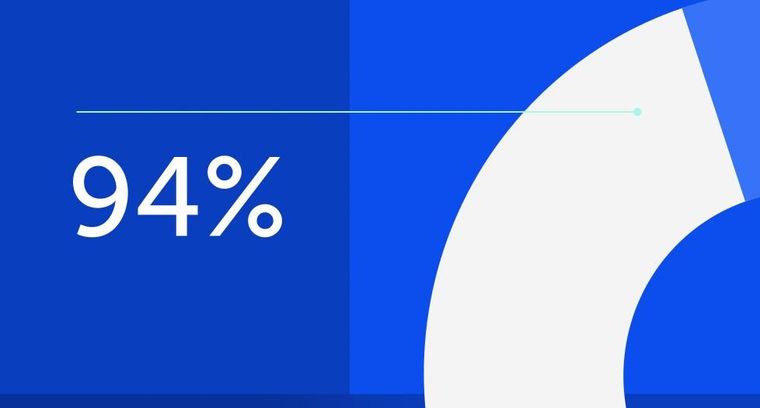
94% of researchers rate our articles as excellent or good
Learn more about the work of our research integrity team to safeguard the quality of each article we publish.
Find out more
BRIEF RESEARCH REPORT article
Front. Public Health, 27 January 2021
Sec. Infectious Diseases – Surveillance, Prevention and Treatment
Volume 8 - 2020 | https://doi.org/10.3389/fpubh.2020.626090
This article is part of the Research TopicModeling Epidemics - Why Are Models Wrong?View all 8 articles
Objective: To investigate the association between socioeconomic deprivation and the persistence of SARS-CoV-2 clusters.
Methods: We analyzed 3,355 SARS-CoV-2 positive test results in the state of Geneva (Switzerland) from February 26 to April 30, 2020. We used a spatiotemporal cluster detection algorithm to monitor SARS-CoV-2 transmission dynamics and defined spatial cluster persistence as the time in days from emergence to disappearance. Using spatial cluster persistence measured outcome and a deprivation index based on neighborhood-level census socioeconomic data, stratified survival functions were estimated using the Kaplan-Meier estimator. Population density adjusted Cox proportional hazards (PH) regression models were then used to examine the association between neighborhood socioeconomic deprivation and persistence of SARS-CoV-2 clusters.
Results: SARS-CoV-2 clusters persisted significantly longer in socioeconomically disadvantaged neighborhoods. In the Cox PH model, the standardized deprivation index was associated with an increased spatial cluster persistence (hazard ratio [HR], 1.43 [95% CI, 1.28–1.59]). The adjusted tercile-specific deprivation index HR was 1.82 [95% CI, 1.56–2.17].
Conclusions: The increased risk of infection of disadvantaged individuals may also be due to the persistence of community transmission. These findings further highlight the need for interventions mitigating inequalities in the risk of SARS-CoV-2 infection and thus, of serious illness and mortality.
There has been an active debate regarding the socioeconomic determinants contributing to the pandemic, several studies highlighting that the SARS-CoV-2 virus disproportionately affects socioeconomically disadvantaged individuals (1–3). Recent evidence has suggested that neighborhood environmental and socioeconomic factors including poor housing quality, overcrowding and inability to work from home may influence SARS-CoV-2 transmission (4). However, the association between neighborhood socioeconomic deprivation and SARS-CoV-2 transmission dynamics remains to be examined.
SARS-CoV-2 spreads via close contact during daily activities which results in geographic clustering of cases (5). The location and duration of persistence of these clusters—monitored using spatiotemporal cluster detection techniques—can provide unique insights into the determinants of transmission (6). We hypothesized that the increased risk of infection within disadvantaged communities is the result of conditions favoring sustained and persistent community transmission. Hence, socioeconomically deprived neighborhoods would have longer-lasting SARS-COV-2 transmission clusters than less deprived neighborhoods. We investigated this hypothesis by combining spatiotemporal cluster detection with cluster survival analysis, an approach similar to the one applied to cancer data by Huang et al. (7).
We analyzed data from 3,355 SARS-CoV-2 RT-PCR positive test results among 17,698 individuals tested in the state of Geneva, Switzerland, covering the first phase of the pandemic (February 26 to April 30, 2020). All included patients were confirmed to be infected with SARS-CoV-2 by RT-PCR assays. The Virology Laboratory at Geneva University Hospitals did the tests and provided anonymized data, including residential addresses. Only individuals who provided a valid residential address and who resided state of Geneva were included.
Residential addresses were geocoded by address matching on the reference dataset of Swiss addresses (www.housing-stat.ch). SARS-CoV-2 transmission patterns were monitored through space and time using the modified space-time density-based spatial clustering of application with noise (6) (MST-DBSCAN) algorithm available in the Python package pysda. The MST-DBSCAN algorithm was run with a maximum spatial distance of 600 m, a minimum time-distance value of 1 day, and a maximum time-distance value of 14 days. We then replicated the analysis using different spatial windows (200, 400, 800, and 1,000 m) and observed similar results. The MST-DBSCAN algorithm is one among various density-based clustering methods to detect disease clusters. This modified version of the spatiotemporal DBSCAN presents the advantage of incorporating the effect of the incubation period. The MST-DBSCAN detects clusters of cases but also identifies the daily evolution type of each cluster (6). We projected the daily evolution type of space-time clusters onto the 2,830 Swiss Areas (SA) neighborhoods (www.microgis.ch) of the state of Geneva. This approach allowed to record the kind of cluster evolution each SA neighborhood underwent each day (i.e., increase, keep, decrease, no cluster). The SA neighborhood was used as it constitutes the smallest spatial unit characterized by aggregated census socioeconomic data in Switzerland.
An index of socioeconomic deprivation was calculated at the SA neighborhood-level using a method developed by Lalloué et al. (8) and used in various studies to analyze environmental and health inequalities. The socioeconomic data characterize the 2,830 SA neighborhoods of the state of Geneva and include data on occupation, education, median income, median rent, unemployment, and nationality. Principal component analysis was used to synthesize the information from these data. To obtain a single index for all neighborhoods, the inertia of the first component was maximized by discarding variables only weakly correlated with the first component and variables contributing lower than the average (8).
We defined spatial cluster persistence as the time in days from emergence to disappearance, and censored clusters remaining on the last day of the study period. Using spatial cluster persistence as the measured outcome, we estimated survival functions stratified by terciles of neighborhood-level socioeconomic deprivation with the Kaplan–Meier estimator. The contribution of the neighborhood-level socioeconomic deprivation to spatial cluster persistence was estimated in a Cox proportional hazards (PH) regression model (7) with robust standard errors. We then estimated the contribution of each individual component of the socioeconomic deprivation index [i.e., median income, foreigners (%), median rent, unemployment (%), occupation and education] as continuous independent variables in a separate Cox PH model (Table 1). Models were adjusted for neighborhood-level population density, and covariates were standardized.
Table 1. Hazard ratios and corresponding 95% confidence intervals for cluster persistence from a multi-variable Cox Proportional Hazards model.
We identified 1,079 spatial clusters over the 65 days study period, which, once projected covered, 1,931 neighborhoods of the state of Geneva (Figure 1). The median neighborhood-level SARS-CoV-2 incidence rate ranged from 0 cases per 100,000 (interquartile range, IQR = 650) in the least deprived tercile to 465 (IQR = 866) in the most deprived tercile. Clusters emerged on average 4 days earlier in the most deprived tercile compared to the moderately deprived and 6 days compared to the least deprived terciles (Supplementary Table 1). The persistence of clusters varied substantially across terciles of the neighborhood-level deprivation index (Figure 2). Two months after the emergence of SARS-CoV-2 clusters, almost 85% of the spatial clusters remained in the most deprived areas, compared to around 70% in the moderately deprived areas and around 30% in the least deprived areas. This trend was confirmed by the Cox PH model adjusted for neighborhood-level population density in which the standardized deprivation index was associated with an increased spatial cluster persistence (hazard ratio, HR = 1.43 [95% confidence interval, CI = 1.28–1.59], P < 0.005) and the adjusted tercile-specific deprivation index HR was 1.82 [95% CI, 1.56–2.17]. Hazard ratios and confidence intervals of the Cox PH model including the individual components of the socioeconomic deprivation index are presented in Table 1. Low median income, low median rent, high percentage of foreigners and high unemployment were associated with spatial cluster persistence to varying, but statistically significant, degrees (Table 1). There was no statistically significant association between spatial cluster persistence and tertiary education and the percentage of actives in the primary sector (Table 1).
Figure 1. Bivariate choropleth map: Relationship between terciles of neighborhood-level deprivation index and of SARS-CoV-2 cluster persistence in the canton of Geneva, Switzerland.
Figure 2. Neighborhood-level socioeconomic deprivation association with SARS-CoV-2 cluster persistence. Kaplan Meier survival estimates of SARS-CoV-2 clusters (600 m spatial window) according to terciles of the socioeconomic deprivation index. The hazard ratio and 95% CI correspond to a deprivation tercile change. The MST-DBSCAN algorithm was run with a maximum spatial distance of 600 m, a minimum time-distance value of 1 day, and a maximum time distance value of 14 days. Similar results were observed using different spatial windows (200, 400, 800, and 1,000 m).
We combined spatiotemporal cluster detection with survival analysis and found that neighborhood-level socioeconomic deprivation was associated with persistent spatial clustering of SARS-CoV-2. This result supports our hypothesis, suggesting that the increased risk of infection of disadvantaged communities may also be due to the persistence of community transmission. This suggestion is of importance, considering that socioeconomically disadvantaged individuals are also at risk of worse COVID-19 outcomes due to a greater burden of obesity and other chronic diseases (9). Moreover, digital COVID-19 public health tools, such as contact tracing apps, have been developed and deployed since the first phase of the pandemic. While data remain scarce, evidence suggests that socioeconomic status is a determinant of attitudes toward these technologies (10). Public health attention and locally tailored interventions are required in socioeconomically disadvantaged communities to prevent the intersectionality of these multiple aspects of disadvantage further compounding the risk of infection, the risk of serious illness and thus, of mortality (3, 11, 12).
The place of infection being unknown, we were not able to differentiate between persistence of spatial clusters driven by an increased local transmission or by an increased importation of cases in the community (e.g., from the workplace) or by a coalescence of both. We cannot exclude that the contact tracing strategy—which consisted in tracing and testing close contacts of positive cases—and socioeconomic variations in testing rate influenced spatial cluster persistence.
These findings bring unique insights into the determinants of transmission and suggest that the increased risk of infection of disadvantaged individuals may also be due to the persistence of community transmission. The persistence of transmission in disadvantaged populations further highlights the pressing need for public health interventions preventing an exacerbation of inequalities in the risk of SARS-CoV-2 infection, of serious illness and thus, of mortality.
The dataset analyzed during the current study is available from the corresponding author upon reasonable request. The dataset could not be made publicly available due to the sensitivity of individual georeferenced SARS-CoV-2 testing data. Requests to access these datasets should be directed to Idris Guessous, aWRyaXMuZ3Vlc3NvdXNAaGN1Z2UuY2g=.
DD performed the data analyses and drafted the manuscript. DD, IG, and SJ conceived the study and completed the manuscript. JS, NV, AA, and SS participated in the design of the study and helped to draft the manuscript. All authors read and approved the final manuscript.
The authors declare that the research was conducted in the absence of any commercial or financial relationships that could be construed as a potential conflict of interest.
The Supplementary Material for this article can be found online at: https://www.frontiersin.org/articles/10.3389/fpubh.2020.626090/full#supplementary-material
1. Wadhera RK, Wadhera P, Gaba P, Figueroa JF, Joynt Maddox KE, Yeh RW, et al. Variation in COVID-19 hospitalizations and deaths across New York City boroughs. JAMA. (2020) 323:2192–5. doi: 10.1001/jama.2020.7197
2. Williamson EJ, Walker AJ, Bhaskaran K, Bacon S, Bates C, Morton CE, et al. Factors associated with COVID-19-related death using OpenSAFELY. Nature. (2020) 584:430–6. doi: 10.1038/s41586-020-2521-4
3. Singu S, Acharya A, Challagundla K, Byrareddy SN. Impact of social determinants of health on the emerging COVID-19 pandemic in the United States. Front Public Heal. (2020) 8:406. doi: 10.3389/fpubh.2020.00406
4. Emeruwa UN, Ona S, Shaman JL, Turitz A, Wright JD, Gyamfi-Bannerman C, et al. Associations between built environment, neighborhood socioeconomic status, and SARS-CoV-2 infection among pregnant women in New York City. JAMA. (2020) 324:390–2. doi: 10.1001/jama.2020.11370
5. De Ridder D, Sandoval J, Vuilleumier N, Stringhini S, Spechbach H, Joost S, et al. Geospatial digital monitoring of COVID-19 cases at high spatiotemporal resolution. Lancet Digit Health. (2020) 2:e393–4. doi: 10.1016/S2589-7500(20)30139-4
6. Kuo F-Y, Wen T-H, Sabel CE. Characterizing diffusion dynamics of disease clustering: a modified space-time DBSCAN (MST-DBSCAN) algorithm. Ann Am Assoc Geogr. (2018) 208:1168–86. doi: 10.1080/24694452.2017.1407630
7. Huang L, Pickle LW, Stinchcomb D, Feuer EJ. Detection of spatial clusters: application to cancer survival as a continuous outcome. Epidemiology. (2007) 18:73–87. doi: 10.1097/01.ede.0000249994.30736.24
8. Lalloué B, Monnez JM, Padilla C, Kihal W, Le Meur N, Zmirou-Navier D, et al. A statistical procedure to create a neighborhood socioeconomic index for health inequalities analysis. Int J Equity Health. (2013) 12:21. doi: 10.1186/1475-9276-12-21
9. Townsend MJ, Kyle TK, Stanford FC. Outcomes of COVID-19: disparities in obesity and by ethnicity/race. Int J Obesity. (2020) 44:1807–9. doi: 10.1038/s41366-020-0635-2
10. Ipsos MORI PA. The Health Foundation COVID-19 Survey A Report of Survey Findings on Public Attitudes Towards a Potential Smartphone App to “Track and Trace” Coronavirus Outbreaks. (2020). Available online at: https://www.health.org.uk/sites/default/files/2020-06/Health-Foundation-polling-contact-tracing-app-May-2020.pdf (accessed October 1, 2020).
11. Morabia A. COVID-19: health as a common good. Am J Public Health. (2020) 110:1111–2. doi: 10.2105/AJPH.2020.305802
Keywords: SARS-CoV-2, COVID-19, socioeconomic inequalities, spatial clustering analysis, cluster persistence, transmission dynamics
Citation: De Ridder D, Sandoval J, Vuilleumier N, Azman AS, Stringhini S, Kaiser L, Joost S and Guessous I (2021) Socioeconomically Disadvantaged Neighborhoods Face Increased Persistence of SARS-CoV-2 Clusters. Front. Public Health 8:626090. doi: 10.3389/fpubh.2020.626090
Received: 04 November 2020; Accepted: 31 December 2020;
Published: 27 January 2021.
Edited by:
Theodore Gyle Lewis, Naval Postgraduate School, United StatesReviewed by:
Qiqing Yu Yu, Binghamton University, United StatesCopyright © 2021 De Ridder, Sandoval, Vuilleumier, Azman, Stringhini, Kaiser, Joost and Guessous. This is an open-access article distributed under the terms of the Creative Commons Attribution License (CC BY). The use, distribution or reproduction in other forums is permitted, provided the original author(s) and the copyright owner(s) are credited and that the original publication in this journal is cited, in accordance with accepted academic practice. No use, distribution or reproduction is permitted which does not comply with these terms.
*Correspondence: David De Ridder, ZGF2aWQuZGVyaWRkZXJAdW5pZ2UuY2g=; Idris Guessous, SWRyaXMuR3Vlc3NvdXNAaGN1Z2UuY2g=
†These authors have contributed equally to this work
Disclaimer: All claims expressed in this article are solely those of the authors and do not necessarily represent those of their affiliated organizations, or those of the publisher, the editors and the reviewers. Any product that may be evaluated in this article or claim that may be made by its manufacturer is not guaranteed or endorsed by the publisher.
Research integrity at Frontiers
Learn more about the work of our research integrity team to safeguard the quality of each article we publish.