- 1Division of Intramural Research, National Institute on Minority Health and Health Disparities, Bethesda, MD, United States
- 2Department of Environmental Health Sciences, University of Alabama at Birmingham, Birmingham, AL, United States
- 3Department of Health Services Management and Policy, College of Public Health, East Tennessee State University, Johnson City, TN, United States
Introduction: Long–standing disparities in colorectal cancer (CRC) outcomes and survival between Whites and Blacks have been observed. A person–centered approach using latent class analysis (LCA) is a novel methodology to assess and address CRC health disparities. LCA can overcome statistical challenges from subgroup analyses that would normally impede variable–centered analyses like regression. Aim was to identify risk profiles and differences in malignant CRC survivorship outcomes.
Methods: We conducted an LCA on the Surveillance, Epidemiology, and End Results data from 1975 to 2016 for adults ≥18 (N = 525,245). Sociodemographics used were age, sex/gender, marital status, race, and ethnicity (Hispanic/Latinos) and stage at diagnosis. To select the best fitting model, we employed a comparative approach comparing sample-size adjusted BIC and entropy; which indicates a good separation of classes.
Results: A four–class solution with an entropy of 0.72 was identified as: lowest survivorship, medium-low, medium-high, and highest survivorship. The lowest survivorship class (26% of sample) with a mean survival rate of 53 months had the highest conditional probabilities of being 76–85 years–old at diagnosis, female, widowed, and non-Hispanic White, with a high likelihood with localized staging. The highest survivorship class (53% of sample) with a mean survival rate of 92 months had the highest likelihood of being married, male with localized staging, and a high likelihood of being non-Hispanic White.
Conclusion: The use of a person–centered measure with population-based cancer registries data can help better detect cancer risk subgroups that may otherwise be overlooked.
Introduction
Colorectal cancer (CRC) remains the third most diagnosed cancer, and the second leading cause of cancer-related death in both men and women in the United States (US) (1). There are an estimated 147,950 new cases and 53,200 deaths expected in 2020, accounting for 8.4% of all cancer deaths (1, 2). Despite the current estimates, CRC incidence and mortality rates have been decreasing overall since 2000 (1, 3), while incidence rates among adults aged ≤50 years have increased since the mid-1990s (3–5). The reasons for the decline in CRC incidence and mortality rates include advancements in biomedical sciences, leading to early detection and diagnosis, as well as improved treatment, increased screening outreach on a population basis, and adherence to interventions on behavioral lifestyle risk factors like smoking cessation (1, 3).
Regardless of the decline in CRC incidence, mortality, and survival, geographical and racial/ethnic disparities persist (1, 3, 6–12). For instance, between 1995 and 2014 the lowest CRC incidence rate was 29.7/100,000 individuals in Utah while the highest was 49.2/100,000 in Kentucky (1). Similarly, CRC mortality rates range from 11.0/100,000 population in Connecticut to 18.3/100,000 population in Mississippi (1). In terms of race/ethnicity, evidence has shown that non-Hispanic Blacks and American Indians/Alaska Natives experience the highest CRC incidence and mortality rates, compared to non-Hispanic Whites (1). Therefore, there is critical need for research to understand these disparities and to inform the development of interventions to reduce/eliminate them.
The stage of CRC diagnosis is important to treatment, recovery, and survival (3). According to the American Cancer Society, the overall 5-year relative rate for localized stage diagnosis is 90%, regional 71%, distant 14%, and all stages combined 63% (13). Modifiable and non-modifiable risk factors, including age, genetics, sedentary lifestyle, and socioeconomic status (SES) have been known to affect CRC development (7, 14–16). Several studies have examined differences in CRC incidence, mortality, and survival by these factors (12, 16–31). Zhang et al. (31) used the Surveillance, Epidemiology, and End Results (SEER) data from 2007 to 2013 to investigate the impact of SES on overall CRC survival. Results revealed that patients with CRC who were non-Hispanic Black, widowed, on Medicaid, and with the lowest education had relatively poor prognoses. However, studies involving the analysis of overall survivorship of patients with CRC in population subgroups in the US are sparse. As such, this exploratory study aimed to identify profiles and determine disparities in malignant CRC survivorship outcomes using SEER 9 cancer registry program incidence databases from 1975 to 2016. The findings will help identify heterogenous, mutually exclusive profiles and provide important information about how interventions should be tailored to different subpopulations.
Materials and Methods
Source and Study Population
This study used data from the SEER database of the National Cancer Institute. The SEER 9 covers ~10% of the US population with data from nine cancer registries in the states of Georgia (Atlanta), Connecticut, Michigan (Detroit), Hawaii, Iowa, New Mexico, California (San Francisco), Washington (Seattle-Puget Sound), and Utah (32). Patients included in this study were non-Hispanic Blacks and Whites age ≥18 years diagnosed from 1975 through 2016 with malignant, histologically confirmed primary colon and rectal cancer under the International Classification of Diseases for Oncology, Third Edition (ICD-O-3) histology and behavior code. This study was exploratory and not designed to be diagnostic in nature.
Latent Class Analysis
We used latent class analysis (LCA), a person-centered approach, to identify latent or hidden profiles in data. LCA transitions us from variable-centered approaches that examine relationships between variables to a person-centered context in which we can further identify subgroups of risk by profile. We conducted an LCA on SEER 9 data to identify and assess differences in CRC survivorship by observed indicators of sociodemographic factors and derived cancer stage. Observed indicators within the profiles were assessed as conditional probabilities, i.e., likelihood of each indicator being present within the profile with all other indicators present. The distal outcome of survivorship was a continuous measure of survival in total number of months from cancer diagnosis until recorded all-cause death. An automatic Bolck, Croon, and Hagenaars (BCH) method in our LCA was used to account for the distal continuous outcome of survivorship and assess mean differences by profile identified. This approach minimizes bias as algorithms, not the researcher, identify profiles based on observed indicators and survivorship.
Observed indicators from patient sociodemographic characteristics included in our LCA were age, sex/gender, race, Hispanic/Latino origin, and marital status. Age at diagnosis was categorized using the US Preventive Service Task Force screening age recommendation (33) (i.e., 18–49; 50–75; 76–85; 85 and older). Sex/gender was based on dichotomous male or female categories, and race was made into three categories. The first two racial categories included individuals that self-identified as either White or Black. The third racial category, Other race, was a combination of participants that self-identified as American Indian/Alaska Natives and Asian or Pacific Islanders, based on the SEER race recode changes (34). We included Hispanic/Latino as a dichotomous yes or no category based on North American Association of Central Cancer Registries Hispanic Identification Algorithm (35). Marital status was categorized as single/never married; married/common law; divorced/separated; and widow/widower. The derived stage of CRC was categorized as localized, regional, or distant.
Model Fit Assessment for Latent Class Analysis
Multiple models were created based on number of classes (i.e., 1-, 2-, 3-, 4-, 5-class solutions) and compared using the following criteria: (1) entropy [i.e., the acceptable quality of classification and indication of good separation of classes]; (2) Bayesian information criterion (BIC) and sample-size-adjusted BIC (ssa-BIC); and (3) theoretical implications (36). This comparative approach allowed us to select our final model for interpretation based on high entropy, as well as parsimony assessed via BIC, ssa-BIC, and practical application. All statistical analyses were conducted using Mplus 8.4 (Muthén and Muthén).
Results
Sociodemographic Characteristics
A total of 525,245 patients with malignant CRC were included. Of these patients, a little over half were males (50.4%), aged 50–75 years (57%), and married (57%) at the time of CRC diagnosis. Most of the patients were non-Hispanic White (83.5%) with localized stage disease (41%). The mean survival time from CRC diagnosis was 77.3 ± SE months [SE = 0.123, 95% CI: 77.1–77.6] (Table 1).
Patients' risk profiles/survival subgroups were identified using LCA model fit assessment (Table 2). The best model fit selected was a four-class solution that had a low ssa-BIC (4,662,336.8) and an entropy of 0.72, which indicated a clear separation of classes or profiles. The classes were named by relative survival in months from CRC diagnosis until death from all-cause mortality.
Latent Class Analysis Subgroups/Profiles of Survivorship
Class 1, or the lowest survivorship group (26% of sample), had ~53 months of survival from diagnosis. The lowest survival group had the highest conditional probabilities of being 76–85 years old at time of diagnosis (43.8%), female (78.9%), and widowed (85.2%) and had a high likelihood of being White (86.8%). This subgroup had the highest conditional probability of having malignant localized stage disease (40.8%).
Class 2, or the medium-low survivorship group (3% of sample), had 71.7 months of survival from diagnosis until death (Table 3). This subgroup had the highest conditional probabilities of being White (99%), Hispanic/Latino (100%) and male (58.2%) or female (41.8%). The medium-low survivorship group had high probabilities of being 50–75 years old at the time of diagnosis (66.8%), married (63.3%), and of localized stage CRC (39.3%). In addition, this subgroup had the second highest probability of regional stage malignant CRC diagnosis (38%).
The Class 3, or medium-high survivorship group represents 17% of the study sample (Table 3). The Class 3 group also had 73.5 months of survival since diagnosis with the highest conditional probabilities of being 50–75 (71.0%) years at diagnosis, single/never married (31.1%), divorced/separated (22.3%), and Black (25.7%). This group also had an almost equal probability of being male (52.3%) or female (48.7%) with distant stage disease (28.1%).
The last risk profile subgroup identified in our LCA is Class 4, or the highest survivorship group (53% of sample), with 91.7 months of survival from diagnosis (Table 3). This subgroup had the highest conditional probabilities of being male (65.1%), married/common law marriage (91.7%), and localized stage CRC diagnosis (44.3%). Class 4 had high probabilities of being between 50 and 75 years old (64.6%) and non-Hispanic White (88.1%). The highest survivorship group also had the lowest conditional probability of distant stage disease (17.9%). See Table 3 for detailed mean survivorship and conditional probabilities.
Equity test of survivorship means across classes using the automatic BCH procedure with 3 degrees of freedom for overall test was found significant (x2 = 17587.5, p < 0.001; see Table 4). That is, mean survivorship was significantly different between profiles.
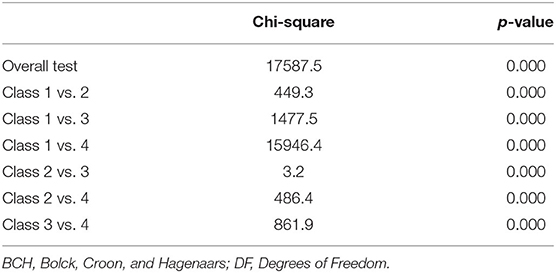
Table 4. Equity test survivorship means across classes using BCH procedure with 3 degrees of freedom for overall test (N = 525,245).
Discussion
This study used LCA, a person-centered method, to identify profiles of survivorship among patients with malignant CRC in a large population-based SEER cancer registry. This analysis of more than 525,000 patients with CRC diagnosed between 1975 and 2016 found heterogenous profiles by survivorship, age at diagnosis, sex/gender, race, ethnicity, marital status, and cancer derived staging. Four profiles of CRC survivorship were identified: lowest survivorship (53.0 months), medium-low survivorship (71.7 months), medium-high survivorship (73.5 months), and highest survivorship (91.7 months).
We identified that the highest survivorship profile (91.7 months; Class 4) had the highest conditional likelihoods of being married and diagnosed with localized disease, followed by a high likelihood of being 50–75 years of age, White, and male. The lowest survivorship profile (Class 1) with 53.0 months from diagnosis to death had the highest likelihoods of being female, widowed, older (i.e., 76–85 years of age), and with regional disease. The lowest survivorship profile also had the second highest likelihood of localized disease when compared to the highest survivorship profile. As such, marital status and sex/gender had the greatest disparity in survivorship. These findings were consistent with Aizer et al. (37), Jin et al. (38), Johansen et al. (39), Li et al. (40), and Wang et al. (41) who reported that married patients with cancer were less likely to present with CRC metastasis and survived significantly longer, compared to unmarried and widowed patients.
The profile identified with medium-low survivorship was found to be exclusively Hispanic/Latino and had the second highest likelihoods of being diagnosed at a distant stage and of being between 50 and 75 years. While the medium-low profile also had the second highest likelihood of being married (63%) when compared to all other profiles, it also had the second highest likelihoods of being single/never married and divorced/separated. The medium-high survivorship profile had the highest likelihood of being Black when compared to all other profiles. This profile also had the highest likelihoods of being single/never married and divorced/separated. Our findings revealed that disparities in CRC survival outcomes may not be attributable to race/ethnicity alone, but to other factors related to marital status for both males and females. Studying the impact of marriage on CRC stage at diagnosis and survival using SEER dataset, Li et al. (40) found that CRC cause specific survival among the married group was almost 70% compared to the never married (59%), divorced/separated/widowed groups (60%). The reason for these disparities are attributed to higher rates of depression, anxiety, medication non-adherence, and negative emotions among widowed patients (42–44).
Overall, while disparities in CRC mortality and survivorship have been found in prior studies, our study has expanded the limited literature concerning CRC disparities using a person-centered approach. We have identified four heterogenous survivorship profiles that are affected by multiple interacting factors, not just by racial/ethnic categories. While prior studies have found associations in CRC incidence and survival by race/ethnicity and age group (5, 20, 21, 45–48), these associations have been found to vary by database. For instance, Gabriel et al. (20) used the National Cancer Data Base (NCDB) from 2006 to 2012 to analyze CRC differences in demographic and pathologic factors with age related rates and overall survival. Results indicated disparities in overall survival, but African American and Hispanic/Latino patients aged ≤50 years experienced increased morality (20). In contrast, Murphy et al. (5) investigated CRC incidence and relative survival using SEER 13 registries data (1992–2014) among younger adults, aged ≤50, and found that while absolute CRC incidence was higher for Blacks than Whites, Blacks experienced a slightly higher 5-year relative survivorship improvement with colon cancer, and increased survival with rectal cancer (i.e., from 55.5 to 70.8%) (5).
Racial and ethnic health disparities have long been associated with CRC disparities, with many persisting if not worsening and shifting the burden of morbidity and mortality to other medically underserved and underrepresented groups. Our exploratory, person-centered study identified racial/ethnic CRC disparities in survivorship among CRC patients. By identifying the unique and inextricable context of racial/ethnic groups that may play a critical role in disease progression may also play a role in efficiently and efficaciously addressing CRC disparities. For instance, we found that the medium-low and medium-high survivorship profiles had the highest likelihoods to belonging to an ethnic/racial minority (Hispanic or non-Hispanic Black, respectively). We observed, however, that in these profiles there were increased likelihoods of being single/never married or divorced/separated. Additionally, these profiles had a decreased likelihood of being diagnosed at a localized stage; especially, when compared to the lowest and highest survivorship profiles that were primarily racially White and non-Hispanic. Epidemiological studies have previously found that minority and underserved populations, like that of US Blacks, have worse CRC prognoses compared to Whites (1, 3–5, 7), with only few studies reporting no significant difference (49, 50).
Our findings revealed that racial/ethnic disparities in the context of available sociodemographic characteristics have heterogenous profiles of survivorship based on race/ethnicity but nuanced by marital status. While cancer registries are expanding data collection to discern risk factors for cancer incidence, prevalence, and outcomes, marital status may be a more reliable indicator for survivorship in the absence of available contextual risk factors. For instance, in a Tennessee cancer registry study by Montiel Ishino et al. (51), among patients with malignant CRC, White widowed women were found to have the greatest likelihood of delay for CRC surgical treatment followed by Blacks regardless of health insurance status when compared to White married men, i.e., the profile with the lowest likelihood of surgical treatment delay. Black patients were also more likely to be single/never married or divorced/separated, with a lower likelihood of delayed surgical treatment, than White widowed women. However, they had a higher likelihood of delay when compared to White married men (51).
Our LCA study is among the first to differentiate between profiles using the distal continuous outcome of survivorship. Policy-level and public health recommendations, as well as clinical implications, can be garnered from our exploratory, person-centered analysis and findings. Considerations should be given to improved data collection at cancer registries to enhance risk assessments. In this manner, CRC interventional studies can be designed by leveraging large datasets such as SEER that are publicly available to better tailor interventions and prevention programs. Using person-centered methods, we can move beyond associations between variables to examine the context of variables among subpopulations. By using these person-centered approaches, we can better approximate CRC patient profiles to identify the most salient factors within profiles and prioritize care and access at a clinical level. In this manner we can better promote and tailor screenings and intervene upon factors related to decreased survivorship among CRC patient subpopulations. For instance, while CRC screening interventions are in place, the role of marital status must be further examined within the scope of these interventions to understand the direct and indirect effects it has on survivorship. Furthermore, indicators such as SES, access to quality care and provider expertise must also be assessed to truly capture a person-centered, multilevel context. However, these factors were not available in the SEER 9 database. Geographic and ecological data would have made the person-centered context much richer by including socioeconomic status (e.g., family income; education) and environmental exposures (e.g., tobacco smoke; pollutants).
Our findings indicated that derived stage of CRC alone may not be sufficient to predict CRC survival outcomes, but rather it is a constellation of social determinants. It is, therefore, crucial that while we focus on the social determinants of health in understanding cancer disparities that we contextually examine risk factors that interact at the person-level to mitigate subpopulation disparities and promote health equity. The relationship between race and cancer survival is a complex one (52, 53). Several interacting factors including tumor type, grade, stage, comorbidities, access to healthcare/quality services, provider expertise, and SES are known to confound this relationship and contribute to these disparities (1, 3, 15, 46). Regardless, our LCA, using a distal continuous outcome of survivorship, provides a proof of concept to identify the complex context of CRC associated variables to account for multiple complex interactions on possible risk profiles. Future research directions would explore the effects of race/ethnicity, social support, and cancer staging to understand the complex and dynamic interaction of multiple determinants of health and cancer health disparities. We would then examine the protective and risk factors that may be associated with marital status, in addition to how psychological characteristics correlate with survivorship.
Limitations
This study adds to the current literature by identifying how CRC survival outcome disparities exist using a large population-based SEER database, as well as differentiating between profiles to demarcate the extent of the disparity. Nonetheless, a number of limitations should be addressed. The first is the level of representativeness to generalize findings to the US population, although the sample is very large. SEER datasets primarily include data from White individuals in urban metro areas. Second, SEER registries do not collect SES variables such as income, education, employment, health insurance status, as well as quality of healthcare patients received. In addition, some sociodemographic variables reported may be inaccurate. For example, marital status is only collected at the time of diagnosis. Individuals whose status changed are never updated and other environmental factors are also not available. Despite these limitations, the SEER program has a reputation of reporting long-term, high quality incidence, prevalence, and survival data (3). Currently, the program covers over 28% of the US population, which serves as a major data source for cancer stage distribution, stage-specific survival, and lifetime incidence of developing cancer (54).
Conclusions
The use of a person–centered measures such as LCA with population-based cancer registry data can help better detect cancer risk subgroups that may otherwise be overlooked. This study identified four risk subgroups: lowest, medium-low, medium-high, and highest survivorship subgroups. Of interest is the fact that racial or sociodemographic disparities alone do not account for differences in invasive CRC survival. Hence, this study revealed that Whites have almost equal chances of both good and poor CRC prognosis while Blacks continue to experience worse outcomes. Females, Hispanics, and widowed patients have poorer survival outcomes among the risk profiles/subgroup identified in this study. Thus, in developing tailored interventions for CRC, these high-risk subgroup populations should be considered in order to improve malignant CRC survivorship.
Data Availability Statement
Publicly available datasets were analyzed in this study. These data can be found at: https://seer.cancer.gov/data-software/.
Author Contributions
FM: conceptualization, methodology, software, formal analysis, writing-original draft preparation, and visualization. EO: writing-original draft preparation and writing-review and editing. KV: writing-review and editing, software, and validation. XL: software, data curation, and validation. BS: data curation and writing-review and editing. HM: supervision and writing-reviewing and editing. FW: project administration, supervision, resources, and writing-reviewing and editing. All authors: contributed to the article and approved the submitted version.
Funding
BS, KV, FM, XL, and FW efforts were supported by the Division of Intramural Research, National Institute on Minority Health and Health Disparities, National Institutes of Health.
Disclaimer
The content is solely the responsibility of the authors and does not necessarily reflect the views of the National Institutes of Health.
Conflict of Interest
The authors declare that the research was conducted in the absence of any commercial or financial relationships that could be construed as a potential conflict of interest.
The reviewer SG declared a shared affiliation, with no collaboration, with several of the authors, FM, KV, XL, BS, and FW to the handling editor at the time of review.
References
1. Siegel RL, Miller KD, Goding Sauer A, Fedewa SA, Butterly LF, Anderson JC, et al. Colorectal cancer statistics, 2020. CA Cancer J Clin. (2020) 70:145–64. doi: 10.3322/caac.21601
2. National Cancer Institute Surveillance E and End Results Program. Cancer Stat Facts: Colorectal Cancer. (2020). Available online at: https://seer.cancer.gov/statfacts/html/colorect.html
3. Siegel RL, Miller KD, Jemal A. Cancer statistics, 2020. CA Cancer J Clin. (2020) 70:7–30. doi: 10.3322/caac.21590
4. Holowatyj AN, Ruterbusch JJ, Rozek LS, Cote ML, Stoffel EM. Racial/ethnic disparities in survival among patients with young-onset colorectal cancer. J Clin Oncol. (2016) 34:2148–56. doi: 10.1200/JCO.2015.65.0994
5. Murphy CC, Wallace K, Sandler RS, Baron JA. Racial disparities in incidence of young-onset colorectal cancer and patient survival. Gastroenterology. (2019) 156:958–65. doi: 10.1053/j.gastro.2018.11.060
6. Callahan KE, Ponce CP, Cross CL, Sy FS, Pinheiro PS. Low colorectal cancer survival in the Mountain West state of Nevada: a population-based analysis. PLoS ONE. (2019) 14:e0221337. doi: 10.1371/journal.pone.0221337
7. Carethers JM, Doubeni CA. Causes of socioeconomic disparities in colorectal cancer and intervention framework and strategies. Gastroenterology. (2020) 158:354–67. doi: 10.1053/j.gastro.2019.10.029
8. White A, Vernon SW, Franzini L, Du XL. Racial disparities in colorectal cancer survival: to what extent are racial disparities explained by differences in treatment, tumor characteristics, or hospital characteristics? Cancer. (2010) 116:4622–31. doi: 10.1002/cncr.25395
9. Andrilla CHA, Moore TE, Man Wong K, Evans DV. Investigating the impact of geographic location on colorectal cancer stage at diagnosis: a national study of the SEER cancer registry. J Rural Health. (2019) 36:316–25. doi: 10.1111/jrh.12392
10. Carnahan LR, Jones L, Brewer KC, Watts EA, Peterson CE, Ferrans CE, et al. Race and gender differences in awareness of colorectal cancer screening tests and guidelines among recently diagnosed colon cancer patients in an urban setting. J Cancer Educ. (2019). doi: 10.1007/s13187-019-01666-4. [Epub ahead of print].
11. Carroll R, Zhao S. Trends in colorectal cancer incidence and survival in Iowa SEER data: the timing of it all. Clin Colorectal Cancer. (2019) 18:e261–74. doi: 10.1016/j.clcc.2018.12.001
12. Frankenfeld CL, Menon N, Leslie TF. Racial disparities in colorectal cancer time-to-treatment and survival time in relation to diagnosing hospital cancer-related diagnostic and treatment capabilities. Cancer Epidemiol. (2020) 65:101684. doi: 10.1016/j.canep.2020.101684
13. American Cancer Society. Colorectal Cancer Survial. (2021). Available online at: https://www.cancer.org/cancer/colon-rectal-cancer/detection-diagnosis-staging/survival-rates.html
14. Dekker E, Tanis PJ, Vleugels JLA, Kasi PM, Wallace MB. Colorectal cancer. Lancet. (2019) 394:1467–80. doi: 10.1016/S0140-6736(19)32319-0
15. Sineshaw HM, Ng K, Flanders WD, Brawley OW, Jemal A. Factors that contribute to differences in survival of black vs white patients with colorectal cancer. Gastroenterology. (2018) 154:906–15.e7. doi: 10.1053/j.gastro.2017.11.005
16. Zhang D, Matthews CE, Powell-Wiley TM, Xiao Q. Ten-year change in neighborhood socioeconomic status and colorectal cancer. Cancer. (2019) 125:610–7. doi: 10.1002/cncr.31832
17. Akinyemiju T, Sakhuja S, Waterbor J, Pisu M, Altekruse SF. Racial/ethnic disparities in de novo metastases sites and survival outcomes for patients with primary breast, colorectal, and prostate cancer. Cancer Med. (2018) 7:1183–93. doi: 10.1002/cam4.1322
18. Al-Husseini MJ, Saad AM, Gad MM, Rachid A, Salama AH, Hilal T. Racial disparities in colorectal cancer survival: a population-based cohort. J Clin Oncol. (2018) 36(Suppl. 15):e15643. doi: 10.1200/JCO.2018.36.15_suppl.e15643
19. Fields AC, Welten VM, Lu P, Goldberg JE, Irani J, Bleday R, et al. Does race impact survival for patients with anal squamous cell carcinoma? J Surg Oncol. (2019) 120:1201–7. doi: 10.1002/jso.25712
20. Gabriel E, Attwood K, Al-Sukhni E, Erwin D, Boland P, Nurkin S. Age-related rates of colorectal cancer and the factors associated with overall survival. J Gastrointest Oncol. (2018) 9:96–110. doi: 10.21037/jgo.2017.11.13
21. Goyal H, Desai R, Aloysius MM, Jecmenica M, Enders GH, Bansal P. Young-onset colorectal cancer: hospitalization trends and gender disparities in the United States 2010-2014. Int J Colorectal Dis. (2019) 34:1611–5. doi: 10.1007/s00384-019-03360-9
22. Hayes L, Forrest L, Adams J, Hidajat M, Ben-Shlomo Y, White M, et al. Age-related inequalities in colon cancer treatment persist over time: a population-based analysis. J Epidemiol Commun Health. (2019) 73:34–41. doi: 10.1136/jech-2018-210842
23. Koblinski J, Jandova J, Pandit V, Omesiete P, Nfonsam V. Disparities in colon and rectal cancer queried individually between Hispanics and Whites. J Gastroint Oncol. (2019) 10:632–40. doi: 10.21037/jgo.2019.02.08
24. Kurani SS, McCoy RG, Lampman MA, Doubeni CA, Finney Rutten LJ, Inselman JW, et al. Association of neighborhood measures of social determinants of health with breast, cervical, and colorectal cancer screening rates in the US midwest. JAMA Netw Open. (2020) 3:e200618. doi: 10.1001/jamanetworkopen.2020.0618
25. Lu PW, Fields AC, Yoo J, Irani J, Goldberg JE, Bleday R, et al. Sociodemographic predictors of surgery refusal in patients with stage I-III colon cancer. J Surg Oncol. (2020) 121:1306–13. doi: 10.1002/jso.25917
26. Melkonian SC, Jim MA, Haverkamp D, Wiggins CL, McCollum J, White MC, et al. Disparities in cancer incidence and trends among American Indians and Alaska Natives in the United States, 2010-2015. Cancer Epidemiol Biomarkers Prev. (2019) 28:1604–11. doi: 10.1158/1055-9965.EPI-19-0288
27. Nuche-Berenguer B, Sakellariou D. Socioeconomic determinants of cancer screening utilisation in Latin America: a systematic review. PLoS ONE. (2019) 14:e0225667. doi: 10.1371/journal.pone.0225667
28. Robles A, Bashashati M, Contreras A, Chavez LO, Cerro-Rondon AD, Cu C, et al. Colorectal cancer in Hispanics living near the U.S.-Mexico border. Revista Invest Clin. (2019) 71:306–10. doi: 10.24875/RIC.19003026
29. Wu J, Ye J, Wu W, Fang D, Wang K, Yang L, et al. Racial disparities in young-onset patients with colorectal, breast and testicular cancer. J Cancer. (2019) 10:5388–96. doi: 10.7150/jca.32435
30. Zerhouni YA, Trinh QD, Lipsitz S, Goldberg J, Irani J, Bleday R, et al. Effect of medicaid expansion on colorectal cancer screening rates. Dis Colon Rectum. (2019) 62:97–103. doi: 10.1097/DCR.0000000000001260
31. Zhang Q, Wang Y, Hu H, Huang R, Xie L, Liu E, et al. Impact of socioeconomic status on survival of colorectal cancer patients. Oncotarget. (2017) 8:106121. doi: 10.18632/oncotarget.20859
32. National Cancer Institute Surveillance, Epidemiology, and End Results Program. SEER Cancer Statistics Review, 1975-2011. (n.d.). Available online at: https://seer.cancer.gov/archive/csr/1975_2011/
33. United States Preventive Services Task Force. Final Recommendation Statement: Colorectal Cancer Screening 2016. (2016). Available online at: https://www.uspreventiveservicestaskforce.org/uspstf/recommendation/colorectal-cancer-screening
34. National Cancer Institute Surveillance, Epidemiology, and End Results Program. Race Recode Changes. (n.d.). Available online at https://seer.cancer.gov/seerstat/variables/seer/race_ethnicity/
35. North American Association of Central Cancer Registries. NAACCR Race and Ethnicity Work Group. NAACCR Guideline for Enhancing Hispanic/Latino Identification: Revised NAACCR Hispanic/Latino Identification Algorithm [NHIA v2.2.1]. Springfield (IL): North American Association of Central Cancer Registries. September 2011. (2011). Available online at: https://www.naaccr.org/wp-content/uploads/2016/11/NHIA_v2_2_1_09122011.pdf
36. Nylund KL, Asparouhov T, Muthén BO. Deciding on the number of classes in latent class analysis and growth mixture modeling: a Monte Carlo simulation study. Struct Equation Model. (2007) 14:535–69. doi: 10.1080/10705510701575396
37. Aizer AA, Chen M-H, McCarthy EP, Mendu ML, Koo S, Wilhite TJ, et al. Marital status and survival in patients with cancer. J Clin Oncol. (2013) 31:3869. doi: 10.1200/JCO.2013.49.6489
38. Jin J-J, Wang W, Dai F-X, Long Z-W, Cai H, Liu X-W, et al. Marital status and survival in patients with gastric cancer. Cancer Med. (2016) 5:1821–9. doi: 10.1002/cam4.758
39. Johansen C, Schou G, Soll-Johanning H, Mellemgaard A, Lynge E. Influence of marital status on survival from colon and rectal cancer in Denmark. BJC. (1996) 74:985–8. doi: 10.1038/bjc.1996.470
40. Li Q, Gan L, Liang L, Li X, Cai S. The influence of marital status on stage at diagnosis and survival of patients with colorectal cancer. Oncotarget. (2015) 6:7339. doi: 10.18632/oncotarget.3129
41. Wang L, Wilson SE, Stewart DB, Hollenbeak CS. Marital status and colon cancer outcomes in US Surveillance, Epidemiology and End Results registries: does marriage affect cancer survival by gender and stage? Cancer Epidemiol. (2011) 35:417–22. doi: 10.1016/j.canep.2011.02.004
42. Goldzweig G, Andritsch E, Hubert A, Brenner B, Walach N, Perry S, et al. Psychological distress among male patients and male spouses: what do oncologists need to know? Ann Oncol. (2010) 21:877–83. doi: 10.1093/annonc/mdp398
43. Jin Shin K, Jin Lee Y, Ryoul Yang Y, Park S, Suh P-G, Yung Follo M, et al. Molecular mechanisms underlying psychological stress and cancer. Curr Pharm Des. (2016) 22:2389–402. doi: 10.2174/1381612822666160226144025
44. Thong MSY, Kaptein AA, Krediet RT, Boeschoten EW, Dekker FW, for the Netherlands Cooperative Study on the Adequacy of Dialysis (NECOSAD) Study Group. Social support predicts survival in dialysis patients. Nephrol Dial Transpl. (2006) 22:845–50. doi: 10.1093/ndt/gfl700
45. Gonzalez-Pons M, Torres M, Perez J, Velez A, Betancourt JP, Marcano L, et al. Colorectal cancer survival disparities among puerto rican hispanics: a comparison to racial/ethnic groups in the United States. Cancer Clin Oncol. (2016) 5:29–37. doi: 10.5539/cco.v5n2p29
46. Siegel RL, Fedewa SA, Anderson WF, Miller KD, Ma J, Rosenberg PS, et al. Colorectal cancer incidence patterns in the United States, 1974–2013. J Natl Cancer Inst. (2017) 109:djw322. doi: 10.1093/jnci/djw322
47. Loomans-Kropp HA, Umar A. Increasing incidence of colorectal cancer in young adults. J Cancer Epidemiol. (2019) 2019:9841295. doi: 10.1155/2019/9841295
48. Ohri A, Robinson A, Liu B, Bhuket T, Wong R. Updated assessment of colorectal cancer incidence in the U.S. by age, sex, and race/ethnicity. Dig Dis Sci. (2019) 65:1838–49. doi: 10.1007/s10620-019-05913-y
49. Dominitz JA, Samsa GP, Landsman P, Provenzale D. Race, treatment, and survival among colorectal carcinoma patients in an equal-access medical system. Cancer. (1998) 82:2312–20. doi: 10.1002/(SICI)1097-0142(19980615)82:12<2312::AID-CNCR3>3.0.CO;2-U
50. Gray KD, Ballard BR, Washington MK, Belue R, Grady WM, Chapman WC, et al. Do adverse histopathologic findings in colorectal cancer patients explain disparate outcomes? J Natl Med Assoc. (2006) 98:348.
51. Montiel Ishino FA, Odame EA, Villalobos K, Whiteside MA, Mamudu H, Williams F. Applying latent class analysis on cancer registry data to identify and compare health disparity profiles in colorectal cancer surgical treatment delay. J Public Health Manag Pract. (in press).
52. Braveman P, Gottlieb L. The social determinants of health: it's time to consider the causes of the causes. Public Health Rep. (2014) 129(Suppl. 2):19–31. doi: 10.1177/00333549141291S206
53. Williams F, Colditz GA, Hovmand P, Gehlert S. Combining community-engaged research with group model building to address racial disparities in breast cancer mortality and treatment. J Health Disparities Res Pract. (2018) 11:160–78.
54. National Cancer Institute Surveillance E, and End Results Program. Surveillance, Epidemiology, and End Results Program 2020. Available online at: https://seer.cancer.gov/registries/.
Keywords: colorectal (colon) cancer, cancer health disparities, latent class analyses, survivorship (public health), person-centered analysis
Citation: Montiel Ishino FA, Odame EA, Villalobos K, Liu X, Salmeron B, Mamudu H and Williams F (2021) A National Study of Colorectal Cancer Survivorship Disparities: A Latent Class Analysis Using SEER (Surveillance, Epidemiology, and End Results) Registries. Front. Public Health 9:628022. doi: 10.3389/fpubh.2021.628022
Received: 10 November 2020; Accepted: 27 January 2021;
Published: 25 February 2021.
Edited by:
Tung-Sung Tseng, Louisiana State University, United StatesReviewed by:
Shahinaz Gadalla, Division of Cancer Epidemiology and Genetics (NCI), United StatesKaylin Beiter, Louisiana State University Health Sciences Center, United States
Copyright © 2021 Montiel Ishino, Odame, Villalobos, Liu, Salmeron, Mamudu and Williams. This is an open-access article distributed under the terms of the Creative Commons Attribution License (CC BY). The use, distribution or reproduction in other forums is permitted, provided the original author(s) and the copyright owner(s) are credited and that the original publication in this journal is cited, in accordance with accepted academic practice. No use, distribution or reproduction is permitted which does not comply with these terms.
*Correspondence: Francisco A. Montiel Ishino, ZnJhbmNpc2NvLm1vbnRpZWxpc2hpbm9AbmloLmdvdg==