- 1Department of Population Health, London School of Hygiene and Tropical Medicine, London, United Kingdom
- 2MRC/WITS Rural Public Health Transitions Research Unit (Agincourt), Faculty of Health Sciences, School of Public Health, University of Witwatersrand, Johannesburg, South Africa
- 3Kilimanjaro Christian Medical University College, Moshi, Tanzania
- 4MeSH Consortium, Department of Public Health Environments and Society, Faculty of Public Health and Policy, London School of Hygiene and Tropical Medicine, London, United Kingdom
Background: Monitoring progress toward global treatment targets using HIV programme data in sub-Saharan Africa has proved challenging. Constraints in routine data collection and reporting can lead to biased estimates of treatment outcomes. In 2010, South Africa introduced an electronic patient monitoring system for HIV patient visits, TIER.Net. We compare treatment status and outcomes recorded in TIER.Net to outcomes ascertained through detailed record review and tracing in order to assess discrepancies and biases in retention and mortality rates.
Methods: The Agincourt Health and Demographic Surveillance System (HDSS) in north-eastern South Africa is served by eight public primary healthcare facilities. Since 2014, HIV patient visits are logged electronically at these clinics, with patient records individually linked to their HDSS record. These data were used to generate a list of patients >90 days late for their last scheduled clinic visit and deemed lost to follow-up (LTFU). Patient outcomes were ascertained through a review of the TIER.Net database, physical patient files, registers kept by two non-government organizations that assist with patient tracing, cross-referencing with the HDSS records and supplementary physical tracing. Descriptive statistics were used to compare patient outcomes reported in TIER.Net to their outcome ascertained in the study.
Results: Of 1,074 patients that were eligible for this analysis, TIER.Net classified 533 (49.6%) as LTFU, 80 (7.4%) as deceased, and 186 (17.3%) as transferred out. TIER.Net misclassified 36% of patient outcomes, overestimating LTFU and underestimating mortality and transfers out. TIER.Net missed 40% of deaths and 43% of transfers out. Patients categorized as LTFU in TIER.Net were more likely to be misclassified than patients classified as deceased or transferred out.
Discussion: Misclassification of patient outcomes in TIER.Net has consequences for programme forecasting, monitoring and evaluation. Undocumented transfers accounted for the majority of misclassification, suggesting that the transfer process between clinics should be improved for more accurate reporting of patient outcomes. Processes that lead to correct classification of patient status including patient tracing should be strengthened. Clinics could cross-check all available data sources before classifying patients as LTFU. Programme evaluators and modelers could consider using correction factors to improve estimates of outcomes from TIER.Net.
Introduction
At the end of 2017, it was estimated that there were 36.9 million people living with HIV (PLHIV) worldwide, with 70% of the disease burden situated in sub-Saharan Africa (1, 2). The World Health Organization's (WHO) revised HIV treatment guidelines in 2015 call for immediate provision of lifelong antiretroviral therapy (ART) to all people testing positive for HIV. By the end of 2017, 60% of PLHIV in sub-Saharan Africa were on ART (1, 2). Whilst ART initiation rates have been increasing over time, in order to reduce HIV transmission rates and achieve 90-90-90 AIDS elimination goals, there is a need for accelerated increases in treatment adherence and retention in care (3–5). South Africa has the largest population of PLHIV worldwide, with an estimated 18.8% of the adult population aged 15–49 years old living with HIV, representing 7.2 million people (1, 2). By the end of 2018, an estimated 68% of PLHIV in South Africa were on ART (1, 2).
The rapid growth in access to ART has accentuated the need for an affordable and accurate way to monitor and evaluate treatment programmes (6–8), including documenting the number of people alive and on treatment, and programme impact on mortality. In the past, the progress of patients on ART was mainly monitored through patient cohorts (9) and tallying numbers of services rendered to inform resource allocation (8). However, evaluation of HIV programmes has proved challenging due to multiple data constraints. These include concerns about data reliability (8), and continued use of paper registers which often lack unique identifiers, suffer from incompleteness (10), and are cumbersome to use with increasing patient numbers and length of patient follow-up (11, 12). Another major concern is “silent transfers” whereby patients change clinics informally and without accompanying documentation, a phenomenon which has become more prevalent with the expansion of ART programmes (13, 14). As a result, there is concern that many high-burden countries are ill-equipped to report on the outcomes of patients in care and on treatment (6, 7, 15–17).
In order to address these concerns, many countries are scaling-up the use of electronic patient registers (11). However, challenges persist including insufficient linkages between clinics (10), insufficient training of staff who are responsible for entering this information (10), and staff shortages (18–21), resulting in some staff responsible for data management being stretched across multiple roles (22). This sometimes leads to poor workflow, and staff resistance which results in poor change management. Privacy and security issues (22) are also a major concern.
In 2010, South Africa adopted TIER.Net, a three-tiered monitoring approach involving paper registers (TIER 1—recommended for facilities with <500 patients), an offline electronic register (TIER 2—recommended for facilities with 500–2,000 patients) and networked electronic medical records (TIER 3—recommended for facilities with more than 2,000 patients) (11). This allowed for different tiers to be implemented in each facility based on the context and resources available at the time of implementation and typically involved a phased evolution, beginning with preparation for TIER.Net, installation and training, back capturing, live capturing and finally a live site able to produce monthly and quarterly reports with staff on-site to manage it. In 2014, an estimated 3,000 out of 4,000 public sector clinics in South Africa were using TIER.Net (23, 24) in one of the three phases of implementation. As of 2017, TIER 3 was still in its pilot phase (25).
ART patient outcomes have evolved since the start of national HIV treatment programmes. In several cohort studies of ART programmes in sub-Saharan Africa, there have been reports of higher rates of LTFU among patients who initiated ART in later years compared to earlier years (26–28). This may be explained by patients increasingly initiating treatment while less severely ill (29), as well as a negative consequence of patient numbers increasing such as facility workloads (30), raising concerns about the sustainability of these programmes. Some systematic reviews have shown that the percentage of patients LTFU who have died has decreased in later years as eligibility criteria have evolved to include less immunologically compromised patients, and as the proportion of patients LTFU has increased (13, 31). Furthermore, scale-up and decentralization of these programmes means ART can be offered at clinics closer to patients' homes, which may serve as an incentive to self-transfer in order to continue treatment at more convenient locations (13, 32).
Unpublished TIER.Net analyses from 2018 showed LTFU rates to range from 11 to 15% in the first three months and from 27 to 34% in the first year of ART (Y.Pillay, HIV Think-tank update, March 19, 2019). The high percentages of LTFU present many issues. Firstly, if these patients have really stopped ART then they have a higher mortality risk (33–35), and are more likely to transmit HIV (36–39). Given that patients that are LTFU have poorer outcomes, LTFU can also through misclassification bias event rates such as mortality downwards (40), leading to biased performance indicators for ART programmes. Accurate mortality rates are also important as they are used as parameters for projections such as in the UNAIDS spectrum package (41, 42).
We compare patient outcomes recorded in TIER.Net to the outcomes ascertained through a record review and tracing study for patients deemed lost to follow-up in eight public sector health facilities in rural north-eastern South Africa. We aimed to assess misreporting in TIER.Net and potential biases in the national programme statistics reported from the TIER.Net database.
Methods
Setting
The Agincourt Health and Demographic Surveillance System (Agincourt HDSS) is located in rural north-eastern South Africa, in Mpumalanga province which has the second highest prevalence of HIV at 14.1% (43). HIV prevalence among people 15 years of age or older in the HDSS was estimated at 19.4% in 2010 (44). The Agincourt HDSS comprises of 31 villages covering an area of 475 square kilometers with an estimated population of 115,000 people (45, 46).
There are five primary health facilities and three secondary community health centers located within the Agincourt HDSS. Every HIV-positive patient has a clinical file that is opened when they first register at an ART clinic and updated at each clinic visit. Following the clinic visit, visit-level information from the patient file is entered into the national electronic database, TIER.Net. All health facilities routinely trace patients that are late for a scheduled clinic appointment. This tracing is done in conjunction with two non-profit organizations, Right to Care (RtC) and Home-Based Carers (HBC). Clinic staff must contact all patients first by phone and if this does not yield a satisfactory outcome, a home visit is organized. Patients are classified as lost to follow-up (LTFU) if they have not returned 90 days after their scheduled visit.
Demographic Surveillance
Data collection aims to capture all demographic events for the Agincourt HDSS population. Fertility, mortality and migration data are based on a comprehensive household registration system that has been in operation since 1992. Following the baseline demographic surveillance survey in 1992 and three update rounds until 1998, the site has conducted annual surveys since 1999 (45, 47–49). Trained fieldworkers visit each household and interview the most knowledgeable adult available. During the visit, individual-level information on all household members is checked and updated and any events that have occurred since the last census round are recorded. Starting in 2017, data have been collected utilizing an electronic data collection system using tablets (50).
Point-of-Contact Interactive Record Linkage (PIRL)
A key element of the data infrastructure for this study consists of HIV patient visit logs collected by a study fieldworker in the health facilities that provide ART in the area. This work started in April 2014 at seven government facilities and was extended in 2016 to include one additional health facility. In addition to logging patient visits, these records are linked to the Agincourt HDSS using a procedure that we have previously described as Point-of-Contact Interactive Record Linkage (PIRL) (51, 52). In brief, a fieldworker conducts a short uptake interview with patients in the waiting area of the clinic. Patients who consent are asked to declare a few personal identifiers that are used to search a local copy of the Agincourt HDSS database using a probabilistic algorithm. Matches are confirmed in interaction with the patients, and the names of other household members are used as a key attribute to adjudicate between possible matches.
Record Review and Tracing Study
Through the PIRL database, we identified patients who were more than 90 days late for a scheduled clinic appointment from HIV services on August 15, 2017 (the date of data extraction) at any of the eight health facilities located in the Agincourt HDSS area. These patients were recruited into a cohort and followed up to ascertain their treatment and vital status i.e., whether they were still alive.
All PLHIV aged 18 years or over, who had ever declared residency in Agincourt HDSS, and had enrolled in the HIV treatment and care programme since 2014 (after the Agincourt HDSS record linkage was established at the health facilities) were eligible for inclusion in the study.
Trained and supervised fieldworkers conducted a thorough record review, comparing the list of patients LTFU against (a) TIER.Net (b) patient clinic files, and (c) logbooks kept by the RtC and the HBC. The PIRL database was also reviewed for duplicate patients (different clinical records linking to the same individual in the Agincourt HDSS database, which was taken as evidence of silent transfers), and residency and vital status were extracted from the Agincourt HDSS database. This was done on a case-by-case basis.
HBC conducted a visit to the households of all patients for whom a definitive outcome (defined as death, transfer out, stopped ART, migrated, re-engaged in care, and alive with ART status unknown) could not be established, or for whom routine patient tracing was not done. Finally, all patients who remained LTFU after the HBC visit, were searched for in TIER.Net databases at clinics in close proximity to their home residence to capture any further silent transfers.
We also reviewed the records for a stratified random sample of 162 patient records who were not LTFU as of August 15, 2017, in order to assess whether TIER.Net misclassified any patients that were still in care. This sample was chosen to include 18 patients from every clinic (six men, six non-pregnant women, and six women who initiated ART while pregnant) with the exception of one clinic which had recently merged with another, and from which we sampled 18 patients who had enrolled whilst in each of the clinics prior to the merger.
Definitions
Definitions of terms used in this article are provided in Table 1.
Statistical Analyses
For patients included in the record review and tracing study, we calculated counts and proportions for socio-demographic and baseline clinical characteristics, TIER.Net treatment status, the final outcome, and cross-tabulated TIER.Net status and the final outcome.
To assess the degree and direction of mis-reporting of patient outcomes in TIER.Net, we graphically present TIER.Net treatment status and the final outcome proportions by some selected patient characteristics. A Pearson's chi-square test was used to compare whether TIER.Net treatment status and the final outcome varied by all the categorical variables. We also present a cross-tabulation of patient outcomes from the two sources.
A binary outcome variable was created to indicate whether TIER.Net had misclassified a patient's treatment status, with a second outcome created to identify whether the patient was recorded as LTFU in TIER.Net. All cases where an electronic record could not be found were removed from further analysis.
To explore factors associated with misclassification in TIER.Net, we ran bi-variate analyses with patient-level treatment characteristics, demographic characteristics and facility-level characteristics. All variables with p < 0.1 were included in the multivariable logistic regression model. A parsimonious model was achieved using Wald tests. This same procedure was followed in order to understand what factors were associated with being reported LTFU in TIER.Net. All analyses were conducted in Stata 15 (53) and all data visualization was done using R (54).
Ethics
Ethical approval was obtained from the London School of Hygiene and Tropical Medicine, the University of Witwatersrand and the Mpumalanga Department of health.
Results
Database Population Characteristics
Over the study period, 4,089 patients were added to the PIRL database and met the inclusion criteria. Of these 4,089, 1,325 (32.4%) met the LTFU criteria and were eligible for inclusion into the record review and tracing study. Of these 1,325 patients, 166 (12.5%) did not have an ART initiation date and were assumed to be pre-ART. Further investigation of these 166 patients found 46 (27.7%) had initiated ART after record linkage, 59 (35.5%) were genuine pre-ART patients and 61 (36.7%) had initiated ART before record linkage began. These 61 patients were excluded from further analyses. Of the remaining 1,264 patients, 190 (15.0%) were found to have data errors (mostly due to missing clinic visits in the PIRL database) and were excluded from further analysis (Supplementary Figure 1).
Of 1,074 remaining patients, 280 (26.1%) initiated ART for prevention of mother-to-child transmission (PMTCT), 737 (68.6%) met the ART initiation criteria for non-pregnant adults, and 57 (5.3%) had not initiated ART yet (pre-ART).
Thirteen (8.0%) of the 162 patients still in care were excluded from the analysis because they had not declared residency in the HDSS. The remaining 149 from the random sample of patients still in care were also assessed to see if misclassification also occurred for those who remained engaged in care (Table 2).
TIER.Net Treatment Status
Of the 1,074 patients who remained eligible for this analysis, 533 (49.6%) were categorized as LTFU, 222 (20.7%) as still in care, 186 (17.3%) as transferred out, 80 (7.5%) as deceased, and 53 (4.9%) could not be found in the TIER.Net database (Table 2).
There was a statistically significant difference (all p < 0.001) in the TIER.Net treatment status by sex, age, ART initiation status and reason, year of ART initiation, baseline CD4 count, time on ART, clinic visit schedule, health facility, and time since a missed appointment (see Supplementary Figure 2). Women who initiated ART for PMTCT were less likely to be categorized as deceased and more likely to be LTFU. All 149 patients sampled as still in care were also reported as still in care in TIER.Net.
Outcomes After Record Review and Tracing Study
Of the 1,074 patients who remained eligible for this analysis, 326 (30.3%) were found to have transferred to another clinic, 234 (21.8%) to have re-engaged in care, 132 (12.3%) were deceased, 117 (10.9%) were alive with ART status unknown, 81 (7.5%) were alive but not on treatment, 53 (4.9%) had migrated to another place of residence, and 131 (12.2%) were still LTFU (Table 3). These outcomes differed (all p < 0.001) by sex, age, ART initiation status and reason, baseline CD4 count, time on ART, clinic visit schedule, health facility, whether the patient record was successfully linked to an Agincourt HDSS record, and time since a missed appointment (some selected variables illustrated in Figure 1).
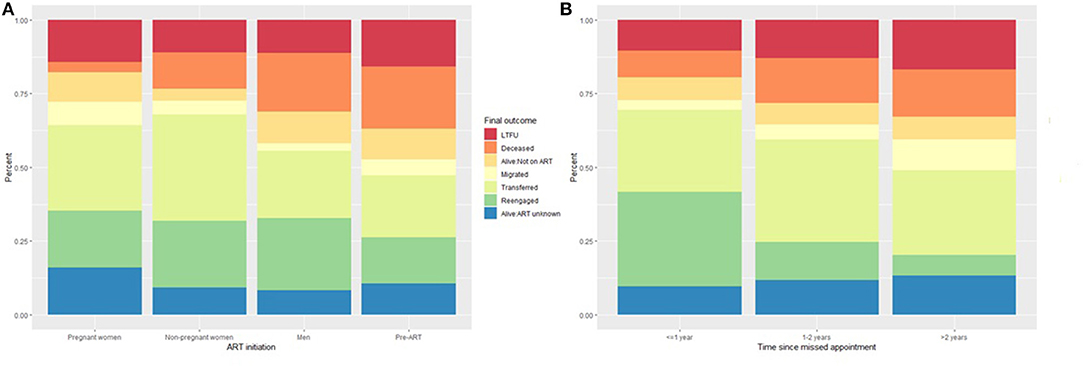
Figure 1. Outcome ascertained through record review and tracing stratified by (A) ART initiation status and (B) time since the last appointment.
Differences Between TIER.Net Treatment Status and Final Outcomes
Records of deceased or transferred out patients documented in TIER.Net aligned with patients' final outcome (i.e., no inaccuracies found for these two statuses). However, TIER.Net misclassified 52 (39.4%) of 132 deaths. Of these 52, 38 (73.1%) were classified as LTFU, 6 (11.5%) as still in care, and 8 (15.4%) were not found in the system at all.
TIER.Net also misclassified 53 patients as still in care. Of these, 10 (18.9%) were found to be LTFU, 16 (30.2%) to have transferred, 12 (22.6%) as alive with unknown ART status, 8 (15.1%) alive but not on treatment, 6 (11.3%) to have died, and 1 (1.9%) to have migrated to another place of residence. TIER.Net correctly captured 186 (57.1%) of 326 transfers.
Of 533 patients classified as LTFU by TIER.Net, 116 (21.8%) were found to have transferred to another clinic, 70 (13.1%) to be alive but not on treatment, 47 (8.8%) to have migrated to another place of residence, 38 (7.1%) to have died, and 56 (10.5%) to have re-engaged in care (38 of whom were resolved by new visit data in the PIRL database and so it is possible that their TIER.Net status could have also changed back to still in care) (Figure 2 and Supplementary Figure 3).
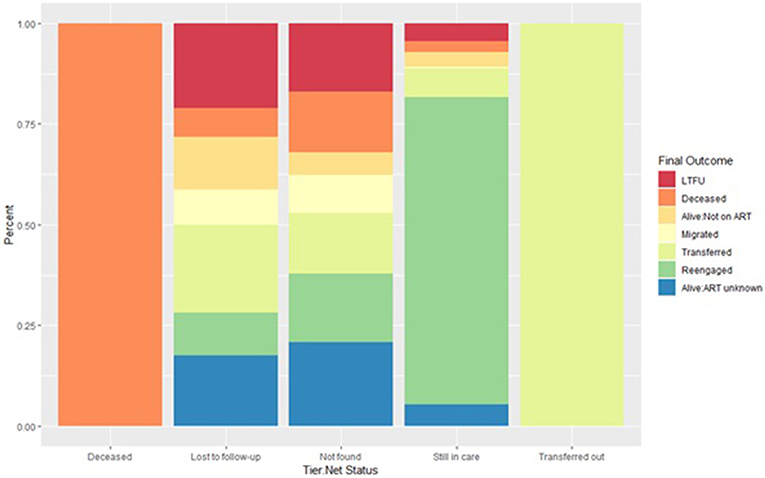
Figure 2. Outcome ascertained through record review and tracing stratified by each TIER.Net status. A deceased or transferred status in TIER.Net is accurate. Inaccuracies in the LTFU and still in care categories. Some patients categorized as still in care are LTFU, not currently on treatment, transfers out or dead.
As patients classified as LTFU in TIER.Net were more likely to be misclassified we report on the factors associated with being classified as LTFU in TIER.Net (Supplementary Data and Supplementary Table 1).
Factors Associated With Misclassification
In the multivariable model (Table 4), men (OR: 1.47, p = 0.021) had higher odds of misclassification when compared to women who initiated ART for non-PMTCT reasons (CD4, WHO stage, tuberculosis coinfection). Higher baseline CD4 (CD4 100-199 OR: 1.95. p = 0.002, CD4 ≥ 500 OR: 1.81, p = 0.014) was also associated with higher odds of misclassification when compared to patients who initiated treatment with CD4 <100. Health facility also remained statistically significant suggesting that facility level variability plays a role in misclassification. Patients who were linked to an Agincourt HDSS record in the PIRL database (OR: 2.09, p < 0.001) were more likely to be misclassified. Finally, patients who were between 1 and 2 years late (OR: 1.62, p = 0.001) were more likely to be misclassified. Older age (30–44 years OR: 0.73, p = 0.046, 45–59 years OR: 0.63, p = 0.046) was associated with lower odds of misclassification and patients on longer refill schedules (>3 months OR: 0.31, p = 0.009) were less likely to be misclassified.
Discussion
In this paper, we described the discrepancies between the treatment, vital and residency status of HIV patients enrolled in care between April 2014 and August 2017 in a rural South African setting as recorded in the national treatment database (TIER.Net) and their treatment outcome following a comprehensive record review and tracing study.
We found that TIER.Net misclassified 36% of the patient outcomes. ART initiation reason, baseline CD4, health facility attended, PIRL linkage, time since the last appointment, age, and ART refill schedule were all found to be significantly associated with misclassification. TIER.Net underestimated mortality and overestimated the number of patients who were LTFU. Seventy-nine percent of patients classified as LTFU in TIER.Net had a final outcome ascertained, mirroring findings from a systematic review of low and middle income country ART programmes which found that tracing generated higher estimates of mortality and lower estimates of LTFU (55). Our findings show that LTFU is still an important problem in ART programmes in this setting, even with routine patient tracing in place. TIER.Net also missed 43% of transfers with these silent transfers being the biggest contributor to misclassification among those documented as LTFU. We also found that 21.8% of patients had re-engaged in care, a phenomenon that was previously not well understood, but which is now increasingly recognized as becoming a common feature of ART programmes (55). Using our findings to revise LTFU figures to reflect re-engagement may help improve programme evaluation and forecasting.
In our study, we found that 40% of deaths were missed by TIER.Net, indicating that mortality of ART patients would be underestimated if relying on this data source. Given the role that national statistics play in HIV/AIDS projections (41, 42, 56), our findings suggest a need for correction factors for the estimates of the effect of ART on mortality. Although South Africa has a good vital registration system in place (57), these data are not currently linked to clinic-based information. However, with the move to registering patient national ID numbers, clinics should consider matching patients that are LTFU to the national death registry and other available databases such as the national health laboratory services database to ascertain vital status as this has proved useful in other studies in South Africa (58–60). Clinics in the Agincourt HDSS study area and other HDSS sites could also consider using vital status data from annual demographic surveillance to ascertain vital status for all patients.
The number of patient transfers to another clinic that were missed in TIER.Net suggests that communication between clinics is sub-optimal and that the current system for transferring patients between clinics can be improved. With studies reporting patient fear and concern about provider reactions if they return to care after a treatment interruption (61, 62) it is possible that some patients considered it less stressful to self-transfer or restart treatment at another nearby clinic, rather than returning to the facility where they had initiated treatment. These silent transfers could lead to double counting of patients currently on treatment, the second of the 90-90-90 targets, potentially suggesting that the programme is performing better than it is. Furthermore, given that the national treatment programme relies on data from TIER.Net to plan and procure ART based on active patient numbers, misclassification in the database, and more specifically double-counting due to silent transfers may lead to inaccurate drug forecasts and misestimation of medicines and other commodities at the national level. This bias will only increase as the South African ART programme expands with more patients potentially moving into new clinics closer to their homes and more people initiate ART with the move to test and treat. Future work will consider how application of correction factors from this research would change programme statistics and drug forecasts.
It is also important to consider the risk that silent transfers pose with regards to drug resistance, as this misclassifies treatment experienced patients as treatment naïve and may lead to patients being offered regimens that have lost their optimal therapeutic benefit. This is particularly concerning because resistance testing is not commonly used in these settings, and can potentially lead to increases in levels of transmitted drug resistance (63). Better referral systems, patient education, regular information exchange between clinics, and provider training (64), could improve recording of transfers and clinic staff attitudes toward less adherent patients. The WHO also recommends enforcement of unique identifiers as paramount to improve patient safety, improve the efficient use of programme resources by reducing duplications, and to improve programme monitoring and evaluation (65). With national IDs becoming mandatory at clinic registration, information exchange could prove useful in identifying silent transfers. This should also become less of an issue when TIER.Net is upgraded to a fully networked database.
We found several factors to be associated with misclassification of outcome in TIER.Net, with older age and longer ART refill schedules found to be protective factors. Older patients were less likely to be classified as LTFU in TIER.Net which probably explains why they were subsequently less likely to be misclassified. Given that longer ART refill schedules are synonymous with previous good adherence (66), these patients accounted for 11% of the patients LTFU and were also more likely to have re-engaged in care, a category that contributed very little to misclassification. Patients whose clinic record was successfully linked to an Agincourt HDSS record in the PIRL database were more likely to be misclassified. They were also more likely to have been resolved which could explain this association. Health facilities were also positively associated with misclassification, with the facilities with the highest proportion of patients classified as LTFU in TIER.Net being more likely to misclassify patients. Two of these clinics also had issues with routine tracing, with one clinic not undertaking any physical tracing at all, emphasizing an additional benefit of routine tracing. Finally, patients who had been LTFU for a longer duration were more likely to be classified as LTFU in TIER.Net, more likely to have transferred to another clinic, and less likely to have re-engaged in care which probably explains their higher likelihood of misclassification.
This analysis has several limitations. Firstly, TIER.Net was only consulted at a specific point in time. The cross-sectional nature of TIER.Net outcomes means that some may have changed, but we would have no way to ascertain this. We checked TIER.Net 12 months after the initial record review for all patients whose outcome after record review and tracing was still LTFU and 85% of the outcomes had not changed. However, for patients whose final outcome was resolved through new visit data in the PIRL database, it is likely that their TIER.Net outcome also changed. It is also possible that some of the patients categorized as LTFU in TIER.Net are due to the rigidity of the system as TIER.Net only allows for four possible outcomes; still in care, transferred out, LTFU and deceased (11, 67, 68). It is possible that for some patients, their outcomes were ascertained, but the rigidity meant that they could not be recorded in the database and may call for the inclusion of other possible outcomes in the database. The exclusion of patients for whom an electronic record could not be found from the multivariable analyses might bias our findings. However, given the relatively small number we expect that this bias is fairly small. Finally, we did not adjudicate causes of death, so it is possible that patients died from causes other than those related to HIV/AIDS. A strength of this study is that we attempted to trace all patients that were LTFU and not a sample. Therefore, the findings might be more generalizable to other settings. The multiple methods, data sources and levels of follow-up used to trace patients are also a strength.
In conclusion, although TIER.Net misclassified 36% of patient outcomes, this reflects the various challenges with the processes and upstream factors that lead to this misclassification and calls for their improvement rather than the utility of the database itself, as patients classified as LTFU were most likely to be misclassified. Clinics should consider training staff about ascertaining patient outcomes, putting more emphasis into patient tracing and using other data sources such as the national death register to improve ascertainment of patient treatment outcomes. For policy and planning purposes, programme evaluators should consider using correction factors to improve the accuracy of estimates from TIER.Net.
Data Availability Statement
The data used in these analyses are not yet publicly available as they currently being utilized for the first author's Ph.D. research. They will be deposited with the Agincourt HDSS data manager and made available on request at the end of his Ph.D. in 2021. Data from the PIRL database are also available by making a data request to the Agincourt HDSS data manager.
Ethics Statement
Ethical approval was obtained from the London School of Hygiene and Tropical Medicine, the University of Witwatersrand and the Mpumalanga Department of health.
Author Contributions
The study was conceived by DE, AW, and GR. Fieldwork was planned and executed by DE, FG-O, and CK. Data collection was supervised by DE. Analyses were conducted by DE with input from all authors. The manuscript was drafted by DE with input from BR, JR and all the other authors. All authors contributed to the interpretation of the findings and read and approved the final manuscript.
Funding
This study was made possible with support from the Economic and Social Research Council (ES/JS00021/1), the Bill and Melinda Gates Foundation for the MeSH Consortium (OPP1120138), the Bill and Melinda Gates Foundation ALPHA grant (OPP1164897), and the MRC SHAPE UTT grant (MR/P014313/1).
Conflict of Interest
The authors declare that the research was conducted in the absence of any commercial or financial relationships that could be construed as a potential conflict of interest.
The reviewer PA declared a shared affiliation, with no collaboration, with the authors to the handling editor at the time of review.
Acknowledgments
The authors would like to thank all the participants in the study.
Supplementary Material
The Supplementary Material for this article can be found online at: https://www.frontiersin.org/articles/10.3389/fpubh.2020.00100/full#supplementary-material
References
1. WHO. Data Statistics. WHO. Available online at: http://www.who.int/hiv/data/en/ (accessed February 23, 2019).
2. AIDSinfo. UNAIDS. Available online at: http://aidsinfo.unaids.org/ (accessed October 11, 2018).
3. Tanser F, Barnighausen T, Grapsa E, Zaidi J, Newell M-L. High coverage of ART associated with decline in risk of HIV acquisition in Rural KwaZulu-Natal, South Africa. Science. (2013) 339:966–71. doi: 10.1126/science.1228160
4. Joint United Nations Programme on HIV/AIDS. Fast-Track: Ending the AIDS Epidemic by 2030. (2014). Available online at: http://www.unaids.org/sites/default/files/media_asset/JC2686_WAD2014report_en.pdf (accessed March 14, 2019).
5. Sidibé M, Loures L, Samb B. The UNAIDS 90-90-90 target: a clear choice for ending AIDS and for sustainable health and development. J Int AIDS Soc. (2016) 19:21133. doi: 10.7448/IAS.19.1.21133
6. Rabkin M, El-Sadr WM, De Cock KM, Bellagio HIV/Health Systems Working Group1. The impact of HIV scale-up on health systems: a priority research agenda. J Acquir Immune Defic Syndr 1999. (2009) 52 (Suppl. 1):S6–11. doi: 10.1097/QAI.0b013e3181bbcd69
7. Nash D, Elul B, Rabkin M, Tun M, Saito S, Becker M, et al. Strategies for more effective monitoring and evaluation systems in HIV programmatic scale-up in resource-limited settings: implications for health systems strengthening. J Acquir Immune Defic Syndr 1999. (2009) 52 (Suppl. 1):S58–62. doi: 10.1097/QAI.0b013e3181bbcc45
8. Mate KS, Bennett B, Mphatswe W, Barker P, Rollins N. Challenges for routine health system data management in a large public programme to prevent mother-to-child HIV transmission in South Africa. PLoS ONE. (2009) 4:e5483. doi: 10.1371/journal.pone.0005483
9. Maher D. The power of health information–the use of cohort monitoring in managing patients with chronic non-communicable diseases. Trop Med Int Health TM IH. (2012) 17:1567–8. doi: 10.1111/j.1365-3156.2012.03094.x
10. Gourlay A, Wringe A, Todd J, Michael D, Reniers G, Urassa M, et al. Challenges with routine data sources for PMTCT programme monitoring in East Africa: insights from Tanzania. Glob Health Action. (2015) 8:29987. doi: 10.3402/gha.v8.29987
11. Osler M, Hilderbrand K, Hennessey C, Arendse J, Goemaere E, Ford N, et al. A three-tier framework for monitoring antiretroviral therapy in high HIV burden settings. J Int AIDS Soc. (2014) 17:18908. doi: 10.7448/IAS.17.1.18908
12. Douglas GP, Gadabu OJ, Joukes S, Mumba S, McKay MV, Ben-Smith A, et al. Using touchscreen electronic medical record systems to support and monitor national scale-up of antiretroviral therapy in malawi. PLoS Med. (2010) 7:e1000319. doi: 10.1371/journal.pmed.1000319
13. Wilkinson LS, Skordis-Worrall J, Ajose O, Ford N. Self-transfer and mortality amongst adults lost to follow-up in ART programmes in low- and middle-income countries: systematic review and meta-analysis. Trop Med Int Health TM IH. (2015) 20:365–79. doi: 10.1111/tmi.12434
14. Geng EH, Odeny TA, Lyamuya R, Nakiwogga-Muwanga A, Diero L, Bwana M, et al. Retention in care and patient-reported reasons for undocumented transfer or stopping care among HIV-infected patients on antiretroviral therapy in Eastern Africa: application of a sampling-based approach. Clin Infect Dis. (2016) 62:935–44. doi: 10.1093/cid/civ1004
15. Tassie J-M, Malateste K, Pujades-Rodríguez M, Poulet E, Bennett D, Harries A, et al. Evaluation of three sampling methods to monitor outcomes of antiretroviral treatment programmes in low- and middle-income countries. PLoS ONE. (2010) 5:e13899. doi: 10.1371/journal.pone.0013899
16. Navario P. PEPFAR's biggest success is also its largest liability. Lancet. (2009) 374:184–5. doi: 10.1016/S0140-6736(09)61312-X
17. Forster M, Bailey C, Brinkhof MWG, Graber C, Boulle A, Spohr M, et al. Electronic medical record systems, data quality and loss to follow-up: survey of antiretroviral therapy programmes in resource-limited settings. Bull World Health Organ. (2008) 86:939–47. doi: 10.2471/BLT.07.049908
18. WHO. A Universal Truth: No Health Without a Workforce. (2014). Available online at: http://www.who.int/workforcealliance/knowledge/resources/GHWA-a_universal_truth_report.pdf (accessed February 23, 2019).
19. Hoyler M, Finlayson SRG, McClain CD, Meara JG, Hagander L. Shortage of doctors, shortage of data: a review of the global surgery, obstetrics, and anesthesia workforce literature. World J Surg. (2014) 38:269–80. doi: 10.1007/s00268-013-2324-y
20. Okyere E, Mwanri L, Ward P. Is task-shifting a solution to the health workers' shortage in Northern Ghana? PLoS ONE. (2017) 12:e0174631. doi: 10.1371/journal.pone.0174631
21. Sprague C, Chersich MF, Black V. Health system weaknesses constrain access to PMTCT and maternal HIV services in South Africa: a qualitative enquiry. AIDS Res Ther. (2011) 8:10. doi: 10.1186/1742-6405-8-10
22. Katurura MC, Cilliers L. Electronic health record system in the public health care sector of South Africa: A systematic literature review. Afr J Prim Health Care Fam Med. (2018) 10:e1–8. doi: 10.4102/phcfm.v10i1.1746
23. UCT Team's HIV-Monitoring Software Exported. Available online at: http://www.news.uct.ac.za/article/-2014-09-22-uct-teams-hiv-monitoring-software-exported (accessed February 23, 2019).
24. Mafika SA Team Develops HIV Monitoring Tool. Brand South Africa (2014). Available online at: https://www.brandsouthafrica.com/investments-immigration/science-technology/hiv-register-290914 (accessed February 23, 2019).
25. Skiti V. Qualitative Assessment of the Utilisation of Tier.Net Health Information Among Facility And Programme Managers In Ekurhuleni District, Gauteng. (2017). Available online at: http://etd.uwc.ac.za/xmlui/bitstream/handle/11394/5942/Skiti%20ecos.pdf?sequence=1 (accessed February 23, 2019).
26. Mutevedzi PC, Lessells RJ, Newell M-L. Disengagement from care in a decentralised primary health care antiretroviral treatment programme: cohort study in rural South Africa. Trop Med Int Health TM IH. (2013) 18:934–41. doi: 10.1111/tmi.12135
27. Koole O, Kalenga L, Kiumbu M, Menten J, Ryder RW, Mukumbi H, et al. Retention in a NGO supported antiretroviral program in the Democratic Republic of Congo. PLoS ONE. (2012) 7:e40971. doi: 10.1371/journal.pone.0040971
28. Ambia J, Kabudula C, Risher K, Rice BD, Reniers G, Etoori D. Outcomes of patients lost to follow-up after antiretroviral therapy initiation in rural north-eastern South Africa. Trop Med Int Health TM IH. (2019) 24:747–56. doi: 10.1111/tmi.13236
29. Kerschberger B, Schomaker M, Ciglenecki I, Pasipamire L, Mabhena E, Telnov A, et al. Programmatic outcomes and impact of rapid public sector antiretroviral therapy expansion in adults prior to introduction of the WHO treat-all approach in rural Eswatini. Trop Med Int Health TM IH. (2019) 24:701–14. doi: 10.1111/tmi.13234
30. Johnson LF, Estill J, Keiser O, Cornell M, Moolla H, Schomaker M, et al. Do increasing rates of loss to follow-up in antiretroviral treatment programs imply deteriorating patient retention? Am J Epidemiol. (2014) 180:1208–12. doi: 10.1093/aje/kwu295
31. Brinkhof MWG, Pujades-Rodriguez M, Egger M. Mortality of patients lost to follow-up in antiretroviral treatment programmes in resource-limited settings: systematic review and meta-analysis. PLoS ONE. (2009) 4:e5790. doi: 10.1371/journal.pone.0005790
32. Auld AF, Mbofana F, Shiraishi RW, Sanchez M, Alfredo C, Nelson LJ, et al. Four-year treatment outcomes of adult patients enrolled in Mozambique's rapidly expanding antiretroviral therapy program. PLoS ONE. (2011) 6:e18453. doi: 10.1371/journal.pone.0018453
33. Kranzer K, Ford N. Unstructured treatment interruption of antiretroviral therapy in clinical practice: a systematic review. Trop Med Int Health TM IH. (2011) 16:1297–313. doi: 10.1111/j.1365-3156.2011.02828.x
34. Strategies for Management of Antiretroviral Therapy (SMART) Study Group, El-Sadr WM, Lundgren JD, Neaton JD, Gordin F, Abrams D, et al. CD4+ count-guided interruption of antiretroviral treatment. N Engl J Med. (2006) 355:2283–96. doi: 10.1056/NEJMoa062360
35. Ananworanich J, Gayet-Ageron A, Le Braz M, Prasithsirikul W, Chetchotisakd P, Kiertiburanakul S, et al. CD4-guided scheduled treatment interruptions compared with continuous therapy for patients infected with HIV-1: results of the Staccato randomised trial. Lancet Lond Engl. (2006) 368:459–65. doi: 10.1016/S0140-6736(06)69153-8
36. Hoffman RM, Black V, Technau K, van der Merwe KJ, Currier J, Coovadia A, et al. Effects of highly active antiretroviral therapy duration and regimen on risk for mother-to-child transmission of HIV in Johannesburg, South Africa. J Acquir Immune Defic Syndr 1999. (2010) 54:35–41. doi: 10.1097/QAI.0b013e3181cf9979
37. Eyawo O, de Walque D, Ford N, Gakii G, Lester RT, Mills EJ. HIV status in discordant couples in sub-Saharan Africa: a systematic review and meta-analysis. Lancet Infect Dis. (2010) 10:770–7. doi: 10.1016/S1473-3099(10)70189-4
38. Donnell D, Baeten JM, Kiarie J, Thomas KK, Stevens W, Cohen CR, et al. Heterosexual HIV-1 transmission after initiation of antiretroviral therapy: a prospective cohort analysis. Lancet Lond Engl. (2010) 375:2092–8. doi: 10.1016/S0140-6736(10)60705-2
39. Myer L, Phillips TK. Beyond “Option B+”: understanding antiretroviral therapy (ART) adherence, retention in care and engagement in art services among pregnant and postpartum women initiating therapy in Sub-saharan Africa. J Acquir Immune Defic Syndr. (2017) 75(Suppl 2):S115–22. doi: 10.1097/QAI.0000000000001343
40. Kristman V, Manno M, Côté P. Loss to follow-up in cohort studies: how much is too much? Eur J Epidemiol. (2004) 19:751–60. doi: 10.1023/B:EJEP.0000036568.02655.f8
41. Stover J. AIM: A Computer Program for Making HIV/AIDS Projections and Examining the Demographic and Social Impacts of AIDS. USAID (2007). Available online at: http://data.unaids.org/pub/manual/2007/aim_manual_2007_en.pdf (accessed February 29, 2019).
42. Ghys PD, Brown T, Grassly NC, Garnett G, Stanecki KA, Stover J, et al. The UNAIDS Estimation and Projection Package: a software package to estimate and project national HIV epidemics. Sex Transm Infect. (2004) 80 (Suppl 1):i5–9. doi: 10.1136/sti.2004.010199
43. HIV statistics for South Africa. Prevalence, Incidence, ARVs, Deaths. TB Facts. TB, Tests, Drugs, Statistics. Available online at: https://www.tbfacts.org/hiv-statistics-south-africa/ (accessed February 23, 2019).
44. Gómez-Olivé FX, Angotti N, Houle B, Klipstein-Grobusch K, Kabudula C, Menken J, et al. Prevalence of HIV among those 15 and older in rural South Africa. AIDS Care. (2013) 25:1122–8. doi: 10.1080/09540121.2012.750710
45. Kahn K, Collinson MA, Gómez-Olivé FX, Mokoena O, Twine R, Mee P, et al. Profile: Agincourt health and socio-demographic surveillance system. Int J Epidemiol. (2012) 41:988–1001. doi: 10.1093/ije/dys115
46. Kabudula CW, Houle B, Collinson MA, Kahn K, Tollman S, Clark S. Assessing changes in household socioeconomic status in rural south africa, 2001–2013: a distributional analysis using household asset indicators. Soc Indic Res. (2017) 133:1047–73. doi: 10.1007/s11205-016-1397-z
47. Tollman SM. The Agincourt field site–evolution and current status. South Afr Med J Suid-Afr Tydskr Vir Geneeskd. (1999) 89:853–8.
48. Tollman SM, Herbst K, Garenne M, Gear JS, Kahn K. The Agincourt demographic and health study–site description, baseline findings and implications. South Afr Med J Suid-Afr Tydskr Vir Geneeskd. (1999) 89:858–64.
49. Tollman S, Kahn K. Report on the meeting 'Strengthening ties: the Agincourt field site in its African context. Trop Med Int Health. (1997) 2:920–3. doi: 10.1046/j.1365-3156.1997.d01-424.x
50. Data Collection - MRC/Wits Agincourt Unit. Available online at: https://www.agincourt.co.za/?page_id=1805 (accessed April 2, 2019).
51. Rentsch CT, Kabudula CW, Catlett J, Beckles D, Machemba R, Mtenga B, et al. Point-of-contact Interactive Record Linkage (PIRL): A software tool to prospectively link demographic surveillance and health facility data. Gates Open Res [Internet]. (2017) 1:8. doi: 10.12688/gatesopenres.12751.1
52. Kabudula CW, Clark BD, Gómez-Olivé FX, Tollman S, Menken J, Reniers G. The promise of record linkage for assessing the uptake of health services in resource constrained settings: a pilot study from South Africa. BMC Med Res Methodol. (2014) 14:71. doi: 10.1186/1471-2288-14-71
54. R Core Team. R: A language and Environment for Statistical Computing. Vienna: R Foundation for Statistical Computing (2019). Available online at: https://www.R-project.org (accessed January 28, 2020).
55. McMahon JH, Elliott JH, Hong SY, Bertagnolio S, Jordan MR. Effects of physical tracing on estimates of loss to follow-up, mortality and retention in low and middle income country antiretroviral therapy programs: a systematic review. PLoS ONE. (2013) 8:e56047. doi: 10.1371/journal.pone.0056047
56. Stover J. Projecting the demographic consequences of adult HIV prevalence trends: the Spectrum Projection Package. Sex Transm Infect. (2004) 80 (Suppl. 1):i14–8. doi: 10.1136/sti.2004.010157
57. Statistics South Africa. Mortality and Causes of Death in South Africa, 2016: Findings From Death Notification. (2018). Available online at: http://www.statssa.gov.za/publications/P03093/P030932016.pdf (accessed February 27, 2019).
58. Van Cutsem G, Ford N, Hildebrand K, Goemaere E, Mathee S, Abrahams M, et al. Correcting for mortality among patients lost to follow up on antiretroviral therapy in South Africa: a cohort analysis. PLoS ONE. (2011) 6:e14684. doi: 10.1371/journal.pone.0014684
59. Fox MP, Brennan A, Maskew M, MacPhail P, Sanne I. Using vital registration data to update mortality among patients lost to follow-up from ART programs: evidence from the themba lethu clinic, South Africa. Trop Med Int Health TM IH. (2010) 15:405–13. doi: 10.1111/j.1365-3156.2010.02473.x
60. Clouse K, Vermund SH, Maskew M, Lurie MN, MacLeod W, Malete G, et al. Mobility and clinic switching among postpartum women considered lost to HIV care in South Africa. J Acquir Immune Defic Syndr 1999. (2017) 74:383–9. doi: 10.1097/QAI.0000000000001284
61. Tweya H, Feldacker C, Estill J, Jahn A, Ng'ambi W, Ben-Smith A, et al. Are they really lost? “true” status and reasons for treatment discontinuation among HIV infected patients on antiretroviral therapy considered lost to follow up in Urban Malawi. PLoS ONE. (2013) 8:e75761. doi: 10.1371/journal.pone.0075761
62. Ware NC, Wyatt MA, Geng EH, Kaaya SF, Agbaji OO, Muyindike WR, et al. Toward an understanding of disengagement from HIV treatment and care in sub-Saharan Africa: a qualitative study. PLoS Med. (2013) 10:e1001369; discussion e1001369. doi: 10.1371/journal.pmed.1001369
63. Castro H, Pillay D, Sabin C, Dunn DT, UK Collaborative Group on HIV Drug Resistance. Effect of misclassification of antiretroviral treatment status on the prevalence of transmitted HIV-1 drug resistance. BMC Med Res Methodol. (2012) 12:30. doi: 10.1186/1471-2288-12-30
64. Egger M, Spycher BD, Sidle J, Weigel R, Geng EH, Fox MP, et al. Correcting mortality for loss to follow-up: a nomogram applied to antiretroviral treatment programmes in sub-Saharan Africa. PLoS Med. (2011) 8:e1000390. doi: 10.1371/journal.pmed.1000390
65. World Health Organisation. Consolidated Guidelines on Person-Centred HIV Patient Monitoring and Case Surveillance. (2017). Available online at: https://www.who.int/hiv/pub/guidelines/person-centred-hiv-monitoring-guidelines/en/ (accessed February 26, 2020).
66. Giving Stable HIV Treatment Patients a 3-Month Prescription Refill of ARV Drugs at One Time Reduces the Need for Frequent Trips to the Clinic. Clinton Health Access Initiative (2017). Available online at: https://clintonhealthaccess.org/giving-stable-hiv-treatment-patients-3-month-prescription-refill-arv-drugs-one-time-reduces-need-frequent-trips-clinic/ (accessed April 8, 2019).
67. Center for Infectious Diseases Epidemiology and Research. Tier.Net: HIV Electronic Register User Guide. Cape Town: University of Cape Town (2011).
68. Osler M, Boulle A. Three Interlinked Electronic Registers (TIER.Net) Project. (2010). Available online at: http://www.publichealth.uct.ac.za/sites/default/files/image_tool/images/108/TIER.Net%20%5B03%20Nov%202010%5D.pdf (accessed February 26, 2019).
Keywords: HIV, retention in care, bias, South Africa, health information systems
Citation: Etoori D, Wringe A, Kabudula CW, Renju J, Rice B, Gomez-Olive FX and Reniers G (2020) Misreporting of Patient Outcomes in the South African National HIV Treatment Database: Consequences for Programme Planning, Monitoring, and Evaluation. Front. Public Health 8:100. doi: 10.3389/fpubh.2020.00100
Received: 25 April 2019; Accepted: 12 March 2020;
Published: 07 April 2020.
Edited by:
Marc Jean Struelens, European Centre for Disease Prevention and Control (ECDC), SwedenReviewed by:
Philip Ayieko, University of London, United KingdomChristen Ann Said, University of California, San Francisco, United States
Deborah K. Glencross, University of the Witwatersrand, South Africa
Copyright © 2020 Etoori, Wringe, Kabudula, Renju, Rice, Gomez-Olive and Reniers. This is an open-access article distributed under the terms of the Creative Commons Attribution License (CC BY). The use, distribution or reproduction in other forums is permitted, provided the original author(s) and the copyright owner(s) are credited and that the original publication in this journal is cited, in accordance with accepted academic practice. No use, distribution or reproduction is permitted which does not comply with these terms.
*Correspondence: David Etoori, ZGF2aWQuZXRvb3JpQGxzaHRtLmFjLnVr