- 1Statistics Online Computational Resource, Department of Health Behavior and Biological Sciences, University of Michigan, Ann Arbor, MI, United States
- 2Neuroscience Graduate Program, University of Michigan, Ann Arbor, MI, United States
- 3Michigan Institute for Data Science, University of Michigan, Ann Arbor, MI, United States
- 4Department of Computational Medicine and Bioinformatics, University of Michigan, Ann Arbor, MI, United States
This perspective provides a rationale for redesigning and a framework for expanding the graduate health science analytics and biomedical doctoral program curricula. It responds to digital revolution pressures, ubiquitous proliferation of big biomedical data, substantial recent advances in scientific technologies, and rapid progress in health analytics. Specifically, the paper presents a set of common prerequisites, a proposal for core computational and data analytic curriculum, and a list of expected outcome competencies for graduates of doctoral health science and biomedical programs. The manuscript emphasizes the necessity for coordinated efforts of all stakeholders, including trainees, educators, academic institutions, funding agencies, and policy makers. Concrete recommendations are presented of how to ensure graduates with terminal health science analytics and biomedical degrees are trained and able to continuously self-learn, effectively communicate across disciplines, and promote adaptation and change to counteract the relentless pace of automation and the law of diminishing returns.
Background
Rapid advances in biomedical research and health science discoveries impact all human experiences. Further progress in this extremely interdisciplinary field requires reexamining policies, funding mechanisms, institutional organizations, graduate education, and training curricula, as well as financial incentives and distribution of limited health resources and services. Future graduates of quantitative biomedical and health science analytics graduate programs will play important roles in legislation, population-wide healthcare policies, and the economic, social, and behavioral determinants of human health. Recent computational, data science, and communication breakthroughs present an opportunity to review and enhance the terminal biomedical and health curricula by adopting modern scientific methods, embracing artificial intelligence analytical strategies, and emphasizing reproducible one-science computational protocols.
Graduates of doctoral biomedical and health science programs should be prepared to continuously self-learn, play active roles in research, participate in health policy, and engage in transdisciplinary collaborations. To be successful in these endeavors, a level of prerequisites is required prior to enrollment in the programs, and the expectations of freshly minted scholars should include technical competencies and transdisciplinary skills to ensure their long-term career success.
Key Points
• None of the health science disciplines (e.g., medicine, nursing, pharmacy, kinesiology, public health, bioinformatics, and genetics) are insular (1–3). Transdisciplinary training and inter-professional education are critical for ethical, collaborative, and reproducible research of complex biomedical and health conditions (4, 5).
• The digital revolution demands substantial quantitative skills, data-literacy, and analytical competence: health science doctoral programs need to be revised and expanded to build basic-science (STEM) expertise, emphasize team-science, rely on holistic understanding of biomedical systems and health challenges, and amplify dexterous abilities to handle, interrogate, and interpret complex multisource information (6–8).
• Exploratory, classification, and predictive Big Data analytics are all pivotally important and complementary to traditional hypothesis-driven confirmatory analyses (9–12).
Why Is Curriculum Redesign Important?
• Graduates of health science doctoral programs may not be fully prepared to participate in, or lead, transdisciplinary translational research projects involving complex information and heterogeneous observations (13–16).
• The range of quantitative expertise in computational and data sciences varies substantially within and between programs (17, 18).
• The NIH-wide 2016–2020 Strategic Plan calls for core data science training and the need for “quantitative and analytical approaches, processes, and systems … to extract knowledge and insights from increasingly large and/orcomplex sets of data” (https://datascience.nih.gov/sites/default/files/NIH_Strategic_Plan_for_Data_Science_Final_508.pdf).
• In health sciences, having a STEM foundation knowledge, possessing high technical skills, and having the abilities to gather, model, process, and interpret large amounts of heterogeneous, multi-source, and time-varying information is gold. The golden rule in advanced evidence-driven patient care, effective biomedical research, and transformative health science is simple: whoever has the gold will make the rules, control the direction of health science, and dominate the translational biomedical research impact. This golden rule is more pertinent to teams of investigators, rather than individual scientists, although the latter form the building blocks of all highly effective teams (19, 20).
Targeted Trainees
The extremely wide range of graduate biomedical, informatics, and health analytics training programs is a direct reflection of the disruptive nature of network-science based discovery, technological advances, and accelerated data-driven innovation (21–23). This manuscript addresses the need for educating and training a very specific cohort of data-savvy quantitative scholars pursuing terminal research-intensive degrees in biomedical and health sciences. Examples of such trainees include students enrolled in doctoral programs in health informatics, biomedical informatics, biostatistics, human genetics, data science, biomathematics, applied statistics, biomedical engineering, pharmacogenomics, and health analytics. This paper does not reflect on the curricular demands, or the quantitative training, of physicians, practicing clinicians, qualitative biosocial scholars, or licensed healthcare providers who are primarily focused on healthcare delivery. At the same time, some of the proposed technical training may be very appropriate for such practitioners as it will allow them to acquire additional skills, promote effective translation of STEM science and advanced analytics into clinical practice, and potentially improve health outcomes, job satisfaction, and patient experiences. Just like quantitative data scientists must possess dexterous artistic skills (8), it's reasonable to assume that exceptional clinicians will have functional quantitative abilities, and productive biomedical scholars would have basic anatomical and health training.
Big Biomedical and Health Data
Characteristics of Big Health Data
Over a decade ago, academic and IBM researchers introduced the qualifying notion of 3Vs of Big Data (volume, velocity, and variety), which later was expanded to 7Vs by adding veracity, variability, value, and visualization (24–26). This earlier framework provided a qualitative formulation expressing challenges related to the emergence and deluge of big biomedical and health data. Our more quantitative approach is formulated by examining dozens of challenging contemporary biomedical case-studies involving complex biomedical and healthcare datasets. There are seven dimensions of Big Biomedical and Health Data–size, format complexity, observation heterogeneity, incompleteness, spatiotemporal variability, multisource components, and multiscale resolution (9, 27). As a proxy of the underlying complex biological, physiological, and medical conditions, such data are important to understand the causes of morbid conditions, model associations between factors, predict risks of treatments, and forecast clinically relevant outcomes. Examples of big biomedical datasets include the UK Biobank (UKBB) (28–30), the Human Connectome Project (HCP) (31, 32), and the Alzheimer's Disease Neuroimaging Initiative (ADNI) (33, 34). UKBB represents a survey of a large population-based cohort including about 500 K individuals assessed at 22 UK medical centers in UK between 2006 and 2010. National Health Service recipients were invited to participate in UKBB and included individuals mostly between 40 and 69 years old (30, 35). HCP includes behavioral data, clinical phenotypes, and unprecedented high-resolution multimodal neuroimaging data for over 1,000 young adults (36). ADNI collected serial data for several thousands of participants including imaging (e.g., sMRI/fMRI, dMRI, PET), biological markers, clinical, genetics, cognitive, and neuropsychological assessment to measure the disease progression from normal aging to mild cognitive impairment (MCI) and early dementia (33). All of these large-scale studies face a number of challenges like balancing the (large) sample sizes with (small) effect sizes, incongruences, heterogeneity, time variability, and confounding effects. Once such datasets are represented as computable objects, data analytical strategies to extract valuable information and build actionable knowledge include model-based prediction vs. model-free inference, multiple comparison problems, and reproducibility (12, 27, 37).
Successes and Failures
Innovation is by definition uncertain and risky! The future of biomedical and health science discovery is bright and there are bound to be spectacular failures as well as breathtaking triumphs. Skeptics may point that major challenges of big data-driven transdisciplinary discoveries include communication barriers and the potential for bias inherent to dealing with complex and voluminous information. Others may argue that the quantity of observed data may obfuscate the key scientific questions transforming the traditional hypothesis-based (confirmatory) research based on a priori observations and inquiries into a new paradigm of data-driven inference, empirical knowledge derivation, and the formulation of novel hypotheses. The 2011 Google Flu Trends (GFT) report (38) was an example where GFT prediction problems were identified in 2013 (39) and partially attributed to overfitting. The GFT original report intended to predict future doctor office visits associated with influenza-like illness, which can be compared to the corresponding flu cases reported by the Centers for Disease Control and Prevention (CDC). In February 2013, independent investigators reported significantly higher GFT-predictions relative to the CDC forecast over the same period of time. The GFT model, which was built on 50-million web search terms over 1,152 data points, predicted increased likelihood of web-search terms matching the propensity of the flu. This may be explained by structurally unrelated queries that may have artificially inflated GFT predictions.
There have also been a number of mind-boggling reports representing successful transdisciplinary work that was only possible using enormous amounts of data interrogated by teams of scientists with broad and deep domain expertise using artificial intelligence (40). For instance, BANDIT (Bayesian ANalysis to determine Drug Interaction Targets), represents a novel data-driven paradigm for target identification and drug discovery using multisource big data in a Bayesian machine-learning framework (41). Applying BANDIT on 2,000 different small molecules, scientists identified likely targets and achieved predictive accuracy of 90%, which was an improvement of prior published target identifications. Similarly, a handful of small molecules with no known targets yielded 4,000 new molecule-target predictions. This target identification along with experimental validation using a set of microtubule inhibitors suggested three candidate compounds for cancer cells resistant to state-of-the-art clinical anti-microtubule treatment. Another example of successful biomedical and health application of transdisciplinary strategies to interrogate big data includes machine-learning techniques. To determine the top determinants of a health outcome, researchers discovered interesting combinations of indicators that affect health outcomes (e.g., life expectancy and anxiety disorders) and identified subpopulations representing analogous clinical phenotypes (42). A 2017 Kaggle Data Science Bowl competition offered $1M prize to a team that improved the specificity of automatic lung nodule characterization to improve screening mammography accuracy (43). Fusion algorithms and computational intelligence were used to efficiently process and visualize 40 GB of data in 10-min (44). Patient-centric eHealth ecosystems provide multi-layer architectures integrating connected devices, computing interfaces, and Cloud services to empower handling of complex data and ensure privacy (45).
Outside biomedical and health science, a recent data-driven discovery used partial differential equations to model large-scale time series measurements in Eulerian (spatially fixed sensors) or Lagrangian (dynamically moving sensors) frameworks. The model distinguishes between linear and Korteweg-de Vries equations, and enables discovery of the physical laws and the corresponding parametric spatiotemporal equations where derivations from first-principle derivations may be challenging (46).
Analytics Health Science Curriculum
Contemporary health science methods and analytics curricula are somewhat out of step with the accelerated scientific and technological advances in the twenty first century. Modernizing the graduate health science education and training will require substantial efforts to blend quantitative computational and data science methods with qualitative approaches, research ethics, and reproducible open science principles. The Data Science and Predictive Analytics (DSPA) course1 provides one complete, openly-accessible, and technology-enhanced example of an advanced quantitative graduate course for health sciences.
Prerequisites
There are expected variations between different biomedical and health science doctoral programs. Student backgrounds, career interests, motivations, expectations, and learning styles present additional levels of anticipated disparities. Although neither necessary nor sufficient, the prerequisites listed in Table 1 serve as a guideline of the foundational knowledge and prior experience that provide the basis for successful completion of a solid quantitative doctoral program in the biomedical and health sciences.
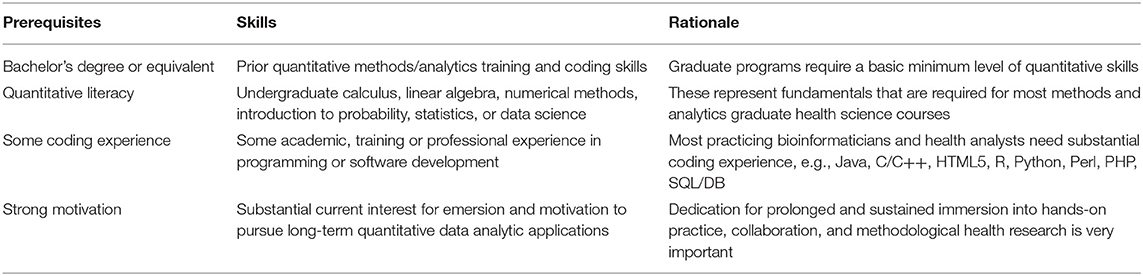
Table 1. Examples of prerequisites for strong biomedical and health sciences quantitative doctoral programs.
Potential trainees that have insufficient prior domain expertise, e.g., in college-level mathematics, numerical methods, probabilistic modeling, statistical analysis, or software programming, may need to complete relevant bootcamps or remediation coursework prior to matriculation. A wide range of MOOCs may provide the necessary prerequisites, e.g., Coursera, EdX, Khan Academy, Udacity. Examples of remediation courses provided to satisfy some of the Data Science and Predictive Analytics (DSPA) prerequisites are included in the DSPA self-assessment (pretest).
Core Curriculum
Indeed, each Institution and each quantitative biomedical or health sciences doctoral program will have their own customized curricula. At the same time, certain types of fundamental topics are expected to be common and share core principles, coverage, and methods. Table 2 illustrates examples of types of computational and data science courses that graduate students2 at any of the 12 disciplines part of the Program in Biomedical Sciences (PIBS)3 at the University of Michigan choose from. Many of these courses have analogs at other Institutions and attract young scholars interested in data-intense transdisciplinary research, development, and training.
At the most basic level, graduates should receive analytical training in three complementary domains—mathematics, statistics, and engineering. The mathematical foundations should emphasize basic understanding of multi-variable calculus, complex variables and functions, linear algebra, matrix computing, differential equations, numerical methods, and optimization. Statistics knowledge should stress practical experience with at least a couple of different statistical computing software packages, understanding of probability theory, distribution functions, and Bayesian inference, as well as parametric and non-parametric statistical tests. Finally, it is important to enhance the graduates' engineering abilities, develop working knowledge of some compiled and interpreted programming languages, data ingestion, management, and visualization.
In addition to quantitative analytical training, all program graduates should be exposed to qualitative and common-sense human-intelligence training including data quality challenges, model interpretability, research ethics, privacy and security, health policy and regulatory guidelines, and implementation research.
• Data quality challenges are always present in big biomedical and health studies, this includes understanding the importance of tracking provenance and assessing data quality, “fitness for use,” completeness, and complexity (47–49).
• Model interpretability and transparency is important to be understood, disclosed, and properly interpreted to contextualize the performance, bias, implementation approach, reported findings, potential limitations, and possible unintended consequences (50).
• Research ethics blends the individual scholar values, e.g., honesty and personal integrity, and treatment of other individuals involved in the research, e.g., informed consent, confidentiality, anonymity, and courtesy (51).
• Information security, and privacy protection training are absolutely necessary and will play a vital role throughout all professional activities of graduates (52).
• The landscape health policies are constantly created and updated to drive healthcare research and influence health achievements. Legislative and regulatory guidelines also impact biomedical and health research (53). These are intendend to standardize and control types of scholarly nad organizational behavior, monitor, and enforce policies and licensing, and accreditation.
• Implementation research amalgamates scienctific research and healthcare practice. It is focused on the creation knowledge that can be applied to improve health policies, clinical programs, medical practice, and the borader public health (54).
Due to substantial heterogeneities in institutional course offerings, depth and breadth of program coverage, and variations in individual backgrounds, learning-styles, and scholarly interests, “one-curriculum-plan-does-not-fit-all.” It's difficult to prescribe one unique curriculum that includes a specific number of courses to complete, a concrete course-series ordering, and a single completion timeframe. In principle, each Health Science doctoral program will comprise a set of core courses, required for all trainees, a complementary set of specialization and elective courses, and alternative practical experiences (e.g., mentored lab rotations, internships, apprentice shadowing, hands-on capstone projects, etc.).
Table 3 outlines some hypothetical curriculum plans that may be customized and adopted in various quantitative graduate health science and analytical programs. The longitudinal flow (columns) and thematic variability (rows) are neither complete, not exhaustive, or mandatory.
Expected Competencies
In addition to their core area of specialization, graduating doctoral students should be expected to have moderate modeling, computational, and analytic competency in at least two of each of the three competency areas listed in Table 4.
One important point to emphasize is that in addition to the proposed quantitative outcomes of any graduate biomedical and health training program, trainees should be expected to acquire a number of complementary qualitative skills. Such abilities include transitional science expertise, behavior change adoptability, and aptitude for identification of significant findings for clinical implementation. The focus of this specific manuscript is on the quantitative part of the training, i.e., the methods and analytics curricula for health science doctoral programs; however, soft skills, human intelligence, and artistic abilities are also important (8).
Conclusions
The role of continuous self-learning is paramount in the future on-demand economy, where rapid developments and technological advances quickly render static technical skills obsolete. One of the best lessons biomedical and health science doctoral program graduates should learn is the value of sustained lifelong commitment to learning, retooling, knowledge refreshing, and dynamic skill building. This is neither easy, quick, nor necessarily intuitive; however, it is absolutely essential for a perpetually successful career. The main factors driving the need for sustained self-learning include the relentless pace of automation (55), world-wide competition and the rise of the rest (56), the growth of network-based team science (57), the unrelenting anticipation of progress and increase of human well-being over time (58, 59), and the law of diminishing returns (60). The latter asserts that as equal efforts, resources or infrastructure are provided to support an R&D activity, the resulting output from these endeavors will initially increase monotonically with the input up to a certain point, after which, adding additional resources will result in steadily and disproportional decrease where the incremental additive outcome will tend to zero (61).
In addition to the technical, methodological, and analytical skills, there are other qualitative abilities skills that all premier graduate health and biomedical programs should emphasize. As health sciences are both deep and broad in scope, consideration needs to be made to improve inter-professional training and interdisciplinary collaborations (62, 63). Ability to communicate across disciplines is vital to establish, grow and sustain team science, crowdsourcing accomplishments, and citizen scholars, which recently demonstrated forward advances (57). For instance, the Galaxy Zoo project had over 250,000 contributors (Zooites) that completed about 200 million classifications of distance images from the Sloan Digital Sky Survey (SDSS), and over 200,000 users contributed to the Foldit project aiming to quickly enhance our understanding of protein folding via a computer game platform. Active and constructive participation in transdisciplinary teams will require well-rounded background with sufficient depth in specific scientific area and ability to broadly communicate with other experts.
It is undeniable that we need to reorganize the graduate health education and biomedical research training to keep up with the exponential increase of information, the broad knowledge field interactions, and the expeditious technological advances. The broader academic community needs to respond to this digital revolution challenge by balancing the need to preserve basic science rigor at the same time strongly emphasizing transdisciplinary network team-science. As no two programs are the same and there will be enormous progress ahead, there is a need for constant community-based revisions and expansions of the advanced quantitative health science analytics curriculum. All such programs will require environment-specific implementations and the need for contributions from all stakeholders (students, instructors, funding agencies, institutional leaders, and program directors).
It is hard to predict what specific recommendations may guarantee long-term success because the two key components of innovation are uncertainty and risk. However, aversion to either of these would virtually guarantee colossal failures. Coordinated efforts by policy makers, funding organizations, academic institutions, graduate biomedical, and health science curriculum committees, course instructors, and trainees will be vital to meet the demand for effective, fair, and consistent progress in improving human well-being and enhancing human experiences. Foundations and scholarly work funding agencies should diversify the pool of peer reviewers, embrace risky and unconventional approaches, reduce their multilevel bureaucracy (e.g., on-demand dynamic program staff selection and proposal formatting barriers), and acknowledge serendipity in scientific discovery (64).
There is an urgent need for strong commitment from all stakeholders to increase the availability of data, access to compute resources, open-science principles, and their embedding directly into all graduate program curricula. Improving the efficiencies of data acquisition, utilization of rich and diverse computational protocols, and research ethics training should augment the core program coursework. These burdens fall primarily on non-student stakeholders, e.g., instructors, advisors, curriculum committees, institutional administration, state and federal regulators, and policymakers. Careful planning and thoughtful implementation would be critical to avoid extreme and unreasonable policies, limit the unexpected consequences, and reduce unconstructive overregulation.
It is important to point out that curriculum design and its effective implementation are two separate aspects of equal importance. Deficiencies in either of these will strongly impact the final program and potentially lead to very different outcomes. The success of any graduate academic program redesign depends on many different factors including (1) the specific curriculum design plan, (2) sustained faculty engagement, (3) long-term financial support, (4) strong institutional backing, (5) appropriate trainee prescreening and selection, and (6) organizational infrastructure. It is impossible to make specific recommendations on the required levels of commitment for each of these vital components to “guarantee” successful launch and sustained programmatic triumph. Neither financial backing, infrastructure, expertise, or organization environment is by itself necessary or sufficient for establishing a successful program. The exact blend of these factors that leads to an exceptional quantitative graduate health science methods-and-analytics program will vary. In some institutions, funding may be more important than infrastructure. In others, existence of appropriate computational services or reliable lab equipment may be more influential than candidate prescreening. However, strengths in more than one of these six factors would certainly increase the likelihood of a successful and lasting curricula implementation. Finally, the role of the program teaching, research, and practice faculty, along with their continuing (re)training, strategic recruitment, cultivation, and retention cannot be overestimated.
Federal, state and local public officials should enact egalitarian policies that stimulate research, innovation, development, and productization without compromising individual privacy, research ethics, or sensitive information. The academic institutions that embrace diverse financial endowments, without compromising impartiality, and implement strategies to democratize transdisciplinary collaborations will likely reap substantial benefits and chart the course forward. Individual instructors should adapt open-science principles in their courses, collaborate and share with others their learning modules, source materials, and champion direct connections to other courses, disciplines, techniques, or learning resources. Last but certainly not least, trainees represent the focal point and the future of the effort to enhance the capability and capacity of the biomedical and health workforce. Graduating students should realize that the era of the 9-to-5, long-term job-security, repetitive occupations, and stagnant knowledge career paths ended as the twentieth-century came to a close. Top graduate biomedical and health educational institutions will provide the fundamentals and train scholars how to self-learn, utilize Cloud-knowledge resources, and expand their know-how. The rest is up to individual researchers, their close scholarly networks, and the administrative staff that manages research, development, and translation activities. The lead article in a recent issue of the Economist, “Doctor You: How Data will transform Health Care” (65), predicts an upcoming health care digital revolution that will empower patients, improve diagnosis, lower costs, and introduce apps as alternatives to conventional drugs. However, this sea change is only possible when networks of well-trained researchers jointly design, implement, support, and continuously expand advanced clinical decision support systems.
The stakes of failing to restructure doctoral biomedical and health science education are high for two reasons. The first corresponds to failure of raising a cadre of computationally skilled and data-literate researchers to support the innovation backbone of future healthcare and biomedical discoveries. Second, there will be a very substantial loss-of-opportunity cost associated with lack of appreciation for the urgent need to change quantitative graduate biomedical education. In 1746, in his “Golden Rules” for “Young Tradesman,” Benjamin Franklin wrote that “time is money” (66), referring to idleness as a direct loss. The analog for this eighteenth century work-lethargy loss of revenue, translates in the twenty first century as a societal deficit of equitable, effective, and progressive human health experiences, due to vegetative investment of resources or lackadaisical education vision. The golden rule for the future young biomedical and health science scholars may be “time is life.”
Author Contributions
The author confirms being the sole contributor of this work and has approved it for publication.
Funding
This work was partially supported by NIH grants P20 NR015331, P50 NS091856, P30 DK089503, U54 EB020406, UL1TR002240, R01 CA233487, and R01MH121079, and NSF grants 1916425, 1734853, 1636840, 1416953, 0716055, and 1023115.
Conflict of Interest
The author declares that the research was conducted in the absence of any commercial or financial relationships that could be construed as a potential conflict of interest.
Acknowledgments
Many colleagues from the Statistics Online Computational Resource (SOCR), Center for Complexity and Self-management of Chronic Disease (CSCD), Big Data Discovery Science (BDDS), and the Michigan Institute for Data Science (MIDAS) provided ideas and feedback.
Abbreviations
AdaBoost, Adaptive boosting (ensemble machine learning strategy); AD, Alzheimer's Disease; ADNI, Alzheimer's Disease Neuroimaging Initiative; API, Application Programming Interface; BANDIT, Bayesian ANalysis to determine Drug Interaction Targets; BDDS, Big Data Discovery Science; CDC, Centers for Disease Control and Prevention; CNN, Convolutional Neural Networks; CSCD, Center for Complexity and Self-management of Chronic Disease; DB, Database; DSPA, Data Science and Predictive Analytics; EDA, Exploratory Data Analysis; GB, Gigabyte; GFT, Google Flue Trends; HCP, Human Connectome Project; HTML5, Hypertext Markup Language (version 5); JSON, JavaScript Object Notation; MCI, Mild Cognitive Impairment; MIDAS, Michigan Institute for Data Science; MOOC, Massive Open Online Course; NIH, National Institutes of Health; NoSQL, non-SQL (database); NSF, National Science Foundation; OLAP, Online Analytical Processing; PHP, Hypertext Preprocessor; PIBS, Program in Biomedical Sciences; Q-Q, Quantile-Quantile (plot); R&D, Research and Development; SDSS, Sloan Digital Sky Survey; SOAP, Simple Object Access Protocol; SOCR, Statistics Online Computational Resource; SQL, Structured Query Language; STEM, Science, Technology, Engineering and Mathematics; SVM, Support Vector Machines; UKBB, United Kingdom Biobank; XML, Extensible Markup Language.
Footnotes
1. ^http://DSPA.predictive.space
2. ^http://midas.umich.edu/certificate/approved-courses
3. ^https://medicine.umich.edu/medschool/education/phd-programs/about-pibs/pibs-curriculum
References
1. Kreps GL, Maibach EW. Transdisciplinary science: the nexus between communication and public health. J Commun. (2008) 58:732–48. doi: 10.1111/j.1460-2466.2008.00411.x
2. Boyack KW, Klavans R, Börner K. Mapping the backbone of science. Scientometrics. (2005) 64:351–74. doi: 10.1007/s11192-005-0255-6
3. Pfau M, Epistemological and disciplinary intersections. J Commun. (2008). 58:597–602. doi: 10.1111/j.1460-2466.2008.00414.x
4. Korazim-Kőrösy Y, Mizrahi T, Bayne-Smith M, García ML. Professional determinants in community collaborations: interdisciplinary comparative perspectives on roles and experiences among six disciplines. J Commun Pract. (2014) 22:229–55. doi: 10.1080/10705422.2014.901267
5. Albert M, Paradis E, Kuper A. Interdisciplinary promises versus practices in medicine: the decoupled experiences of social sciences and humanities scholars. Soc Sci Med. (2015) 126:17–25. doi: 10.1016/j.socscimed.2014.12.004
6. Davies R, Trowsdale J. The value of instability: lessons from reviewing how and why creativity and the arts might interact with STEM education. Eur J Curric Stud. (2017) 4:584–600. Available online at: http://clok.uclan.ac.uk/21342
7. Leek JT, Jager LR. Is most published research really false? Annu Rev Stat Appl. (2017) 4:109–22. doi: 10.1101/050575
8. Dinov ID. Quant data science meets dexterous artistry. Int J Data Sci Anal. (2019) 7:81–6. doi: 10.1007/s41060-018-0138-6
9. Dinov ID. Volume and value of big healthcare data. J Med Stat Inform. (2016) 4:1–7. doi: 10.7243/2053-7662-4-3
10. Dinov I, Heavner B, Tang M, Glusman G, Chard K, Darcy M, Madduri R, et al. Predictive big data analytics: a study of parkinson's disease using large, complex, heterogeneous, incongruent, multi-source and incomplete observations. PLoS ONE. (2016) 1:e0157077. doi: 10.1371/journal.pone.0157077
11. Amirian P, van Loggerenberg F, Lang T, editors. Data Science and Analytics. In: Big Data in Healthcare. Cham: Springer (2017). p. 15–37. doi: 10.1007/978-3-319-62990-2_2
12. Dinov I. Data Science and Predictive Analytics: Biomedical and Health Applications using R. Computer Science. Cham: Springer International Publishing (2018). 800 p. doi: 10.1007/978-3-319-72347-1
13. Henly SJ, McCarthy DO, Wyman JF, Heitkemper MM, Redeker NS, Titler MG, et al. Emerging areas of science: recommendations for nursing science education from the council for the advancement of nursing science idea festival. Nurs Outlook. (2015) 63:398–407. doi: 10.1016/j.outlook.2015.04.007
14. Held ML, Mallory KC, Cummings S. Preparing social work students for integrated health care: results from a national study. J Soc Work Educ. (2017) 53:435–48. doi: 10.1080/10437797.2016.1269707
15. Bangasser DA, Rozensky RH, Fowler GA, Kraha A, Lopez AA, O'Connor M, et al. Psychology's core knowledge, scientific subfields, and health service specialization: preparing a competent workforce-recommendations from the Opening Doors Summit. Train Educ Prof Psychol. (2016) 10:84. doi: 10.1037/tep0000117
16. Fuhrmann CN, Halme DG, O'Sullivan PS, Lindstaedt B. Improving graduate education to support a branching career pipeline: recommendations based on a survey of doctoral students in the basic biomedical sciences. CBE-Life Sci Educ. (2011) 10:239–49. doi: 10.1187/cbe.11-02-0013
17. Pittayachawan S, Macauley P, Evans T. Revealing future research capacity from an analysis of a national database of discipline-coded Australian PhD thesis records. J High Educ Policy Manage. (2016) 38:562–75. doi: 10.1080/1360080X.2016.1196936
18. van Schalkwyk SC, Murdoch-Eaton D, Tekian A, van der Vleuten C, Cilliers F. The supervisor's toolkit: a framework for doctoral supervision in health professions education: AMEE Guide No. 104. Med Teach. (2016) 38:429–42. doi: 10.3109/0142159X.2016.1142517
19. Kim MJ, Park CG, McKenna H, Ketefian S, Park SH, Klopperet H, et al. Quality of nursing doctoral education in seven countries: survey of faculty and students/graduates. J Adv Nurs. (2015) 71:1098–109. doi: 10.1111/jan.12606
20. Rahbar MH, Dickerson AS, Ahn C, Carter RE, Hessabi M, Lindsell CJ, et al. Characteristics of biostatistics, epidemiology, and research design programs in institutions with clinical and translational science awards. Acad Med. (2017) 92:229–36. doi: 10.1097/ACM.0000000000001350
21. Sarkar IN. Biomedical informatics and translational medicine. J Transl Med. (2010) 8:22. doi: 10.1186/1479-5876-8-22
23. Kienholz ML, Crowleyet RS, Bergal JM, Levine AS. Transformative changes to embrace, manage, and exploit “Big Data” in: Wartman SA, editor. The Transformation of Academic Health Centers. Boston, MA: Elsevier (2015). p. 159–68. doi: 10.1016/B978-0-12-800762-4.00016-5
24. Treinish LA. Scientific Data Models for Large-Scale Applications. New York, NY: IBM TJ Watson Research Center (2004).
25. Poornima S, Pushpalatha M. A journey from big data towards prescriptive analytics. ARPN J Eng Appl Sci. (2006) 11. Available online at: http://www.arpnjournals.org/jeas/research_papers/rp_2016/jeas_1016_5099.pdf
26. Nunes MB. Understanding big data for industrial innovation and design: the missing information systems perspective. J Data Inform Sci. (2009) 2:1–6. doi: 10.1515/jdis-2017-0017
27. Dinov I. Methodological challenges and analytic opportunities for modeling and interpreting big healthcare data. GigaScience. (2016) 5:1–15. doi: 10.1186/s13742-016-0117-6
28. Zhou Y, Zhao L, Zhou N, Zhao Y, Marino S, Wang T, et al. Predictive big data analytics using the UK biobank data. Sci Rep. (2019) 9:6012. doi: 10.1038/s41598-019-41634-y
29. Sudlow C, Gallacher J, Allen N, Beral V, Burton P, Danesh J, et al. UK biobank: an open access resource for identifying the causes of a wide range of complex diseases of middle and old age. PLoS Med. (2015) 12:e1001779. doi: 10.1371/journal.pmed.1001779
30. Biobank U. UK Biobank: Protocol For A Large-Scale Prospective Epidemiological Resource. Cheshire: UK Biobank Coordinating Centre (2007).
31. Sporns O. The human connectome: origins and challenges. Neuroimage. (2013) 80:53–61. doi: 10.1016/j.neuroimage.2013.03.023
32. Van Essen DC, Ugurbil K, Auerbach E, Barch D, Behrens TE, Bucholz R, et al. The human connectome project: a data acquisition perspective. Neuroimage. (2012) 62:2222–31. doi: 10.1016/j.neuroimage.2012.02.018
33. Jack CR, Bernstein MA, Fox NC, Thompson P, Alexander G, Harvey D, et al. The Alzheimer's disease neuroimaging initiative (ADNI): MRI methods. J Magn Reson Imaging. (2008) 27:685–91. doi: 10.1002/jmri.21049
34. Moon S, Dinov ID, Kim J, Zamanyan A, Hobel S, Thompson PM, et al. Structural neuroimaging genetics interactions in Alzheimer's disease. J Alzheimers Dis. (2015). 48:1051–63. doi: 10.3233/JAD-150335
35. Mason KE, Pearce N, Cummins S. Associations between fast food and physical activity environments and adiposity in mid-life: cross-sectional, observational evidence from UK Biobank. Lancet Public Health. (2018) 3:e24–33. doi: 10.1016/S2468-2667(17)30212-8
36. Van Essen DC, Smith SM, Barch DM, Behrens TE, Yacoub E, Ugurbil K, et al. The WU-minn human connectome project: an overview. NeuroImage. (2013) 80:62–79. doi: 10.1016/j.neuroimage.2013.05.041
37. Smith SM, Nichols TE. Statistical challenges in “big data” human neuroimaging. Neuron. (2018) 97:263–8. doi: 10.1016/j.neuron.2017.12.018
38. Cook S, Conrad C, Fowlkes AL, Mohebbi MH. Assessing google flu trends performance in the United States during the 2009 influenza virus A (H1N1) Pandemic. PLOS ONE. (2011) 6:e23610. doi: 10.1371/journal.pone.0023610
39. Lazer D, Kennedy R, King G, Vespignani A. The parable of Google Flu: traps in big data analysis. Science. (2014) 343:1203–5. doi: 10.1126/science.1248506
40. Topol EJ. High-performance medicine: the convergence of human and artificial intelligence. Nat Med. (2019) 25:44–56. doi: 10.1038/s41591-018-0300-7
41. Madhukar NS, Khade PK, Huang L, Gayvert K, Galletti G, Stogniew M, et al. A new big-data paradigm for target identification and drug discovery. bioRxiv. (2017) 134973. doi: 10.1101/134973
42. Katsis Y, Balac N, Chapman D, Kapoor M, Block J, Griswoldet WG, et al. Big data techniques for public health: a case study. In: 2017 IEEE/ACM International Conference on Connected Health: Applications, Systems and Engineering Technologies (CHASE). Washington, DC (2017).
43. Kruskal JB, Berkowitz S, Geis JR, Kim W, Nagy P, Dreyeret K, et al. Big data and machine learning-strategies for driving this bus: a summary of the 2016 intersociety summer conference. J Am Coll Radiol. (2017) 14:811–7. doi: 10.1016/j.jacr.2017.02.019
44. Chang V. Computational intelligence for medical imaging simulations. J Med Syst. (2018). 42:10. doi: 10.1007/s10916-017-0861-x
45. Farahani B, Firouzi F, Chang V, Badaroglu M, Constant N, Mankodiyaet K, et al. Towards fog-driven IoT eHealth: promises and challenges of IoT in medicine and healthcare. Future Gener Comput Syst. (2018) 78:659–76. doi: 10.1016/j.future.2017.04.036
46. Rudy SH, Brunton SL, Proctor JL, Kutzet JN. Data-driven discovery of partial differential equations. Sci Adv. (2017) 3:e1602614. doi: 10.1126/sciadv.1602614
47. Kahn MG, Callahan TJ, Barnard J, Bauck AE, Brown J, Davidson BN, et al. A harmonized data quality assessment terminology and framework for the secondary use of electronic health record data. Egems. (2016) 4:1244. doi: 10.13063/2327-9214.1244
48. Sahoo SS, Nguyen V, Bodenreider O, Parikh P, Minning T, Sheth AP, et al. A unified framework for managing provenance information in translational research. BMC Bioinformatics. (2011) 12:1. doi: 10.1186/1471-2105-12-461
49. Dinov I, Lozev K, Petrosyan P, Liu Z, Eggert P, Pierce J, et al. Neuroimaging study designs, computational analyses and data provenance using the LONI pipeline. PLoS ONE. (2010) 5:e13070. doi: 10.1371/journal.pone.0013070
50. Tonekaboni S, Joshi S, McCradden MD, Goldenberg A. What clinicians want: contextualizing explainable machine learning for clinical end use. arXiv:1905.05134 (2019). Available online at" https://arxiv.org/abs/1905.05134
52. Abouelmehdi K, Beni-Hessane A, Khaloufi H. Big healthcare data: preserving security and privacy. J Big Data. (2018) 5:1. doi: 10.1186/s40537-017-0110-7
53. Atasoy H, Greenwood BN, McCullough JS. The digitization of patient care: a review of the effects of electronic health records on health care quality and utilization. Annu Rev Public Health. (2019) 40:487–500. doi: 10.1146/annurev-publhealth-040218-044206
54. Theobald S, Brandes N, Gyapong M, El-Saharty S, Proctor E, Diaz T, et al. Implementation research: new imperatives and opportunities in global health. Lancet. (2018) 392:2214–28. doi: 10.1016/S0140-6736(18)32205-0
55. Rotman D. The Relentless Pace of Automation. MIT Technology Review (2017). Retreived from https://www.technologyreview.com/s/603465/the-relentless-pace-ofautomation (accessed February 2, 2020).
56. Zakaria F. The rise of the rest. Newsweek. (2008) 12:24–31. Available online at: https://www.jstor.org/stable/20032649
57. Franzoni C, Sauermann H. Crowd science: the organization of scientific research in open collaborative projects. Res Policy. (2014) 43:1–20. doi: 10.1016/j.respol.2013.07.005
58. Lange M. Stanford Encyclopedia of Philosophy: Progress. (2011). Available online at: http://plato.stanford.edu/archives/spr2011/entries/progress (accessed February 2, 2020).
59. Blackmar FW. The Story of Human Progress, Blackmar FW, editor. Leavenworth, WA: Press of Ketcheson & Reeves (1896).
60. Brue SL. Retrospectives: the law of diminishing returns. J Econ Perspect. (1993) 7:185–92. doi: 10.1257/jep.7.3.185
61. Shephard RW, Färe R. The law of diminishing returns. Zeitschrift für Nationalökonomie. (1974) 34:69–90. doi: 10.1007/BF01289147
62. Murphy JE, Liles AM, Bingham AL, Chamberlin KW, Dang DK, Hill LG, et al. Interprofessional education: principles and application. An update from the american college of clinical pharmacy. J Am Coll Clin Pharm. (2019) 1:e17–e28. doi: 10.1002/jac5.1025
63. Black EW, Blue AV, Davidson R, McCormack WT. Using team-based learning in a large interprofessional health science education experience. J Inter Prof Educ Pract. (2016) 5:19–22. doi: 10.1016/j.xjep.2016.09.002
64. Dinov I. Flipping the grant application review process. Stud High Educ. (2019) 1:1–9. doi: 10.1080/03075079.2019.1628201
65. Beddoes S. Doctor you: how data will transform health care. Economist. (2018) 426:9–10. Available online at: https://www.economist.com/news/leaders/21736138-welcome-doctor-you-revolution-health-care-coming
Keywords: doctoral training, health science, graduate curricula, methods, analytics, data science, quantitative education
Citation: Dinov ID (2020) Modernizing the Methods and Analytics Curricula for Health Science Doctoral Programs. Front. Public Health 8:22. doi: 10.3389/fpubh.2020.00022
Received: 17 July 2019; Accepted: 23 January 2020;
Published: 13 February 2020.
Edited by:
Harshad Thakur, Tata Institute of Social Sciences, IndiaReviewed by:
Jagmeet S. Kanwal, Georgetown University, United StatesDonna Jeanne Petersen, University of South Florida, United States
Copyright © 2020 Dinov. This is an open-access article distributed under the terms of the Creative Commons Attribution License (CC BY). The use, distribution or reproduction in other forums is permitted, provided the original author(s) and the copyright owner(s) are credited and that the original publication in this journal is cited, in accordance with accepted academic practice. No use, distribution or reproduction is permitted which does not comply with these terms.
*Correspondence: Ivo D. Dinov, c3RhdGlzdGljc0B1bWljaC5lZHU=