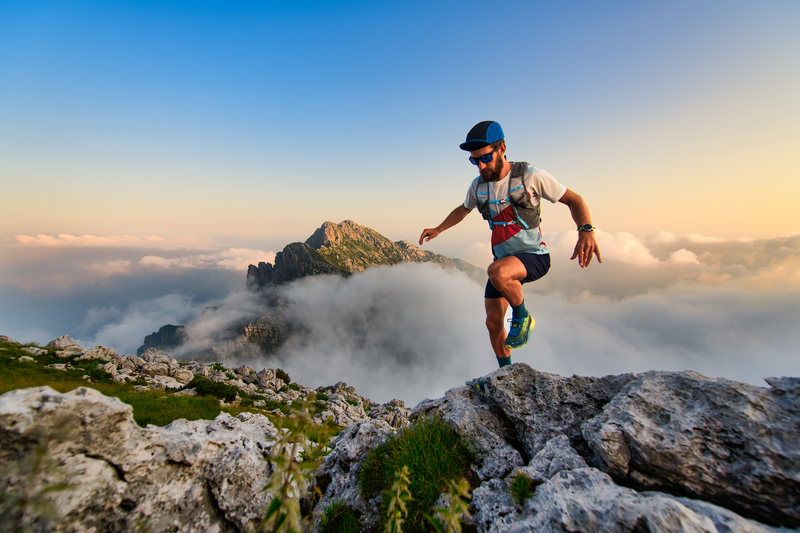
94% of researchers rate our articles as excellent or good
Learn more about the work of our research integrity team to safeguard the quality of each article we publish.
Find out more
ORIGINAL RESEARCH article
Front. Public Health , 03 May 2021
Sec. Health Economics
Volume 9 - 2021 | https://doi.org/10.3389/fpubh.2021.658706
This article is part of the Research Topic Age-related Diseases Through the Lens of Health Economics View all 13 articles
Objective: Metabolic diseases have been a clinical challenge worldwide and a major public health issue. Very few studies from China investigated the impact of metabolic multimorbidity on healthcare and health outcomes at the national level. This study aims to examine the association of metabolic multimorbidity with health service utilization, spending, functional and mental health.
Materials and Methods: This is a nationally representative cross-sectional study, utilizing the data from the China Health and Retirement Longitudinal Study in 2015, including 11,377 participants aged 45 years and older. Multivariable regression models were used to assess the association of metabolic multimorbidity with healthcare, out-of-pocket expenditure (OOPE), the activities of daily living (ADL) limitation, the instrumental activities of daily living (IADL) limitation, and depression.
Results: Overall, 30.50% of total participants had metabolic multimorbidity in 2015 in China. Compared with single disease, metabolic multimorbidity were associated with the number of outpatient visits [incident rate ratio (IRR) = 1.30, 95% CI = 1.05, 1.62] and days of inpatient care (IRR = 1.52, 95% CI = 1.28, 1.81). Metabolic multimorbidity was positively associated with the OOPE on outpatient care (coefficient = 82.99, 95% CI = 17.70, 148.27) and physical functional difficulties, including ADL limitation (odds ratio = 1.36, 95% CI = 1.18, 1.57).
Conclusions: Metabolic multimorbidity is associated with higher levels of health-care service use, greater expenditure for outpatient care, and more difficulties in ADL among Chinese adults. China's health-care systems need to shift from single-disease models to new financing and service delivery models to effectively manage metabolic multimorbidity.
Metabolic diseases have been a major public health issue and a clinical challenge worldwide, which is linked with the increased risk of cardiovascular diseases (1, 2) and all-cause mortality (3, 4). The prevalence of metabolic multimorbidity (defined as presence of two or more chronic conditions including hypertension, dyslipidaemia, diabetes, hyperuricemia and central obesity) is increasing rapidly. Recently, the Emerging Risk Factor Collaboration of 91 cohort studies showed that a particular form of cardiometabolic multimorbidity, was associated with a risk of death substantially greater than that for each of these diseases on their own. For example, at age 60 years, people with one cardiometabolic disease had a life expectancy 6–10 years shorter than those with no such disease, whereas people with cardiometabolic multimorbidity had a life expectancy shorter by up to 15 years (5). Over the past decade, a rapid increase was found in the number of individuals suffering from metabolic syndrome multimorbidity in China and low-and middle income countries (LMICs) (6–8).
Multimorbidity is associated with higher healthcare utilization, worse health status and depression in European countries, challenging the single-disease framework by which most of healthcare is configured (9). While there have been many studies conducted in high-income countries (HICs) on the impacts of chronic disease multimorbidity (10–12), this topic is still an emerging area of research inquiry in LMICs. Currently, only a couple of small studies in certain parts of China have examined this issue (13, 14), such as a study focused on Guangdong which looked at the health service utilization arising from multimorbidity of 162,464 subjects (15). Emerging evidence exists about the impact of single chronic condition alone. However, evidence is sparse about the economic and financial impact among people who have two or more metabolic conditions concurrently. No current study from China has estimated the impact of metabolic multimorbidity on functional limitation and mental health at the national level (16, 17). This study aims to systemically examine the association of metabolic multimorbidity with healthcare utilization, out-of-pocket expenditure (OOPE), functional health and depression, using nationally representative population-based data.
This is a nationally representative cross-sectional study, using the newest round of data from the China Health and Retirement Longitudinal Study (CHARLS) conducted in 2015. CHARLS is a biennial survey conducted by the National School of Development at Peking University, which aimed to be representative of Chinese residents aged 45 years and older. The data was collected in a survey in which four-stage, stratified, cluster sampling was used to select eligible individuals (18). Brie?y, 150 counties were selected, proportional to population size. Then three villages/communities were selected from each county as primary sampling units (PSUs). In each of the 450 PSUs, 80 households were randomly selected. In each household, persons aged 45 years and over, as well as their spouses, were interviewed using structured questionnaires. The main questionnaire includes information on basic demographics, health status and functioning, healthcare and insurance, work, retirement and pensions, income and consumption, household assets, and several biomarkers. Written informed consent was obtained from all participants. CHARLS received ethics approval from the Peking University Biomedical Ethics Review Committee (Ref. no. IRB00001052-11015) in 2011 (18).
The total sample size of the CHARLS baseline survey was 17,708 individual respondents. Ongoing follow-up surveys were conducted once every 2 years. For this study, we identified 13,420 respondents with blood test and biomarker information. After removing respondents aged below 45 years and those individuals with missing values of dependent or independent variables, our final sample consisted of 11,377 respondents accounting for 84.8% of those without loss-to-follow-up.
In this study, we counted the number of chronic diseases for each participant, identifying those with multimorbidity (19, 20). Hypertension was defined as systolic blood pressure ≥140 mmHg and/or diastolic blood pressure ≥90 mmHg, and/or being on antihypertensive medication for raised blood pressure (21). Diabetes was defined by (1) a fasting plasma glucose level of ≥126 mg/dL (7.0 mmol/L); and/or (2) HbA1c concentration of ≥6.5%; and/or (3) being insulin treatment and/or taking medication for raised blood sugar (22). Dyslipidaemia was defined by (1) total cholesterol (TC) ≥ 240 mg/dL (6.22 mmol/L); and/or (2) low-density lipoprotein cholesterol (LDL-C) ≥ 160 mg/dL (4.14 mmol/L); and/or (3) high-density lipoprotein cholesterol (HDL-C) <40 mg/dL (1.04 mmol/L); and/or (4) triglyceride (TG) ≥ 200 mg/dL (2.26 mmol/L); and/or (5) taking anti-dyslipidaemia medication (23). Hyperuricemia was defined as a blood uric acid concentration > 7.0 mg/dL for men and 6.0 mg/dL for women (24). Central obesity was defined as a waist circumference > 90 cm for men and 85 cm for women in participants with Body mass index (BMI) ≥ 30 kg/m2 (25).
Respondents were asked about their utilization of healthcare services, including the frequency of outpatient visits and days of inpatient care: “How many times did you visit a general hospital, specialized hospital, clinic or other medical facilities for outpatient care in the past month? and How many days did you stay in hospital over the past year?” CHARLS also collected the information on how much respondents paid in total and how much out-of-pocket (deducting the reimbursed expenses) for their outpatient visits during the last month and inpatient care during the last year.
Functional health was assessed by activities of daily living (ADL) limitation and instrument activities of daily living (IADL) limitation (26). The ADL includes six activities: bathing, dressing, feeding oneself, using the toilet, getting in or out of bed, and controlling urination and defecation. Answers were categorized as: “can do it by myself,” “have some difficulties,” “need help,” and “cannot do it.” The IADL refers to difficulty in doing household chores, cooking, shopping, making telephone calls, taking medications, and managing finances. Binary variables of ADL/IADL were constructed, and ADL/IADL disability was defined as having difficulty in one or more ADL/IADL items. This binary coding of ADL/IADL variables was also used as dependent variables in the multivariate regression analysis.
In terms of mental health, depression was assessed by the 10-item Center for Epidemiologic Studies Depression Scale (CES-D 10) (27), which has been identified as a valid, reliable, and useful mental health assessment tool for those aged 60 and above in China (28). The answers of the CES-D 10 include 4 options: (1) rarely, (2) some days (1–2 days per week), (3) occasionally (3–4 days per week), (4) most of the time (5–7 days per week). The participants' answers were recorded as 0 (rarely) to 3 (most of the time) for the negative questions in this study. For two positive questions, the items were reversed as 3 (rarely) to 0 (most of the time). The total scores of the CESD-10 range from 0 to 30. In this study, a binary variable of mental health was also constructed by defining an individual whose CESD-10 score was < 10 as having depression symptoms.
The negative binomial regression models were applied to investigate the association of multimorbidity with the frequency of outpatient visits and inpatient care. Covariates included age, gender, marital status (married and partnered, unmarried and others), education (illiterate, primary school and below, secondary school, college and above), residence place (rural, urban), geographical region (east, central and west), economic status quartiles (yearly per capita household consumption expenditure), and health insurance status (yes, no). Linear regression models were used to examine the relationships between multimorbidity and outpatient and inpatient care OOPE. Multivariable logistic regression models were used to estimate the association of multimorbidity with functional limitation and depression. We also performed sensitivity analyses for the impacts of multimorbidity on health service by using Poisson regression models and for the impacts on OOPE by using generalized linear models with a logarithm transfer.
For the negative binomial regression analysis, the incident rate ratio (IRR) were reported with results of 95% confidence intervals (CI) included in the Appendix. For linear regression models, we reported the coefficient (β) and 95% CI. For the logistic regression analysis, the adjusted odds ratio (AOR) and 95% CI were reported. Descriptive analysis of prevalence of multimorbidity and regression analysis were weighted to account for the multi-stage PPS design of CHARLS. All statistical analyses were conducted using STATA 15.0. P < 0.05 were considered as statistically significant.
Our analysis included data from 11,377 participants. The mean age of respondents was 60.29 years in 2015. Among the participants, 52.62% were women, 43.10% of the participants were illiterate, 63.18% were residing in rural areas and 82.23% were enrolled in social health insurance schemes. The prevalence of metabolic multimorbidity was 32.47% and increased with age, ranging from 24.61% in those aged 45–54 years, and 41.62% for those aged ≥ 75 years. People living in urban areas and those covered by social health insurance were suffering from a higher percentage of multimorbidity in China, compared with rural residents and individuals without health insurance (Table 1).
Table 1. Characteristics of participants, the prevalence of single condition, and metabolic multimorbidity.
Table 2 showed the prevalence of main metabolic diseases and multimorbidity across gender and age group. Among the middle-aged and older population, more than a third of Chinese adults suffered from hypertension and hyperlipidaemia in 2015. The prevalence of diabetes, hyperuricemia and concentric obesity was 19.75, 13.70, and 4.92%, respectively. The prevalence of hypertension and metabolic multimorbidity increased with age among both males and females, but concentric obesity decreased with age in female populations. For diabetes and hyperuricemia, the senior older group (aged ≥ 75 years) suffered from the highest prevalence.
Table 2. The proportion of metabolic diseases and multimorbidity among Chinese adults by gender and age group.
Table 3 indicated that multimorbidity was positively associated with health service use. Compared with people with single metabolic disease, patients with multimorbidity were likely to report more frequent outpatient visits (IRR = 1.30) and days of inpatient care (IRR = 1.52). The days of inpatient care increased substantially with age. Female patients used both outpatient and inpatient healthcare service more frequently than male patients. Individuals with a higher economic level and those patients in economically underdeveloped regions had more days of hospitalization compared with their counterparts.
Table 3 also showed that the prevalence of metabolic multimorbidity had a positive relationship with healthcare expenditure. The out-of-pocket spending on outpatient care was higher for patients with multimorbidity than those with a single disorder (β = 82.99). OOPE on outpatient care was significantly higher among female patients and those living in rural areas than spending among the male and urban citizens (Figure 1). The affluent population was likely to spend more out-of-pocket money on outpatient care (β = 128.44) and hospitalization care (β = 1,218.2) than those in a lower economic level. There was no statistically significant association between multimorbidity and OOPE for inpatient care. People enrolled in health insurance were likely to spend more on hospitalization care (β = 526.56) than those individuals without health insurance.
Figure 1. Frequency of healthcare, health expenditure, and functional limitation by the number of disease and residence place.
Table 4 showed that multimorbidity was associated with a higher likelihood of having functional difficulties. Compared with people with single metabolic disease, patients with multimorbidity were more likely to have an ADL limitation (AOR = 1.36). Older patients, females, those people with lower education status, and living in rural areas and undeveloped regions were reported higher levels of ADL limitation, IADL limitation and depression than their counterparts. No statistically significant association was found between multimorbidity and depression.
In terms of sensitivity analyses, we found similar associations between metabolic multimorbidity and frequency of outpatient and inpatient care as well as OOPE (Supplementary Tables 1, 2). The results were consistent with our original findings showing metabolic multimorbidity was associated with an increase in the number of outpatient visits (IRR = 1.30) and days of hospitalization (IRR = 1.52). Similarly, the days of hospitalization increased substantially with age. Female patients used both the outpatient and inpatient care service more frequently than male patients. People with a higher economic level and those patients in economically underdeveloped regions had more days in hospital compared with their counterparts.
This study used nationally representative data to assess the prevalence and impact of metabolic multimorbidity among middle-aged and elderly Chinese adults. It was found that metabolic multimorbidity was common, especially among elderly participants and those living in urban areas. We identified that metabolic multimorbidity was positively associated a greater use of both outpatient and inpatient care utilization, as well as greater health expenditure for outpatient care. Moreover, we found that metabolic multimorbidity was positively associated with a higher likelihood of having functional difficulties (ADL limitation). However, we did not identify a positive association between metabolic multimorbidity and depression.
This study showed that 30.5% of persons aged ≥45 years have metabolic multimorbidity in China. Based on previous studies, the overall prevalence of metabolic syndrome was 16.5% in 2000 (29) and 23.3% in 2009 (7) among the Chinese adults. A meta-analysis study in 2016 revealed that the pooled estimate of metabolic syndrome prevalence was 24.5% among subjects in Mainland China (30). It is indicated that China is experiencing an emerging epidemic of metabolic syndrome, which might be related to accelerating changes in lifestyle and nutrition caused by rapid economic development and urbanization (31). The increasing prevalence of metabolic multimorbidity in developing countries may eventually become similar to that in developed countries. Several studies have reported a high prevalence of metabolic syndrome in United States (35%), Turkey (44%), and Iran (37%) (32–34). Variations in the prevalence of metabolic syndrome from different studies might be either due to real disparities in different countries or regions, or because of potential differences in the definitions, methods of data collection, and sampling of study populations (34).
Our results indicated that metabolic multimorbidity was positively associated with older age, urban area, and health insurance. Consistent with other studies (35, 36), the prevalence of metabolic multimorbidity increased with age, which can be attributed to the declining metabolic function during the aging process. Additionally, individuals living in urban areas are more likely to suffer from metabolic multimorbidity than those living in rural areas, which is in line with previous findings (30, 37). This may be attributable to the impact of urbanization, including unhealthy dietary patterns, decreased physical activity, uptake of a high caloric diet, excessive intake of fat and salt, all of which lead to the development of metabolic conditions (37–39). Notably, this study provides new evidence on the association between social health insurance and metabolic multimorbidity in China. People covered by social health insurance were more likely to suffer from metabolic multimorbidity, compared with individuals without health insurance.
Previous studies have demonstrated that multimorbidity is significantly associated with greater healthcare utilization and higher level of health expenditure, which has been well-documented in developed countries (40, 41). Similarly, our study revealed that individuals with metabolic multimorbidity were more likely to have more frequent outpatient visits and more days of hospitalization, as well as to spend more out-of-pocket money on outpatient care. However, we found there was no significant association between metabolic multimorbidity and OOPE for inpatient care. The reason may be that individuals with metabolic multimorbidity have fewer complications than patients with other patterns of multimorbidity (e.g., cardiovascular diseases, musculoskeletal diseases, and respiratory diseases), so they require fewer health services and spend less money on inpatient care at the early stage of disease. Therefore, prioritizing health and medical resource allocation will be needed to prevent and control metabolic multimorbidity and the complications in China (37). Social health insurance should play a greater role in financial risk protection by reducing OOPE on healthcare. Expanded insurance coverage and improved benefits packages for individuals with metabolic multimorbidity are warranted.
Existing studies have revealed that multimorbidity has a significantly negative effect on physical and mental health outcomes (15–17). However, our findings indicated that individuals with co-existing metabolic diseases were more likely to have ADL limitation, but metabolic multimorbidity was not significantly associated with IADL limitation and depression. This may be explained by that metabolic multimorbidity is less likely to impair the instrumental activities and mental health of patients than other patterns of multimorbidity. Even so, metabolic diseases have been demonstrated to play a dominant role in multiple multimorbidity patterns (39). Among multimorbidity groups, the musculoskeletal group, as well as the cardiovascular and metabolic groups, were identified as having a significant risk of ADL limitation (16). It has been documented that the association between metabolic syndrome and negative cardiovascular outcomes or mortality (42). Our study provides new evidence that significant associations of metabolic multimorbidity with functional limitations are found among in rural areas rather than urban areas.
The literature on the impact of multimorbidity on healthcare utilization and spending among individuals with metabolic diseases is relatively limited in developing countries. This is the first nationally representative study that examined the effect of metabolic multimorbidity on health service use, costs and health outcomes in China, by using metabolic biomarkers for the disease diagnosis. However, our study has several limitations. First, we examined the effect of multimorbidity by simply counting the number of chronic conditions without accounting for the different clusters and severity of chronic diseases, hence the accuracy of the findings may be affected for the types of metabolic multimorbidity. Second, this study is a cross-sectional design, so it is difficult to demonstrate the causal relationship between metabolic multimorbidity and healthcare utilization and spending. Third, this study only included middle-aged and older populations due to unavailable data of younger populations, which may exaggerate the prevalence of metabolic multimorbidity and its impacts.
In conclusion, metabolic multimorbidity has become a huge public health challenge to individuals and healthcare systems in China and other developing countries. There is a growing need to provide effective services to counter the impact of chronic metabolic multimorbidity. Targeting strategies and measures must be taken to control and reduce the increasing prevalence of metabolic multimorbidity. Healthcare systems need to shift from single-disease models to integrated care models to more effectively manage metabolic diseases and multimorbidity. Prioritizing health and medical resources allocation is needed to prevent, screen, and treat metabolic multimorbidity in the future.
The datasets presented in this study can be found in online repositories. The names of the repository/repositories and accession number(s) can be found at: The datasets generated and analyzed during the current study are available in the China Health and Retirement Longitudinal Study repository. http://charls.pku.edu.cn/pages/data/111/en.html.
YZ and HW conceived, designed the study, and wrote the first draft of the paper. YZ did the initial analysis. HW supervised data analysis. PZ, BO, JL, AH, TH, and HW critically revised the first draft. All authors reviewed and approved the final manuscript submitted for publication.
This work was supported by the Shandong Province Natural Science Foundation of China (ZR2016GQ02).
The authors declare that the research was conducted in the absence of any commercial or financial relationships that could be construed as a potential conflict of interest.
We gratefully acknowledge the China Health and Retirement Longitudinal Study team for providing data and training in using the datasets. We are grateful to the students who participated in the survey for their cooperation. The authors thank all volunteers and staff involved in this research.
The Supplementary Material for this article can be found online at: https://www.frontiersin.org/articles/10.3389/fpubh.2021.658706/full#supplementary-material
1. Alberti KG, Eckel RH, Grundy SM, Zimmet PZ, Cleeman JI, Donato KA, et al. Harmonizing the metabolic syndrome: a joint interim statement of the international diabetes federation task force on epidemiology and prevention; national heart, lung, and blood institute; American heart association; World heart federation; international atherosclerosis society; and international association for the study of obesity. Circulation. (2009) 120:1640–5. doi: 10.1161/CIRCULATIONAHA.109.192644
2. Meigs JB. Invited commentary: insulin resistance syndrome? Syndrome X? Multiple metabolic syndrome? A syndrome at all? Factor analysis reveals patterns in the fabric of correlated metabolic risk factors. Am J Epidemiol. (2000) 152:908–11. doi: 10.1093/aje/152.10.908
3. Alberti KG, Zimmet P, Shaw J, Group IDFETFC. The metabolic syndrome–a new worldwide definition. Lancet. (2005) 366:1059–62. doi: 10.1016/S0140-6736(05)67402-8
4. Rutter MK, Meigs JB, Wilson PW. Cardiovascular risk and the metabolic syndrome. Metab Syndr Relat Disord. (2006) 4:252–60. doi: 10.1089/met.2006.4.252
5. Kivimaki M, Kuosma E, Ferrie JE, Luukkonen R, Nyberg ST, Alfredsson L, et al. Overweight, obesity, and risk of cardiometabolic multimorbidity: pooled analysis of individual-level data for 120813 adults from 16 cohort studies from the USA and Europe. Lancet Public Health. (2017) 6:e277–85. doi: 10.1016/S2468-2667(17)30074-9
6. Gu D, Reynolds K, Wu X, Chen J, Duan X, Reynolds RF, et al. Prevalence of the metabolic syndrome and overweight among adults in China. Lancet. (2005) 365:1398–405. doi: 10.1016/S0140-6736(05)66375-1
7. Xi B, He D, Hu Y, Zhou D. Prevalence of metabolic syndrome and its influencing factors among the Chinese adults: the China Health and Nutrition Survey in 2009. Prev Med. (2013) 57:867–71. doi: 10.1016/j.ypmed.2013.09.023
8. Boyd CM, Fortin M. Future of multimorbidity research: how should understanding of multimorbidity inform health system design? Public Health Rev. (2010) 32:451–74. doi: 10.1007/BF03391611
9. Palladino R, Lee JT, Millett C. Association between multimorbidity and healthcare utilisation and health outcomes among elderly people in Europe. Eur J Public Health. (2014) 24:49. doi: 10.1093/eurpub/cku151.118
10. Shang X, Peng W, Hill E, Szoeke C, He M, Zhang L. Incidence, progression, and patterns of multimorbidity in community-dwelling middle-aged men and women. Front Public Health. (2020) 8:404. doi: 10.3389/fpubh.2020.00404
11. Aubert CE, Fankhauser N, Marquesvidal P, Stirnemann J, Aujesky D, Limacher A, et al. Multimorbidity and healthcare resource utilization in Switzerland: a multicentre cohort study. BMC Health Serv Res. (2019) 19:1–9. doi: 10.1186/s12913-019-4575-2
12. Chandraratne NK, Pathirathna KG, Harrison C, Siriwardena AN. A comparison of policies and guidelines related to multimorbidity in the UK, Australia and Sri Lanka. Aust J Gen Pract. (2018) 47:14–19. doi: 10.31128/AFP-09-17-4346
13. Chen H, Cheng M, Zhuang Y, Broad JB. Multimorbidity among middle-aged and older persons in urban China: Prevalence, characteristics and health service utilization. Geriatr Gerontol Int. (2018) 18:1447–52. doi: 10.1111/ggi.13510
14. Chen H, Chen Y, Cui B. The association of multimorbidity with health care expenditure among the elderly patients in Beijing, China. Arch Gerontol Geriatr. (2018) 79:32–8. doi: 10.1016/j.archger.2018.07.008
15. Wang HH, Wang JJ, Wong SY, Wong MC, Li FJ, Wang PX, et al. Epidemiology of multimorbidity in China and implications for the health care system: cross-sectional survey among 162,464 community household residents in southern China. BMC Med. (2014) 12:188. doi: 10.1186/s12916-014-0188-0
16. Wang X, Lin W, Chen X, Lin Y, Huang L, Zhang S, et al. Multimorbidity associated with functional independence among community-dwelling older people: a cross-sectional study in southern china. Health Q Life Outcomes. (2017) 15:73. doi: 10.1186/s12955-017-0635-7
17. Arokiasamy P, Uttamacharya U, Jain K, Biritwum RB, Yawson AE, Wu F, et al. The impact of multimorbidity on adult physical and mental health in low- and middle-income countries: what does the study on global ageing and adult health (SAGE) reveal? BMC Med. (2015) 13:178. doi: 10.1186/s12916-015-0402-8
18. Zhao Y, Hu Y, Smith JP, Strauss J, Yang G. Cohort profile: the China Health and Retirement Longitudinal Study (CHARLS). Int J Epidemiol. (2014) 43:61–8. doi: 10.1093/ije/dys203
19. Barnett K, Mercer SW, Norbury M, Watt G, Wyke S, Guthrie B. Epidemiology of multimorbidity and implications for health care, research, and medical education: a cross-sectional study. Lancet. (2012) 380:37–43. doi: 10.1016/S0140-6736(12)60240-2
20. Zhao Y, Atun R, Oldenburg B, McPake B, Tang S, Mercer SW, et al. Physical multimorbidity, health service use, and catastrophic health expenditure by socioeconomic groups in China: an analysis of population-based panel data. Lancet Glob Health. (2020) 8:e840–9. doi: 10.1016/S2214-109X(20)30127-3
21. Chobanian AV, Bakris GL, Black HR, Cushman WC, Green LA, Izzo JL Jr, et al. National heart, lung, and blood institute joint national committee on prevention, detection, evaluation, and treatment of high blood pressure; national high blood pressure education program coordinating committee: the seventh report of the joint national committee on prevention, detection, evaluation, and treatment of high blood pressure: the JNC 7 report. JAMA. (2003) 289:2560–72. doi: 10.1001/jama.289.19.2560
22. Xu Y, Wang L, He J, Bi Y, Li M, Wang T, et al. Prevalence and control of diabetes in Chinese adults. JAMA. (2013) 310:948–59. doi: 10.1001/jama.2013.168118
23. Zhang M, Deng Q, Wang L, Huang Z, Zhou M, Li Y, et al. Prevalence of dyslipidemia and achievement of low-density lipoprotein cholesterol targets in Chinese adults: a nationally representative survey of 163,641 adults. Int J Cardiol. (2018) 260:196–203. doi: 10.1016/j.ijcard.2017.12.069
24. Feig DI, Kang DH, Johnson RJ. Uric acid and cardiovascular risk. N Engl J Med. (2008) 359:1811–21. doi: 10.1056/NEJMra0800885
25. Association CDSoM. Guideline to the prevention and treatment of type 2 diabetes in China (2013 Edition). Chin J Endocrinol Metab. (2014) 10:893–942. doi: 10.3760/cma.j.issn.1000-6699.2014.10.020
26. Lawton MP, Brody EM. Assessment of older people: self-maintaining and instrumental activities of daily living. Gerontologist. (1969) 9:179–86. doi: 10.1093/geront/9.3_Part_1.179
27. Andersen EM, Malmgren JA, Carter WB, Patrick DL. Screening for depression in well older adults: Evaluation of a short form of the CES-D. Am J Prev Med. (1994) 10:77–84. doi: 10.1016/S0749-3797(18)30622-6
28. Boey KW. Cross-validation of a short form of the CES-D in Chinese elderly. Int J Geriatr Psychiatry. (1999) 14:608–17. doi: 10.1002/(SICI)1099-1166(199908)14:8<608::AID-GPS991>3.0.CO;2-Z
29. Yang W, Reynolds K, Chen J, He J, Gu D. A comparison of two proposed definitions for metabolic syndrome in the Chinese adult population. Am J Med Sci. (2007) 334:184–9. doi: 10.1097/MAJ.0b013e3180a6ed66
30. Li R, Li W, Lun Z, Zhang H, Sun Z, Kanu JS, et al. Prevalence of metabolic syndrome in mainland china: a meta-analysis of published studies. BMC Public Health. (2016) 16:296. doi: 10.1186/s12889-016-2870-y
31. Lao XQ, Ma WJ, Sobko T, Zhang YH, Xu YJ, Xu XJ, et al. Dramatic escalation in metabolic syndrome and cardiovascular risk in a Chinese population experiencing rapid economic development. BMC Public Health. (2014) 14:983. doi: 10.1186/1471-2458-14-983
32. Aguilar M, Bhuket T, Torres S, Liu B, Wong RJ. Prevalence of the metabolic syndrome in the United States, 2003-2012. JAMA. (2015) 313:1973–4. doi: 10.1001/jama.2015.4260
33. Gundogan K, Bayram F, Gedik V, Kaya A, Karaman A, Demir O, et al. Metabolic syndrome prevalence according to ATP III and IDF criteria and related factors in Turkish adults. Arch Med Sci. (2013) 9:243–53. doi: 10.5114/aoms.2013.34560
34. Amirkalali B, Fakhrzadeh H, Sharifi F, Kelishadi R, Zamani F, Asayesh H, et al. Prevalence of metabolic syndrome and its components in the Iranian adult population: a systematic review and meta-analysis. Iran Red Crescent Med J. (2015) 17:e24723. doi: 10.5812/ircmj.24723
35. Kshatri JS, Palo SK, Bhoi T, Barik SR, Pati S. Prevalence and patterns of multimorbidity among rural elderly: findings of the AHSETS study. Front Public Health. (2020) 8:582663. doi: 10.3389/fpubh.2020.582663
36. Stephens CR, Easton JF, Robles-Cabrera A, Fossion R, de la Cruz L, Martínez-Tapia R, et al. The impact of education and age on metabolic disorders. Front Public Health. (2020) 8:180. doi: 10.3389/fpubh.2020.00180
37. Lu J, Wang L, Li M, Xu Y, Jiang Y, Wang W, et al. Metabolic syndrome among adults in China - the 2010 China noncommunicable disease surveillance. J Clin Endocrinol Metabo. (2016) 102:507–15. doi: 10.1210/jc.2016-2477
38. Wikstrom K, Lindstrom J, Harald K, Peltonen M, Laatikainen T. Clinical and lifestyle-related risk factors for incident multimorbidity: 10-year follow-up of Finnish population-based cohorts 1982-2012. Eur J Internal Med. (2015) 26:211–6. doi: 10.1016/j.ejim.2015.02.012
39. Noe G, Ai K, Somnath C, Tyrovolas S, Olaya B, Leonardi M, et al. Global multimorbidity patterns: a cross-sectional, population-based, multi-country study. J Gerontol. (2016) 71:205–14. doi: 10.1093/gerona/glv128
40. Palladino R, Tayu Lee J, Ashworth M, Triassi M, Millett C. Associations between multimorbidity, healthcare utilisation and health status: evidence from 16 European countries. Age Ageing. (2016) 45:431–5. doi: 10.1093/ageing/afw044
41. Bähler C, Huber CA, Brüngger B, Reich O. Multimorbidity, health care utilization and costs in an elderly community-dwelling population: a claims data based observational study. BMC Health Serv Res. (2015) 15:23. doi: 10.1186/s12913-015-0698-2
Keywords: metabolic disease, multimorbidity, healthcare spending, health outcome, Chinese adults
Citation: Zhao Y, Zhang P, Lee JT, Oldenburg B, Heusden Av, Haregu TN and Wang H (2021) The Prevalence of Metabolic Disease Multimorbidity and Its Associations With Spending and Health Outcomes in Middle-Aged and Elderly Chinese Adults. Front. Public Health 9:658706. doi: 10.3389/fpubh.2021.658706
Received: 27 January 2021; Accepted: 31 March 2021;
Published: 03 May 2021.
Edited by:
Mingsheng Chen, Nanjing Medical University, ChinaReviewed by:
Amâncio António De Sousa Carvalho, University of Trás-os-Montes and Alto Douro, PortugalCopyright © 2021 Zhao, Zhang, Lee, Oldenburg, Heusden, Haregu and Wang. This is an open-access article distributed under the terms of the Creative Commons Attribution License (CC BY). The use, distribution or reproduction in other forums is permitted, provided the original author(s) and the copyright owner(s) are credited and that the original publication in this journal is cited, in accordance with accepted academic practice. No use, distribution or reproduction is permitted which does not comply with these terms.
*Correspondence: Haipeng Wang, d2FuZ2hhaXBlbmdAc2R1LmVkdS5jbg==
Disclaimer: All claims expressed in this article are solely those of the authors and do not necessarily represent those of their affiliated organizations, or those of the publisher, the editors and the reviewers. Any product that may be evaluated in this article or claim that may be made by its manufacturer is not guaranteed or endorsed by the publisher.
Research integrity at Frontiers
Learn more about the work of our research integrity team to safeguard the quality of each article we publish.