- 1Zhejiang Provincial People's Hospital, Hangzhou, China
- 2Zhongnan Hospital, Wuhan University, Wuhan, China
- 3Fred Hutchinson Cancer Research Center, Seattle, WA, United States
- 4Gennlife (Beijing) Technology Co. Ltd., Beijing, China
Background: The COVID-19 global pandemic has posed unprecedented challenges to health care systems all over the world. The speed of the viral spread results in a tsunami of patients, which begs for a reliable screening tool using readily available data to predict disease progression.
Methods: Multicenter retrospective cohort study was performed to develop and validate a triage model. Patient demographic and non-laboratory clinical data were recorded. Using only the data from Zhongnan Hospital, step-wise multivariable logistic regression was performed, and a prognostic nomogram was constructed based on the independent variables identifies. The discrimination and calibration of the model were validated. External independent validation was performed to further address the utility of this model using data from Jinyintan Hospital.
Results: A total of 716 confirmed COVID-19 cases from Zhongnan Hospital were included for model construction. Men, increased age, fever, hypertension, cardio-cerebrovascular disease, dyspnea, cough, and myalgia are independent risk factors for disease progression. External independent validation was carried out in a cohort with 201 cases from Jinyintan Hospital. The area under the curve (AUC) was 0.787 (95% confidence interval [CI]: 0.747–0.827) in the training group and 0.704 (95% CI: 0.632–0.777) in the validation group.
Conclusions: We developed a novel triage model based on basic and clinical data. Our model could be used as a pragmatic screening aid to allow for cost efficient screening to be carried out such as over the phone, which may reduce disease propagation through limiting unnecessary contact. This may help allocation of limited medical resources.
Introduction
In December 2019, Chinese and World Health Organization (WHO) health experts identified a growing number of pneumonia of unknown cause cases leading to substantial health issues for many citizens located in Wuhan, China (1, 2). Identified as a virus, infection caused severe respiratory syndromes and commonly used treatments were often ineffective. Today, we now know the cause to be a novel coronavirus known as the severe acute respiratory syndrome coronavirus 2 (SARS-CoV-2), or COVID-19.
COVID-19 is the latest threat to global health. On March 11, 2020, WHO declared COVID-19 to be a global pandemic, as infection cases were reported in at least 114 countries (3). As of March 15, 2021, a total of more than 119,452,269 cases and 2,647,662 deaths were confirmed worldwide and the number of new cases was expected to increase (4). The high number of COVID-19 cases has overwhelmed health systems globally.
As health system resources are limited—even in highly developed countries—it is crucial to conduct clinical research to determine the best utilization of resources. For example, due to the limited number of medical professionals and equipment (e.g., N95 masks and respirators) available at such short notice, it is almost impossible to provide meticulous, high resource (e.g., intensive care level) health care for every single case of COVID-19 infection. However, this may be acceptable given the fact that initial epidemiologic studies demonstrated that most of COVID-19 cases were classified as mild (81% with non-pneumonia or mild pneumonia) and did not require intensive medical care (5). However, this important finding can only help if we can prioritize resources to those who need it most. Thus, it is important for front-line medical professionals to have a reliable and technically easy way to differentiate those at higher risk for severe and critical symptoms from those at lower risk.
Given that health care systems globally are overwhelmed with the exponential growth of COVID-19 cases and health care resources are limited, it is of extraordinary importance to allocate medical resources effectively and fairly (6). Decisions on resource allocation must be able to be made right at initial patient presentation in order to optimize resource use up front. Thus, it is of notable value to develop a triage model using only patient characteristics and clinical data (i.e., data readily available to medical professionals without the need for additional resources, such as laboratory, and/or imaging technology). In the present study, we create and validate such a novel triage model based on patient data from Chinese COVID-19 epicenter.
Methods
Data Sources
This retrospective cohort study was approved by Research Ethics Commission of Zhongnan Hospital of Wuhan University (2020032), Jinyintan Hospital (KY-2020-50.01), and Zhejiang Provincial People's Hospital (2020QT068). The requirement of informed consent was waived due to its retrospective design. On March 10, 2020, all medical records of inpatients diagnosed with COVID-19 in Zhongnan Hospital of Wuhan University and Jinyintan Hospital, Wuhan, China were reviewed. Epidemiological, demographic, clinical symptoms, signs, and comorbidities information were extracted from electronic medical records. All data were examined by two of the listed authors (LNS and ZJ) independently to ensure accuracy.
Definitions
For this study, the severity of COVID-19 infection was defined according to the World Health Organization (WHO) interim guidance (7). Mild type infection is defined as cases where patients have non-pneumonia or mild pneumonia (5). Severe type infection is diagnosed when at least one of the following three diagnostic criteria is met: (1) respiratory distress (RR ≥ 30/min); (2) resting blood oxygen saturation = < 93%; or (3) arterial blood oxygen partial pressure (PaO2)/FiO2 = <300 mmHg. Critical type is diagnosed when at least one of the following three diagnostic criteria is met: (1) respiratory failure needing mechanical oxygenation; (2) shock; or (3) development of other organ failure, requiring intensive care unit (ICU) care. Fever was defined as axillary temperature of at least 37.3°C. Using these criteria, patient cases were divided into two groups: (1) mild, which could be treated via isolation at home or at the temporary hospital; and (2) severe/critical, which should be admitted for inpatient care within a hospital with full resources/equipment as soon as possible.
Statistical Analysis
Baseline characteristics of the patients of two groups (mild and severe/critical) were described using counts and percentages for categorical variables and medians with interquartile ranges (IQR) for continuous variables. Differences between groups were tested using the χ2 test or Fisher's exact test for categorical variables and t test or Wilcoxon test for continuous variables, depending on the nature of the distribution. No imputation was made for missing data. To explore factors associated with severe/critical COVID-19 infection, univariate and multivariate logistic regression models were performed. Independent variables with p < 0.05 in univariate analyses were entered in a multivariate model, in which the predictors with p < 0.05 were further selected in the final multivariate logistic regression model. Sex and age were selected for all multivariate models for effect-adjustment purpose.
A nomogram was developed based on the final model. Internal and independent validations were implemented to evaluate the predictive performance of the derived nomogram, in terms of discrimination and calibration. For internal validation, we used bootstrap resampling with 1,000 samples to compute bias-corrected estimates. For independent validation, to account for potential discrepancy between the model-development dataset and independent-validation dataset, calibration plots were created for the original and recalibrated nomogram, with recalibration based on the intercept and slope framework as originally proposed by D.R. COX (8). Discrimination was assessed by the receiver operating characteristic (ROC) curve and area under the curve (AUC). Calibration was assessed by comparing observed severe/critical COVID-19 rates with predictions from the final model. All statistical analyses were conducted by one of the authors listed as (JYZ), who works as an independent statistician and was not involved in patient care. All statistical analyses were performed using R software, version 3.6.2 (R Foundation for Statistical Computing), and a two-sided α of <0.05 was considered statistically significant for all tests.
Results
Demographic Data and Symptoms
A total of 1,181 patients with suspected or confirmed COVID-19 infection were admitted to Zhongnan Hospital of Wuhan University between December 30, 2019 and March 10, 2020. Among these patients, 406 patients (34%) were excluded because they were assumed positive for COVID-19 infection based only on clinical symptoms and/or CT scan prior to testing kits becoming readily available (performed in Hubei Province only in certain period of time). Another 59 cases (5%) were excluded because of missing clinical data in electronic medical records. Ultimately, a final sample of 716 patients (61%) with confirmed COVID-19 infection was included as a training data set. Same set of data of a cohort of 201 patients with COVID-19 from Jinyintan Hospital was included for independent validation.
The basic epidemiological, demographic, clinical characteristics for the training set (medium age 55, 46.9% male) and the validation set (medium age 63, 49.3% male) were shown in Table 1. In the training set, 161 cases (22.5%) were diagnosed as severe/critical type, while in the validation set, 90 cases (44.8%) were diagnosed as severe/critical. In both set, patients in severe/critical group were significantly older than those in mild group (p < 0.001). Hypertension, diabetes and cardio-cerebrovascular disease were the most common comorbidities (Table 1). The most common symptoms on admission were fever, cough, dyspnea, and myalgia (Table 1).
Independent Risk Factor Identification
Univariate analysis identified that sex, age, presence of fever, current smoker, former smoker, alcohol consumption, hypertension, diabetes mellitus, cardio-cerebrovascular disease, dyspnea, cough, and myalgia were significantly associated with progression of COVID-19 from mild to severe/critical (Table 2).
Using the results of the univariate analysis, a multivariate logistic regression model was developed, which identified that sex (man), increased age, presence of fever, current smoker, hypertension, cardio-cerebrovascular disease, dyspnea, cough, and myalgia were independently associated with increased odds of progression of COVID-19 disease from mild to severe/critical. Woman sex was the only characteristic associated with decreased risk of disease progression (Table 2).
Nomogram Development
The probability of progressing from the mild to severe/critical group was assessed based on the results of the final multivariate logistic regression. The final multivariate logistic regression model for constructing the nomogram can be expressed as + 1.41 cardio − cerebrovascular disease + 0.81 dyspnea + 0.54 cough + 0.68 myalgia where Psevere/critical denotes the probability for a patient with COVID-19 to progress to severe/critical COVID-19.
Nomogram Construction and Validation
A prognostic nomogram for early recognition of those cases that would likely progress to severe/critical cases was constructed using the multivariate logistic regression results. Points were assigned to the identified factors according to the absolute maximum beta value based on the logistic regression model, given that the units are different for the continuous (age) and categorical predictors (sex, fever, smoke, hypertension, cardio-cerebrovascular disease, dyspnea, cough, and myalgia). Though with the smallest beta coefficient of 0.03, the calculated absolute maximum beta value (Beta × value range of the predictor) of age is 0.03 × 89 = 2.67, which means that it has the greatest impact on the probability of the event compared with the other seven predictors (Figure 1). As shown in the nomogram, patients with the following characteristics were more likely to progress to the severe/critical group: sex (man), older in age, presence of fever, current smoker, hypertension, cardio-cerebrovascular disease, dyspnea, cough, and myalgia. Summing all points led to a total score. Locating the total score on the nomogram scale, the risk of progressing to the severe/critical group could be determined at patient presentation.
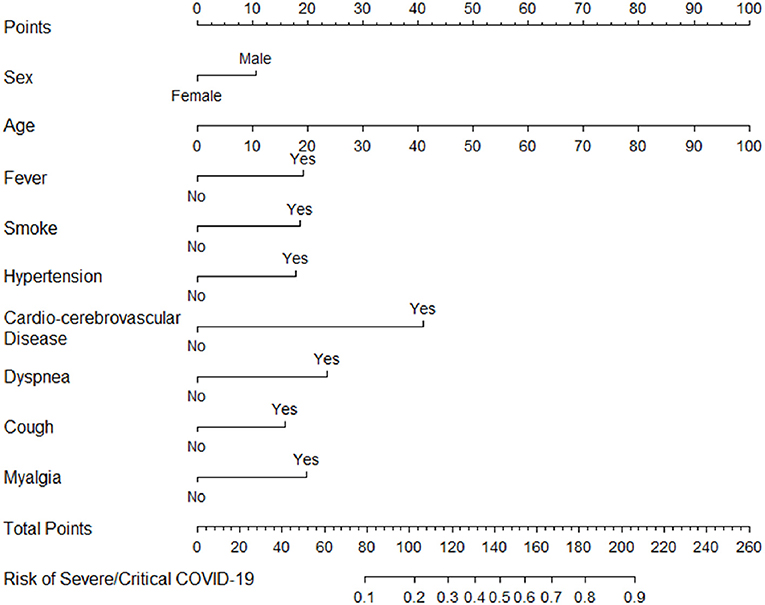
Figure 1. Nomogram of probability to develop severe/critical COVID-19. To use the nomogram, draw an upward vertical line from each covariate to the points bar to calculate the number of points. Based on the sum of the covariate points, draw a downward vertical line from the total points line to calculate the probability of developing severe/critical COVID-19.
Internal and External Independent Validation
To evaluate the discrimination of the model and to reduce overfitting bias, internal validation was performed using a bootstrapping technique with 1,000 resamples as qualified. Figure 2 showed the internal validation of the nomogram using a receiver operating characteristic (ROC) curve with an area under the curve (AUC) of 0.787 (95% confidence interval [CI]: 0.747–0.827). We performed external independent validation of our nomogram as well, which demonstrated as AUC of 0.704 (95% CI: 0.632–0.777). The calibration curve showed excellent accordance between the nomogram prediction and the actual observation of severe/critical cases of COVID-19 (Figure 3). An external calibration plot for Jinyintan dataset based on the original nomogram and on the recalibrated nomogram is shown in Figure 4.
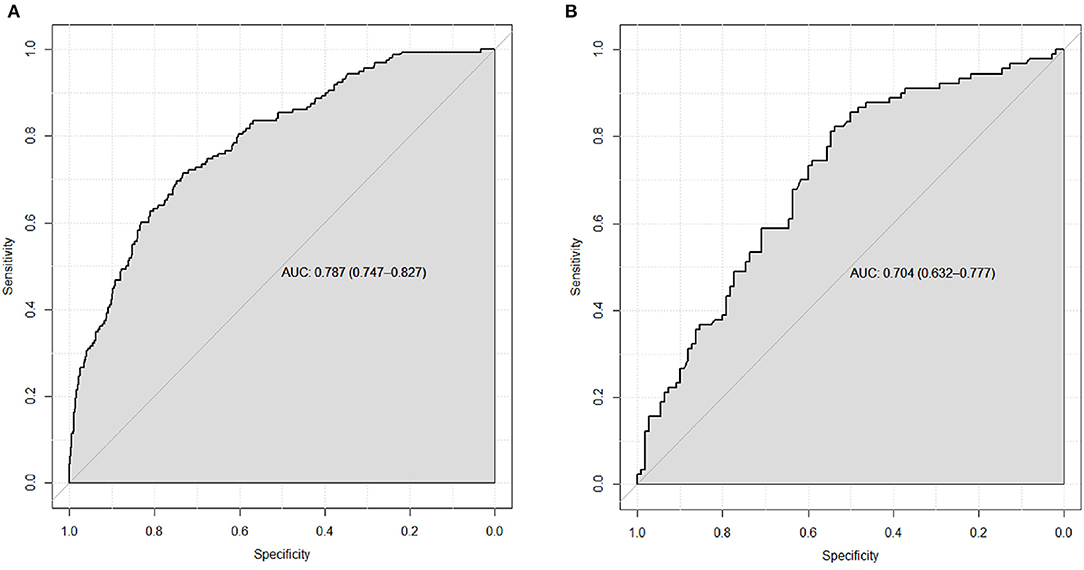
Figure 2. (A) ROC curve for the nomogram based on the full Zhongnan Hospital dataset. The bias-corrected AUC is 0.772 based on internal validation using bootstrap resampling (1,000 patients) (B) ROC curve from an external, independent validation using the Jinyintan Hospital dataset. The estimate of AUC and its 95% confidence interval are shown in the plots. Key: ROC, receiver operating characteristic. AUC, area under the curve.
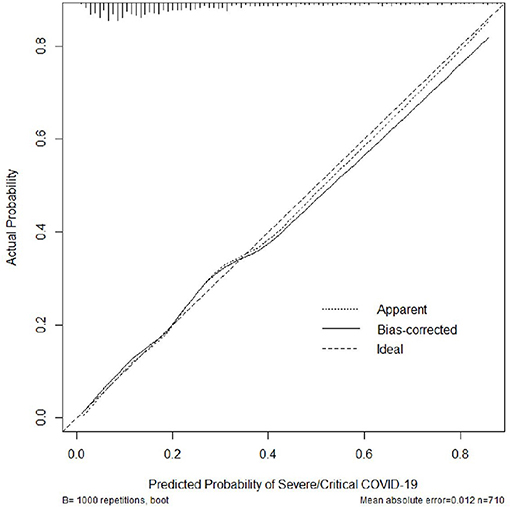
Figure 3. Calibration plot for the nomogram. The bias-corrected (overfitting-corrected) estimates of predicted vs. observed values were obtained based on bootstrap resampling with 1,000 samples for internal validation purpose.
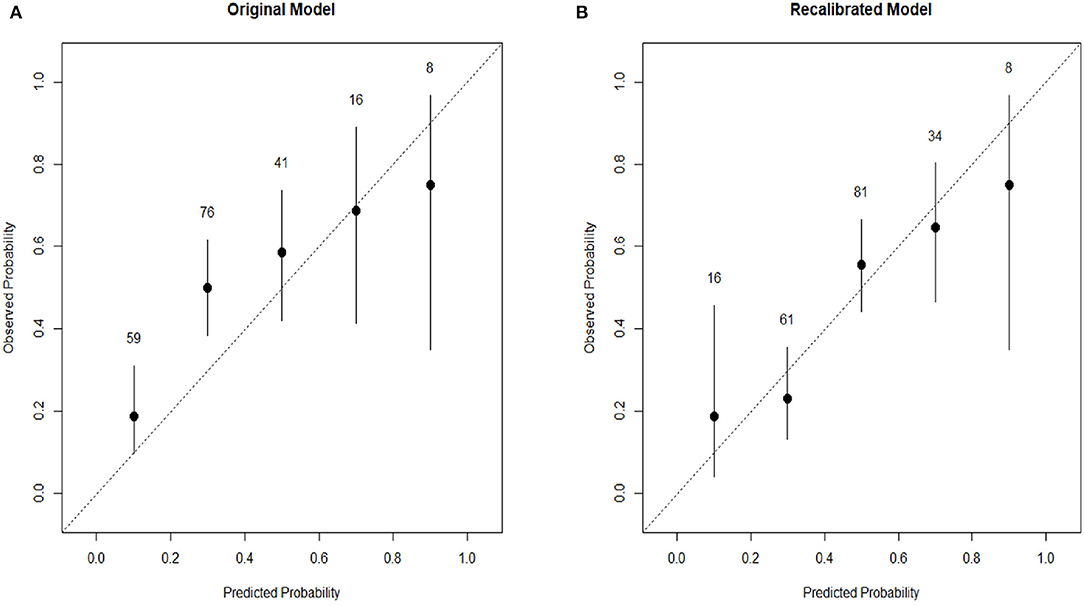
Figure 4. (A) External calibration plot for Jinyintan dataset based on the original nomogram. (B) External calibration plot for Jinyintan dataset based on the recalibrated nomogram. The nomogram was recalibrated by the intercept and slope framework as originally proposed by D.R. Cox.8 The plots are grouped into five bins based on their predicted probabilities, and then the bin prevalence (the ratio of plots in this bin with observed number of severe/critical COVID-19 vs. the total number of plots in this bin) is calculated for each bin. The confidence interval for each bin is also plotted, and the total number of plots is labeled above each the bin. Confidence intervals are calculated for the binomial bin counts using the F distribution.
Discussion
The COVID-19 global pandemic has caused great strain on the world's economies and health systems. Without a vaccine or therapeutic available, the number of confirmed cases continues to rise in many areas with many patients requiring hospitalization and a great deal of health care resources. However, health care resources are limited and optimizing their use is critical to successfully tackling this pandemic. In this present study, patient and clinical (non-laboratory) data on 917 patients from two different hospitals, Zhongnan Hospital of Wuhan University and Jinyintan Hospital, in Wuhan, China with confirmed COVID-19 infection were retrospectively reviewed. Step-wise multivariate logistic regression was used to identify risk factors for progression from mild to severe/critical disease. This information was utilized to produce a nomogram predictive model. Men, older in age, presence of fever, current smoker, hypertension, cardio-cerebrovascular disease, dyspnea, cough, and myalgia were all characteristics associated with higher risk for disease progression. Woman sex was the only protective factor. This information can help medical professionals and governments maximize the use of their medical resources by prioritizing patients with greater odds of progressing to severe/critical disease.
Given COVID-19 is a novel coronavirus that was only identified in December 2019, there is an overall paucity of literature to date. However, of the limited prior research, one previous study indicated that older age was an important independent variable associated with mortality in critical COVID-19 patients (9). While our study did not directly examine mortality, our research identified the importance of older age as a variable associated with the progression of COVID-19 disease from mild to severe/critical. The underlying mechanism causing age-related issues could be an age-dependent deficiency in B-cell and T-cell function and the dysfunction of viral elimination due to the excess production of type 2 cytokines, leading to prolonged pro-inflammatory responses (10).
In addition to age, sex is an important factor to consider. The limited literature to date reported that men account for a high proportion of COVID-19 cases, ranging from 58 to 67% (9, 11–13). One hypothesis as to why the literature from China suggests this disease predilection for men is that majority workers in Huanan Seafood Wholesale Market, where the disease appears to have originated, were men (14, 15). Intriguingly, our sample was nearly split evenly by sex. However, our results indicate that men with COVID-19 infection have higher risk for disease progression. While more severe disease in men is consistent with media reports, this is the first study, to our knowledge, that confirms this finding scientifically. However, additional research from other pandemic epicenters is warranted to further evaluate the impact of sex on disease progression and mortality (16).
Presenting clinical symptoms are also crucial elements of initial evaluation of patients with COVID-19 infection. One of the most common presenting symptoms of COVID-19 infection is fever (5, 14, 17). In our study, we found that 67.5% of the cases had fever at presentation. The impact of fever on poor clinical outcomes could be associated with IL-6, which is generally known as a strong pro-inflammatory cytokine and highly expressed in non-survivor groups with severe/critical disease in previous studies (9, 18). The other three symptoms that were independently associated with increased risk of disease progression were dyspnea, cough, and myalgia. Because of the impact of COVID-19 infection on the respiratory system, these were not unexpected finding; however, it remains important for front-line medical professions to consider these specific symptoms as alarming risk factors when treating patients who initially present for care with COVID-19 infection.
Comorbidities are also important to consider when evaluating risk factors for disease progression. Among all of the comorbidities analyzed, hypertension and cardio-cerebrovascular disease were associated with disease progression. Our results showed a significantly higher proportion of patients with hypertension in the severe/critical group than in the mild group (43.1 vs. 18.6%, p < 0.01). Hypertension was identified as a risk factor for disease progression, which is partially consistent with previous studies (9, 12, 14). Cardio-cerebrovascular disease was also significantly associated with higher risk of disease progression in our model. Despite its low incidence (7.4% in training cohort and 10.4% in validation cohort), cardio-cerebrovascular disease is of notable concern and medical professionals should be aware of such a diagnosis. Cardio-cerebrovascular disease is a well-known risk factor due to its strong association with all-cause dementia and depression and all-cause mortality (19–21). Our previous study also found that the cases with COVID-19 who was transferred to ICU had a higher proportion of cardio-cerebrovascular disease comorbidity (14).
There are several limitations of our study. First, our analysis included patients from only one country; therefore, the generalizability of our findings to other areas of the world is unknown. However, our findings scientifically verify many of the global media reports and can be considered by public health officials making resource utilization decisions. Second, we included all patients with confirmed COVID-19 infection at their time of initial presentation; however, we did not account for any difference in the duration of symptoms prior to presentation. Because all were aware of this concerning disease, we suspect many did not present with delay. Further, by including all who presented for care, we feel selection biased was reduced. Third, we did not include results from any laboratory or radiographic tests. Such information, could potentially provide additional insight as to factors associated with disease progression. However, our model provides an efficient and easy approach to triaging patients at initial presentation based strictly on patient characteristics, comorbidities, and symptoms. Further, this type of approach is of value in areas where medical supplies and resources are of substantial shortage. Lastly, due to our limited sample size and retrospective cohort study design, we believe a prospective, randomized clinical trial with larger sample size would be helpful to confirm our findings and/or validate new findings. However, given the overwhelming nature of this global pandemic, such a study design may be challenging to perform, especially as new and experimental interventions are being introduced nearly daily. Our study demonstrates the natural disease process for those not undergoing experimental therapeutic intervention.
Overall, we determined which factors are associated with progression of COVID-19 infection from mild to severe/critical. Based on these results, a validated nomogram was developed to help triage patients at presentation and then externally validated. We believe our study findings could be applied in outpatient clinic or emergency department settings to better triage the growing number of newly confirmed COVID-19 cases during this global pandemic. This could help optimize resource utilization within health care systems globally, which is critical at this time of concerned shortages.
Data Availability Statement
The raw data supporting the conclusions of this article will be made available by the authors, without undue reservation.
Ethics Statement
This retrospective cohort study was approved by Research Ethics Commission of Zhongnan Hospital of Wuhan University (2020032), Jinyintan Hospital (KY-2020-50.01), and Zhejiang Provincial People's Hospital (2020QT068). The requirement of informed consent was waived due to its retrospective design.
Author Contributions
YT, XZ, LS, JZhe, JZha, HH, YZ, and MG conceived of the presented idea. JWa, MW, JWu, JZhu, RY, YJ, LC, DZ, HW, SC, RL, and JL collected clinical data for this study. JZhe, YW, and WT performed statistical analysis. JZha, HH, YZ, and MG encouraged YT, XZ, LS, and JZhe to investigate and supervised the findings of this work. All authors discussed the results and contributed to the final manuscript.
Funding
This study was supported by grants from the National Natural Science Foundation of China (81900097 to XZ), the Emergency Response Project of Hubei Science and Technology Department (2020FCA023 to YZ), the Emergency Diagnostic and Therapeutic Center of Central China (to XZ and YZ), the Zhejiang Provincial Health Bureau Science Foundation of China (2018KY017 to JZha and 2020382745 to JZha), the Scientific Research Fund of the National Health Commission of China (No. WKJ-ZJ-2102 to MHG), the General Scientific Research Projects of Zhejiang Education Department (No. Y202044644 to HJH), and the General Scientific Research Projects of Zhejiang Education Department (No. Y202044571 to JZ and No. Y202044502 to JWa).
Conflict of Interest
The authors declare that the research was conducted in the absence of any commercial or financial relationships that could be construed as a potential conflict of interest.
References
1. Pneumonia of unknown cause - China: disease outbreak news. Geneva: World Health Organization (2020). Available online at: https://www.npr.org/sections/goatsandsoda/2020/03/11/814474930/coronavirus-covid-19-is-now-officially-a-pandemic-who-says (accessed at: March 20, 2020).
2. Zhu N, Zhang D, Wang W, Li X, Yang B, Song J, et al. A novel coronavirus from patients with pneumonia in China, 2019. N Engl J Med. (2020) 382:727–33. doi: 10.1056/NEJMoa2001017
3. Coronavirus: COVID-19 is now officially a pandemic WHO says. (2020). Available online at: https://www.who.int/csr/don/05-january-2020-pneumonia-of-unkown-cause-china/en/ (accessed at: March 20, 2020).
4. World Health Organization. Diseases/Coronavirus Disease (COVID-19): Coronavirus Disease (COVID-19) Pandemic. Available online at: http://www.who.int/emergencies/diseases/novel-coronavirus-2019 (accessed at: March 15, 2021).
5. Wu Z, McGoogan JM. Characteristics of and important lessons from the coronavirus disease 2019 (COVID-19) outbreak in china: summary of a report of 72 314 cases from the chinese center for disease control and prevention. JAMA. (2020) 323:1239–42. doi: 10.1001/jama.2020.2648
6. Truog RD, Mitchell C, Daley G. The toughest triage-Allocating ventilators in a pandemic. N Engl J Med. (2020) 382:1973–5. doi: 10.1056/NEJMp2005689
7. World Health Organization. Clinical Management of Severe Acute Respiratory Infection When Novel Coronavirus (2019-nCoV) Infection Is Suspected: Interim Guidance. (2020). Available online at: https://www.who.int/docs/default-source/coronaviruse/clinical-management-of-novel-cov.pdf (accessed at: March 17, 2020).
8. Cox DR. Two further applications of a model for binary regression. Biometrika. (1958) 45:562–5. doi: 10.2307/2333203
9. Zhou F, Yu T, Du R, Fan G, Liu Y, Liu Z, et al. Clinical course and risk factors for mortality of adult inpatients with COVID-19 in Wuhan, China: a retrospective cohort study. Lancet. (2020) 395:1054–62. doi: 10.1016/S0140-6736(20)30566-3
10. Opal SM, Girard TD, Ely EW. The immunopathogenesis of sepsis in elderly patients. Clin Infect Dis. (2005) 41(Suppl 7):S504–12. doi: 10.1086/432007
11. Yang X, Yu Y, Xu J, Shu H, Xia J, Liu H, et al. Clinical course and outcomes of critically ill patients with SARS-CoV-2 pneumonia in Wuhan, China: a single-centered, retrospective, observational study. Lancet Respir Med. (2020) 8:475–81. doi: 10.1016/S2213-2600(20)30079-5
12. Guan W, Ni Z, Hu Y, Liang W, Ou C, He J, et al. Clinical characteristics of coronavirus disease 2019 in China. N Engl J Med. (2020) 382:1708–20. doi: 10.1056/NEJMoa2002032
13. Chen N, Zhou M, Dong X, Wu J, Gong F, Han Y, et al. Epidemiological and clinical characteristics of 99 cases of 2019 novel coronavirus pneumonia in Wuhan, China: a descriptive study. Lancet. (2020) 395:507–13. doi: 10.1016/S0140-6736(20)30211-7
14. Wang D, Hu B, Hu C, Zhu F, Liu X, Zhang J, et al. Clinical characteristics of 138 hospitalized patients with 2019 novel coronavirus-infected pneumonia in Wuhan, China. JAMA. (2020) 323:1061–9. doi: 10.1001/jama.2020.1585
15. Li Q, Guan X, Wu P, Wang X, Zhou L, Tong Y, et al. Early transmission dynamics in Wuhan, China, of novel coronavirus–infected pneumonia. N Engl J Med. (2020) 382:1199–207. doi: 10.1056/NEJMoa2001316
16. Why Is the Coronavirus So Much More Deadly for Men Than For Women? (2020). Available online at: https://www.latimes.com/science/story/2020-03-21/why-is-the-coronavirus-more-deadly-for-men-than-for-women (accessed at: March 27, 2020).
17. Xu X, Wu X, Jiang X, Xu K, Ying L, Ma C, et al. Clinical findings in a group of patients infected with the 2019 novel coronavirus (SARS-Cov-2) outside of Wuhan, China: retrospective case series. BMJ. (2020) 368:m606. doi: 10.1136/bmj.m606
18. Ruan Q, Yang K, Wang W, Jiang L, Song J. Clinical predictors of mortality due to COVID-19 based on an analysis of data of 150 patients from Wuhan, China. Intensive Care Med. (2020) 46:846–8. doi: 10.1007/s00134-020-05991-x
19. Rensma SP, van Sloten TT, Launer LJ, Stehouwer CDA. Cerebral small vessel disease and risk of incident stroke, dementia and depression, and all-cause mortality: a systematic review and meta-analysis. Neurosci Biobehav Rev. (2018) 90:164–73. doi: 10.1016/j.neubiorev.2018.04.003
20. Novo S, Peritore A, Trovato RL. Preclinical atherosclerosis and metabolic syndrome increase cardio- and cerebrovascular events rate: a 20-year follow up. Cardiovasc Diabetol. (2013) 12:155. doi: 10.1186/1475-2480-12-155
Keywords: COVID-19, pandemic, risk factor, nomogram, triage
Citation: Tu Y, Zhou X, Shao L, Zheng J, Wang J, Wang Y, Tong W, Wang M, Wu J, Zhu J, Yan R, Ji Y, Chen L, Zhu D, Wang H, Chen S, Liu R, Lin J, Zhang J, Huang H, Zhao Y and Ge M (2021) Predicting Progression of COVID-19 Infection to Prioritize Medical Resource Allocation: A Novel Triage Model Based on Patient Characteristics and Symptoms at Presentation. Front. Public Health 9:610280. doi: 10.3389/fpubh.2021.610280
Received: 25 September 2020; Accepted: 17 March 2021;
Published: 11 May 2021.
Edited by:
Zisis Kozlakidis, International Agency For Research On Cancer (IARC), FranceCopyright © 2021 Tu, Zhou, Shao, Zheng, Wang, Wang, Tong, Wang, Wu, Zhu, Yan, Ji, Chen, Zhu, Wang, Chen, Liu, Lin, Zhang, Huang, Zhao and Ge. This is an open-access article distributed under the terms of the Creative Commons Attribution License (CC BY). The use, distribution or reproduction in other forums is permitted, provided the original author(s) and the copyright owner(s) are credited and that the original publication in this journal is cited, in accordance with accepted academic practice. No use, distribution or reproduction is permitted which does not comply with these terms.
*Correspondence: Minghua Ge, Z2VtaW5naHVhJiN4MDAwNDA7aG1jLmVkdS5jbg==; Yan Zhao, ZG9jdG9yeWFuemhhbyYjeDAwMDQwO3dodS5lZHUuY24=; Haijun Huang, aHVhbmdoYWlqdW4wODI2JiN4MDAwNDA7MTYzLmNvbQ==; Jun Zhang, c3BpbmV6aGFuZ2p1biYjeDAwMDQwO2FsaXl1bi5jb20=
†These authors have contributed equally to this work