- 1Division of Human Genetics, Department of Pediatrics, Cincinnati Children's Hospital Medical Center, University of Cincinnati School of Medicine, Cincinnati, OH, United States
- 2Division of Asthma Research, Department of Pediatrics, Cincinnati Children's Hospital Medical Center, University of Cincinnati School of Medicine, Cincinnati, OH, United States
- 3Department of Rehabilitation, Exercise and Nutrition, Sciences, College of Allied Health Sciences, University of Cincinnati, Cincinnati, OH, United States
Background: Biobanks can accelerate research by providing researchers with samples and data. However, hospital-based recruitment as a source for controls may create bias as who comes to the hospital may be different from the broader population.
Methods: In an effort to broadly improve the quality of research studies and reduce costs and challenges associated with recruitment and sample collection, a group of diverse researchers at Cincinnati Children's Hospital Medical Center led an institution-supported initiative to create a population representative pediatric “Greater Cincinnati Childhood Cohort (GCC).” Participants completed a detailed survey, underwent a brief physician-led physical exam, and provided blood, urine, and hair samples. DNA underwent high-throughput genotyping.
Results: In total, 1,020 children ages 3–18 years living in the 7 county Greater Cincinnati Metropolitan region were recruited. Racial composition of the cohort was 84% non-Hispanic white, 15% non-Hispanic black, and 2% other race or Hispanic. Participants exhibited marked demographic and disease burden differences by race. Overall, the cohort was broadly used resulting in publications, grants and patents; yet, it did not meet the needs of all potential researchers.
Conclusions: Learning from both the strengths and weaknesses, we propose leveraging a community-based participatory research framework for future broad use biobanking efforts.
Introduction
Biobanks allow storage of biological specimens and corresponding data for biomedical research, particularly omics studies (1). While providing convenient access to human samples, sample utility can be variable. Biobanks relying on residual sample may have limited sample types and accompanying data. Further, participants in hospital-based biobanks may not be population representative. These differences introduce possible confounding (2, 3).
Population-based biobanks offer an alternative. Population sampling ensures subjects are recruited from the same source as cases (4). A population-based approach can capture specific racial/ethnic populations that may be underrepresented (5). As racial/ethnic minorities make up nearly 40% of the US population (6), minority inclusion is critical for clinical research generalizability (5, 7), healthcare equity (8, 9), and ancestry/ethnicity-specific analyses (10). Unfortunately, costs and poor participation often precludes such sampling for independent researchers (4).
To address these issues, a group of researchers at Cincinnati Children's Hospital Medical Center (CCHMC) led an initiative supported by institutional funds to create a pediatric population-based representative biobank entitled the “Greater Cincinnati Childhood Cohort (GCC).” The goal of the study was to collect a representative sample of the Greater Cincinnati Metropolitan children for use in clinical and translational studies.
Methods
Development of Institutional Initiative
In 2006, a meeting was held to discuss research projects that might benefit from an institutional cohort gauging interest from faculty involved with numerous rare and common conditions. Based on the broad interest, Cincinnati Children's Research Foundation decided to fund a pediatric biobank for the greater good of its research endeavors.
Sampling Design
The goal was to obtain a population representative sample which would benefit research endeavors. While many sampling designs were considered, recruitment based on race, sex, age, and income strata representative of the Greater Cincinnati area according to the 2000 and 2005 United States (US) Census population estimates was chosen.
Inclusion criteria were as follows: participants were between the ages of 3 and 18 years (prior to 18th birthday) at the time of enrollment living in Greater Cincinnati [Hamilton (OH), Clermont (OH), Butler (OH), Warren (OH), Boone (KY), Kenton (KY), Campbell (KY)]. Parents/guardians provided permission through written informed consent. This consent requested permission for use of the survey and exam data and the samples (including DNA) for ongoing and future studies related to the health and well-being of children. Children aged 11 years or greater provided written assent. Once a child turned 18 years of age, they were sent a letter which would allow them to cease participation. Exclusion criteria included participating in an investigational study in the past thirty days and having a parent reported diagnosis of a genetic syndrome. One participant per family was allowed to participate. Prior to study initiation, the study was presented to and received feedback from our Patient Advisory Council. This cohort was approved by Cincinnati Children's Hospital Medical Center's Institutional Review Board.
Recruitment
Recruitment for the GCC was multi-faceted. First, participants of a previously established early childhood study were invited. Second, flyers were placed on research study notification boards at CCHMC as well as shared with ~20 community organizations including elementary schools (flyers sent home with children), social service agencies, day cares, summer camps, at a church and at health fairs. Our data suggested that the most participants heard about the study from friends of CCHMC patients.
Potential subjects were screened by telephone to ensure eligibility. US Census tract monitoring ensured cohort diversity and representativeness (an equal number of males and females, and ~85% white non-Hispanic, 12% African-American, and 3% Asian, Hispanic and other minorities, which represented the population distribution of the Greater Cincinnati Metropolitan area). Within these racial categories, an equal number of males and females in each of 5 age groupings (age under 6, ages 6 to <9, ages 9 to <12, ages 12 to <15, ages 15 to <18 years) was considered the target enrollment. Parental-reported household income of participants was also tracked to ensure that the biobank reflected the metropolitan area (20% of the participants with household income < $20,000, 25% of the participants with incomes between $20,000 and $39,999, 31% of participants with incomes between $40,000 and $74,999, and 24% of participants with incomes of at least $75,000). Review of population representativeness was carried out bimonthly and marketing was modified to target specific strata.
Study Procedures
Participants were mailed the informed consent document after phone screening. At the study visit, study staff reviewed the informed consent document with the participant and parent/legal guardian. A questionnaire administered by a clinical research coordinator captured information on demographics, family/home environment, medical history, and medication use. A licensed physician performed a brief physical exam.
Height, weight, waist and hip circumferences, blood pressure, and heart rate were collected in duplicate or triplicate if notable differences were observed. Anthropometrics were collected following a protocol used by CCHMC investigators (11). To ensure consistency between repeated measures, the coefficient of variation between the measures for each subject was calculated; most exhibited strong consistency (CV <5%). When CV > 5% among triplicate measures, the two values which yielded the CV <5% were averaged, otherwise the observation was set to missing. Averages were used in the final analyses. Body mass index (BMI) was calculated as weight(kg)/height(m)2; age and sex adjusted percentile and z-scores were determined based on CDC 2000 growth curves (12).
Serum, plasma and whole blood were collected to allow for DNA isolation, RNA isolation, clinical diagnostic and biomarker analysis. Urine and hair were collected and stored in sterile non-contaminating receptacles.
Data Management and Access
Phenotypic data was housed in SQL. Projects which use only de-identified data are reviewed at the Director/co-Director level for scientific merit. Projects which use personal health information or samples are reviewed by a Scientific Advisory Committee, made up of 12 faculty researchers spread across multiple divisions within the Department of Pediatrics. Investigators using the GCC data were expected to consult with the IRB with respect to their specific projects prior to obtaining data.
Data Analysis
The cohort was described using mean ± standard deviation or frequencies. To test whether there were differences in participant characteristics by race, whites and black participants were compared using chi-square goodness of fit tests or t-tests. To determine if the population was representative of the general catchment area, 2010–2012 3 year estimates for the Greater Cincinnati Metropolitan area from the US Census American Communities Survey and national estimates were used (13). GCC publications were entered into SCOPUS to determine article metrics.
Results
Characteristics of the Cohort
Between 2007 and 2011, 1,020 children were enrolled (Figure 1). The cohort was 84% non-Hispanic white, 15% non-Hispanic black, and 2% other race or Hispanic (Table 1). Age and sex were distributed similarly between whites and blacks (p > 0.05). Blacks had lower parental education (p < 0.0001), lower income (p < 0.0001), and lower rates of private insurance (p < 0.0001). Racial distribution of the GCC were similar to Greater Cincinnati Metropolitan area and the United States (Supplementary Figure 1).
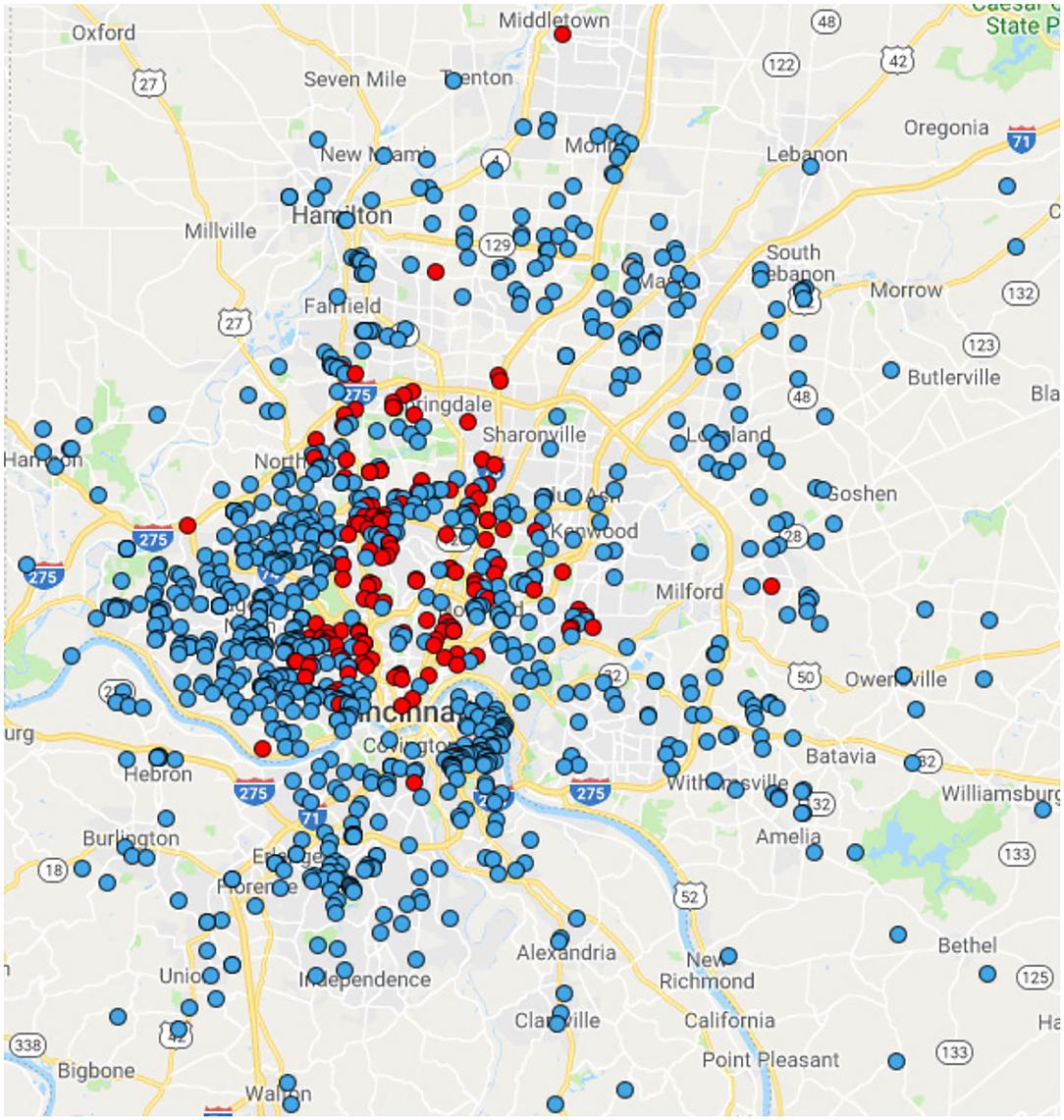
Figure 1. Geographic distribution of the GCC Participants. Blue dots represent individuals who self-report as white. Red dots represent individuals who self-report as black.
Overall, 659 (64.6%) participants reported a medical condition, with the most common conditions being injuries (26.2%), tonsillectomy and adenoidectomy (12.7%), and ear tubes (9.5%). Health status with respect to common conditions differed by race, with blacks having higher rates of obesity, asthma, and very low birth weight (under 2500 g) than whites (Table 2).
Cohort Utilization
From 2007 to 2018, there were 65 projects which requested data and/or samples (Figure 2) resulting in 60 publications (14–73), a patent application, and 9 externally funded grants. These projects focused on a diverse set of phenotypes including arthritis, asthma, epilepsy, obesity, and lupus. Most projects (59.6%) had a genetic component. Slightly over one third of projects used bio-specimens (serum, PBMC, or urine). Just under 5% of the studies only used phenotype data. The lead investigators for the projects included 39 individuals from 17 divisions across CHMC. For the publications, 161 authors were listed, with the median number of publications being 2 per author (interquartile range 2–3; maximum 20). Among these publications, the h-index was 31 with 2,596 citations. Publication major research areas included medicine (77%), immunology and microbiology (41%), and biochemistry, genetics, and molecular biology (36%). With respect to grant funding (from 6 PIs in 5 divisions), 6 of the grants were program project or U-series grants demonstrating the wide impact of the GCC.
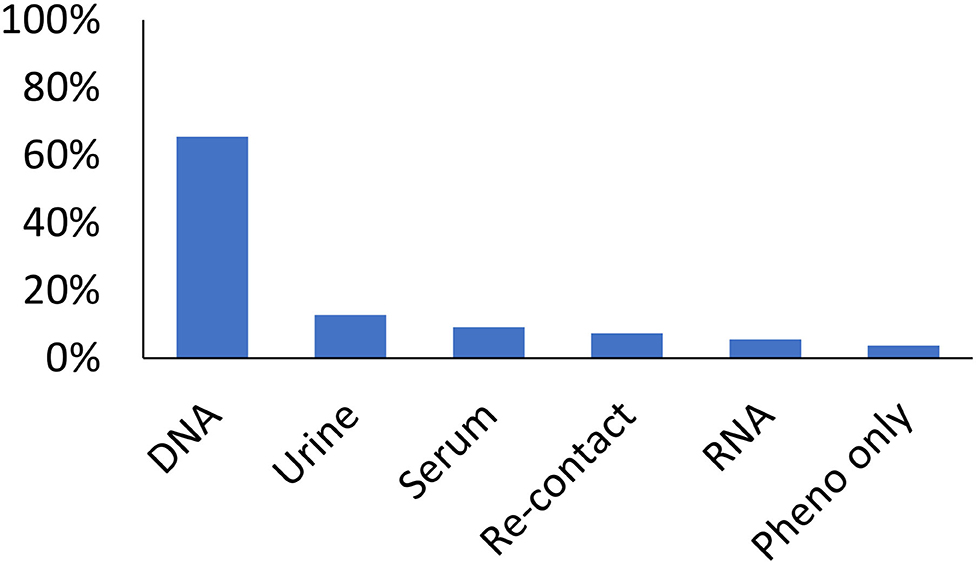
Figure 2. Utilization of the GCC by type of sample by the 65 studies which requested data. Bars represent the proportion of studies utilizing a sample/data. Studies may have utilized multiple types of data/sample.
Benefits of the Cohort
There were two common reasons researchers reported using the GCC. First, researchers noted need for a highly specific group of controls or samples collected in a specific manner. Many required samples from children free from specific a disease or co-morbid condition. Several researchers noted challenges in using publicly available data which did not have required information or had substantially different population distributions compared to their cases. Moreover, researchers noted that residual diagnostic samples were often not collected in a sufficient manner or did not have the necessary accompanying data. Students and trainees noted funding and time limitations for de novo collection.
Limitations of the Cohort
In managing the cohort, several limitations were noted. First, the sample size for non-white participants was insufficient for genetic studies. Second, the cross-sectional design is a missed opportunity as a child's health status is dynamic. Third, some investigators were unable to use the resource because of sample requirements or data availability. Fourth, some data and samples (hair) were never used, wasting resources. Fifth, while there was a diverse group of investigators who used the data, this user group represented a small fraction of potential users. Lastly, participant communication was minimal, limited to annual birthday cards and holiday cards unless re-contact was medically indicated. In later years, a newsletter was sent to highlight the cohort's successes and thank subjects for participation.
Discussion
Meeting the needs of a diverse set of investigators is a central premise for biobanks. When planning the Greater Cincinnati Childhood Cohort (GCC), an institutionally-supported biobank, investigators sought to enable broad use across a wide variety of studies. Recognizing the bias in recruiting only patients seeking care within the hospital, investigators employed a population-based design. Our experiences with the GCC after 10 years have allowed us to consider the benefits and challenges. The wide-ranging cohort utilization and return on investment is evidence of success. However, limitations included minimal racial diversity and static design. Seeking to maximize benefits and minimize challenges for future endeavors, we consider leveraging community-based participatory research approaches.
Strengths of the GCC and Population Representative Biobanks
Over its lifespan, the GCC has advanced a diverse set of research projects as evidence through publications and grants. GCC's success can be attributed to three factors. First, while not as large as many biobanks, a distinct advantage was consistent phenotyping and non-biased recruitment. Having complementary phenotypic data and biological samples is beneficial (74–76). GCC participants were not necessarily free of disease, as to be expected with population recruitment. GCC participants had similar rates of prematurity, but higher rates of asthma and obesity compared to US estimates (77–80). The presence of disease in population-based controls highlights the need to systematically capture phenotypes, especially for common diseases.
Second, there were a diverse set of samples. While a major motivation for GCC's initiation was to serve as a resource for genetic studies, many projects were not genetics focused. Our collection of samples beyond blood, is not typical for most biobanks where the majority of have only collected serum or plasma (77%), few have collected urine (30%) or hair (3%) (81). Paired samples further expand utility.
Third, the GCC cohort was designed to benefit a broad array of research endeavors. While many researchers required a subset of the data, recruitment of participants who could be used in multiple studies was cost effective. Currently, the approach to develop a general institutional research resource is less common than disease focused approaches. Indeed, only 29% of biobanks are developed to facilitate a broad spectrum of research (81).
Limitations of the GCC
While the GCC exhibited many benefits, there were also limitations. First, as the GCC was designed to be population-based in a region that is predominantly white, it had limited numbers of minority participants. This is a problem because blacks are more likely to reside in under-resourced neighborhoods and to experience health disparities (82–87). As inclusion of minority subjects and mitigating health disparities is increasingly a priority of researchers, biomedical research studies, therefore, must ensure that racial diversity is captured (88). Since there are many barriers to participation by racial/ethnic minorities (89), over sampling of minority participants may be needed. Indeed, “All of Us,” NIH's precision medicine initiative, and NHANES oversample minorities to ensure sufficient representation (90).
Although the protocol allowed recall and follow-up of cohort participants, the lack of resources supporting the cohort's follow-up resulted in having a cross-sectional design. While detailed phenotypic information was collected, health status is dynamic with some conditions not diagnosed until later ages. For example, tests for asthma diagnosis are difficult to perform in children under six (91). Likewise, obesity status may change through childhood (92). The cross-sectional nature of the cohort means that the only samples/data available are the ones collected from a single point in time. Continuously evolving technological advances such as genome editing (93) and metabolomics (94) cannot be applied to GCC samples. Further, some data and samples have yet to be used, reducing return on investment.
Lastly, participant outreach was minimal. Specifically, no research findings were shared. This is a problem as one of the seven ethical principles of research is respect for subjects which includes having a mechanism to inform participants of the impact of their participation (95). Unfortunately, dissemination of aggregate research results occurs infrequently (96), even though research participants state that they want such results (97, 98). Further, in addition to aggregate research results, providing education, and sharing clinically relevant individual results to study participants would encourage continued participation. Additionally, when using data/samples, there was no mechanism to consider the participants point of view. While research participant advisory groups often are used when designing studies, inclusion of participant advocates on the advisory committee could provide additional participant protections.
Starting Over: Design a Generalizable Resource for Biomedical Research
Given the benefits and limitations, biobanks that capture generalizable information are essential. Prior work has demonstrated challenges in capturing community diversity using hospital based recruitment (99, 100). However, population representative sampling may lead to insufficient minority numbers. To overcome these issues, we propose to work with community partners to establish a research registry serving as a resource for researchers looking for data and samples for studies, sharing relevant clinical information between healthcare information exchanges or directly with participants, sharing aggregate data with both participants and community partners (e.g., via tailored reports, websites, and newsletters), and spawning community-based participatory research (CBPR) efforts (101). CBPR benefits include increased trust, improved data quality and validity due to participant input, and enhanced data relevance given the input from diverse vested individuals (102). Notably, CBPR consistently increases research participation in under-resourced populations (103–105).
CPBR designs benefit both the community and researchers. Community members gain empowerment and the opportunity to gain understanding on matters important to them. Researchers gain access to a population open to research, an infrastructure for sample and data collection, as well as feedback on the relevance of questions. To ensure a mutually beneficial relationship, researchers would be expected to share results with the community and individual participants as appropriate, providing value for the community. To maximize the utility of the resource, researchers would also be expected to share the data with the biobank, facilitating capacity building. Including a researcher-led component yields dynamic data and sample collection, driven by research questions to ensure maximal utility. Further, following pediatric biobank participants into adulthood should be considered.
While CBPR is an attractive approach, substantial upfront investment is required. Forging partnerships can be challenging (102). Academic partners must create an environment where community members feel comfortable raising issues, sharing opinions, and asking questions. Another challenge is communication. Many under-resourced populations have lower health literacy levels (106–109). Thus, academic partners may need to consider educational efforts on health, science, research, and digital literacy. Lastly, traditional researchers may be uncomfortable and unfamiliar with CBPR research. The strategies and tools used for CBPR research often differ from traditional clinical research and require a degree of flexibility with data capture. As community members are engaged in endeavors, special consideration for human subjects and data protections is required. Consequently, CBPR educational efforts, possibly co-designed with community members, and CBPR training and support for researchers is needed. Further, recognition that some researchers may not be well-suited to work directly with community and options for other ways these researchers could leverage existing data and samples would be essential.
Conclusions
In summary, numerous studies benefit from an institutional biobank such as the GCC. Availability of appropriate controls with existing phenotype data as well as a wide variety of available samples purposefully collected can (1) substantially lower cost and time, and (2) inspire and enable unique exploratory efforts. While the population-based sampling employed in the GCC enabled broad utilization, partnering with communities to establish research registries could provide a cost effective mutually beneficial resource.
Data Availability Statement
The datasets generated for this study are available on request to the corresponding author.
Ethics Statement
The studies involving human participants were reviewed and approved by Cincinnati Children's Institutional Review Board. Written informed consent to participate in this study was provided by the participants' legal guardian/next of kin.
Author Contributions
LMa conceived the study, performed the analyses, interpreted the data, and drafted the manuscript. LMu interpreted the results and provided critical revision. MBK conceived the study, interpreted the results, and provided critical revision.
Funding
This work was supported by Cincinnati Children's Hospital Medical Center.
Conflict of Interest
The authors declare that the research was conducted in the absence of any commercial or financial relationships that could be construed as a potential conflict of interest.
Acknowledgments
We would like to acknowledge Dr. David Glass, without his vision and passion to develop a biobank for Cincinnati Children's Hospital Medical Center, the GCC would not exist. We would like to thank the research subjects and their parents for their participation. Lastly, we would like to thank the leadership of Cincinnati Children's Hospital Medical Center especially Drs. Thomas Boat and Arnold Strauss who supported this endeavor.
Supplementary Material
The Supplementary Material for this article can be found online at: https://www.frontiersin.org/articles/10.3389/fpubh.2020.535116/full#supplementary-material
Supplementary Figure 1. Demographic comparison of the GCC to US census data.
References
1. Artene SA, Ciurea ME, Purcaru SO, Tache DE, Tataranu LG, Lupu M, et al. Biobanking in a constantly developing medical world. Sci World J. (2013) 2013:343275. doi: 10.1155/2013/343275
2. Wacholder S, McLaughlin JK, Silverman DT, Mandel JS. Selection of controls in case-control studies. I. Principles. Am J Epidemiol. (1992) 135:1019–28. doi: 10.1093/oxfordjournals.aje.a116396
3. Keyes KM, Westreich D. UK Biobank, big data, and the consequences of non-representativeness. Lancet. (2019) 393:1297. doi: 10.1016/S0140-6736(18)33067-8
4. Wacholder S, Silverman DT, McLaughlin JK, Mandel JS. Selection of controls in case-control studies. III. Design options. Am J Epidemiol. (1992) 135:1042–50. doi: 10.1093/oxfordjournals.aje.a116398
5. Yancey AK, Ortega AN, Kumanyika SK. Effective recruitment and retention of minority research participants. Annu Rev Public Health. (2006) 27:1–28. doi: 10.1146/annurev.publhealth.27.021405.102113
6. United States Census Bureau. Quick Facts; United States census.gov: US Department of Commerce (2018). Available online at: https://www.census.gov/quickfacts/fact/table/US/PST045218.
7. Hussain-Gambles M, Atkin K, Leese B. Why ethnic minority groups are under-represented in clinical trials: a review of the literature. Health Soc Care Community. (2004) 12:382–8. doi: 10.1111/j.1365-2524.2004.00507.x
8. Davis S, Wright PW, Schulman SF, Hill LD, Pinkham RD, Johnson LP, et al. Participants in prospective, randomized clinical trials for resected non-small cell lung cancer have improved survival compared with nonparticipants in such trials. Cancer. (1985) 56:1710. doi: 10.1002/1097-0142(19851001)56:7<1710::AID-CNCR2820560741>3.0.CO;2-T
9. Heiat A, Gross CP, Krumholz HM. Representation of the elderly, women, and minorities in heart failure clinical trials. Arch Intern Med. (2002) 162:1682–8. doi: 10.1001/archinte.162.15.1682
10. Miranda J, Nakamura R, Bernal G. Including ethnic minorities in mental health intervention research: a practical approach to a long-standing problem. Cult Med Psychiatry. (2003) 27:467–86. doi: 10.1023/B:MEDI.0000005484.26741.79
11. Dolan LM, Bean J, D'Alessio D, Cohen RM, Morrison JA, Goodman E, et al. Frequency of abnormal carbohydrate metabolism and diabetes in a population-based screening of adolescents. J Pediatr. (2005) 146:751–8. doi: 10.1016/j.jpeds.2005.01.045
12. Center for Disease Control. Center for Disease Control and Prevention: A SAS Program for the CDC Growth Charts. (2002). Available online at: www.cdc.gov/nccdphp/dnpa/growthcharts/sas.htm.
13. U.S. Census Bureau. 2010 Census SUmmary FIl1: Table DP05: U.S. Census Bureau (2010). [Generated by Lisa Martin using American FactFinder]. Available online at: http://factfinder2.census.gov.
14. Suzuki M, Wiers KM, Klein-Gitelman MS, Haines KA, Olson J, Onel KB, et al. Neutrophil gelatinase-associated lipocalin as a biomarker of disease activity in pediatric lupus nephritis. Pediatr Nephrol. (2008) 23:403–12. doi: 10.1007/s00467-007-0685-x
15. Barnes MG, Grom AA, Thompson SD, Griffin TA, Pavlidis P, Itert L, et al. Subtype-specific peripheral blood gene expression profiles in recent-onset juvenile idiopathic arthritis. Arthrit Rheumatism. (2009) 60:2102–12. doi: 10.1002/art.24601
16. Daniels ZS, Nick TG, Liu C, Cassedy A, Glauser TA. Obesity is a common comorbidity for pediatric patients with untreated, newly diagnosed epilepsy. Neurology. (2009) 73:658–64. doi: 10.1212/WNL.0b013e3181ab2b11
17. Griffin TA, Barnes MG, Ilowite NT, Olson JC, Sherry DD, Gottlieb BS, et al. Gene expression signatures in polyarticular juvenile idiopathic arthritis demonstrate disease heterogeneity and offer a molecular classification of disease subsets. Arthrit Rheumatism. (2009) 60:2113–23. doi: 10.1002/art.24534
18. Hollenbach JA, Thompson SD, Bugawan TL, Ryan M, Sudman M, Marion M, et al. Juvenile idiopathic arthritis and HLA class I and class II interactions and age-at-onset effects. Arthrit Rheumatism. (2010) 62:1781–91. doi: 10.1002/art.27424
19. Moncrieffe H, Hinks A, Ursu S, Kassoumeri L, Etheridge A, Hubank M, et al. Generation of novel pharmacogenomic candidates in response to methotrexate in juvenile idiopathic arthritis: correlation between gene expression and genotype. Pharmacogenet Genom. (2010) 20:665–76. doi: 10.1097/FPC.0b013e32833f2cd0
20. Sherrill JD, Gao PS, Stucke EM, Blanchard C, Collins MH, Putnam PE, et al. Variants of thymic stromal lymphopoietin and its receptor associate with eosinophilic esophagitis. J Allergy Clin Immunol. (2010) 126:160–5.e3. doi: 10.1016/j.jaci.2010.04.037
21. Thompson SD, Sudman M, Ramos PS, Marion MC, Ryan M, Tsoras M, et al. The susceptibility loci juvenile idiopathic arthritis shares with other autoimmune diseases extend to PTPN2, COG6, and ANGPT1. Arthrit Rheumat. (2010) 62:3265–76. doi: 10.1002/art.27688
22. Thomson G, Marthandan N, Hollenbach JA, Mack SJ, Erlich HA, Single RM, et al. Sequence Feature Variant Type (SFVT) Analysis of the HLA Genetic Association in Juvenile Idiopathic Arthritis. Pacific Symposium on Biocomputing Pacific Symposium on Biocomputing (2010). p. 359–70. doi: 10.1142/9789814295291_0038
23. Baye TM, Butsch Kovacic M, Biagini Myers JM, Martin LJ, Lindsey M, Patterson TL, et al. Differences in candidate gene association between European ancestry and African American asthmatic children. PLoS ONE. (2011) 6:e16522. doi: 10.1371/journal.pone.0016522
24. Hinks A, Moncrieffe H, Martin P, Ursu S, Lal S, Kassoumeri L, et al. Association of the 5-aminoimidazole-4-carboxamide ribonucleotide transformylase gene with response to methotrexate in juvenile idiopathic arthritis. Annals Rheumat Dis. (2011) 70:1395–400. doi: 10.1136/ard.2010.146191
25. Hunt KA, Smyth DJ, Balschun T, Ban M, Mistry V, Ahmad T, et al. Rare and functional SIAE variants are not associated with autoimmune disease risk in up to 66,924 individuals of European ancestry. Nat Genet. (2011) 44:3–5. doi: 10.1038/ng.1037
26. Kovacic MB, Myers JM, Wang N, Martin LJ, Lindsey M, Ericksen MB, et al. Identification of KIF3A as a novel candidate gene for childhood asthma using RNA expression and population allelic frequencies differences. PLoS ONE. (2011) 6:e23714. doi: 10.1371/journal.pone.0023714
27. Nylund CM, D'Mello S, Kim MO, Bonkowski E, Dabritz J, Foell D, et al. Granulocyte macrophage-colony-stimulating factor autoantibodies and increased intestinal permeability in Crohn disease. J Pediatr Gastroenterol Nutrition. (2011) 52:542–8. doi: 10.1097/MPG.0b013e3181fe2d93
28. Epstein TG, LeMasters GK, Bernstein DI, Ericksen MB, Martin LJ, Ryan PH, et al. Genetic variation in small proline rich protein 2B as a predictor for asthma among children with eczema. Ann Allergy Asthma Immunol. (2012) 108:145–50. doi: 10.1016/j.anai.2012.01.004
29. Ercan A, Barnes MG, Hazen M, Tory H, Henderson L, Dedeoglu F, et al. Multiple juvenile idiopathic arthritis subtypes demonstrate proinflammatory IgG glycosylation. Arthritis Rheumat. (2012) 64:3025–33. doi: 10.1002/art.34507
30. Hinks A, Cobb J, Sudman M, Eyre S, Martin P, Flynn E, et al. Investigation of rheumatoid arthritis susceptibility loci in juvenile idiopathic arthritis confirms high degree of overlap. Annals Rheumat Dis. (2012) 71:1117–21. doi: 10.1136/annrheumdis-2011-200814
31. Martin LJ, Gupta J, Jyothula SS, Butsch Kovacic M, Biagini Myers JM, Patterson TL, et al. Functional variant in the autophagy-related 5 gene promotor is associated with childhood asthma. PLoS ONE. (2012) 7:e33454. doi: 10.1371/journal.pone.0033454
32. Prahalad S, Thompson SD, Conneely KN, Jiang Y, Leong T, Prozonic J, et al. Hierarchy of risk of childhood-onset rheumatoid arthritis conferred by HLA-DRB1 alleles encoding the shared epitope. Arthritis Rheumatism. (2012) 64:925–30. doi: 10.1002/art.33376
33. Thompson SD, Marion MC, Sudman M, Ryan M, Tsoras M, Howard TD, et al. Genome-wide association analysis of juvenile idiopathic arthritis identifies a new susceptibility locus at chromosomal region 3q13. Arthritis Rheumatism. (2012) 64:2781–91. doi: 10.1002/art.34429
34. Faraco J, Lin L, Kornum BR, Kenny EE, Trynka G, Einen M, et al. ImmunoChip study implicates antigen presentation to T cells in narcolepsy. PLoS Genet. (2013) 9:e1003270. doi: 10.1371/journal.pgen.1003270
35. Gorelik M, Fall N, Altaye M, Barnes MG, Thompson SD, Grom AA, et al. Follistatin-like protein 1 and the ferritin/erythrocyte sedimentation rate ratio are potential biomarkers for dysregulated gene expression and macrophage activation syndrome in systemic juvenile idiopathic arthritis. J Rheumatol. (2013) 40:1191–9. doi: 10.3899/jrheum.121131
36. Hinks A, Cobb J, Marion MC, Prahalad S, Sudman M, Bowes J, et al. Dense genotyping of immune-related disease regions identifies 14 new susceptibility loci for juvenile idiopathic arthritis. Nat Genet. (2013) 45:664–9. doi: 10.1038/ng.2614
37. Hinks A, Martin P, Thompson SD, Sudman M, Stock CJ, Thomson W, et al. Autoinflammatory gene polymorphisms and susceptibility to UK juvenile idiopathic arthritis. Pediatr Rheumatol Online J. (2013) 11:14. doi: 10.1186/1546-0096-11-14
38. Namjou B, Keddache M, Marsolo K, Wagner M, Lingren T, Cobb B, et al. EMR-linked GWAS study: investigation of variation landscape of loci for body mass index in children. Front Genet. (2013) 4:268. doi: 10.3389/fgene.2013.00268
39. Prahalad S, Conneely KN, Jiang Y, Sudman M, Wallace CA, Brown MR, et al. Susceptibility to childhood-onset rheumatoid arthritis: investigation of a weighted genetic risk score that integrates cumulative effects of variants at five genetic loci. Arthritis Rheumatism. (2013) 65:1663–7. doi: 10.1002/art.37913
40. Biagini Myers JM, Martin LJ, Kovacic MB, Mersha TB, He H, Pilipenko V, et al. Epistasis between serine protease inhibitor Kazal-type 5 (SPINK5) and thymic stromal lymphopoietin (TSLP) genes contributes to childhood asthma. J Allergy Clin Immunol. (2014) 134:891–9.e3. doi: 10.1016/j.jaci.2014.03.037
41. Kaufman KM, Linghu B, Szustakowski JD, Husami A, Yang F, Zhang K, et al. Whole-exome sequencing reveals overlap between macrophage activation syndrome in systemic juvenile idiopathic arthritis and familial hemophagocytic lymphohistiocytosis. Arthrit Rheumatol. (2014) 66:3486–95. doi: 10.1002/art.38793
42. Kim-Howard X, Sun C, Molineros JE, Maiti AK, Chandru H, Adler A, et al. Allelic heterogeneity in NCF2 associated with systemic lupus erythematosus (SLE) susceptibility across four ethnic populations. Human Mol Genet. (2014) 23:1656–68. doi: 10.1093/hmg/ddt532
43. Kottyan LC, Davis BP, Sherrill JD, Liu K, Rochman M, Kaufman K, et al. Genome-wide association analysis of eosinophilic esophagitis provides insight into the tissue specificity of this allergic disease. Nat Genet. (2014) 46:895–900. doi: 10.1038/ng.3033
44. Mitsnefes M, Scherer PE, Friedman LA, Gordillo R, Furth S, Warady BA, et al. Ceramides and cardiac function in children with chronic kidney disease. Pediatr Nephrol. (2014) 29:415–22. doi: 10.1007/s00467-013-2642-1
45. Namjou B, Marsolo K, Caroll RJ, Denny JC, Ritchie MD, Verma SS, et al. Phenome-wide association study (PheWAS) in EMR-linked pediatric cohorts, genetically links PLCL1 to speech language development and IL5-IL13 to Eosinophilic Esophagitis. Front Genet. (2014) 5:401. doi: 10.3389/fgene.2014.00401
46. Namjou B Ni Y Harley IT Chepelev I Cobb B Kottyan LC . The effect of inversion at 8p23 on BLK association with lupus in Caucasian population. PLoS ONE. (2014) 9:e115614. doi: 10.1371/journal.pone.0115614
47. Verma SS, de Andrade M, Tromp G, Kuivaniemi H, Pugh E, Namjou-Khales B, et al. Imputation and quality control steps for combining multiple genome-wide datasets. Front Genet. (2014) 5:370. doi: 10.3389/fgene.2014.00370
48. Woo D, Falcone GJ, Devan WJ, Brown WM, Biffi A, Howard TD, et al. Meta-analysis of genome-wide association studies identifies 1q22 as a susceptibility locus for intracerebral hemorrhage. Am J Human Genet. (2014) 94:511–21. doi: 10.1016/j.ajhg.2014.02.012
49. Angeles-Han ST, McCracken C, Yeh S, Jang SR, Jenkins K, Cope S, et al. HLA Associations in a cohort of children with juvenile idiopathic arthritis with and without uveitis. Invest Ophthalmol Vis Sci. (2015) 56:6043–8. doi: 10.1167/iovs.15-17168
50. Bennett MR, Nehus E, Haffner C, Ma Q, Devarajan P. Pediatric reference ranges for acute kidney injury biomarkers. Pediatr Nephrol. (2015) 30:677–85. doi: 10.1007/s00467-014-2989-y
51. Butsch Kovacic M, Martin LJ, Biagini Myers JM, He H, Lindsey M, Mersha TB, et al. Genetic approach identifies distinct asthma pathways in overweight vs normal weight children. Allergy. (2015) 70:1028–32. doi: 10.1111/all.12656
52. Crosslin DR, Carrell DS, Burt A, Kim DS, Underwood JG, Hanna DS, et al. Genetic variation in the HLA region is associated with susceptibility to herpes zoster. Genes Immunity. (2015) 16:1–7. doi: 10.1038/gene.2014.51
53. LeMasters GK, Khurana Hershey GK, Sivaprasad U, Martin LJ, Pilipenko V, Ericksen MB, et al. N-acetyltransferase 1 polymorphism increases cotinine levels in Caucasian children exposed to secondhand smoke: the CCAAPS birth cohort. Pharmacogenom J. (2015) 15:189–95. doi: 10.1038/tpj.2014.44
54. Mersha TB, Martin LJ, Biagini Myers JM, Kovacic MB, He H, Lindsey M, et al. Genomic architecture of asthma differs by sex. Genomics. (2015) 106:15–22. doi: 10.1016/j.ygeno.2015.03.003
55. Namjou B, Marsolo K, Lingren T, Ritchie MD, Verma SS, Cobb BL, et al. A GWAS study on liver function test using eMERGE network participants. PLoS ONE. (2015) 10:e0138677. doi: 10.1371/journal.pone.0138677
56. Ombrello MJ, Remmers EF, Tachmazidou I, Grom A, Foell D, Haas JP, et al. HLA-DRB1*11 and variants of the MHC class II locus are strong risk factors for systemic juvenile idiopathic arthritis. Proc Natl Acad Sci USA. (2015) 112:15970–5. doi: 10.1073/pnas.1520779112
57. Xiao C, Biagini Myers JM, Ji H, Metz K, Martin LJ, Lindsey M, et al. Vanin-1 expression and methylation discriminate pediatric asthma corticosteroid treatment response. J Allergy Clin Immunol. (2015) 136:923–31.e3. doi: 10.1016/j.jaci.2015.01.045
58. Xiao N, Devarajan P, Inge TH, Jenkins TM, Bennett M, Mitsnefes MM. Subclinical kidney injury before and 1 year after bariatric surgery among adolescents with severe obesity. Obesity. (2015) 23:1234–8. doi: 10.1002/oby.21070
59. Yucesoy B, Kaufman KM, Lummus ZL, Weirauch MT, Zhang G, Cartier A, et al. Genome-wide association study identifies novel loci associated with diisocyanate-induced occupational Asthma. Toxicol Sci. (2015) 146:192–201. doi: 10.1093/toxsci/kfv084
60. Basil JS, Santoro SL, Martin LJ, Healy KW, Chini BA, Saal HM. Retrospective study of obesity in children with down syndrome. J Pediatr. (2016) 173:143–8. doi: 10.1016/j.jpeds.2016.02.046
61. Davis BP, Epstein T, Kottyan L, Amin P, Martin LJ, Maddox A, et al. Association of eosinophilic esophagitis and hypertrophic cardiomyopathy. J Allergy Clin Immunol. (2016) 137:934–6.e5. doi: 10.1016/j.jaci.2015.08.026
62. Kaddourah A, Goldstein SL, Basu R, Nehus EJ, Terrell TC, Brunner L, et al. Novel urinary tubular injury markers reveal an evidence of underlying kidney injury in children with reduced left ventricular systolic function: a pilot study. Pediatr Nephrol. (2016) 31:1637–45. doi: 10.1007/s00467-016-3360-2
63. Liu K, Kurien BT, Zimmerman SL, Kaufman KM, Taft DH, Kottyan LC, et al. X chromosome dose and sex bias in autoimmune diseases: increased prevalence of 47, XXX in systemic lupus erythematosus and sjogren's syndrome. Arthrit Rheumatol. (2016) 68:1290–300. doi: 10.1002/art.39560
64. Adelsperger S, Prows CA, Myers MF, Perry CL, Chandler A, Holm IA, et al. Parental perception of self-empowerment in pediatric pharmacogenetic testing: the reactions of parents to the communication of actual and hypothetical CYP2D6 test results. Health Commun. (2017) 32:1104–11. doi: 10.1080/10410236.2016.1214216
65. Ambroggio L, Florin TA, Shah SS, Ruddy R, Yeomans L, Trexel J, et al. Emerging biomarkers of illness severity: urinary metabolites associated with sepsis and necrotizing methicillin-resistant Staphylococcus aureus Pneumonia. Pharmacotherapy. (2017) 37:1033–42. doi: 10.1002/phar.1973
66. Bennett MR, Ma Q, Ying J, Devarajan P, Brunner H. Effects of age and gender on reference levels of biomarkers comprising the pediatric renal activity index for lupus nephritis (p-RAIL). Pediatr rheumatol Online J. (2017) 15:74. doi: 10.1186/s12969-017-0202-0
67. Johansson E, Biagini Myers JM, Martin LJ, He H, Pilipenko V, Mersha T, et al. KIF3A genetic variation is associated with pediatric asthma in the presence of eczema independent of allergic rhinitis. J Allergy Clin Immunol. (2017) 140:595–8.e5. doi: 10.1016/j.jaci.2017.02.003
68. Myers MF, Zhang X, McLaughlin B, Kissell D, Perry CL, Veerkamp M, et al. Prior opioid exposure influences parents' sharing of their children's CYP2D6 research results. Pharmacogenomics. (2017) 18:1199–213. doi: 10.2217/pgs-2017-0091
69. Turnier JL, Fall N, Thornton S, Witte D, Bennett MR, Appenzeller S, et al. Urine S100 proteins as potential biomarkers of lupus nephritis activity. Arthritis Res Ther. (2017) 19:242. doi: 10.1186/s13075-017-1444-4
70. Davis S, Nehus E, Inge T, Zhang W, Setchell K, Mitsnefes M. Effect of bariatric surgery on urinary sphingolipids in adolescents with severe obesity. Surg Obes Relat Dis. (2018) 14:446–51. doi: 10.1016/j.soard.2017.12.017
71. Jensen ET, Kuhl JT, Martin LJ, Langefeld CD, Dellon ES, Rothenberg ME. Early-life environmental exposures interact with genetic susceptibility variants in pediatric patients with eosinophilic esophagitis. J Allergy Clin Immunol. (2018) 141:632–7.e5. doi: 10.1016/j.jaci.2017.07.010
72. Jensen ET, Kuhl JT, Martin LJ, Rothenberg ME, Dellon ES. Prenatal, intrapartum, and postnatal factors are associated with pediatric eosinophilic esophagitis. J Allergy Clin Immunol. (2018) 141:214–22. doi: 10.1016/j.jaci.2017.05.018
73. Martin LJ, He H, Collins MH, Abonia JP, Biagini Myers JM, Eby M, et al. Eosinophilic esophagitis (EoE) genetic susceptibility is mediated by synergistic interactions between EoE-specific and general atopic disease loci. J Allergy Clin Immunol. (2018) 141:1690–8. doi: 10.1016/j.jaci.2017.09.046
74. Riegman PH, Dinjens WN, Oosterhuis JW. Biobanking for interdisciplinary clinical research. Pathobiology. (2007) 74:239–44. doi: 10.1159/000104451
75. Riegman PH, Morente MM, Betsou F, de Blasio P, Geary P Marble arch international working group on biobanking for biomedical R. Biobanking for better healthcare. Mol Oncol. (2008) 2:213–22. doi: 10.1016/j.molonc.2008.07.004
76. Brisson AR, Matsui D, Rieder MJ, Fraser DD. Translational research in pediatrics: tissue sampling and biobanking. Pediatrics. (2012) 129:153–62. doi: 10.1542/peds.2011-0134
77. Ogden CL, Carroll MD, Curtin LR, Lamb MM, Flegal KM. Prevalence of high body mass index in US children and adolescents, 2007-2008. JAMA. (2010) 303:242–9. doi: 10.1001/jama.2009.2012
78. Skinner AC, Skelton JA. Prevalence and trends in obesity and severe obesity among children in the United States, 1999-2012. JAMA Pediatr. (2014) 168:561–6. doi: 10.1001/jamapediatrics.2014.21
79. Moorman J, Akinbami LJ, Bailey CM. National Surveillance of Asthma: United States, 2001-2010. National Center for Health Statistics: Vital Health Stat (2012). p. 1–67.
80. Martin JA, Hamilton BE, Osterman MK, CUrtin SC, Mathews TJ. Births: Final Data for 2012. National Vital Statistics Reports In: Services USDoHaH, editor. Hyattsville, MD National Center for Health Statistics (2013). p. 1–87.
81. Henderson GE, Cadigan RJ, Edwards TP, Conlon I, Nelson AG, Evans JP, et al. Characterizing biobank organizations in the U.S.: results from a national survey. Genome Med. (2013) 5:3. doi: 10.1186/gm407
82. Beck AF, Moncrief T, Huang B, Simmons JM, Sauers H, Chen C, et al. Inequalities in neighborhood child asthma admission rates and underlying community characteristics in one US county. J Pediatr. (2013) 163:574–80. doi: 10.1016/j.jpeds.2013.01.064
83. Guilbert T, Zeiger RS, Haselkorn T, Iqbal A, Alvarez C, Mink DR, et al. Racial disparities in asthma-related health outcomes in children with severe/difficult-to-treat Asthma. J Allergy Clin Immunol Pract. (2018) 7:568–77. doi: 10.1016/j.jaip.2018.07.050
84. Univerisity of Cincinnati Institute for Policy Research. Greater Cincinnati Communiyt Health Status Survey. Spring 2017: Interact for Health (2017).
85. City of Cincinnati. Communty Health Assesmsent. Cincinnati OH: City of Cincinnati Health Commissioner's Accreditation Lead Team (2017).
86. City of Cincinnat. Neighborhood Life Expectancy Data 2019. Available online at: https://www.cincinnati-oh.gov/health/community-health-data/neighborhood-life-expectancy-data/.
87. The Health Collaborative. Community Health Needs Assessment: A regional collaborative report produced by the Health Collaborative. The Health Collaborative (2016).
88. Allmark P. Should research samples reflect the diversity of the population? J Med Ethics. (2004) 30:185–9. doi: 10.1136/jme.2003.004374
89. George S, Duran N, Norris K. A systematic review of barriers and facilitators to minority research participation among African Americans, Latinos, Asian Americans, and Pacific Islanders. Am J Public Health. (2014) 104:e16–31. doi: 10.2105/AJPH.2013.301706
90. Ezzati-Rice TM, Murphy RS. Issues associated with the design of a national probability sample for human exposure assessment. Environ Health Perspect. (1995) 103(Suppl 3):55–9. doi: 10.1289/ehp.95103s355
91. Ducharme FM, Dell SD, Radhakrishnan D, Grad RM, Watson WT, Yang CL, et al. Diagnosis and management of asthma in preschoolers: a Canadian thoracic society and canadian paediatric society position paper. Paediatr Child Health. (2015) 20:353–71. doi: 10.1093/pch/20.7.353
92. Cheung PC, Cunningham SA, Narayan KM, Kramer MR. Childhood obesity incidence in the United States: a systematic review. Child Obes. (2016) 12:1–11. doi: 10.1089/chi.2015.0055
93. Ben Jehuda R, Shemer Y, Binah O. Genome editing in induced pluripotent stem cells using CRISPR/Cas9. Stem Cell Rev. (2018) 14:323–36. doi: 10.1007/s12015-018-9811-3
94. Clish CB. Metabolomics: an emerging but powerful tool for precision medicine. Cold Spring Harb Mol Case Stud. (2015) 1:a000588. doi: 10.1101/mcs.a000588
95. Emanuel EJ, Wendler D, Grady C. What makes clinical research ethical? JAMA. (2000) 283:2701–11. doi: 10.1001/jama.283.20.2701
96. Chen PG, Diaz N, Lucas G, Rosenthal MS. Dissemination of results in community-based participatory research. Am J Prev Med. (2010) 39:372–8. doi: 10.1016/j.amepre.2010.05.021
97. Shalowitz DI, Miller FG. Communicating the results of clinical research to participants: attitudes, practices, and future directions. PLoS Med. (2008) 5:e91. doi: 10.1371/journal.pmed.0050091
98. Partridge AH, Wong JS, Knudsen K, Gelman R, Sampson E, Gadd M, et al. Offering participants results of a clinical trial: sharing results of a negative study. Lancet. (2005) 365:963–4. doi: 10.1016/S0140-6736(05)71085-0
99. Sutton-Tyrrell K. Assessing bias in case-control studies. Proper selection of cases and controls. Stroke. (1991) 22:938–42. doi: 10.1161/01.STR.22.7.938
100. Mezei G, Kheifets L. Selection bias and its implications for case-control studies: a case study of magnetic field exposure and childhood leukaemia. Int J Epidemiol. (2006) 35:397–406. doi: 10.1093/ije/dyi245
101. Israel BA, Schulz AJ, Parker EA, Becker AB. Review of community-based research: assessing partnership approaches to improve public health. Annu Rev Public Health. (1998) 19:173–202. doi: 10.1146/annurev.publhealth.19.1.173
102. Israel BA, Parker EA, Rowe Z, Salvatore A, Minkler M, Lopez J, et al. Community-based participatory research: lessons learned from the Centers for Children's Environmental Health and Disease Prevention Research. Environ Health Perspect. (2005) 113:1463–71. doi: 10.1289/ehp.7675
103. Skinner HG, Calancie L, Vu MB, Garcia B, DeMarco M, Patterson C, et al. Using community-based participatory research principles to develop more understandable recruitment and informed consent documents in genomic research. PLoS ONE. (2015) 10:e0125466. doi: 10.1371/journal.pone.0125466
104. De las Nueces D, Hacker K, DiGirolamo A, Hicks LS. A systematic review of community-based participatory research to enhance clinical trials in racial and ethnic minority groups. Health Serv Res. (2012) 47(3 Pt 2):1363–86. doi: 10.1111/j.1475-6773.2012.01386.x
105. Hanza MM, Goodson M, Osman A, Porraz Capetillo MD, Hared A, Nigon JA, et al. Lessons learned from community-led recruitment of immigrants and refugee participants for a randomized, community-based participatory research study. J Immigr Minor Health. (2016) 18:1241–5. doi: 10.1007/s10903-016-0394-2
106. Shea JA, Beers BB, McDonald VJ, Quistberg DA, Ravenell KL, Asch DA. Assessing health literacy in African American and Caucasian adults: disparities in rapid estimate of adult literacy in medicine (REALM) scores. Fam Med. (2004) 36:575–81.
107. Chesser A, Burke A, Reyes J, Rohrberg T. Navigating the digital divide: a systematic review of eHealth literacy in underserved populations in the United States. Inform Health Soc Care. (2016) 41:1–19. doi: 10.3109/17538157.2014.948171
108. Valerio MA, Peterson EL, Wittich AR, Joseph CL. Examining health literacy among urban African-American adolescents with asthma. J Asthma. (2016) 53:1041–7. doi: 10.1080/02770903.2016.1175473
Keywords: children, research, epidemiology, community, genetics
Citation: Martin LJ, Murrison LB and Butsch Kovacic M (2021) Building a Population Representative Pediatric Biobank: Lessons Learned From the Greater Cincinnati Childhood Cohort. Front. Public Health 8:535116. doi: 10.3389/fpubh.2020.535116
Received: 14 February 2020; Accepted: 15 December 2020;
Published: 14 January 2021.
Edited by:
Dora Il'yasova, Georgia State University, United StatesReviewed by:
Bartha Maria Knoppers, McGill University, CanadaJoy D. Doll, Creighton University, United States
Copyright © 2021 Martin, Murrison and Butsch Kovacic. This is an open-access article distributed under the terms of the Creative Commons Attribution License (CC BY). The use, distribution or reproduction in other forums is permitted, provided the original author(s) and the copyright owner(s) are credited and that the original publication in this journal is cited, in accordance with accepted academic practice. No use, distribution or reproduction is permitted which does not comply with these terms.
*Correspondence: Lisa J. Martin, lisa.martin@cchmc.org