theoretical foundation and hypotheses are presented in the next section. Section Methodology describes the research methodology and data collection. Next, section Results and Discussions covers the data analysis as well as hypotheses testing. The results are then presented and discussed, and section Conclusions summarizes the contributions, limitations, and recommendations for future research.
Literature Review and Hypotheses Development
Retailing Channels
Since the last two decades, the retailing industry has experienced a “single-multi-omni” evolution process. “Single” refers to retail service providers that sell products or services to customers through only one distribution channel. In this regard, the single-channel model may include the traditional business model (e.g., brick and mortar stores, catalogs, mail-order) or e-commerce (i.e., online stores) (42, 43). A “multi” retailer is a firm that adopts a hybrid sales structure by utilizing a variety of different channels as additional service options from their traditional sales structures (4, 44). Therefore, by definition, the difference between the single-channel and multichannel is the number of channels available to consumers. In recent years, both traditional retailers (e.g., Best Buy and Walmart) and online retailers (Amazon and Xiaomi) have been extending their business from “single” to “multi,” and it has been reported that such strategic changes are helping their financial performance (8). However, it should be noted that channels within the multichannel retailing setting are relatively parallel; that is, the design and operations of these channels are rather independent. For example, transaction data and customer management are not well-integrated, the information transition in different channels is occasionally mismatched, and the “touch-and-feel” is hindered from the online shopping context (45, 46). In this regard, being “omni” has obtained much attention as a new business model aimed at integrating all available channels within a firm and eliminating the barriers among these channels (47). Specifically, omnichannel is defined as “a unified approach that manages channels as intermingled touch points to allow consumers to have a seamless experience within an ecosystem” (4, 10, 11, 28, 29, 46). In the omnichannel context, consumers are not limited by the channels' characteristics and can migrate across them without any information loss or reiteration.
However, implementing an omnichannel practice is not without difficulties. Some studies have identified a variety of challenges and opportunities for firms' transforming to omnichannels. For example, to operate consistently, “omni” requires the fundamental alignment of firms' strategies (18, 48), whereas, in practice, misalignment happens due to conflicts of interest between channels, organizational silos, reduced communication, the isolation of databases, and low logistics service quality (47–51). Despite the significance of these studies, most current studies lack a theory guide, with theoretical research from a consumer behavior perspective only, surprisingly, just starting to emerge. Table 1 summarizes some representative research that is theoretically guided. Some studies have examined channel integration, resource configuration, and operational quality under omnichannel retailing from the view of dynamic capabilities (47, 51), social exchange theory (15), and resource-based theory (53, 54). However, few studies have been conducted to explain consumers' usage behavior, which motivates this study.
Theoretical Background
The theoretical background for this study stems from the protection motivation theory (PMT) (34) and the extension of the extended parallel process model (E-EPPM) (35). PMT theorizes that people's decisions to undertake self-protective actions when facing a noxious event are broadly initiated and maintained through two cognitive appraisal processes: threat appraisal and coping appraisal (34, 55, 56). In other words, whether to adopt the recommended measure to protect themself is contingent on their assessment of the threat magnitude of the event (threat appraisal) and the effectiveness of the recommended measure (coping appraisal). If an event is considered harmful and the recommended coping measures have high perceived efficacy, as well as low costs, they would exhibit a high motivation to use the recommended coping measures to protect themselves from being influenced or, more seriously, being injured (57).
Threat appraisal refers to one's assessment of the perceived severity and vulnerability, which examines the potential severity of an event (i.e., perceived severity) and what (mostly negative) consequences it would probably lead to (i.e., perceived vulnerability). A coping appraisal consists of three constructs, namely, self-efficacy, response efficacy, and response costs, which refers to one's evaluation of the following: (a) beliefs or confidence in their ability to engage in the coping response (i.e., self-efficacy); (b) the degree of perceived efficiency of the recommended response in avoiding the threat (i.e., response efficacy); and (c) costs would incur when the recommended response is taken (i.e., response costs). To illustrate: when considering whether to contribute to reducing greenhouse emissions through the use of public transportation, an individual would evaluate the risk caused by greenhouse emissions (perceived severity) and the individual's susceptibility to the risk of greenhouse emissions (perceived vulnerability). At the same time, the individual would also assess their capability to use public transport (self-efficacy), the effectiveness of public transport in reducing air pollution (response efficacy), and the costs associated with using public transport (response costs).
There is consistent evidence that PMT is a robust model for explaining and promoting health and safety-related attitudes and behaviors (36, 38), and “can be applied to any threat that an individual can assess and respond to accordingly” (55). In fact, it has been widely utilized in a diverse disciplines, such as medical care (36, 58, 59); environmental sciences (60–62); information security (63–65); and transportation (66–68). Recently, it has been observed that extensive epidemiological research employing PMT to study behavioral or psychological changes related to COVID-19 has been conducted. For example, the fear of traveling (69) and social distancing (70, 71). Therefore, we believe that PMT could be used as the foundation of our research model. Consistent with much PMT-related literature that excluded response costs from the research framework [e.g., see (33, 72)], responses costs were excluded in our conceptual framework. This approach was taken since numerous studies have suggested that omnichannel retailing is a novel technology that forms a seamless retail world for consumers, and the adoption of such shopping methods does not require additional efforts and does not cause advantage losses to other shopping methods (11, 29, 73).
Indeed, the evolving COVID-19 pandemic has caused much psychological fallout, the most prominent consequence of which can be concluded as fear. Although PMT includes, to some extent, the effect of affective assessments on protection motivation, the role of fear as an antecedent is ignored. This drawback can be addressed by introducing EPPM (74), and the cognitive appraisal theory of emotions (75). It holds that an individual's threat appraisal of an event is triggered by fear, and a coping appraisal would be provoked when the degree of fear somewhat exceeds a specific threshold. However, Witte (76) sought to adequately explain the association between perceptions and behaviors by pointing out that the tenets of EPPM (i.e., “putting the fear back into fear appeals” and “heavy focus on cognition”) can lead to biased outcomes and the failure. Therefore, the extension of the extended parallel process model (E-EPPM) is chosen as part of our conceptual framework to provide a better theoretically-grounded explanation. Different from EPPM, E-EPPM distinguishes itself by redefining constructs such as perceived risk. Also, a new component, i.e., anxiety, is considered a critical factor in the model. Since fear and anxiety are two different emotions and have distinct central thematic associations, we believe that the role of anxiety as an antecedent also requires examination (77). Moreover, since extensive research has suggested a tight linkage among COVID-19, fear, anxiety, and behaviors (37, 78, 79), we argue that E-EPPM is more suitable for this context defined by the COVID-19 pandemic. The study's dependent variable is consumers' behavioral intentions, and, in the context of this research, it is defined as the aim and plan of repeatedly using omnichannel retail services for their shopping activities (10, 11).
Drawing on the interpretation of the theories, we propose a modified theoretical model to investigate the antecedents influencing consumers' behavioral intentions to use omnichannel retailing by incorporating the theoretical lens of PMT and E-EPPM, which is depicted in Figure 1.
Hypotheses Development
In this study, perceived severity is defined as an individual's evaluation of the negative consequences of the COVID-19 pandemic, while perceived fear refers to their psychological distress due to these consequences (80, 81). Earlier studies have confirmed a positive relationship between perceived severity and perceived fear (82–84). In the context of COVID-19, its impact on people's health and livelihoods is considered a possible consequence that leads to their perceived fear. Indeed, as of December 28, 2021, more than 280 million cases, including over 5 million COVID-19 deaths, have been reported worldwide (85). Critically, the ongoing pandemic is still yet to be effectively controlled, with significant outbreaks occurring from time to time in certain areas (e.g., South Korea, India, and Colombia). Thus, we argue that such a rapidly evolving situation will likely evoke people's fear-based emotions. Therefore, the following hypothesis is proposed:
H1. The perceived severity of COVID-19 positively influences the perceived fear of COVID-19.
Perceived vulnerability refers to an individual's evaluation of the probability that the depicted event may potentially harm them. According to an empirical study conducted in Belgium, significant results reflected that respondents perceived themselves as susceptible to COVID-19 (86), noting that, while it is generally accepted that the less time people stay outdoors, the safer they will be (i.e., stay-at-home policies), the findings were not influenced by respondents' commuting distances. This outcome is supported by a cross-country study, which indicated that respondents perceived threats, as well as fear of being affected by COVID-19, as high (87). Thus, the following hypothesis is proposed:
H2. The perceived vulnerability to COVID-19 positively influences the perceived fear of COVID-19.
Despite perceived severity and vulnerability being included in our model for the COVID-19 threat appraisal, the study also considers the role of anxiety because E-EPPM posits that anxiety is critical to an individual's threat perception and plays an essential role in stimulating protective responses (35). Since COVID-19 is considered a health crisis, we narrow the variable anxiety to a more health-focused perspective, i.e., health anxiety. In this study, health anxiety is defined as a person's apprehension or concern about their health status in the context of COVID-19 (79, 88). Many studies have indicated that the person who is always suspicious that that have, or are suffering from, a serious illness tends to have a higher perceived fear (89–91). Moreover, according to So et al. (77), anxiety is another critical factor, other than perceived fear, that is expected to have an essential impact on protection motivation. Along the same lines, Knowles and Olatunji (92) supported this view by illustrating a causal relation between anxiety and safety behaviors. On this basis, the following is hypothesized:
H3. Health anxiety positively influences perceived COVID-19 fear.
H4. Health anxiety positively influences protection motivation.
Many researchers believe there is a positive correlation between perceived fear and protective motivation (37, 93–95). For example, Yuen et al. (96) reported that panic buying occurred globally at the outbreak of COVID-19 because the sudden emergence of the disease made people feel threatened and induced fear of the unknown, thus somehow motivating them to stockpile large amounts of supplies as their protective behavior. In addition, the consumption behavior relating to the siginificant surge in the consumption of personal protective equipment out of fear of contracting COVID-19 was witnessed, and the increasing trend was strongly tied to the pandemic (93). Therefore, the following hypothesis is proposed:
H5: Perceived COVID-19 fear affects protection motivation positively.
To reiterate: two components are considered in the coping appraisal, i.e., self-efficacy and response efficacy. In the context of this study, self-efficacy is defined as the expectancy of an individual's ability in performing omnichannel retailing as the recommended coping behavior, while response efficacy is the degree to which an individual believes that using omnichannel retailing will be an effective means to avoid being harmed or infected by COVID-19. Consumers who perceive the use of omnichannel retailing as useful in protecting themselves from COVID-19 are likely to be more motivated to take protective action. Furthermore, consumers who assess themselves as being able to utilize omnichannel retailing are also more likely to take protective measures. PMT predicts that both self-efficacy and response efficacy will positively impact protection motivation (94). Therefore, the following hypotheses are proposed:
H6. Self-efficacy positively influences protection motivation.
H7. Response efficacy positively influences protection motivation.
Protecting motivation lies at the core of PMT (34, 55). However, since the protection motivation should precede actual action of using the recommended coping behavior in the pandemic, there is a tendency to explore whether such motivation would transfer to consumers' actual behavior in using omnichannel retailing. According to the World Health Organization, it is important to take precautions, such as staying at home, social distancing, and refraining from spending long periods in crowded environments (97). We argue that consumers' protection motivation is positively related to their use of omnichannel retailing since it can support these suggestions in several ways. For example, by utilizing the flexible options of purchase and pick-up, consumers may use augmented reality technology to select and experience items on their mobile devices. They may then choose online delivery or collect them during low customer flow hours (buy online–in-store-pick-up), thus avoiding extra time and effort, thereby reducing close contact with others. Moreover, in the context of omnichannel retailing, consumers are also allowed to use multiple channels. For example, consumers can ask the retailer to collect the return or randomly bring the items to any retail store at their convenience. This scenario further reinforces the role of omnichannel retail in helping consumers choose the proper engagement method to avoid exposure to relatively dangerous environments. In addition, many studies have reported that COVID-19 has resulted in panic buying because people were motivated to hoard necessities as their coping behavior (27, 30). In this regard, consumers may be more likely to use omnichannel retail services because they have better dynamic information processing capabilities for external conditions (7), and by extension, higher supply chain resilience. Such an improved business model would encounter fewer shortages and, thus, better meet consumer demand. Based on the above discussion, the following hypothesis is proposed:
H8. An increase in consumers' protection motivation increases their behavioral intention to use omnichannel retailing.
Methodology
Survey Design and Measurement Items
A survey methodology that is consistent with existing quantitative protection motivation and omnichannel retailing research was adopted to test the hypotheses empirically [see Bulgurcu et al. (98), Ifinedo (99), Lee et al. (15), and Xu and Jackson (5)]. The questionnaire consists of three sections. Section Introduction was the description, including the background and objective of the survey and an explanation of omnichannel retailing. We sought to ensure common understanding by focusing more on providing straightforward examples describing the usage scenario rather than technical terms to explain the working mechanism of omnichannel retailing. Section Literature Review and Hypotheses Development contained a survey of social-demographic characteristics, including gender, age, education, monthly income, and the most frequently used payment method in daily life. Section Methodology contained items for measuring the eight latent variables in our conceptual model: perceived severity, perceived vulnerability, perceived fear, health anxiety, self-efficacy, response efficacy, protection motivation, and behavioral intention. A screening question was added at the beginning of section Methodology to ensure that respondents fully understood the instructions and answered the questions carefully. It was a multiple-choice question that asked respondents to select the example that was not omnichannel retailing. Furthermore, another question requiring respondents to select “strongly agree” was also inserted in section Methodology.
All items were developed from relevant previous research, and appropriate modifications were made in consultation with a review panel (e.g., three practitioners from the omnichannel retailing industry, two professors whose research interests are retailing, service operations management, and consumer behavioral research, and one doctors) to ensure content validity. A total of 28 measurement items were adapted to operationalize the eight constructs (see Table 2). Specifically, three items were adopted to measure perceived severity (100, 101); a four-item scale was chosen from Bashirian et al. (80) to detect perceived vulnerability; four items measured perceived fear; while respondents' health anxiety was selected for measurement by three items (102, 103). Self-efficacy and response efficacy were measured using three and four items, respectively (69, 104–106). Three measures for behavioral intention were adopted from Chang and Chen (107). A seven-point Likert scale ranging from 1 = “strongly disagree” to 7 = “strongly agree” was used to evaluate these items.
A pretest was administered to 13 native English-speaking participants at the corresponding author's university in South Korea to check the appropriateness of the survey. It was reported that some of the questionnaire items should be improved for more simplicity. Additionally, it was suggested that section Introduction should contain more information to help respondents better comprehend omnichannel retailing, such as more examples regarding the usage scenario differences from other channels. Therefore, some revisions were made to the phrasing of the measures and the description sheet.
Data Collection
The questionnaire was administered to residents in Seoul, Korea. Seoul was selected because the lock-down policy was not implemented during the COVID-19 pandemic. Therefore, outdoor activities were not restricted. Previous evidence highlighted that compatibility and connectivity play a significant role in consumers' perceptions of omnichannel shopping (2, 108). Since lock-down may disrupt some components of the value chain (e.g., the operations of physical stores), it may influence the consumer's understanding of omnichannel retailing, which affects the data quality.
A translation and back-translation approach was used to ensure semantic equivalence and enable the questionnaire to be fully and easily understood by respondents. First, a professional translator was assigned to translate the original English version into Korean. Afterward, the Korean version of the questionnaire was back-translated by another translator who did not see the original version of the questionnaire. After completing the translation process, the authors and translators engaged in discussions to compare both the original and back-translation versions of the questionnaire to identify discrepancies and revise the Korean version of the questionnaire. Finally, the refined questionnaire was generated online on Google Forms, and a QR code generator was used to create a QR code that provides access to the questionnaire.
The survey was conducted simultaneously at five randomly selected shopping areas with high customer flow. They included Myeongdong, Dongdaemun, Hongdae, Apgujeong, and Yongsan. Customers who were willing to participate in the survey were invited to scan the QR code via their mobile devices. Note that respondents' email addresses were automatically collected to email them the coffee gift card. The survey was conducted for 2 weeks in February 2021, from 9:00 a.m. to 9:00 p.m. A total of 579 questionnaires were received. It was discovered that respondents generally took more than 5 min to answer all the questions during the pretest. As a result, respondents who were recorded as finishing the survey too quickly (taking <2 min) were regarded as invalid responses. In addition, responses with incorrect answers to the screening questions were also discarded. Ultimately, 398 questionnaires were effectively used for further analysis, representing a conversion rate of 69%.
Results and Discussions
Demographic Statistics
The respondents' demographic profiles, as well as the characteristics of their most frequently used payment methods are shown in Table 3. The proportion of males (52.01%) is marginally larger than females (49.99%). The majority of respondents are either young (60.80% were under 30) or middle-aged (37.19% are between 30 and 49). They also have a high level of education, with most respondents (71.61%) having received a bachelor's degree or above. Next, around 62.06% of respondents have a monthly income between 2.5 and 4.5 million KRW (~US$ 2,250–4,000). Regarding the payment method, the most frequently used by respondents in their daily lives are credit cards (80.65%), followed by mobile devices (11.56%), cash (7.29%), and others (0.5%).
Measurement Model Assessment
Confirmatory factor analysis is performed to evaluate the reliability and validity of the measurement model fit, and the results are presented in Tables 4, 5. According to Hu and Bentler (109), a good model fit is considered as fulfilling the following criteria: (1) the ratio of the Chi-square value to the degree of freedom (χ2/df ∈(1, 3)); (2) the Tucker-Lewis index (TLI) > 0.9; (3) the comparative fit index (CFI) > 0.9; (4) the root mean square error of approximation (RMSEA) < 0.08; and (5) the standardized root mean square residual (SRMR) < 0.08. The results of the study show that all the indices are within the cut-off range (χ2/df = 2.10, df = 320, CFI = 0.96, TLI = 0.95, RMSEA = 0.05, SRMR = 0.06). Therefore, the model's goodness of fit was supported.
In addition to the model fit, the measurement model is also evaluated for reliability, convergent validity, and discriminant validity. Regarding reliability, Hair et al. (110) proposed that the composite reliability (CR) of each construct should exceed 0.70, and standardized factor loadings (λ) should be higher than 0.50, ideally, 0.70. According to Table 3, the CRs of the constructs are all above 0.80. Therefore, the constructs of the measurement model are considered reliable. In addition, as displayed in Table 4, standardized factor loadings varied between 0.68 and 0.93, demonstrating that all measurement items are acceptable. For the convergent validity, scholars have suggested that the constructs' average variance extracted (AVE) should exceed 0.50 (111). Therefore, the convergent validity of the model is confirmed as the average variances extracted (AVEs) in Table 4, which are all above the threshold value.
Regarding discriminant validity, three conditions should be satisfied: (1) the inter-correlation values between constructs should be less than the square root of the AVE, (2) the construct's AVE value should be greater than its corresponding maximum shared variance (MSV), and (3) the average shared variance (ASV) of the construct should be smaller than its AVE (112, 113). As shown in Table 5, all the constructs have fulfilled the above conditions, suggesting confirmation of discriminant validity.
Structural Model Assessment
The structural equation model (SEM) is applied to evaluate the structural model. Note that control variables, including age, education, and income, are also added to the model to analyze their effects on consumers' behavioral intentions to use omnichannel retailing. Hypotheses are examined through the significance and the standardized estimated correlations of the constructs. In addition, squared multiple correlations (R2) are calculated to determine the percentage variance explained by the latent variables. The results are graphically depicted in Figure 2.
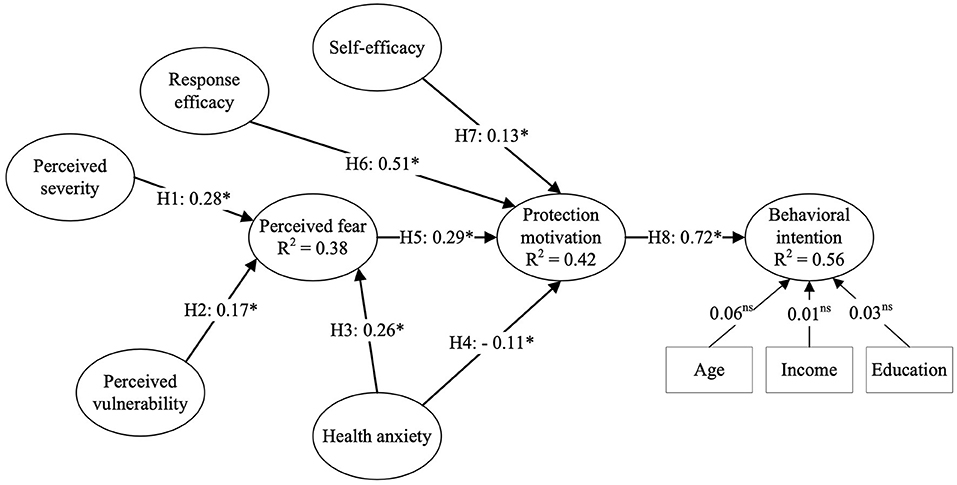
Figure 2. Results of structural model analysis. Model fit indices: χ2/df = 1.86, CFI = 0.95, TLI = 0.95, RMSEA = 0.05, SRMR = 0.07. *Indicates p < 0.05.
Figure 2 shows that the structural model fit was sufficient (χ2/df = 2.58, CFI = 0.95; TLI = 0.95; RMSEA = 0.06; SRMR = 0.07). Overall, all hypotheses were accepted, except H4, and the relationship between the control variables and consumers' behavioral intentions to use omnichannel shopping was not statistically significant.
Significant positive relationships are found between perceived severity and perceived fear (β = 0.28, p < 0.05); thus, H1 was accepted. This outcome is consistent with previous findings in COVID-19 research, which have reported that consumers who feel unsafe due to the pandemic can lead to a greater perceived fear of COVID-19 (114). Also, in line with previous studies (81), H2 is supported because the positive path from perceived vulnerability to perceived fear was significant (β = 0.17, p < 0.05). Therefore, consumers assess COVID-19 as a health crisis that makes them susceptible to infection, and this concern contributes to their perceived fear of COVID-19.
Moreover, while the predictor health anxiety significantly affects perceived fear and protection motivation (p < 0.05), the path coefficients for the two are 0.26 and −0.11, respectively, thus generating support for H3 but rejection for H4. The acceptance of H3 is expected since the E-EPPM posits that, because people who are health anxious are more sensitive and more afraid of uncertainties than those without health anxiety, they experience higher levels of perceived fear. Interestingly, as displayed in Figure 2, the path loading of health anxiety (β = 0.26) is lower than perceived severity (β = 0.28), implying that there is a consensus on the severity of the COVID-19 pandemic. These antecedents explain 38% of the variance in perceived fear (R2 = 0.38) collectively. However, the rejection of H4 is unexpected, which is inconsistent with previous findings (77, 92).
According to the rejection of H4 and the acceptance of H5 [perceived fear (β = 0.29, p < 0.05)], the indirect effect of health anxiety on consumers' protective motivation to use omnichannel retail services as a response to COVID-19 is found, highlighting the role of perceived fear in explaining consumers' protection motivation.
Furthermore, response efficacy (β = 0.51, p < 0.05) and self-efficacy (β = 0.28, p < 0.05) had significant positive effects on protection motivation, supporting H6 and H7. The positive relationship between response efficacy and protection motivation is aligned with PMT, suggesting that consumers will be more motivated to adopt omnichannel retail services if they assess themselves as capable of using omnichannel retailing. In addition, the positive association between response efficacy and protection motivation is also coherent with the PMT, which states that consumers who perceive the use of omnichannel retailing as being useful to protecting themselves from COVID-19 are likely to be more motivated to take this as protective actions. Together with perceived fear, these three constructs explain 42% of the variance in protection motivation (R2 = 0.42). Finally, the behavioral intention was found to be positively and significantly predicted by protection motivation, with a variance explanation of about 56% (R2 = 0.56). Thus, H8 was accepted.
Notably, no statistically significant linkage was found between the control variables and behavioral intention. This outcome contradicts previous studies. For example, it has been reported that the elderly tend to be more reluctant to new technology than younger people (115). Notwithstanding, this finding confirms that the theoretical constructs are stronger indicators of consumers' behavioral intentions to adopt omnichannel retail services than demographic variables.
Mediation Test
A bootstrap mediation analysis using a sample size of 5,000 for the bias-corrected confidence intervals was conducted (116) to examine the mediated relationships in the conceptual framework, and the results were presented in Table 6. Overall, the study's model does not contain a zero value in terms of the 95% confidence intervals, confirming the statistical significance of the mediating effects. Perceived fear is found to fully mediate the relationship between consumers' protection motivation and perceived severity (bind = 0.08, Boot SE = 0.02, p < 0.001) and perceived vulnerability (bind = 0.05, Boot SE = 0.02, p < 0.01). Because both the direct effect (p < 0.05) and indirect effect (p < 0.001) of health anxiety on protection motivation are statistically significant, perceived fear is a partial mediator of the relationship between health anxiety and protection motivation. Moreover, protection motivation is also confirmed as an effective mediator between consumers' behavioral intention to use omnichannel retailing and the constructs of the PMT and E-EPPM. Specifically, response efficacy has the largest indirect effect on behavioral intention (bind = 0.37, Boot SE = 0.05, p < 0.001), followed by perceived fear (bind = 0.21, Boot SE = 0.04, p < 0.001), self-efficacy (bind = 0.10, Boot SE = 0.06, p < 0.001), perceived severity (bind = 0.06, Boot SE = 0.02, p < 0.001), perceived vulnerability (bind = 0.04, Boot SE = 0.02, p < 0.01), and health anxiety (bind = −0.03, Boot SE = 0.03, p < 0.01), respectively. In this regard, the effects of perceived severity, perceived vulnerability, and health anxiety are channeled via dual mediators (i.e., perceived fear and protection motivation).
Conclusions
Theoretical Contributions
Theoretically, this study contributes to the existing literature in several ways. First, it enriches the literature on COVID-19 by empirically revealing the pandemic's psychological impact on consumers. The results indicate that the severity and vulnerability of COVID-19 and consumers' health anxiety are significantly associated with perceived fear while also demonstrating that, together with response efficacy and self-efficacy, these factors would trigger their protective motivations. Although the positive impact of health anxiety on protection motivation was not statistically validated (H4), the results highlighted the mediating role of perceived fear in explaining consumers' protection motivations.
Second, this study adds knowledge to the body of PMT and E-EPPM research by proposing and validating a research conceptualization that integrates PMT and E-EPPM to identify the antecedents that influence consumers' intentions to use omnichannel retailing. Specifically, the former considers the influence of coping behaviors, while the latter describes the effect of emotional appeals on omnichannel retailing adoption intentions. The results demonstrate that both PMT and E-EPPM are theoretical perspectives appropriate for this context and provide better insight into consumers' behaviors and decision-making processes concerning the selection of retailing services. Overall, the integrated model explains 56% of the variance in consumers' intentions to adopt omnichannel retail services, which is considered sizeable in the context of consumer behavior research.
Third, this study advances the current understanding regarding the determinants of consumers' intentions to use omnichannel retail services. Despite the critical role of consumer behavior in omnichannel retailing, the theoretical foundation related to the consumer perspective has been discovered to be rather underdeveloped (21). This study identifies that consumers' intentions to use omnichannel retailing can be trigged by their safety concerns; that is, perceived fears evoked by perceived severity, vulnerability, and health anxiety would drive them to use omnichannel retailing services as coping measures. Furthermore, factors such as self-efficacy and response efficacy are found to be attributed to consumers' intentions to use omnichannel retailing. By doing so, this study echoes Mishra et al.'s [(21), p.161] call for more research on the predominance of perceptual dimensions of consumers' decision-making in omnichannel retailing.
Managerial Implications
The managerial implications of this study are varied. First, this study confirms that using omnichannel retailing can be adopted as an effective response to COVID-19. Given that the COVID-19 pandemic has resulted in enormous shock to the retail and service industry (24), those service providers that currently only operate a single channel may consider introducing omnichannel retailing to provide a better shopping experience and service quality to attract customers (117).
Second, given the high COVID-19 infection and mortality rate, it is important that omnichannel retail service providers (ORSPs) take safety-related strategies that alleviate customers' perceived fears, as well as enhance response efficacy. For example, for the physical channels, ORSPs can place non-contact temperature assessment machines at the entrance to ensure that customers entering the store do not have fever symptoms and arrange for staff to supervise their use of sanitizers. At the same time, ORSPs also need to control the customer flow in time slots to ensure social distancing among customers. However, since such a restriction may lead to long queues, which may conversely exacerbate customers' fears, a possible solution to this problem may be to provide real-time reservation information on their digital channels. Following this, self-efficacy is the next area of improvement for omnichannel retail services. ORSPs can aim to reduce the complexity of the overall system so that consumers can better master the skills required. Also, supportive programs, such as mentorship and demonstrations, should be arranged to engage consumers to improve their confidence in using omnichannel retailing services.
Limitations and Recommendations
The present study has some limitations, which suggests avenues for future research. The first limitation is the scope of this study. Given that the sample used in this study was collected in Seoul, South Korea, it may prove challenging to directly apply the implications to other cultural or geographical settings. In addition, the majority of the survey respondents were rather young (only 2% were above 50 years old), which may cause the dataset to be questionable in terms of the representativeness of the sampled population. Therefore, further studies that endeavor to overcome the generalizability issues of this study are encouraged. For example, future research may conduct a cross-country study and employ a boosted sample from the older generations to validate our findings. Another limitation is that although this study identified several important factors regarding the determinants of consumers' intentions to use omnichannel retailing, the research model only considered two theoretical lenses: PTM and E-EPPM. Therefore, it is recommended that future studies apply other relevant theories, such as attitude theory (118), self-determination theory (119), trust theory (96), or the unified theory of acceptance, and use the Technology 2 Model (120) to study consumers' intentions to use omnichannel retail services.
Data Availability Statement
The raw data supporting the conclusions of this article will be made available by the authors, without undue reservation.
Ethics Statement
Ethical review and approval was not required for the study on human participants in accordance with the local legislation and institutional requirements. Written informed consent for participation was not required for this study in accordance with the national legislation and the institutional requirements.
Author Contributions
FL, MF, LC, MS, and XW have equally participated in conceptualization, literature review, data analysis, and writing of the paper. All authors contributed to the article and approved the submitted version.
Funding
This work was supported by the National Social Science Fund of China [No. 20BJY131].
Conflict of Interest
The authors declare that the research was conducted in the absence of any commercial or financial relationships that could be construed as a potential conflict of interest.
Publisher's Note
All claims expressed in this article are solely those of the authors and do not necessarily represent those of their affiliated organizations, or those of the publisher, the editors and the reviewers. Any product that may be evaluated in this article, or claim that may be made by its manufacturer, is not guaranteed or endorsed by the publisher.
References
1. Adivar B, Hüseyinoglu IÖY, Christopher M. A quantitative performance management framework for assessing omnichannel retail supply chains. J Retail Consum Serv. (2019) 48:257–69. doi: 10.1016/j.jretconser.2019.02.024
2. Cao L. Business model transformation in moving to a cross-channel retail strategy: a case study. Int J Electron Commer. (2014) 18:69–96. doi: 10.2753/JEC1086-4415180403
3. Gao F, Su X. Omnichannel service operations with online and offline self-order technologies. Manage Sci. (2018) 64:3595–608. doi: 10.1287/mnsc.2017.2787
4. Verhoef PC, Kannan PK, Inman JJ. From multichannel retailing to omni-channel retailing: introduction to the special issue on multichannel retailing. J Retail. (2015) 91:174–81. doi: 10.1016/j.jretai.2015.02.005
5. Xu X, Jackson JE. Examining customer channel selection intention in the omni-channel retail environment. Int J Prod Econ. (2019) 208:434–45. doi: 10.1016/j.ijpe.2018.12.009
6. Xu X, Jackson JE. Investigating the influential factors of return channel loyalty in omni-channel retailing. Int J Prod Econ. (2019) 216:118–32. doi: 10.1016/j.ijpe.2019.03.011
7. Zhang L, Wu L, Huang L, Zhang Y. Wield the power of omni-channel retailing strategy: a capability and supply chain resilience perspective. J Strat Market. (2021) 1–25. doi: 10.1080/0965254X.2021.1972440. [Epub ahead of print].
8. Gao W, Fan H, Li WQ, Wang HL. Crafting the customer experience in omnichannel contexts: the role of channel integration. J Bus Res. (2021) 126:12–22. doi: 10.1016/j.jbusres.2020.12.056
9. Cai L, Yuen KF, Xie D, Fang M, Wang X. Consumer's usage of logistics technologies: integration of habit into the unified theory of acceptance and use of technology. Technol Soc. (2021) 67:101789. doi: 10.1016/j.techsoc.2021.101789
10. Wang X, Wong YD, Liu F, Yuen KF. A push–pull–mooring view on technology-dependent shopping under social distancing: when technology needs meet health concerns. Technol Forecast Soc Change. (2021) 173:121109. doi: 10.1016/j.techfore.2021.121109
11. Wang X, Wong YD, Shi W, Yuen KF. Shoppers' logistics activities in omni-channel retailing: a conceptualisation and an exploration on perceptual differences in effort valuation. Transport Pol. (2021) 115:195–208. doi: 10.1016/j.tranpol.2021.11.014
12. Cao L, Li L. The impact of cross-channel integration on retailers' sales growth. J Retail. (2015) 91:198–216. doi: 10.1016/j.jretai.2014.12.005
13. Gao F, Su X. Online and offline information for omnichannel retailing. Manufact Serv Operat Manag. (2016) 19:84–98. doi: 10.2139/ssrn.2796566
14. Flavian C, Gurrea R, Orus C. Combining channels to make smart purchases: the role of webrooming and showrooming. J Retail Consum Serv. (2020) 52:101923. doi: 10.1016/j.jretconser.2019.101923
15. Lee ZW, Chan TK, Chong AY, Thadani DR. Customer engagement through omnichannel retailing: the effects of channel integration quality. Indust Market Manag. (2019) 77:90–101. doi: 10.1016/j.indmarman.2018.12.004
16. Lemon KN, Verhoef PC. Understanding customer experience throughout the customer journey. J Mark. (2016) 80:69–96. doi: 10.1509/jm.15.0420
17. Li Y, Liu H, Lim ETK, Goh JM, Yang F, Lee MKO. Customer's reaction to cross-channel integration in omnichannel retailing: the mediating roles of retailer uncertainty, identity attractiveness, switching costs. Decis Support Syst. (2018) 109:50–60. doi: 10.1016/j.dss.2017.12.010
18. Picot-Coupey K, Huré E, Piveteau L. Channel design to enrich customers' shopping experiences: synchronizing clicks with bricks in an omni-channel perspective-the Direct Optic case. Int J Retail Distrib Manag. (2016) 44:336–38. doi: 10.1108/IJRDM-04-2015-0056
19. Chen ZS, Peng JH. Should the assembly system with direct omnichannel introduce integrated management service? A game-theoretical modelling study. Electron Commerce Res. (2021) 44. doi: 10.1007/s10660-020-09450-z
20. Mandal P, Basu P, Saha K. Forays into omnichannel: an online retailer's strategies for managing product returns. Eur J Oper Res. (2021) 292:633–51. doi: 10.1016/j.ejor.2020.10.042
21. Mishra R, Singh RK, Koles B. Consumer decision-making in omnichannel retailing: literature review and future research agenda. Int J Consum Stud. (2021) 45:147–74. doi: 10.1111/ijcs.12617
22. Cao J, Liu F, Shang M, Zhou X. Toward street vending in post COVID-19 China: social networking services information overload and switching intention. Technol Soc. (2021) 66:101669. doi: 10.1016/j.techsoc.2021.101669
23. Chen X, Liu C, Liu F, Fang M. Firm sustainable growth during the COVID-19 pandemic: the role of customer concentration. Emerg Markets Finance Trade. (2021) 57:1566–577. doi: 10.1080/1540496X.2021.1904884
24. Goddard E. The impact of COVID-19 on food retail and food service in Canada: preliminary assessment. Can J Agric Econ. (2020) 68:143–9. doi: 10.1111/cjag.12243
25. Su M, Zhao J, Qi G, Kim J, Park KS. Online retailer cold chain physical distribution service quality and consumers: evidence from China during the COVID-19 pandemic. Int J Logist Res Appl. (2021). doi: 10.1080/13675567.2021.1961214. [Epub ahead of print].
26. Zhu YP, Park HW. Development of a COVID-19 web information transmission structure based on a Quadruple Helix Model: webometric network approach using Bing. J Med Int Res. (2021) 23:e27681. doi: 10.2196/27681
27. Li X, Zhou Y, Wong YD, Wang X, Yuen KF. What influences panic buying behaviour? A model based on dual-system theory and stimulus-organism-response framework. Int J Disast Risk Reduct. (2021) 64:102484. doi: 10.1016/j.ijdrr.2021.102484
28. Wang X, Wong YD, Yuen KF. Rise of ‘Lonely'Consumers in the post-COVID-19 era: a synthesised review on psychological, commercial and social implications. Int J Environ Res Public Health. (2021) 18:404. doi: 10.3390/ijerph180204040
29. Wang S, Chen Z, Xiao Y, Lin C. Consumer privacy protection with the growth of AI-empowered online shopping based on the evolutionary game model. Front Public Health. (2021) 9:5777. doi: 10.3389/fpubh.2021.705777
30. Yuen KF, Wang X, Ma F, Li KX. The psychological causes of panic buying following a health crisis. Int J Environ Res Public Health. (2020) 17:3513. doi: 10.3390/ijerph17103513
31. Jiang Y, Wang X, Yuen KF. Augmented reality shopping application usage: the influence of attitude, value, and characteristics of innovation. J Retail Consumer Serv. (2021) 63:102720. doi: 10.1016/j.jretconser.2021.102720
32. Pang Q, Meng H, Fang M, Xing J, Yao J. Social distancing, health concerns, and digitally empowered consumption behavior under COVID-19: a study on livestream shopping technology. Front Public Health. (2021) 9:48. doi: 10.3389/fpubh.2021.748048
33. Youn S-Y, Lee JE, Ha-Brookshire J. Fashion consumers' channel switching behavior during the COVID-19: protection motivation theory in the extended planned behavior framework. Clothing Text Res J. (2021) 39:139–56. doi: 10.1177/0887302X20986521
34. Rogers RW. A protection motivation theory of fear appeals and attitude change1. J Psychol. (1975) 91:93–114. doi: 10.1080/00223980.1975.9915803
35. So J. A further extension of the extended parallel process model (E-EPPM): implications of cognitive appraisal theory of emotion and dispositional coping style. Health Commun. (2013) 28:72–83. doi: 10.1080/10410236.2012.708633
36. McClendon BT, Prentice-Dunn S. Reducing skin cancer risk: an intervention based on protection motivation theory. J Health Psychol. (2001) 6:321–8. doi: 10.1177/135910530100600305
37. Pakpour AH, Griffiths MD. The fear of COVID-19 and its role in preventive behaviors. J Concurr Disord. (2020) 2:58–63. doi: 10.54127/WCIC8036
38. Zhao Y, Ni Q, Zhou R. What factors influence the mobile health service adoption? A meta-analysis and the moderating role of age. Int J Inf Manage. (2018) 43:342–50. doi: 10.1016/j.ijinfomgt.2017.08.006
39. Ilk N, Brusco M, Goes P. Workforce management in omnichannel service centers with heterogeneous channel response urgencies. Decis Support Syst. (2018) 105:13–23. doi: 10.1016/j.dss.2017.10.008
40. Yadav VS, Tripathi S, Singh AR. Bi-objective optimization for sustainable supply chain network design in omnichannel. J Manufact Technol Manag. (2019) 30:972–86. doi: 10.1108/JMTM-06-2017-0118
41. Juaneda-Ayensa E, Mosquera A, Sierra Murillo Y. Omnichannel customer behavior: key drivers of technology acceptance and use and their effects on purchase intention. Front Psychol. (2016) 7:1117. doi: 10.3389/fpsyg.2016.01117
42. Schoenbachler DD, Gordon GL. Multichannel shopping: understanding what drives channel choice. J Consum Market. (2002) 19:42–53. doi: 10.1108/07363760210414943
43. Swaminathan JM, Tayur SR. Models for supply chains in e-business. Manag Sci. (2003) 49:1387–406. doi: 10.1287/mnsc.49.10.1387.17309
44. Thaichon P, Surachartkumtonkun J, Quach S, Weaven S, Palmatier RW. Hybrid sales structures in the age of e-commerce. J Pers Selling Sales Manag. (2018) 38:277–302. doi: 10.1080/08853134.2018.1441718
45. Saghiri S, Wilding R, Mena C, Bourlakis M. Toward a three-dimensional framework for omni-channel. J Bus Res. (2017) 77:53–67. doi: 10.1016/j.jbusres.2017.03.025
46. Shen XL, Li YJ, Sun Y, Wang N. Channel integration quality, perceived fluency and omnichannel service usage: the moderating roles of internal and external usage experience. Decis Support Syst. (2018) 109:61–73. doi: 10.1016/j.dss.2018.01.006
47. Hossain TMT, Akter S, Kattiyapornpong U, Dwivedi Y. Reconceptualizing integration quality dynamics for omnichannel marketing. Ind Market Manag. (2020) 87:225–41. doi: 10.1016/j.indmarman.2019.12.006
48. Hajdas M, Radomska J, Silva SC. The omni-channel approach: a utopia for companies? J Retail Consumer Serv. (in press) 102131. doi: 10.1016/j.jretconser.2020.102131
49. Chopra S. How omni-channel can be the future of retailing. Decision. (2016) 43:135–44. doi: 10.1007/s40622-015-0118-9
50. Hübner AH, Kuhn H, Wollenburg J. Last mile fulfilment and distribution in omni-channel grocery retailing: a strategic planning framework. Int J Retail Distrib Manag. (2016) 44. doi: 10.1108/IJRDM-11-2014-0154
51. Hüseyinoglu IÖY, Sorkun MF, Börühan G. Revealing the impact of operational logistics service quality on omni-channel capability. Asia Pac J Market Logist. (2018) 30:1200–21. doi: 10.1108/APJML-08-2017-0169
52. Cao L, Li L. Determinants of retailers' cross-channel integration: an innovation diffusion perspective on omni-channel retailing. J Interact Market. (2018) 44:1–16. doi: 10.1016/j.intmar.2018.04.003
53. Luo J, Fan M, Zhang H. Information technology, cross-channel capabilities, and managerial actions: evidence from the apparel industry. J Assoc Inf Syst. (2015) 17:308–27. doi: 10.17705/1jais.00429
54. Song G, Song S, Sun L. Supply chain integration in omni-channel retailing: a logistics perspective. Int J Logist Manag. (2019) 30:527–48. doi: 10.1108/IJLM-12-2017-0349
55. Floyd DL, Prentice-Dunn S, Rogers RW. A meta-analysis of research on protection motivation theory. J Appl Soc Psychol. (2000) 30:407–29. doi: 10.1111/j.1559-1816.2000.tb02323.x
56. Rogers RW. Cognitive and Psychological Processes in Fear Appeals and Attitude Change: A Revised Theory of Protection Motivation. Social Psychophysiology: A Sourcebook. Boston, MA (1983). p. 153–76.
57. Ruan W, Kang S, Song H. Applying protection motivation theory to understand international tourists' behavioural intentions under the threat of air pollution: a case of Beijing, China. Curr Issues Tourism. (2020) 23:2027–41. doi: 10.1080/13683500.2020.1743242
58. Makarovs K, Achterberg P. Contextualizing educational differences in “vaccination uptake”: a thirty nation survey. Soc Sci Med. (2017) 188:1–10. doi: 10.1016/j.socscimed.2017.06.039
59. Zare Sakhvidi MJ, Zare M, Mostaghaci M, Mehrparvar AH, Morowatisharifabad MA, Naghshineh E. Psychosocial predictors for cancer prevention behaviors in workplace using protection motivation theory. Adv Prev Med. (2015) 2015:467498. doi: 10.1155/2015/467498
60. Bubeck P, Botzen WJW, Kreibich H, Aerts JCJH. Detailed insights into the influence of flood-coping appraisals on mitigation behaviour. Global Environ Change. (2013) 23:1327–38. doi: 10.1016/j.gloenvcha.2013.05.009
61. Chen M-F. Moral extension of the protection motivation theory model to predict climate change mitigation behavioral intentions in Taiwan. Environ Sci Pollut Res. (2020) 27:13714–25. doi: 10.1007/s11356-020-07963-6
62. Park SE, Marshall NA, Jakku E, Dowd AM, Howden SM, Mendham E, et al. Informing adaptation responses to climate change through theories of transformation. Global Environ Change. (2012) 22:115–26. doi: 10.1016/j.gloenvcha.2011.10.003
63. Giwah AD, Wang L, Levy Y, Hur I. Empirical assessment of mobile device users' information security behavior towards data breach. J Intellectual Capital. (2020) 21:215–33. doi: 10.1108/JIC-03-2019-0063
64. Wang S-T. The effects of risk appraisal and coping appraisal on the adoption intention of m-payment. Int J Bank Market. (2019) 38:21–33. doi: 10.1108/IJBM-10-2018-0272
65. Wottrich VM, van Reijmersdal EA, Smit EG. App users unwittingly in the spotlight: a model of privacy protection in mobile apps. J Consum Affairs. (2019) 53:1056–83. doi: 10.1111/joca.12218
66. Bockarjova M, Steg L. Can protection motivation theory predict pro-environmental behavior? Explaining the adoption of electric vehicles in the Netherlands. Global Environ Change. (2014) 28:276–88. doi: 10.1016/j.gloenvcha.2014.06.010
67. Langbroek JHM, Franklin JP, Susilo YO. The effect of policy incentives on electric vehicle adoption. Energy Policy. (2016) 94:94–103. doi: 10.1016/j.enpol.2016.03.050
68. Dong F, Liu Y. Policy evolution and effect evaluation of new-energy vehicle industry in China. Resour Policy. (2020) 67:101655. doi: 10.1016/j.resourpol.2020.101655
69. Zheng D, Luo Q, Ritchie BW. Afraid to travel after COVID-19? Self-protection, coping and resilience against pandemic ‘travel fear'. Tourism Manag. (2021) 83:104261. doi: 10.1016/j.tourman.2020.104261
70. Adunlin G, Adedoyin ACA, Adedoyin OO, Njoku A, Bolade-Ogunfodun Y, Bolaji B. Using the protection motivation theory to examine the effects of fear arousal on the practice of social distancing during the COVID-19 outbreak in rural areas. J Hum Behav Soc Environ. (2021) 31:168–72. doi: 10.1080/10911359.2020.1783419
71. Okuhara T, Okada H, Kiuchi T. Examining persuasive message type to encourage staying at home during the COVID-19 pandemic and social lock-down: a randomized controlled study in Japan. Patient Educ Couns. (2020) 103:2588–93. doi: 10.1016/j.pec.2020.08.016
72. Alsaad A, Al-Okaily M. Acceptance of protection technology in a time of fear: the case of COVID-19 exposure detection apps. Inf Technol People. (2021). doi: 10.1108/ITP-10-2020-0719. [Epub ahead of print].
73. Hüseyinoglu IÖY, Galipoglu E, Kotzab H. Social, local and mobile commerce practices in omni-channel retailing: insights from Germany and Turkey. Int J Retail Distribut Manag. (2017) 45:711–29. doi: 10.1108/IJRDM-09-2016-0151
74. Witte K. Putting the fear back into fear appeals: the extended parallel process model. Commun Monogr. (1992) 59:329–49. doi: 10.1080/03637759209376276
75. Lazarus RS, Lazarus RS. Emotion and Adaptation. New York, NY: Oxford University Press on Demand (1991).
76. Witte K. Introduction: pathways. Health Commun. (2013) 28:3–4. doi: 10.1080/10410236.2013.743783
77. So J, Kuang K, Cho H. Reexamining fear appeal models from cognitive appraisal theory and functional emotion theory perspectives. Commun Monogr. (2016) 83:120–44. doi: 10.1080/03637751.2015.1044257
78. Ahorsu DK, Lin CY, Imani V, Saffari M, Griffiths MD, Pakpour AH. The fear of COVID-19 scale: development and initial validation. Int J Mental Health Addict. (2020) doi: 10.1007/s11469-020-00270-8. [Epub ahead of print].
79. Asmundson GJ, Taylor S. How health anxiety influences responses to viral outbreaks like COVID-19: what all decision-makers, health authorities, and health care professionals need to know. J Anxiety Disord. (2020) 71:102211. doi: 10.1016/j.janxdis.2020.102211
80. Bashirian S, Jenabi E, Khazaei S, Barati M, Karimi-Shahanjarini A, Zareian S, et al. Factors associated with preventive behaviours of COVID-19 among hospital staff in Iran in 2020: an application of the protection motivation theory. J Hosp Infect. (2020) 105:430–3. doi: 10.1016/j.jhin.2020.04.035
81. Yildirim M, Geçer E, Akgül Ö. The impacts of vulnerability, perceived risk, and fear on preventive behaviours against COVID-19. Psychol Health Med. (2021) 26:35–43. doi: 10.1080/13548506.2020.1776891
82. Mamun MA, Griffiths MD. First COVID-19 suicide case in Bangladesh due to fear of COVID-19 and xenophobia: possible suicide prevention strategies. Asian J Psychiatr. (2020) 51:102073. doi: 10.1016/j.ajp.2020.102073
83. Pakpour AH, Griffiths MD, Lin C-Y. Assessing psychological response to the COVID-19: the fear of COVID-19 scale and the COVID stress scales. Int J Mental Health Addict. (2020) 19:2407–10. doi: 10.1007/s11469-020-00334-9
84. Marroquín B, Vine V, Morgan R. Mental health during the COVID-19 pandemic: effects of stay-at-home policies, social distancing behavior, social resources. Psychiatry Res. (2020) 293:113419. doi: 10.1016/j.psychres.2020.113419
85. World Health Organization. Coronavirus (COVID-19) Dashboard. (2021). Available online at: https://covid19.who.int/
86. De Coninck D, d'Haenens L, Matthijs K. Perceived vulnerability to disease and attitudes towards public health measures: COVID-19 in Flanders, Belgium. Pers Individ Dif. (2020) 166:110220. doi: 10.1016/j.paid.2020.110220
87. Perrotta D, Grow A, Rampazzo F, Cimentada J, Del Fava E, Gil-Clavel S, et al. Behaviours and attitudes in response to the COVID-19 pandemic: insights from a cross-national Facebook survey. EPJ Data Sci. (2021) 10:17. doi: 10.1140/epjds/s13688-021-00270-1
88. Trougakos JP, Chawla N, McCarthy JM. Working in a pandemic: exploring the impact of COVID-19 health anxiety on work, family, health outcomes. J Appl Psychol. (2020) 105:1234. doi: 10.1037/apl0000739
89. Wheaton MG, Abramowitz JS, Berman NC, Fabricant LE, Olatunji BO. Psychological predictors of anxiety in response to the H1N1 (swine flu) pandemic. Cogn Therapy Res. (2012) 36:210–8. doi: 10.1007/s10608-011-9353-3
90. Blakey SM, Abramowitz JS. Psychological predictors of health anxiety in response to the Zika virus. J Clin Psychol Med Settings. (2017) 24:270–8. doi: 10.1007/s10880-017-9514-y
91. Mertens G, Gerritsen L, Duijndam S, Salemink E, Engelhard IM. Fear of the coronavirus (COVID-19): predictors in an online study conducted in March 2020. J Anxiety Disord. (2020) 74:102258. doi: 10.1016/j.janxdis.2020.102258
92. Knowles KA, Olatunji BO. Anxiety and safety behavior usage during the COVID-19 pandemic: the prospective role of contamination fear. J Anxiety Disord. (2021) 77:102323. doi: 10.1016/j.janxdis.2020.102323
93. Addo PC, Jiaming F, Kulbo NB, Liangqiang L. COVID-19: fear appeal favoring purchase behavior towards personal protective equipment. Serv Ind J. (2020) 40:471–90. doi: 10.1080/02642069.2020.1751823
94. Boss SR, Galletta DF, Lowry PB, Moody GD, Polak P. What do systems users have to fear? Using fear appeals to engender threats and fear that motivate protective security behaviors. MIS Q. (2015) 39:837864. doi: 10.25300/MISQ/2015/39.4.5
95. Reuken PA, Rauchfuss F, Albers S, Settmacher U, Trautwein C, Bruns T, et al. Between fear and courage: attitudes, beliefs, and behavior of liver transplantation recipients and waiting list candidates during the COVID-19 pandemic. Am J Transplant. (2020) 20:3042–50. doi: 10.1111/ajt.16118
96. Yuen KF, Ma F, Wang X, Lee G. The role of trust in influencing consumers' adoption of automated vehicles: an application of the health belief model. Int J Sustain Transport. (2020) 15:837–49. doi: 10.1080/15568318.2020.1821416
97. World Health Organization. Coronavirus Disease (COVID-19) Advice for the Public. (2021). Available online at: https://www.who.int/emergencies/diseases/novel-coronavirus-2019/advice-for-public
98. Bulgurcu B, Cavusoglu H, Benbasat I. Information security policy compliance: an empirical study of rationality-based beliefs and information security awareness. Mis Q. (2010) 34:523–48. doi: 10.2307/25750690
99. Ifinedo P. Understanding information systems security policy compliance: an integration of the theory of planned behavior and the protection motivation theory. Comp Sec. (2012) 31:83–95. doi: 10.1016/j.cose.2011.10.007
100. Chu H, Liu S. Integrating health behavior theories to predict American's intention to receive a COVID-19 vaccine. Pat Educ Counsel. (2021) 104:1878–86. doi: 10.1016/j.pec.2021.02.031
101. Tanoue Y, Nomura S, Yoneoka D, Kawashima T, Eguchi A, Shi S, et al. Mental health of family, friends, and co-workers of COVID-19 patients in Japan. Psychiatry Res. (2020) 291:113067. doi: 10.1016/j.psychres.2020.113067
102. Rather RA. Demystifying the effects of perceived risk and fear on customer engagement, co-creation and revisit intention during COVID-19: a protection motivation theory approach. J Destination Market Manag. (2021) 20:100564. doi: 10.1016/j.jdmm.2021.100564
103. Salkovskis PM, Rimes KA, Warwick HMC, Clark DM. The health anxiety inventory: development and validation of scales for the measurement of health anxiety and hypochondriasis. Psychol Med. (2002) 32:843–53. doi: 10.1017/S0033291702005822
104. Ling M, Kothe EJ, Mullan BA. Predicting intention to receive a seasonal influenza vaccination using protection motivation theory. Soc Sci Med. (2019) 233:87–92. doi: 10.1016/j.socscimed.2019.06.002
105. Farooq A, Laato S, Islam AN. Impact of online information on self-isolation intention during the COVID-19 pandemic: cross-sectional study. J Med Internet Res. (2020) 22:e19128. doi: 10.2196/19128
106. Menard P, Bott GJ, Crossler RE. User motivations in protecting information security: protection motivation theory versus self-determination theory. J Manag Inf Syst. (2017) 34:1203–30. doi: 10.1080/07421222.2017.1394083
107. Chang HH, Chen SW. The impact of online store environment cues on purchase intention: trust and perceived risk as a mediator. Online Inf Rev. (2008) 32:818–41. doi: 10.1108/14684520810923953
108. Shi S, Wang Y, Chen X, Zhang Q. Conceptualization of omnichannel customer experience and its impact on shopping intention: a mixed-method approach. Int J Inf Manag. (2020) 50:325–36. doi: 10.1016/j.ijinfomgt.2019.09.001
109. Hu LT, Bentler PM. Cutoff criteria for fit indexes in covariance structure analysis: conventional criteria versus new alternatives. Struct Eq Model Multidiscip J. (1999) 6:1–55. doi: 10.1080/10705519909540118
110. Hair J, Anderson R, Babin B, Black W. Multivariate Data Analysis: A Global Perspective. 7th ed. Upper Saddle River, NJ: Pearson Education International (2010).
111. Yuen KF, Wang X, Wong YD, Zhou Q. Antecedents and outcomes of sustainable shipping practices: the integration of stakeholder and behavioural theories. Transport Res Part E Logist Transport Rev. (2017) 108:18–35. doi: 10.1016/j.tre.2017.10.002
112. Sarstedt M, Ringle CM, Hair JF. Partial least squares structural equation modeling. Handb Market Res. (2017) 26:1–40. doi: 10.1007/978-3-319-05542-8_15-1
113. Tran TMT, Yuen KF, Wang X, Li KX. The antecedents of sustainable shipping management and organisational performance: resource accumulation and orientation perspectives. Int J Phys Distribut Logist Manag. (2020) 50:833–54. doi: 10.1108/IJPDLM-03-2020-0066
114. Sakib N, Akter T, Zohra F, Bhuiyan AI, Mamun MA, Griffiths MD. Fear of COVID-19 and depression: a comparative study among the general population and healthcare professionals during the COVID-19 pandemic crisis in Bangladesh. Int J Mental Health Addict. (2021). doi: 10.1007/s11469-020-00477-9. [Epub ahead of print].
115. Hudson J, Orviska M, Hunady J. People's attitudes to autonomous vehicles. Transport Res Part A Pol Pract. (2019) 121:164–76. doi: 10.1016/j.tra.2018.08.018
116. Hayes AF. Introduction to Mediation, Moderation, and Conditional Process Analysis: A Regression-Based Approach. New York, NY: Guilford Publications (2017).
117. Sayyida S, Hartini S, Gunawan S, Husin SN. The impact of the Covid-19 Pandemic on retail consumer behavior. Aptisi Transact Manag. (2021) 5:79–88. doi: 10.33050/atm.v5i1.1497
118. Wang X, Yuen KF, Wong YD, Teo C.-C. E-consumer adoption of innovative last-mile logistics services: a comparison of behavioural models. Total Quality Manag Bus Excellence. (2020) 31:1381–407. doi: 10.1080/14783363.2018.1485484
119. Guo Z, Zhou K, Zhang C, Lu X, Chen W, Yang S. Residential electricity consumption behavior: influencing factors, related theories and intervention strategies. Renew Sustain Energy Rev. (2018) 81:399–412. doi: 10.1016/j.rser.2017.07.046
Keywords: COVID-19, omnichannel, protection motivation theory, adoption behavior, health anxiety, retail services
Citation: Liu F, Fang M, Cai L, Su M and Wang X (2022) Consumer Motivations for Adopting Omnichannel Retailing: A Safety-Driven Perspective in the Context of COVID-19. Front. Public Health 9:708199. doi: 10.3389/fpubh.2021.708199
Received: 11 May 2021; Accepted: 20 December 2021;
Published: 13 January 2022.
Edited by:
Muhammad Salman Shabbir, Dhofar University, OmanReviewed by:
Yi Xiao, Zhejiang University, ChinaElisa Martinelli, University of Modena and Reggio Emilia, Italy
Yu Peng Zhu, Yeungnam University, South Korea
Copyright © 2022 Liu, Fang, Cai, Su and Wang. This is an open-access article distributed under the terms of the Creative Commons Attribution License (CC BY). The use, distribution or reproduction in other forums is permitted, provided the original author(s) and the copyright owner(s) are credited and that the original publication in this journal is cited, in accordance with accepted academic practice. No use, distribution or reproduction is permitted which does not comply with these terms.
*Correspondence: Mingjie Fang, bWpfZmFuZ0Brb3JlYS5hYy5rcg==