- 1Theme Aging, Karolinska University Hospital, Stockholm, Sweden
- 2Department of Clinical Neuroscience, Karolinska Institutet, Stockholm, Sweden
SARS-CoV-2, the virus that causes COVID-19, spreads i. a., by respiratory droplets. The use of masks in preventing spread is controversial; masks are considered useless by many, while being mandated in some locations. Here, the effect of masking the general population on a COVID-19-like epidemic is estimated by computer simulation using three separate types of software. The main questions are whether mask use by the general population can limit the spread of SARS-CoV-2 in a country and how to identify opportunities when mask use is cost-effective and safe. To address these questions, the protective effects of different types of masks, the side-effects of masks, and avenues for improvements of masks and masking are addressed. Main results: (i) Any type of mask, even simple home-made ones, may be of value, even if the protective effect of each mask (here dubbed “one mask-protection”) is low. Strict adherence to mask use does not appear to be critical but increasing one mask-protection to >50% was found to be advantageous. (ii) Masks do seem to reduce the number of new cases even if introduced at a late stage in an epidemic, but early implementation helps reduce the cumulative and total number of cases. (iii) The simulations suggest that it might be possible to eliminate a COVID-19 outbreak by widespread mask use during a limited period. There is a brief discussion of why the reported effect size of masking varies widely, and is expected to do so, because of different filtration abilities of different masks, differences in compliance and fitting, other routes of transmission, pre-existing immunity, and because a system of interconnected, disease-prone individuals has non-linear properties. A software solution to visualize infection spread is presented. The results from these simulations are encouraging, but do not necessarily represent the real-life situation, so it is suggested that clinical trials of masks are now carried out while continuously monitoring effects and side-effects. As mask use is not without risks and costs, it is suggested that governments and scientists have an important role in advising the public about the sensible use of masks.
Introduction
Early in the COVID-19 epidemic, the World Health Organization recommended that face masks should only be used by health workers and people with confirmed or suspected coronavirus infection and their carers (1). Certain news items then discouraged the use of face masks (2). However, the WHO quickly changed opinion, and in China, which was reportedly very successful in containing the COVID-19 epidemic, there was widespread use of face masks, including by asymptomatic people (3). Hand hygiene is deemed the cornerstone of infection prevention (4). However, hand hygiene was promoted even before it was definitely known which procedures were active specifically for SARS-CoV-2, as discussed (4). It was noted that definitive claims on the effectiveness of various disinfectants against SARS-CoV-2 could not be made, simply because this is a new virus and the range of disinfectants had never been tested for SARS-CoV-2. Like other respiratory viruses, the new coronavirus spreads from person to person through airborne droplets, but other routes are known or suspected such as surfaces, where it can survive for days (5), so that touching infected surfaces can spread the virus [e.g., (4)].
Simple experiments suggest that masks may be effective against respiratory infections. For example, Johnson and colleagues had participants cough five times onto a Petri dish containing viral transport medium [cited in (6)]. Influenza virus could be detected by RT–PCR from all nine volunteers without a mask; no influenza virus could be detected when participants wore surgical or N95 masks. The same review concluded that there is some evidence to support the wearing of masks or respirators during illness to protect others. Tissue from a surgical mask was found to reduce the risk of COVID-19 transmission in hamsters (7). Hui et al. (8) found that masks can reduce the distance traveled by expelled air during a cough. Tracht and colleagues noticed that people are willing to wear face masks to protect themselves against infection (9). Using mathematical modeling, they concluded that if N95 respirators are 20% effective in reducing susceptibility and infectivity and 10% of the population wear them, the number of H1N1 cases is reduced by 20%.
A variety of masks and related devices exist, designed to protect the wearer or the environment. Medical masks, unfitted and disposable, can be used by infected individuals, healthcare professionals, or laymen to lower the transfer of infectious agents (10). Surgical masks are intended to limit contamination of wounds in surgery. A respirator, a type of mask, is fitted, can be disposable or reusable and protects the wearer against inhalation of harmful material. The National Institute for Occupational Safety and Health (NIOSH) regulates testing and certification of masks and similar respiratory protection equipment (11). In the European Union, similar standards are provided by the European Committee for Standardization. The NIOSH tests requires a minimum filtration efficiency of 95, 99, or 99.97% for an aerosol test (see standards for detailed specifications). The more protective masks may offer noticeable resistance to breathing and related to this, some individuals may find them difficult to wear for extended periods. The N95 respirator is a common mask that nominally filters at least 95% of airborne particles (12). It is indeed possible to obtain very close to 100% protection from respiratory pathogens such as SARS-CoV-2 using a Self-Contained Breathing Apparatus (SCBA). These are costly and require special training (often used by firefighters), see (13). However, an advanced mask, not SCBA, was tested with standardized methods, with influenza, rhinovirus, bacteriophage, Staphylococcus aureus, and model pollutants (14), >99.7% efficiency was found for the exclusion of influenza A virus, rhinovirus 14, and S. aureus, and >99.3% efficiency for paraffin oil and sodium chloride.
An important consideration that is often not mentioned when masks are discussed is the fact that they enable two barriers to be raised between an infected and an uninfected person. Masks worn simultaneously by infected and uninfected individuals would be expected to compound the reduction of transmission as follows: If the protection of one mask (“one mask-protection”) is x, and it is assumed that the size of the protective effect is the same for infected and uninfected persons, the total protection is (1-(1-x)2), illustrated in Figure 1 (solid orange line). This would amplify the protection afforded by the masks, and if an infected individual does not wear the mask properly, the masks of uninfected individuals nearby will offer some degree of protection, and vice versa. It should be noted that masks can be worn by infected individuals to protect other people or by uninfected individuals to avoid respiratory pathogens in their surroundings. Publications do not always make clear the distinction between output protection (from the former situation) and input protection (from the latter situation). Output and input protection may differ, and there is evidence that mask on source is often more effective than mask on receiver (15).
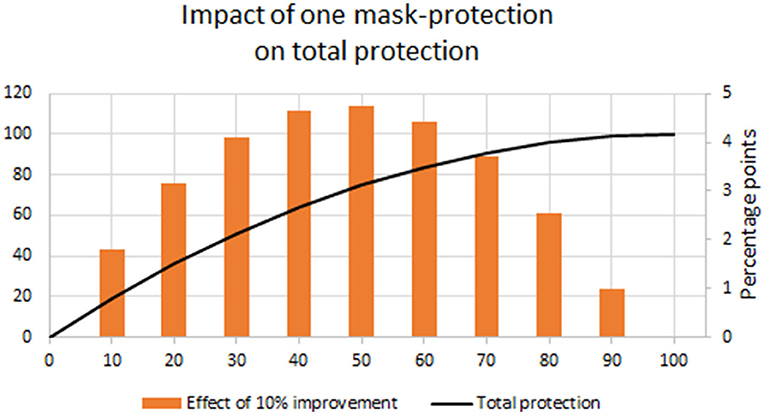
Figure 1. Total protection in % (by masking an infected and a healthy individual who are in contact) (orange line, scale to the left) and marginal utility of increased one mask-protection as a function of one mask-protection. The gray bars with the scale to the right show the effect on total protection (in percentage points) of a 10% increase in one mask-protection.
Transmission of Respiratory Pathogens Through Masks. Different Ways to Measure Transmission and Protection
The protective ability of a mask can be expressed and measured in different ways, but is not always discussed in a comprehensive manner. The share of virions (the individual virus particles that can be visualized by, e.g., electron microscopy) or other particles that pass through a mask is often termed “penetration” and determined as the ratio between the concentrations inside and outside the mask. The “efficiency” of a mask is a measure of how much of the agent is turned away by the mask and how much has 100% penetration (16).
Notice that at very high concentrations of virions (to the right in Figure 2), there is so much excess of virions received that the number of virions received becomes less important and therefore the risk reduction by one or even two masks becomes small. On the other hand, there might be a minimal infectious dose of virions, below which infection does not occur. This could mean that a small reduction in virion count by mask use might completely abolish infection. However, the existence of a minimal infectious dose is under debate, it may be situation-dependent, and for some viruses, the minimal infectious dose may be equal to 1 (17). Of possible relevance here is the curious observation that a high percentage of morphologically identical viral particles in a sample, as determined by electron microscopy, will often be non-infectious. This observation may turn out to be of great importance, as it means that virus particles detected by some methods might be non-infectious. To the author, this suggests that there are inefficiencies in virus production and storage that can be exploited, see below. In line with this, infectious penetration through a barrier can be lower than the physical penetration of virus particles, see below. There is evidence for a minimum infectious dose of MERS that is well above 1 (18). If this is the case for SARS-CoV-2, it might work together with the synergistic effect of two masks in series to multiply the number of virions needed to cause disease (Figure 1). A dose-response (illness) curve has been published for SARS (19).
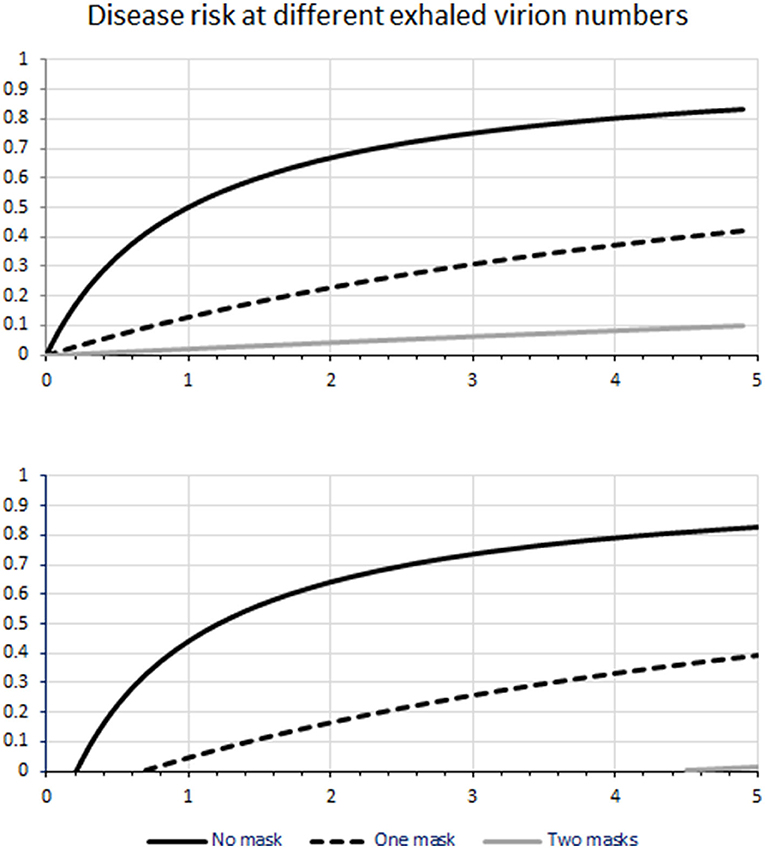
Figure 2. A sketch that attempts to illustrate the transmission of respiratory pathogens through masks worn by infected virus sources and uninfected recipients. The calculations assume a simple bimolecular interaction between the virus and its receptors. The units on the horizontal axis are arbitrary. The vertical axis shows the likelihood of being infected. Notice the short line in the lower right of (B); this figure concerns two mask-protection in case there would be a minimally infectious dose well above 1. (A) shows the situation in the absence of a minimal infectious dose.
Spread of Respiratory Infections: Droplets, Aerosols, and Other Routes
Several routes and modes exist for the transmission of respiratory infectious diseases; droplets may contribute to several of them. The modeling by Stilianakis and colleagues divided droplets into respirable droplets, with droplet diameters <10 μm, and inspirable droplets, with diameters in the range 10–100 μm. According to these authors, droplet dynamics is determined by their size, whereas population dynamics is determined by, i.a., pathogen infectivity and host contact rates. Robinson et al. of the team just mentioned (20) suggested that small droplets (~0.4 μm) have too small a viral load to be significantly infectious and that larger droplets (~4 μm) are the primary vehicle for infection. For SARS-CoV-2, a recent publication (21) argues from global trends in the number of infected individuals that airborne transmission is the dominant route, among several. Tellier et al. (22) has argued that SARS-CoV and MERS-CoV (viruses that cause SARS and MERS) may have to penetrate directly into the lower respiratory tract before causing disease; these authors also note that terminology in this area is not uniform. Whether an infectious agent is transferred by large droplets or airborne/aerosol may be important to the choice of protective equipment, as there is evidence that a conventional surgical mask is insufficient to protect against aerosol transmission, and that more elaborate masks may be more appropriate. It has been suggested that it can be transferred thorough the eyes [see (23)]. If this is a major route, the effect size of mask intervention would be smaller than filter penetration indicates, but eyewear would optimize protection.
Estimates of the Protection Offered by Masks
A recent literature review found evidence that simple cloth masks reduce virus spread, despite their meshes being larger in size than the virus as well as aerosol droplets (24). It was found that under unfavorable conditions, more than 3% of MS2 virions penetrated through filters of N99 and N95 respirators (25). Wiwanitkit and collaborators (26) found that the size of the pores of the N95 mask is about 300–500 nm in diameter whereas the size of the avian flu virus is about 100 nm (SARS size may be similar), i.e., 3–5 times larger than the virus and there is evidence that SARS can pass through N95s (27). Simple fabrics were reported to have 40–90% instantaneous penetration levels of polydisperse NaCl aerosols, much worse than for N95 respirator filter media. In addition, N95 masks also have about a 10% leakage problem around the mask. The study by Rengasamy et al. (28) suggests that the upper level of efficiency for the common N95 mask may be 85%, i.e., 10% leakage on the side and 5% penetration through the filter. At the velocity of coughing, one team found an about 50% filtration efficiency of ultrafine (0.02–0.1 μm) particles by factory-made masks (29).
As most studies on masks have been carried out with other pathogens than SARS-CoV-2, a pertinent question is whether the size of the protection of masks is similar for different respiratory pathogens or not. This is addressed in the work of Zuo et al. (30) which indicates that the efficiencies of masks for excluding different pathogens may be similar. Eninger (31) concluded that studying different masks to measure the penetration of simple NaCl aerosols may generally be appropriate for modeling filter penetration by virions.
Balazy et al. found evidence that different models of the same type of mask can have very different protective properties (25). Zuo et al. (30) found that although physical penetration of adenovirus and influenza virus aerosols through respirators can be substantial, 2–5%, infectivity penetration of adenovirus was much lower. The meta-analysis of Offeddu et al. (32) quantified the protective effect of face masks and respirators against clinical respiratory illness (risk ratio [RR] = 0.59) and influenza-like illness (RR = 0.34). Meta-analysis of observational studies provided evidence of a protective effect of masks (odds ratio OR = 0.13) and respirators (OR = 0.12) against severe acute respiratory syndrome (SARS).
Dbouk and Drikakis (33) presented a fluid dynamics study of the transmission of respiratory droplets through and around a face mask filter during coughing. These authors showed output (mask on infected individual) as well as input (mask on healthy individual) protection but the protection was limited to perhaps 80–90%. The systematic review by Jefferson et al. did not show a clear reduction in respiratory viral infection with the use of medical/surgical masks during seasonal influenza; the selected papers did not allow definite conclusions (34). However, Taiwan reportedly quickly eliminated their COVID-19 outbreak using a combination of interventions that included masking (35). The recent meta-review by Violante and Violante (36) suggests that surgical masks and N95/FFP2 respirators protect equally well-against airborne viral infections. The review just mentioned is also useful in that it summarizes the requirements for different classes of masks including differences between European and US standards, and that it lists some references in the field that are not cited directly in this paper. A German review found only weak evidence for masking being efficient as a hygienic tool to prevent virus spread, but better evidence for close contact scenarios. This review emphasizes potential risks (37). A number of different routes exist for SARS-CoV-2 transmission, some of them involving the eye (23). In the calculations of Sewell et al. (38), the estimated effect of the face mask mandate was a reduction in transmissibility (pt) of 23%. The effect of masking was presumed to be larger than this, because some individuals wore masks in the absence of a mandate. Cases selected here for closer study are shown in Table 1.
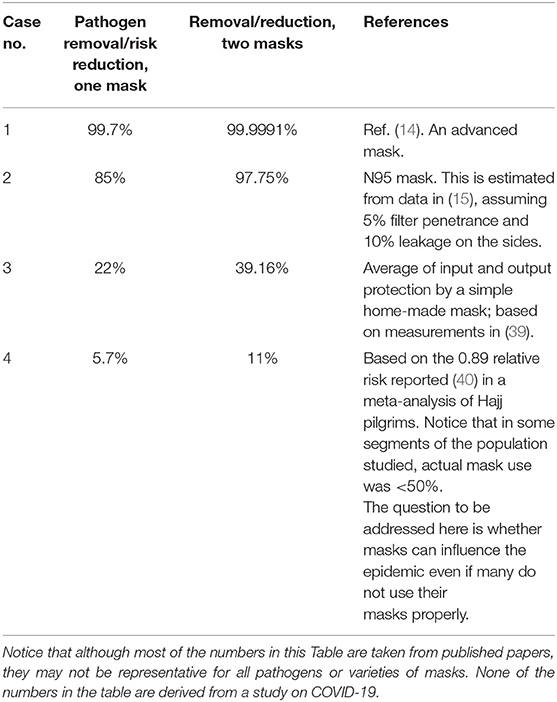
Table 1. Four cases representing four different levels of protection by mask that have been considered in this study and incorporated into the simulations that use COVID-19 Scenarios.
Calculations and Simulations to Address Early and Late Interventions
This study attempts to estimate the effect of masking the general population in a COVID-19 outbreak, first using simple considerations about the basic reproduction number of the epidemic and the level of protection from different types of masks. The basic reproduction number (R0), is an index of the contagiousness of an infection and depends on both the infectious agent and other factors. As R0 is the expected number of secondary infections produced by an index case in a completely susceptible population, it is often used to predict if an outbreak is expected to continue, as R0 >1 indicates that it will and R0 <1 indicates that it will not. However, this is a simplification and the calculations surrounding R0 can be complex (41, 42). After gathering some published numbers regarding the R0 of the COVID-19 epidemic and the protection afforded by masks, this study then moves on to simulations of the effect of masking with three separate types of software, one of them a specialized COVID-19 program and another one a simpler program intended for educational purposes.
Then the effect is addressed using computer simulations. Claims are sometimes made that disease-preventive measures must be enacted early in order to be effective. Early intervention has been claimed to be important also regarding COVID-19 [for example (43, 44)]. Both early and late intervention was simulated using the computer software.
Non-linear Systems Often Show Behavior That Is Not a Simple Function of the Size of a Disturbance or an Intervention
Since the initial results of this study were presented in a seminar and preprint form in the spring and summer of 2020, the finding that masks might have a dramatic effect on COVID-19 has sometimes been met with skepticism. A question asked repeatedly by colleagues is “How can the effect of an intervention be larger than the size of the intervention even to the point of eliminating an outbreak?” A related question sometimes asked is “How big an effect of an intervention such as masks is enough?” Spatial aspects have been brought up by suggestions that masking may be unnecessary in less populated areas [e.g., by Noren in a comment to (45)]. The questions just mentioned are now addressed here by considering a system of interconnected individuals. Highly interconnected systems can result in non-linear effects, e.g., in infection spread, as shown by Heesterbeek et al. (46). In the face of such complexity, mathematical models might aid the understanding of patterns of spread. The paper just cited also points to the existence of paradoxical effects, i.e., an intervention can sometimes have opposite effects depending on the state of the system.
In nervous system functioning as well as infection spread, units (neurons or persons) receive inputs (virus loads or synaptic activity) from many other units; when a threshold is attained (e.g., becoming infected or reaching firing threshold), the nodes distribute their activity back to the network of units (neurons/individuals) by releasing virions or neurotransmitters. Thus, a wave or cascade may move through the system, sometimes described mathematically as an avalanche. Systems prone to avalanches are often said to be in a critical state (47), i.e., within a narrow margin between avalanche propagation and extinction (48). A related but distinct concept is bifurcations, which are more applicable than critical points for certain systems but also indicate states at which a small change in one parameter can dramatically change the functioning of the system. Excitation/depression waves in interconnected networks can be very sensitive to parameters and be controlled by weak external forces (49). Varying a parameter such as synaptic strength (corresponding to transmissibility in infectious epidemiology) can at bifurcations select between dramatically different states of the system (50).
Despite many similarities, a difference between networks of neurons vs. networks of disease-prone humans is that neurons can normally fire nerve impulses many times, whereas a person will often have the same infection only once. This difference between neuronal networks and infectious network is not all-or-none, since neurons can have refractory periods or fatigue during which it is impossible or difficult to elicit a neuronal impulse. On the other hand, it is known that coronaviruses including SARS-CoV-2 can cause re-infection (51).
The programs COVID-19 Scenarios and Epidemix (detalied below in Materials and Methods) do not explicitly model the spatial aspects of infection spread and have turned out to be difficult to understand by some. A third software is therefore introduced in this paper as an attempt at simulate and visualize effects of imperfect interventions such as masks on infection spread in space using network simulation. Unfortunately, computer programs for network simulation that are easy to use are often very limited in scope and those that are flexible may require experience with computer programming and with a particular piece of software. Simbrain 3.0 (52) was chosen for this paper and is a program for the computer simulation of brain circuitry whose graphical user interface speeds the creation of networks. It also allows rather large networks consisting of thousands of components to be built by the writing of scripts. Simbrain can especially visualize the internal states of a network.
Despite seemingly thousands of publications about masks in COVID-19, only a few of them are articles that make use of simulation. Aspects in this paper that are little covered or not at all in previous publications are how mask protection of infected and uninfected wearers and a hypothetical minimal infectious dose combine quantitatively to limit transmission, time effects on effect size, fundamental limits on the impact of masking, and possible routes to improved masks. Of importance in the design of the simulations of this paper is that two existing software programs documented in publications were used with a minimum of modifications to parameters in order to reduce bias.
Materials and Methods
Since a commonly expressed opinion in the author's country was that masks in the general population were of little or no value, the author decided early on to use at least two separately developed and widely used pieces of software to arrive at a conclusive result regarding the effect, or lack thereof, of masking. At the time of inception of this study (early 2020), there were (at least) two major software programs for modeling the specific spread of COVID-19, COVASIM (53) and COVID-19 Scenarios (54). Since the web version of COVASIM (when tried by the author) offered less flexibility to input multiple parallel interventions, COVID-19 Scenarios was chosen. Epidemix 2 was used because of its simplicity, use in education in epidemiology, and because it is well-documented in publications. Simbrain 3.0 was used to begin addressing spatial aspects of COVID-19 spread, as there were large regional differences in COVID-19 cases and because it was proposed that masking could break chains of COVID-19 transmission. To avoid bias, software developed by others for computer simulations of a standard population level epidemiological models (such as COVID-19 Scenarios and Epidemix 2) were preferred, and default parameters of the software was used as much as possible.
As described in detail (54), COVID-19 Scenarios simulates a COVID-19 outbreak with a generalized SEIR model with the total population divided into age-strata (because of known age dependence of COVID-19 outcome) compartments of: susceptible (S), exposed (E), infected (I), hospitalized (H), critical (C), ICU overflow (O), dead (D), and recovered (R) individuals. People transition among the different compartments. The model allows researchers to specify individual interventions with start and end dates to model the existing (social distancing, case isolation, and quarantine) as well as additional interventions. COVID-19 Scenarios provides default parameters estimated from real-life statistics, although the authors emphasize the uncertainty behind these estimates. We ran the simulation for the United States. The model had been calibrated by its authors to match its age structure and the observed epidemiological statistics. The simulations did not consider saturation phenomena that might occur at very high virus counts (Figure 2), neither a possible minimal infectious dose of virions (Figure 2). It was assumed that transmission occurred only through routes that can be blocked by mask use.
Notice that although most of the numbers in Table 1 are taken from published papers, they need not be representative for all pathogens or varieties of masks. None of the numbers in the table are derived from a study with COVID-19.
The second software used in this paper is Epidemix 2 (55), which is a simplified software for teaching and demonstration purposes. It uses a visual interface to access eight models of epidemics without dealing with the details of mathematical equations and program code. The underlying calculations are done by a set of software packages in the programming language R. All models simulate disease spread through a population, allowing the user to select the model and characteristics of the population, interventions, etc.
Curve-fitting to estimate the slope of the curve of scenarios with different late mask interventions was done using the diagram function in Excel for Microsoft 365.
With the third piece of software, Simbrain 3.0 (52), a model was built of 100 nodes (representing persons or groups of persons) that can infect their nearest neighbors (shown by the oblique lines in Figure 3), but not beyond that. There are only excitatory connections. An impulse generator was added that enters activity (corresponding to virus loads) into the network through one of its nodes (in the upper left of the network) at random intervals. During simulations with this model, the effect of an increase in connection strength (i.e., transmissibility) of 10% as well as a 10% decrease in transmissibility is tested. Related work regarding the spread of HIV among interconnected individuals' social networks is described by Delva et al. (56).
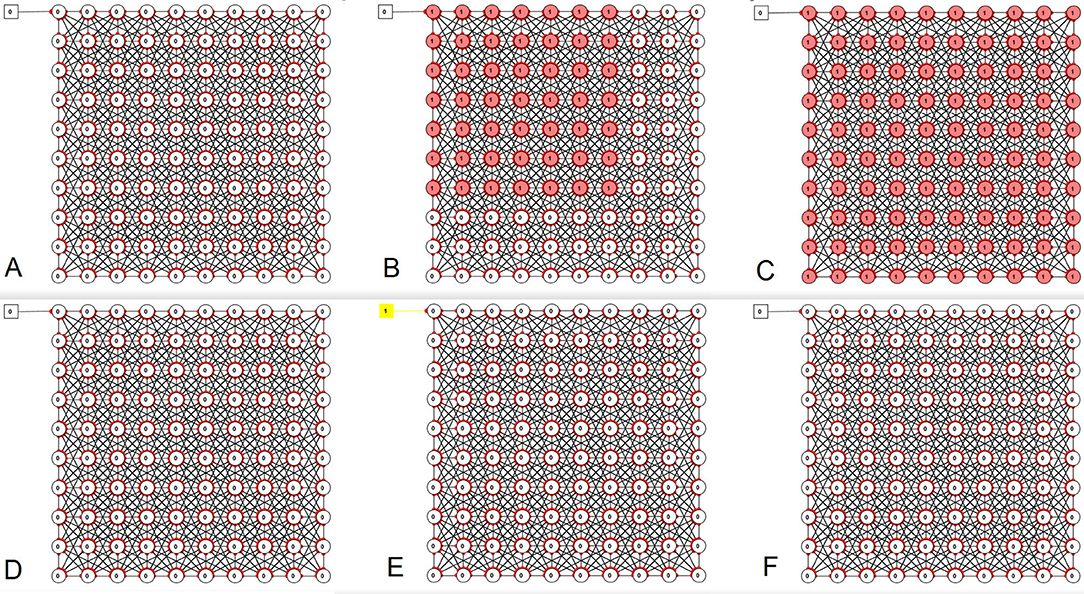
Figure 3. Use of Simbrain 3.0 to simulate infection spread among interconnected individuals. Notice that the current parameters have not been carefully calibrated against any particular real-life situation and should be considered rough first estimates used for illustration purposes. It can be seen that models can easily be found in which a relatively minor change in the transmissibility can have dramatic effects on the system. It can also be demonstrated that for many systems, which could be achieved with masking combined with a set of other interventions, it is impossible or almost so to achieve a wave of infection transmission in the system. Red color shows those nodes that are active (i.e., exhibiting neuronal activity of being infected). Upper row (A–C): Coupling between neurons 10% increased to enable a wave of infections to move through the system. Lower row (D–F): With 10% reduced coupling between individuals, it is very difficult to elicit a wave of infections in the same system, all other parameters are equal. (A/D), (B/E), and (C/F) show three sequential time points.
Results
A Simple Estimation of the Degree of Protection Afforded by Masks and the Protection Needed to Influence the Epidemic
The transmissibility of a virus is measured by the basic reproduction number (R0), which measures the average number of new cases generated per typical infectious case. As described by Rahman et al. (57) and references therein, an R0 of 1.0 is an important threshold value. If the R0 is equal to 1 or less, this indicates that the number of secondary cases will decrease over time and, eventually, the outbreak will peter out. One review evaluated the mean and median of the R0 estimated by the 12 articles and they calculated a final mean and median value of the R0 for COVID-19 of 3.28 and 2.79 (58) in line with a recent review (59). This seemingly provides a rough indication of how much the transmission must be reduced to reverse the epidemic. It appears that a reduction of transmission of at least two thirds is necessary. This is within the range of protection of some but not all masks (some 67% efficiency). However, as there would be two barriers between infected and non-infected individuals, the numbers for two serially connected masks should presumably be calculated, as the mask of the uninfected individual will add to the protection from the mask of the infected individual, indicating that many of the available masks might be adequate.
Simulations Using COVID-19 Scenarios Modeling Masks During the Start of an Outbreak
Figure 4 shows the simulated number of confirmed COVID-19 cases in the U.S. vs. date. The vertical axis is a logarithmic scale. The linearity of this graph shows the exponential growth expected early in an epidemic. We ran the simulation for the period ending with August 31, 2020. This was long enough to see the effects of masks on the time course of the epidemic. The default parameter values of COVID-19 Scenarios, used as input to this program, with mask interventions added as indicated in the text, are shown in Table 2. We wanted to observe the sensitivity of the outcomes to the time when mask intervention was started, rather than the effects of different mitigations implemented at the same time.
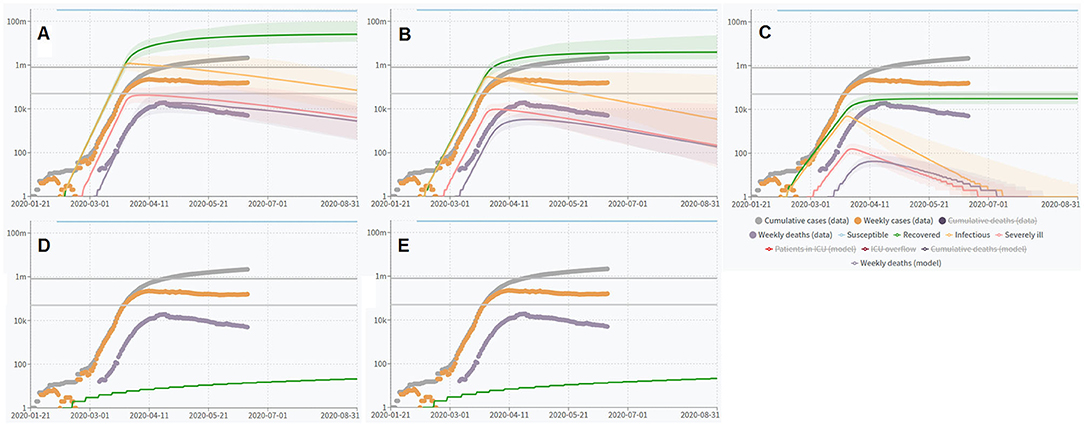
Figure 4. The effect of masking the general public on the COVID-19 epidemic, as estimated using a simulation by COVID-19 Scenarios. (A) No mask, (B) 11% protection; (C) 39% protection, (D) 97.75% protection, and (E) 99.9991% protection.
The results from the first runs with COVID-19 Scenarios show that mask use appears to be effective even at low one mask-protection or limited compliance. Even the lowest efficiency scenario reduced the simulated epidemic if applied from its beginning. The two highest protective abilities seem to practically completely abolish the epidemic. There was no indication of any difference between the two masks with the highest protection modeled (scenarios 1 and 2). It appears that even simple masks (e.g., 21% protection, scenario 2) or low-compliance mask-wearing (11% protection, scenario 1) reduces the number of COVID-19 cases within weeks.
Simulations Using a Simplified Model Using Epidemix Version 2
These simulations used the full defaults of Epidemix version 2, i.e., the parameters were not specifically calibrated for SARS-CoV-2 (deterministic homogenous model, infection states S, Is, R, population size: 100, daily number of effective contacts per unit: 0.4, length of infectious period: 10 days; Figure 5A). An added intervention with 75% two-mask protection, corresponding to a simple mask, was tested by reducing transmission by 75% (Figure 5B).
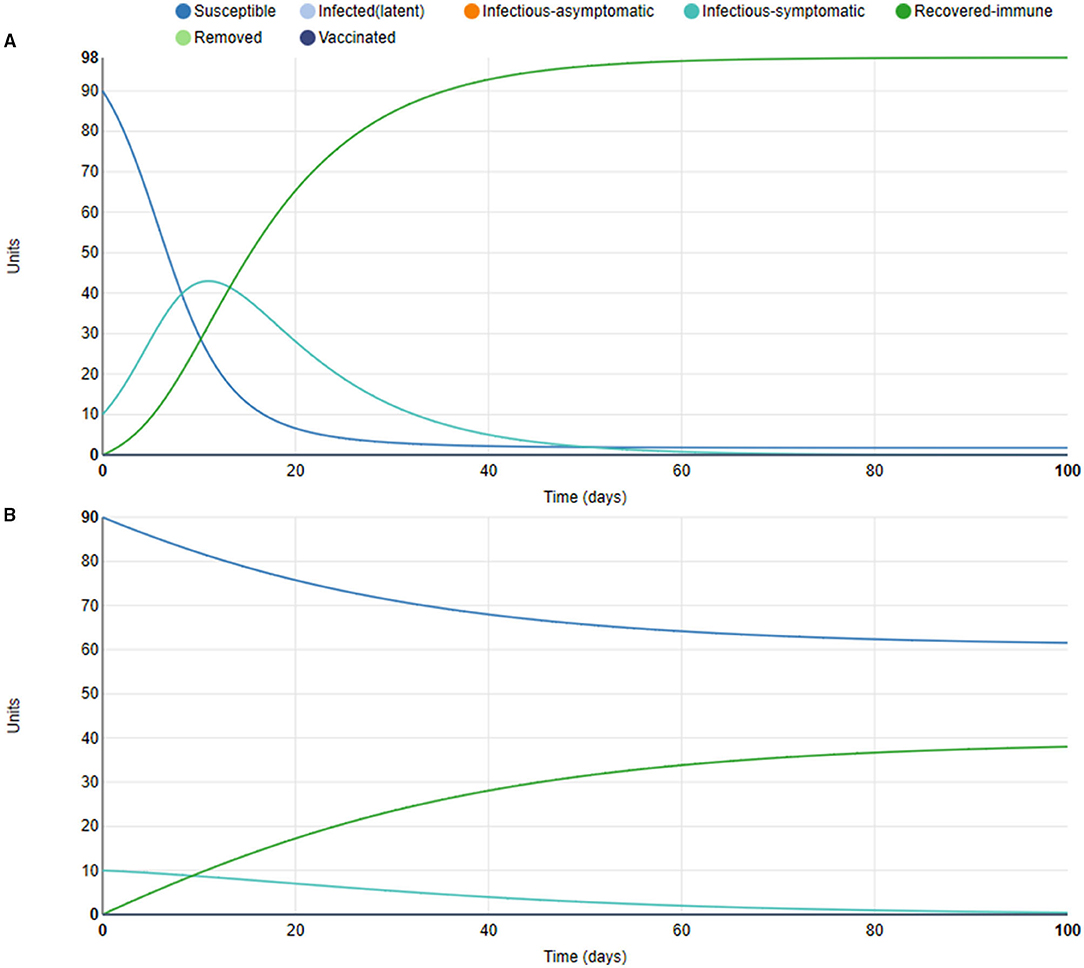
Figure 5. Simulations using Epidemix version 2. (A) 10% infected initially, otherwise full defaults of the Epidemix program. (B) 10% infected initially, 75% two-mask protection, otherwise full defaults of the Epidemix software.
As shown in Figure 5, the cumulative number of infected individuals during the whole simulation was only half in the mask use scenario as compared to the default scenario, with the effect of masking present throughout the period. The result seemed to corroborate the result from the COVID-19 Scenarios model that masks reduce COVID-19 cases.
Do Interventions Have to Be Applied Early?
From the results in Figures 6, 7 that shows curve-fitting of the data in Figures 6A,B for July and August 2020 only, it seems that they do not. When they are applied late, the total number of cases is influenced less than active cases, since a mask will not help individuals who have already been infected. However, the number of active cases is reduced in all mask interventions modeled, with some interventions resulting in a dramatic reduction. With case 4, in 11% two-mask protection, the reduction in new cases was about 11% per week. With case 1, reduction was quite dramatic at approximately 88% per week.
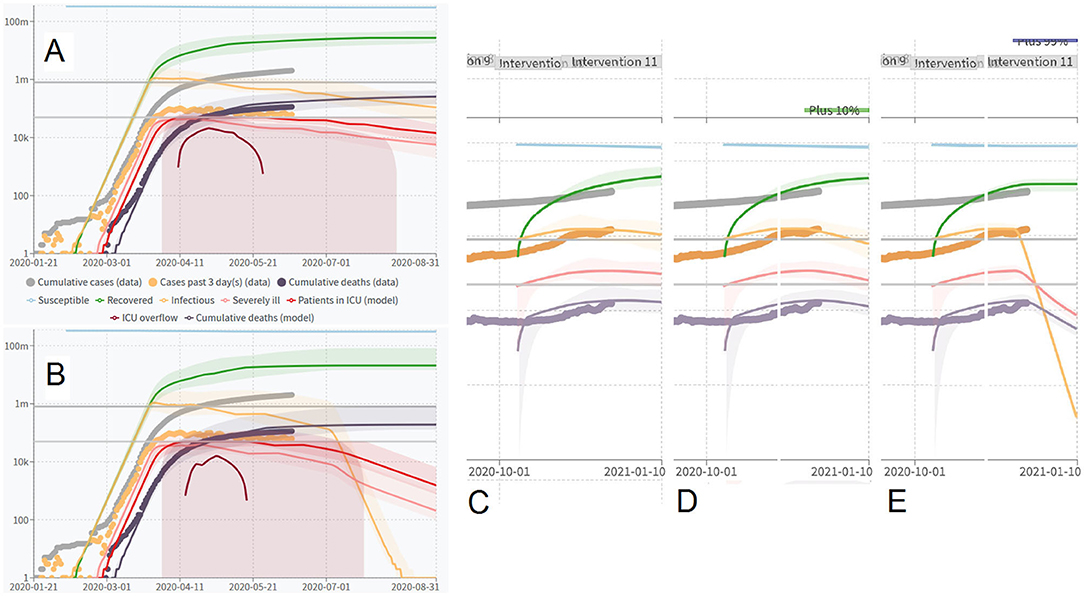
Figure 6. Effects of late mask interventions on the number of active COVID-19 cases. (A) Full defaults according to COVID-19 Scenarios. (B) Full defaults with late mask intervention using advanced mask (99.9991% two-mask protection). (C–E) show the effects of interventions beginning on December 1, 2020: (C), full defaults; (D), the effect of an added 10% protection, and (E), the effect of an added 99% protection intended to approximate the effect of population-wide wearing of SCBA devices.
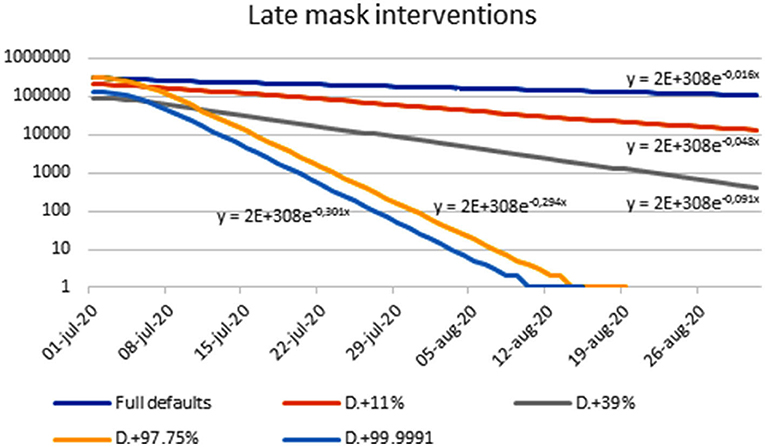
Figure 7. Estimating half-life and weekly reduction from the late use of four different masks (cases 1–4); vertical axis is “infectious” individuals from COVID-19 Scenarios.
An attempt was made to estimate the effect of population-wide masking in a situation when mask use was already high at the end of 2020 (Figures 6C,D) by adding an extra intervention of 10% to the defaults using the latest version of C19S. The effect of a virtually ideal intervention such as SCBA was also estimated (Figure 6E).
An Attempt at Simulating and Visualizing Effects of Imperfect Interventions Such as Masks on Infection Spread Using Simbrain Version 3.0
Using Simbrain, a network of 100 interconnected nodes was set up, feeding stimulating input (representing virions) to each other (Figure 3), originating from an impulse generator (upper left). During simulations with this model, the effect of a small increase in connection strength (i.e., transmissibility) of 10% as well as a 10% decrease in transmissibility was found to produce dramatic differences in the behavior of the interconnected system under some conditions. This shows that an imperfect intervention of about the magnitude of a simple mask, can at times have a dramatic effect on an interconnected system. Videos exemplifying the output of Simbrain are included in the Supplementary Materials.
Discussion
The results seem to indicate that it is possible to identify timepoints and situations at which an intervention of limited size, e.g., masking, will have a major effect on a COVID-19 epidemic and situations at which the wearing of masks is of little help, e.g., where there is a big excess of virions. Given the well-known propensity for “bugs” in large computer programs (presumably including those in epidemiology), this author thinks that publishing simulations with multiple software programs and by multiple, independent research teams is necessary. While it may be unrealistic to equip the whole world with SCBA equipment, widespread use of such equipment seems (even in locations with excess virions) likely to clear a circumscribed area of SARS-CoV-2 contagion. Modeling also seems to be of value for education on the spread of infection and the effects of masking. The effect size of masking may vary even more than from the different performance of different masks modeled above, due to differences in compliance and fitting of the mask, other routes of infection, pre-existing immunity but also because a system of interconnected, disease-prone individuals may have non-linear properties. As even the smallest mask intervention had a long-term effect in the simulations, the simplest mask could result in a large reduction in deaths if intensive care units are working close to capacity. That the effect size was found to compound over time is reminiscent of interest-on-interest in a bank account, when the interest becomes sizable after being applied repeatedly. When mask protection >50% was simulated, the effect on the size of the epidemic was dramatic, from about 1 million to zero fatalities (Table 3).
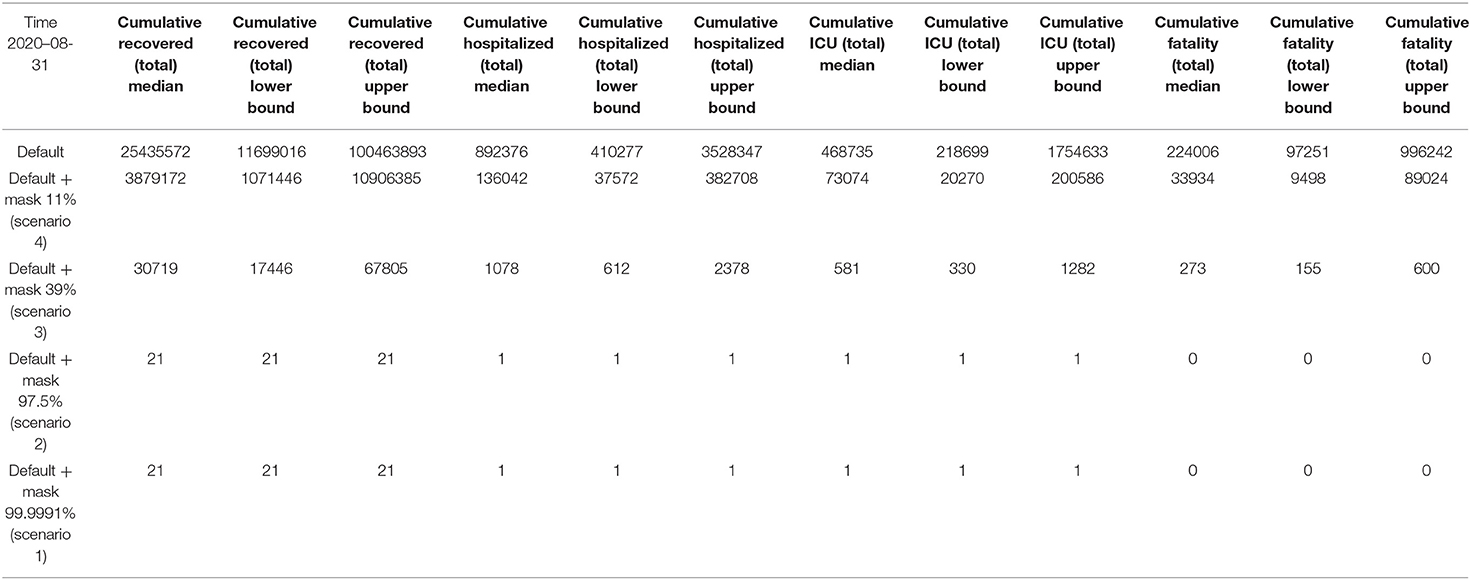
Table 3. Data from COVID-19 Scenarios regarding the number of individuals in the different compartments of the model (simulation performed summer 2020).
As the calculations illustrated in Figure 6 and Table 4 suggest that complete elimination of COVID-19 can be achieved in a closed community with <2 months of intervention with highly protective masks, it would be of interest to identify a community suitable for a clinical trial with such masks. Any such study should be carefully carried out taking local conditions (incl. legislation) into account and use continuous evaluation of infection parameters as well as any side effects of the masks. It should be said that the results above seem to be robust, as the principal results do not seem to depend on the precise model used or its input parameters, as slight variations of some parameters that have been tested have not altered the fundamental results.
Side Effects and Risks of Masks
There may be few comprehensive studies of side effects of masks. A recent systematic review found that side effects of masks were rarely measured in studies of masks against respiratory disease (34). From everyday experience, side effects and risks of mask use are usually limited to minor discomfort. Skin problems are among the most common side effects of masks. Pain and pressure from masks have been described during the COVID-19 pandemic, and remedies have been suggested (60). Gefen et al. studied device-related pressure ulcers in the context of COVID-19 (61). Ju et al. reported contact vitiligo from rubber ear loops from a mask (62). Contact dermatitis due to masks and solutions are described by Altobrando (63). Chaiabutre found skin reactions in persons wearing masks during the COVID-19 pandemic (64). Protruding ears in children has been mentioned as a possible side effect of masking (65). However, serious accidents from some masks may be possible: One of
the more serious side effects of mask-wearing seems to be that some masks may cause increased condensation on eyeglasses when worn concurrently (66), potentially obstructing the view of the wearer; however, counteracting devices are known. Heavy respiratory protective equipment used by firefighters can affect the balance of the user (67), potentially increasing the risk of fall accidents. Effects on breathing and blood gases have been described: Santos-Silva et al. (68) point to increasing resistance to air intake, lowering airflow into lungs, and causing temporary reduction in breathing rhythm. There may be symptoms related to hypercapnia (69)–breathing and gas exchange (70). A German review found evidence for significant respiratory compromise in patients with severe obstructive pulmonary disease, secondary to development of hypercapnia and possibly lung infections which emphasizes their potential risks (37). Kyung and coworkers (71) found that subjects with COPD found increased dyspnea, breathing frequency, and blood oxygen saturation after N95 use. It was suggested that N95 masks should be used with care in patients with more severe COPD. It was pointed out that lint and fibers from textiles are known to contribute to lung problems when inhaled in large quantities (29); it seems unclear how much this applies to masking during an epidemic. Psychological effects have been described: The review by Perna suggests that persons prone to panic attacks might experience discomfort due to increased respiratory physiological burden in RPD wearers' increased breathing resistance, CO2 rebreathing due to CO2 accumulation in the RPD cavity, and decreased inhaled O2 concentration (72). King has suggested that mandatory mask-wearing may give rise to difficulties in emotional communication due to impaired communication of facial expressions (73). Masking has been found to impair identification of faces both by human observers and face recognition computers [(74) and references therein]. One study found that mask reuse and use were associated with depression (75). Survival and growth of microorganisms and spread of infection have been reported: Bacteria can survive on surfaces of masks for several days (27). Personal protective equipment was reported to be a source of airborne infections (66). Bamber and Christmas (76) and others have pointed out that discarded masks may be a biohazard and must be discarded of properly. Reduced physical activity has been reported during mask-wearing (77). This section does not attempt to be a complete list of possible side effects of masks.
Possible Avenues for Improved Masks and Masking
There is scope for improvement of masks. It has been shown that it is possible to improve the protection against a certain class of infectious agents (e.g., chemical treatments) or generally (for example, improving the fitting of the masks against the face of the wearer). 3D printing is beginning to be used to compensate for the shortage of personal protective equipment including masks (78), and could be used with existing knowledge about mask fitting [e.g., (79, 80)] to fit masks to individual anatomy. Furthermore, in situations when there is solid evidence for the value of masking, educational activities could educate the public about this value and help with the selection, purchasing, fitting, use, and disposal of masks.
Viruses have unique biophysical properties including elasticity/deformability, brittleness/hardness, material fatigue, and resistance to osmotic stress (81) that might be targets for antiviral interventions, perhaps also to produce better masks. An antimicrobial surface contains an antimicrobial agent that inhibits the ability of microorganisms to grow on the surface of a material. For example, it was shown that surfaces that are simultaneously hydrophobic and oleophilic have quicker deactivation of enveloped viruses (82) (which include SARS-CoV-2, although influenza A was studied). Another example is that copper and its alloys destroy a wide range of microorganisms. Technology can permanently introduce copper oxide into polymeric materials that are biocidal. Masks filtered above 99.85% of aerosolized influenza and H9N2 virus, and infectious influenza virus could be recovered from the copper-modified masks (83). Quan and colleagues (84) tested functionalization of a surgical mask with a sodium chloride salt coating that dissolved on exposure to virus aerosol and recrystallized during drying; such filters showed better filtration than conventional masks and all mice survived influenza virus penetration through the rough salt-coated filters. It has been proposed that purposefully engineered materials with nanostructured surfaces can eliminate enveloped viruses such as SARS-CoV-2 i.a., on masks and be self-disinfectant (85). Other ways in which materials technology can improve masks is exemplified by a mask under development that lets some SARS-CoV-2 penetrate the mask, but heat-inactivates it (86). Guha et al. suggest that electret materials (i.e., those with a permanent electric charge) may be advantageous for masks (87). Relatively few studies with aerosols for specific illnesses seem to exist. It should be valuable to conduct such studies before an airborne epidemic hits next time.
Questions for Additional Research
In several studies, leakage on the sides of the mask has been greater than filter penetration of an agent. Therefore, efforts to improve fit or educate the public about proper mask-wearing are likely to be effective. The conclusion is that masks should be evaluated as an important addition to other ways of protection. They may have protective effects on the same order of magnitude as vaccines, but with the added advantages of being effective against a wide range of respiratory pathogens and can be prepared in advance and stored.
Questions that can be addressed in future research on masks include first and foremost a systematic study of side effects and risks associated with masks. Other research on masks would be a cost-benefit analysis to decide what level of protection is best when costs and side effects are considered. The gray bars in Figure 1 show the marginal improvement in two-mask protection from a 10% improvement in one mask-protection and indicates that the marginal benefit may be biggest from raising protection above 50%, and less benefit may be received from improvements in one mask-protection beyond 90%. It may be valuable to study masks with aerosols for specific illnesses before an airborne epidemic hits next time. Other research on masks would be to evaluate the shelf-life of different materials used in masks to select materials that allow for long-term storage preparation for future epidemics. Procedures for industrial and home manufacturing could be optimized. How to best educate individuals about the value of masks, how to properly wear a mask and perhaps how to make their own mask, and how to increase adherence to mask use are important questions to ask.
Questions that can be addressed regarding interactions with other interventions include do masking and social distancing work synergistically or do they work best on their own? In the simulations above, it implied that masks and other interventions depress SARS-CoV-2 transmission independently, but this is not necessarily the case in real life. Does the introduction of masks reduce people's compliance with other preventive measures, or do masks serve as a reminder of the epidemic, improving compliance with other measures? Can groups with low vaccine response [e.g., possibly those in (88)] be identified and should they wear a mask instead?
Questions that can be addressed in future research on SARS-CoV-2 (and other pathogens) would be to confirm and quantify different ways of transmission. Such information would inform decisions about whether a mask is of any value and in which situation it may be of value.
The results from these simulations are encouraging, but the only way to be sure about the effects of masks is to conduct prospective, controlled studies; perhaps along the lines of Lin et al. (89). Also, masks and related equipment are associated with significant side effects and risks that should be carefully monitored during any implementation. The numbers used here are consistent with the literature but do not represent the whole literature and many numbers are derived from studies of other agents than SARS-CoV-2. The work described here may still be relevant for SARS-CoV-2 as using slightly different input parameters or slightly different models typically did not change the outcome of a simulation much. During the past several months, many publications have come out in favor of mask use by the public [e.g., (21, 90–93)]. Stutt et al. (94) using somewhat different modeling from ours found parameter ranges in which mask compliance and effectiveness could reduce the R0 enough to slow or stop COVID-19 spread.
The use of simulation may help decide whether a small reduction of transmission with a simple mask is sufficient. It may also help identify situations when an advanced protective device is needed such as an advanced mask or even an SCBA device. A major general question is to identify all pathways for COVID-19 transmission and their relative importance. Alternative pathways will reduce the effect size of mask interventions but are likely amenable to interventions parallel to masking. If an alternative pathway is major, its obliteration may be necessary to achieve the “corona washout” suggested above. From the findings of this study, to optimize a mask intervention, it seems necessary for a government to recruit suitable competence in technology, mathematics, human behavior, and risk management, and to consult with community and religious leaders. For life-changing events such as funerals, trials with masking (or perhaps even with advanced equipment such as SCBA) and contact-tracing can be considered, to arrive at a protective equipment that avoids most infections while retaining the aspects of the ceremony as much as possible. Simulation can give a glimpse of what protection can be achieved and help in the design of such trials. If mask measures, as suggested here, are put into action, it will be important to generate hypotheses that can be tested to see if the actions really work and are cost-effective. Here various modeling strategies may be very helpful. For example, the concentration of virus could be measured in public places with and without the use of masks.
Data Availability Statement
The raw data supporting the conclusions of this article will be made available by the authors, without undue reservation.
Author Contributions
The author confirms being the sole contributor of this work and has approved it for publication.
Funding
This work was funded in part by internal funds of Stockholm County Council and Karolinska Institutet [Agreement concerning research and education of doctors (ALF) and the Research Funds of Karolinska Institutet].
Conflict of Interest
The author declares that the research was conducted in the absence of any commercial or financial relationships that could be construed as a potential conflict of interest.
Publisher's Note
All claims expressed in this article are solely those of the authors and do not necessarily represent those of their affiliated organizations, or those of the publisher, the editors and the reviewers. Any product that may be evaluated in this article, or claim that may be made by its manufacturer, is not guaranteed or endorsed by the publisher.
Acknowledgments
This paper is not health, medical, legal, or other professional advice. Please see disclaimers also for the software packages used in this paper. Some early results from this work haven been presented in preprint and seminar form: https://arxiv.org/abs/2006.15626; https://www.youtube.com/watch?v=ixkrqzSzcqQ.
Supplementary Material
The Supplementary Material for this article can be found online at: https://www.frontiersin.org/articles/10.3389/fpubh.2021.643991/full#supplementary-material
Supplementary Video 1. Examples of output from Simbrain 3.0 that illustrate that a small change in a parameter can cause dramatic changes in an interconnected system.
Supplementary Video 2. This output from Simbrain 3.0 shows that when the coupling between nodes is somewhat increased (synaptic strength 0.53, set with the Synaptic adjustment dialog of Simbrain), waves of activation (i.e., infectious individuals) are easily started. However, when the coupling between nodes is somewhat decreased (synaptic strength 0.48), it is practically impossible to set off such waves (as in Supplementary Video 1).
References
1. World Health Organization. Advice on the Use of Masks in the Community, During Home Care and in Healthcare Settings in the Context of the Novel Coronavirus (2019-nCoV) Outbreak: Interim Guidance. Available online at: https://apps.who.int/iris/handle/10665/330987 (accessed March 29, 2020).
2. H D. Can a Face Mask Protect Me From Coronavirus? Covid-19 Myths Busted. (2020). Available online at: https://www.theguardian.com/world/2020/apr/11/can-a-face-mask-protect-me-from-coronavirus-covid-19-myths-bustedmaincontent (accessed June 08, 2020).
3. Garcia LP. Use of facemasks to limit COVID-19 transmission. Epidemiol Serv Saude. (2020) 29:e2020023. doi: 10.1590/s1679-49742020000400019
4. How we know disinfectants should kill the COVID-19 coronavirus. C&EN Glob Enterprise. (2020) 98:7. doi: 10.1021/cen-09811-scicon2
5. van Doremalen N, Bushmaker T, Morris DH, Holbrook MG, Gamble A, Williamson BN, et al. Aerosol and surface stability of SARS-CoV-2 as compared with SARS-CoV-1. N Engl J Med. (2020) 382:1564–7. doi: 10.1056/NEJMc2004973
6. Cowling BJ, Zhou Y, Ip DK, Leung GM, Aiello AE. Face masks to prevent transmission of influenza virus: a systematic review. Epidemiol Infect. (2010) 138:449–56. doi: 10.1017/S0950268809991658
7. Chan JF, Yuan S, Zhang AJ, Poon VK, Chan CC, Lee AC, et al. Surgical mask partition reduces the risk of non-contact transmission in a golden Syrian hamster model for Coronavirus Disease 2019 (COVID-19). Clin Infect Dis. (2020) 71:2139–49. doi: 10.1093/cid/ciaa644
8. Hui DS, Chow BK, Chu L, Ng SS, Lee N, Gin T, et al. Exhaled air dispersion during coughing with and without wearing a surgical or N95 mask. PLoS ONE. (2012) 7:e50845. doi: 10.1371/journal.pone.0050845
9. Tracht SM, Del Valle SY, Hyman JM. Mathematical modeling of the effectiveness of facemasks in reducing the spread of novel influenza A (H1N1). PLoS ONE. (2010) 5:e9018. doi: 10.1371/journal.pone.0009018
10. Seale H, Dwyer DE, Cowling BJ, Wang Q, Yang P, Macintyre CR. A review of medical masks and respirators for use during an influenza pandemic. Influenza Other Respir Viruses. (2009) 3:205–6. doi: 10.1111/j.1750-2659.2009.00101.x
11. Respirator Trusted-Source Information. Section 1: NIOSH-Approved Respirators. Washington, DC: National Institute for Occupational Safety and Health (2018). Available online at: https://www.cdc.gov/niosh/npptl/topics/respirators/disp_part/respsource1quest1.html (accessed May 8, 2020).
12. Control CfD. Available online at: https://www.cdc.gov/niosh/npptl/topics/respirators/disp_part/n95list1.html (accessed June 22, 2020).
13. National Institute for Occupational Safety and Health. Respirator Fact Sheet. Washington, DC (2020). Available online at: https://www.cdc.gov/niosh/npptl/topics/respirators/factsheets/respfact.html (accessed May 9, 2020).
14. Zhou SS, Lukula S, Chiossone C, Nims RW, Suchmann DB, Ijaz MK. Assessment of a respiratory face mask for capturing air pollutants and pathogens including human influenza and rhinoviruses. J Thorac Dis. (2018) 10:2059–69. doi: 10.21037/jtd.2018.03.103
15. Patel RB, Skaria SD, Mansour MM, Smaldone GC. Respiratory source control using a surgical mask: an in vitro study. J Occup Environ Hyg. (2016) 13:569–76. doi: 10.1080/15459624.2015.1043050
17. Yezli S, Otter JA. Minimum infective dose of the major human respiratory and enteric viruses transmitted through food and the environment. Food Environ Virol. (2011) 3:1–30. doi: 10.1007/s12560-011-9056-7
18. Douglas MG, Kocher JF, Scobey T, Baric RS, Cockrell AS. Adaptive evolution influences the infectious dose of MERS-CoV necessary to achieve severe respiratory disease. Virology. (2018) 517:98–107. doi: 10.1016/j.virol.2017.12.006
19. Watanabe T, Bartrand TA, Weir MH, Omura T, Haas CN. Development of a dose-response model for SARS coronavirus. Risk Anal. (2010) 30:1129–38. doi: 10.1111/j.1539-6924.2010.01427.x
20. Robinson M, Stilianakis NI, Drossinos Y. Spatial dynamics of airborne infectious diseases. J Theor Biol. (2012) 297:116–26. doi: 10.1016/j.jtbi.2011.12.015
21. Zhang R, Li Y, Zhang AL, Wang Y, Molina MJ. Identifying airborne transmission as the dominant route for the spread of COVID-19. Proc Natl Acad Sci U S A. (2020) 117:14857–63. doi: 10.1073/pnas.2009637117
22. Tellier R, Li Y, Cowling BJ, Tang JW. Recognition of aerosol transmission of infectious agents: a commentary. BMC Infect Dis. (2019) 19:101. doi: 10.1186/s12879-019-3707-y
23. Peng M, Dai J, Sugali CK, Rayana NP, Mao W. The role of the ocular tissue in SARS-CoV-2 transmission. Clin Ophthalmol. (2020) 14:3017–24. doi: 10.2147/OPTH.S269868
24. Clase CM, Fu EL, Ashur A, Beale RCL, Clase IA, Dolovich MB, et al. Forgotten Technology in the COVID-19 pandemic: filtration properties of cloth and cloth masks-a narrative review. Mayo Clin Proc. (2020) 95:2204–24. doi: 10.1016/j.mayocp.2020.07.020
25. Balazy A, Toivola M, Adhikari A, Sivasubramani SK, Reponen T, Grinshpun SA. Do N95 respirators provide 95% protection level against airborne viruses, and how adequate are surgical masks? Am J Infect Control. (2006) 34:51–7. doi: 10.1016/j.ajic.2005.08.018
26. Wiwanitkit V. N-95 face mask for prevention of bird flu virus: an appraisal of nanostructure and implication for infectious control. Lung. (2006) 184:373–4. doi: 10.1007/s00408-006-0021-4
27. Brosseau LM, McCullough NV, Vesley D. Recovery and survival of three bacteria on respirator filters and surgical masks. Adv Filtrat Sep Technol. (1997) 11:372–6.
28. Rengasamy S, Miller A, Eimer BC. Evaluation of the filtration performance of NIOSH-approved N95 filtering facepiece respirators by photometric and number-based test methods. J Occup Environ Hyg. (2011) 8:23–30. doi: 10.1080/15459624.2010.515556
29. O'Kelly E, Pirog S, Ward J, Clarkson PJ. Ability of fabric face mask materials to filter ultrafine particles at coughing velocity. BMJ Open. (2020) 10:e039424. doi: 10.1136/bmjopen-2020-039424
30. Zuo Z, Kuehn TH, Pui DY. Performance evaluation of filtering facepiece respirators using virus aerosols. Am J Infect Control. (2013) 41:80–2. doi: 10.1016/j.ajic.2012.01.010
31. Eninger RM, Honda T, Adhikari A, Heinonen-Tanski H, Reponen T, Grinshpun SA. Filter performance of n99 and n95 facepiece respirators against viruses and ultrafine particles. Ann Occup Hyg. (2008) 52:385–96. doi: 10.1093/annhyg/men019
32. Offeddu V, Yung CF, Low MSF, Tam CC. Effectiveness of masks and respirators against respiratory infections in healthcare workers: a systematic review and meta-analysis. Clin Infect Dis. (2017) 65:1934–42. doi: 10.1093/cid/cix681
33. Dbouk T, Drikakis D. On respiratory droplets and face masks. Phys Fluids. (2020) 32:063303. doi: 10.1063/5.0015044
34. Jefferson T, Del Mar CB, Dooley L, Ferroni E, Al-Ansary LA, Bawazeer GA, et al. Physical interventions to interrupt or reduce the spread of respiratory viruses. Cochrane Database Syst Rev. (2020) 11:Cd006207. doi: 10.1002/14651858.CD006207.pub5
35. Cheng HY, Chueh YN, Chen CM, Jian SW, Lai SK, Liu DP. Taiwan's COVID-19 response: timely case detection and quarantine, January to June 2020. J Formos Med Assoc. (2021) 120:1400–4. doi: 10.1016/j.jfma.2020.10.023
36. Violante T, Violante FS. Surgical masks vs respirators for the protection against coronavirus infection: state of the art. Med Lav. (2020) 111:365–71. doi: 10.23749/mdl.v111i5.9692
37. Matuschek C, Moll F, Fangerau H, Fischer JC, Zanker K, van Griensven M, et al. Face masks: benefits and risks during the COVID-19 crisis. Eur J Med Res. (2020) 25:32. doi: 10.1186/s40001-020-00430-5
38. Sewell DK, Miller A, Program CDCM-H. Simulation-free estimation of an individual-based SEIR model for evaluating nonpharmaceutical interventions with an application to COVID-19 in the District of Columbia. PLoS ONE. (2020) 15:e0241949. doi: 10.1371/journal.pone.0241949
39. van der Sande M, Teunis P, Sabel R. Professional and home-made face masks reduce exposure to respiratory infections among the general population. PLoS One. (2008) 3:e2618.
40. Barasheed O, Alfelali M, Mushta S, Bokhary H, Alshehri J, Attar AA, et al. Uptake and effectiveness of facemask against respiratory infections at mass gatherings: a systematic review. Int J Infect Dis. (2016) 47:105–11.
41. Delamater PL, Street EJ, Leslie TF, Yang YT, Jacobsen KH. Complexity of the basic reproduction number (R(0)). Emerg Infect Dis. (2019) 25:1–4. doi: 10.3201/eid2501.171901
42. Volz E, Meyers LA. Epidemic thresholds in dynamic contact networks. J R Soc Interface. (2009) 6:233–41. doi: 10.1098/rsif.2008.0218
43. Jordan R. Stanford-Developed Interactive Model Explores How Different Interventions Affect COVID-19's Spread. (2020). Available online at: https://news.stanford.edu/2020/03/30/modeling-social-distancings-impact/ (accessed June 22, 2020).
44. Pei S, Kandula S, Shaman J. Differential effects of intervention timing on COVID-19 spread in the United States. Sci Adv. (2020) 6:eabd6370. doi: 10.1126/sciadv.abd6370
45. Torén K. Svag evidens för att munskydd minskar smitta hos allmänhet. Läkartidningen. (2020) 117:20141. Available online at: https://lakartidningen.se/klinik-och-vetenskap-1/kommentar/2020/08/svag-evidens-for-att-munskydd-minskar-smitta-hos-allmanhet/; https://lakartidningen.se/wp-content/uploads/2020/08/20141.pdf
46. Heesterbeek H, Anderson RM, Andreasen V, Bansal S, De Angelis D, Dye C, et al. Modeling infectious disease dynamics in the complex landscape of global health. Science. (2015) 347:aaa4339. doi: 10.1126/science.aaa4339
47. Beggs JM, Plenz D. Neuronal avalanches in neocortical circuits. J Neurosci. (2003) 23:11167–77. doi: 10.1523/JNEUROSCI.23-35-11167.2003
48. Ribeiro TL, Copelli M, Caixeta F, Belchior H, Chialvo DR, Nicolelis MA, et al. Spike avalanches exhibit universal dynamics across the sleep-wake cycle. PLoS ONE. (2010) 5:e14129. doi: 10.1371/journal.pone.0014129
49. Hanke W, Wiedemann M, de Lima VMF. Control of the excitability of neuronal tissue by weak external forces. Faraday Discuss. (2001) 120:237–48. doi: 10.1039/b102706a
50. Kundu A, Das P, Roy AB. Stability, bifurcations and synchronization in a delayed neural network model of n-identical neurons. Math Comput Simul. (2016) 121:12–33. doi: 10.1016/j.matcom.2015.07.006
51. Ringlander J, Nilsson S, Westin J, Lindh M, Martner A, Hellstrand K. Low incidence of reinfection with endemic coronaviruses diagnosed by real-time PCR. J Infect Dis. (2021) 223:2013–4. doi: 10.1093/infdis/jiaa627
52. Tosi Z, Yoshimi J. Simbrain 3.0: a flexible, visually-oriented neural network simulator. Neural Netw. (2016) 83:1–10. doi: 10.1016/j.neunet.2016.07.005
53. Kerr CC, Stuart RM, Mistry D, Abeysuriya RG, Rosenfeld K, Hart GR, et al. Covasim: an agent-based model of COVID-19 dynamics and interventions. PLoS Comput Biol. (2021) 17:e1009149. doi: 10.1371/journal.pcbi.1009149
54. Noll NB, Aksamentov I, Druelle V, Badenhorst A, Ronzani B, Jefferies G, et al. COVID-19 Scenarios: an interactive tool to explore the spread and associated morbidity and mortality of SARS-CoV-2. medRxiv [Preprint]. doi: 10.1101/2020.05.05.20091363
55. Muellner U, Fournié G, Muellner P, Ahlstrom C, Pfeiffer DU. epidemix—an interactive multi-model application for teaching and visualizing infectious disease transmission. Epidemics. (2018) 23:49–54. doi: 10.1016/j.epidem.2017.12.003
56. Delva W, Leventhal GE, Helleringer S. Connecting the dots: network data and models in HIV epidemiology. AIDS. (2016) 30:2009–20. doi: 10.1097/QAD.0000000000001184
57. Rahman B, Sadraddin E, Porreca A. The basic reproduction number of SARS-CoV-2 in Wuhan is about to die out, how about the rest of the World? Rev Med Virol. 2020:e2111. doi: 10.1002/rmv.2111
58. Liu Y, Gayle AA, Wilder-Smith A, Rocklöv J. The reproductive number of COVID-19 is higher compared to SARS coronavirus. J Travel Med. (2020) 27:taaa021. doi: 10.1093/jtm/taaa021
59. Hussein M, Toraih E, Elshazli R, Fawzy M, Houghton A, Tatum D, et al. Meta-analysis on serial intervals and reproductive rates for SARS-CoV-2. Ann Surg. (2021) 273:416–23. doi: 10.1097/SLA.0000000000004400
60. Field MH, Rashbrook JP, Rodrigues JN. Hydrocolloid dressing strip over bridge of nose to relieve pain and pressure from Filtered Face Piece (FFP) masks during the coronavirus (COVID-19) pandemic. Ann R Coll Surg Engl. (2020) 102:394–6. doi: 10.1308/rcsann.2020.0095
61. Gefen A, Ousey K. Update to device-related pressure ulcers: SECURE prevention. COVID-19, face masks and skin damage. J Wound Care. (2020) 29:245–59. doi: 10.12968/jowc.2020.29.5.245
62. Ju HJ, Lee JH, Kim GM, Park CJ, Bae JM. Contact vitiligo induced by rubber ear loops from a disposable sanitary mask. Eur J Dermatol. (2018) 28:690–1. doi: 10.1684/ejd.2018.3350
63. Di Altobrando A, La Placa M, Neri I, Piraccini BM, Vincenzi C. Contact dermatitis due to masks and respirators during COVID-19 pandemic: what we should know and what we should do. Dermatol Ther. (2020) 33:e14528. doi: 10.1111/dth.14528
64. Chaiyabutr C, Sukakul T, Pruksaeakanan C, Thumrongtharadol Waranya Boonchai J. Adverse skin reactions following different types of mask usage during the COVID-19 pandemic. J Eur Acad Dermatol Venereol. (2021) 35:e176–78. doi: 10.1111/jdv.17039
65. Zanotti B, Parodi PC, Riccio M, De Francesco F, Zingaretti N. Can the elastic of surgical face masks stimulate ear protrusion in children? Aesthetic Plast Surg. (2020) 44:1947–50. doi: 10.1007/s00266-020-01833-9
66. Morishima M, Kishida K. Understanding attitudes toward hygiene mask use in Japanese daily life by using a repeated cross-sectional survey. Work. (2018) 61:303–11. doi: 10.3233/WOR-182801
67. Brown MN, Char R, Henry SO, Tanigawa J, Yasui S. The effect of firefighter personal protective equipment on static and dynamic balance. Ergonomics. (2019) 62:1193–201. doi: 10.1080/00140139.2019.1623422
68. Santos-Silva PR, Greve JMD, Pedrinelli A. During the coronavirus (Covid-19) pandemic, does wearing a mask improve or worsen physical performance? Revista Brasileira De Medicina Do Esporte. (2020) 26:281–4. doi: 10.1590/1517-869220202604esp001
69. Özdemir L, Azizoglu M, Yapici D. Respirators used by healthcare workers due to the COVID-19 outbreak increase end-tidal carbon dioxide and fractional inspired carbon dioxide pressure. J Clin Anesth. (2020) 66:109901. doi: 10.1016/j.jclinane.2020.109901
70. Bourassa S, Bouchard PA, Lellouche F. Impact of gas masks on work of breathing, breathing patterns, and gas exchange in healthy subjects. Respir Care. (2018) 63:1350–9. doi: 10.4187/respcare.06027
71. Kyung SY, Kim Y, Hwang H, Park JW, Jeong SH. Risks of N95 Face mask use in subjects with COPD. Respir Care. (2020) 65:658–64. doi: 10.4187/respcare.06713
72. Perna G, Cuniberti F, Daccò S, Nobile M, Caldirola D. Impact of respiratory protective devices on respiration: implications for panic vulnerability during the COVID-19 pandemic. J Affect Disord. (2020) 277:772–8. doi: 10.1016/j.jad.2020.09.015
73. King FM. Covid-19: face masks could foster distrust and blame. BMJ. (2020) 369:m2009. doi: 10.1136/bmj.m2009
74. Carragher DJ, Hancock PJB. Surgical face masks impair human face matching performance for familiar and unfamiliar faces. Cogn Res Princ Implic. (2020) 5:59. doi: 10.1186/s41235-020-00258-x
75. Bressington DT, Cheung TCC, Lam SC, Suen LKP, Fong TKH, Ho HSW, et al. Association between depression, health beliefs, and face mask use during the COVID-19 pandemic. Front Psychiatry. (2020) 11:571179. doi: 10.3389/fpsyt.2020.571179
76. Bamber JH, Christmas T. Covid-19: each discarded face mask is a potential biohazard. (2020) 369:m2012. doi: 10.1136/bmj.m2012
77. Bundgaard H, Bundgaard JS, Raaschou-Pedersen DET, von Buchwald C, Todsen T, Norsk JB, et al. Effectiveness of adding a mask recommendation to other public health measures to prevent SARS-CoV-2 infection in danish mask wearers : a randomized controlled trial. Ann Intern Med. (2021) 174:335–43. doi: 10.7326/M20-6817
78. Clifton W, Damon A, Martin AK. Considerations and cautions for three-dimensional-printed personal protective equipment in the COVID-19 crisis. 3D Print Addit Manuf . (2020) 7:97–9. doi: 10.1089/3dp.2020.0101
79. Golshahi L, Telidetzki K, King B, Shaw D, Finlay WH. A pilot study on the use of geometrically accurate face models to replicate ex vivo N95 mask fit. Am J Infect Control. (2013) 41:77–9. doi: 10.1016/j.ajic.2012.01.008
80. O'Kelly E, Arora A, Pearson C, Ward J, Clarkson P. (2020). Performing qualitative mask fit testing without a commercial kit: fit testing which can be performed at home and at work. Disaster Med Public Health Prep. 1–5. doi: 10.1017/dmp.2020.352
81. Buzon P, Maity S, Roos WH. Physical virology: from virus self-assembly to particle mechanics. WIREs Nanomed Nanobiotechnol. (2020) 12:e1613. doi: 10.1002/wnan.1613
82. Mannelli I, Reigada R, Suarez I, Janner D, Carrilero A, Mazumder P, et al. Functionalized surfaces with tailored wettability determine influenza A infectivity. ACS Appl Mater Interfaces. (2016) 8:15058–66. doi: 10.1021/acsami.6b02779
83. Borkow G, Zhou SS, Page T, Gabbay J. A novel anti-influenza copper oxide containing respiratory face mask. PLoS ONE. (2010) 5:e11295. doi: 10.1371/journal.pone.0011295
84. Quan FS, Rubino I, Lee SH, Koch B, Choi HJ. Universal and reusable virus deactivation system for respiratory protection. Sci Rep. (2017) 7:39956. doi: 10.1038/srep39956
85. Toledo GG, Toledo VH, Lanfredi AJC, Escote M, Champi A, Silva M, et al. Promising nanostructured materials against enveloped virus. An Acad Bras Cienc. (2020) 92:e20200718. doi: 10.1590/0001-3765202020200718
86. Faucher S, Daniel JL, Liang XA, Jin X, Phillips R, Parviz D, et al. A virucidal face mask based on the reverse-flow reactor concept for thermal inactivation of SARS-CoV-2. AIChE J. (2021) 67:e17250. doi: 10.1002/aic.17250
87. Guha S, McCaffrey B, Hariharan P, Myers MR. Quantification of leakage of sub-micron aerosols through surgical masks and facemasks for pediatric use. J Occup Environ Hyg. (2017) 14:214–23. doi: 10.1080/15459624.2016.1237029
88. Lopez A, Mariette X, Bachelez H, Belot A, Bonnotte B, Hachulla E, et al. Vaccination recommendations for the adult immunosuppressed patient: a systematic review and comprehensive field synopsis. J Autoimmun. (2017) 80:10–27. doi: 10.1016/j.jaut.2017.03.011
89. Lin A, Ercumen A, Benjamin-Chung J, Arnold BF, Das S, Haque R, et al. Effects of water, sanitation, handwashing, and nutritional interventions on child enteric protozoan infections in rural Bangladesh: a cluster-randomized controlled trial. Clin Infect Dis. (2018) 67:1515–22. doi: 10.1093/cid/ciy320
90. Clase CM, Fu EL, Joseph M, Beale RCL, Dolovich MB, Jardine M, et al. Cloth masks may prevent transmission of COVID-19: an evidence-based, risk-based approach. Ann Intern Med. (2020) 173:489–91. doi: 10.7326/M20-2567
91. Ngonghala CN, Iboi E, Eikenberry S, Scotch M, MacIntyre CR, Bonds MH, et al. Mathematical assessment of the impact of non-pharmaceutical interventions on curtailing the 2019 novel Coronavirus. Math Biosci. (2020) 325:108364. doi: 10.1016/j.mbs.2020.108364
92. Walcott DA. What is the Evidence on Wearing Masks to Stop COVID-19? (2020). Available online at: https://www.weforum.org/agenda/2020/04/should-we-be-promoting-the-widespread-use-of-masks/ (accessed April 19, 2020).
93. Javid B, Weekes MP, Matheson NJ. Covid-19: should the public wear face masks? (2020) 369:m1442. doi: 10.1136/bmj.m1442
Keywords: mask, simulation–computers, visualization, health policies, innovation, COVID-19, side-effects, non-linear systems
Citation: Johansson B (2021) Challenges and Controversies in COVID-19: Masking the General Population may Attenuate This Pandemic's Outbreak. Front. Public Health 9:643991. doi: 10.3389/fpubh.2021.643991
Received: 19 December 2020; Accepted: 22 June 2021;
Published: 08 September 2021.
Edited by:
Lydia Gimenez-Llort, Autonomous University of Barcelona, SpainReviewed by:
Tolulope Olumide Afolaranmi, University of Jos, NigeriaJessica Snowden, University of Arkansas for Medical Sciences, United States
Copyright © 2021 Johansson. This is an open-access article distributed under the terms of the Creative Commons Attribution License (CC BY). The use, distribution or reproduction in other forums is permitted, provided the original author(s) and the copyright owner(s) are credited and that the original publication in this journal is cited, in accordance with accepted academic practice. No use, distribution or reproduction is permitted which does not comply with these terms.
*Correspondence: Björn Johansson, bjorn.johansson@ki.se