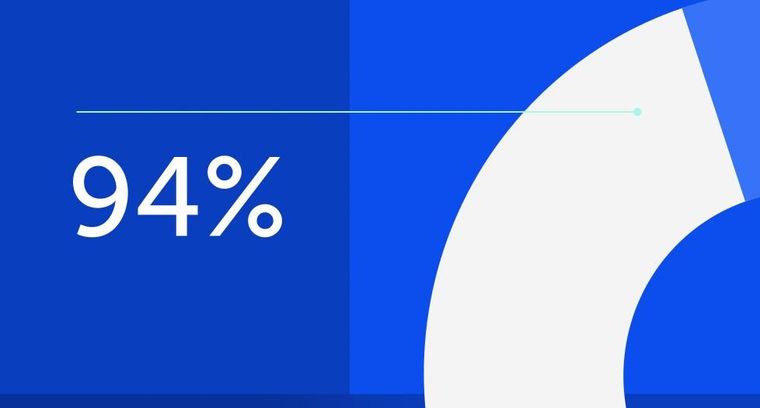
94% of researchers rate our articles as excellent or good
Learn more about the work of our research integrity team to safeguard the quality of each article we publish.
Find out more
ORIGINAL RESEARCH article
Front. Psychiatry, 02 April 2025
Sec. Addictive Disorders
Volume 16 - 2025 | https://doi.org/10.3389/fpsyt.2025.1555372
Background: Internet addiction (IA), especially in young people, has gained increasing attention in recent years. This study examined the prevalence of IA and its associated factors, relationship with quality of life (QoL) and network structure among college students.
Methods: A cross-sectional study was conducted between September and December 2023 in China. Internet addiction symptoms were assessed using the Internet Addiction Test (IAT). Univariate and multivariate analyses were performed to explore the correlates of IA. The relationship between IA and QoL was examined using analysis of covariance (ANCOVA). The Expected Influence (EI) centrality index was used in the network model to characterize the structure of IA symptoms.
Results: A total of 6,514 college students were included. The prevalence of IA was 27.9% [95% confidence interval (CI): 26.8%-29.0%]. A binary logistic regression analysis indicated that living in urban areas (OR=1.135, P=0.032), being in senior grade (OR=1.396, P=0.017), and having current drinking (OR=1.431, P<0.001) were associated with increased risk of IA, while having a major in health (OR=0.796, P<0.001), good health status (OR=0.516, P<0.001), good economic status (OR=0.607, P<0.001), and regular physical exercise (OR=0.727, P<0.001) were associated with reduced risk of IA. ANCOVA revealed that college students with IA had lower QoL score (F (1, 6514) = 128.167, P < 0.001). The most central (influential) symptoms were “Academic efficiency declines” (IAT8, EI value=1.10), “Request an extension for longer time” (IAT16, EI value=1.10) and “Neglect chores to spend more time online” (IAT2, EI value=1.00) in the network model of IA symptoms. The symptom “Form new relationships with online users” (IAT4) had the strongest direct positive relationship with QoL, while “Sleep loss” (IAT14) and “Prefer the excitement online to the time with others” (IAT3) had the strongest direct negative relationship with QoL.
Conclusion: Internet addiction was common among Chinese college students. Interventions targeting the most central symptoms and those closely associated with QoL should be developed to address IA in college students and improve the QoL of those with IA in this population.
Internet use is rapidly increasing worldwide in the past few decades (1). The number of Internet users in China according to the China Internet Network Information Center, has exceeded 1.011 billion, with teenagers and young adults accounting for 13.5% of the population (2). While the Internet has brought many advantages to society, it is important to acknowledge that potential problems can arise from its overuse, such as Internet addiction (IA).
Internet addiction is a serious global public health issue characterized by compulsive online behavior and difficulty in controlling Internet use (3). A meta-analysis of studies conducted across 31 countries/territories estimated that the pooled prevalence of IA was 6.0% among the general population (4). Recent studies found an increased risk of IA among young individuals such as college students during the COVID-19 pandemic (5). Epidemiological surveys conducted during the pandemic revealed a prevalence of IA of 55.8% among college students in China (6), 37.4% in Saudi (7), 30.8% in Czech Republic and 33.1% in Slovakia (8). Furthermore, research found that certain mental health problems, such as depression, anxiety, and insomnia, could increase the risk of IA among college students (9).
Quality of Life (QoL) refers to individuals’ perception of their position in life in the context of their culture and value systems, and in relation to their goals, expectations, standards, and concerns (10). Previous studies found that IA could contribute to poor health including mental health issues (e.g., anxiety, depression and loneliness) (11), physical problems (e.g., poor vision) (12) and excessive sitting (11, 13), all of which could result in lower QoL (14). To mitigate the negative influence of IA on QoL, it is important to identify specific symptoms of IA that are strongly linked to QoL among college students. However, most studies on IA have traditionally relied on total scores of standardized scales (15–17), with less focus on elucidating the inter-relationships between individual symptoms of IA (18), even though the psycho-neurological mechanisms underlying the interactions between individual psychiatric symptoms are usually different (19).
Network analysis has emerged as a useful approach for mapping the interactions among specific symptoms within and between psychiatric disorders/syndromes (20, 21). In network approaches, nodes indicate symptoms, while edges indicate associations between pairs of nodes (22), so that the interactions of symptoms can be visualized and consequently explained. Network analysis can identify central (influential) symptoms (i.e., symptoms with the strongest connections with other symptoms) that are most influential and have the strongest impact within a network of symptoms (23), and the association between two symptoms can be calculated after controlling for other symptoms. Additionally, network analysis can identify the most related nodes with a specific symptom, which can potentially identify useful targets for effective treatment outcomes (24). However, to date, no studies have explored the network structure of IA or its links with measures of functioning (e.g., QoL) among college students in the post-pandemic period.
In this study, we conducted a network analysis of IA symptoms in a large sample of college students and explored the relationships between IA symptoms and QoL.
This cross-sectional, multicenter study was conducted between September 1st and December 31st, 2023, across four universities located in both the northern and southern regions of China, thereby enhancing the geographic representativeness of the study sample. Data collection was conducted using the “Wenjuanxing” application embedded within the WeChat platform, following the method employed in previous studies (25, 26). As WeChat was widely utilized by students in their daily studies within the participating universities, it could be assumed that all students were WeChat users. A Quick Response Code (QR code) linked to the study invitation and questionnaire was generated and distributed to all students throughout the study period. Participants were included if they met the following criteria: 1) enrollment as college students in the participating universities, and 2) ability to comprehend the survey’s purpose and complete the questionnaire. This study was approved by the Ethics Committee of Beijing Anding Hospital, China. All eligible participants provided informed consent form.
Basic socio-demographic information was collected. Internet addiction was measured using the validated Chinese version of the Internet Addiction Test (IAT) (27, 28), which is a self-report questionnaire that includes 20 items assessing behaviors associated with IA. Each item is rated on a 5-point Likert scale from “1” (rarely) to “5” (always). Total IAT score ranges from 20 to 100, with a higher score indicating more severe IA symptoms. Those with a total IAT score of ≥ 50 were considered “having Internet addiction”. Global QoL was evaluated from total scores of the first two item of the World Health Organization Quality of Life-BREF (WHOQOL-BREF) Chinese version, with a higher score indicating a higher QoL. Both the Chinese versions of IAT questionnaire (27) and WHOQOL-BREF (29) have good reliability and validity in Chinese populations.
Univariate and multivariate analyses were conducted using SPSS version 26.0. Normality distributions of continuous variables were assessed using one-sample Kolmogorov-Smirnov tests. The comparisons between IA and non-IA groups in terms of socio-demographic information were performed using independent sample t-test, Mann-Whitney U test and chi-square test, as appropriate. Analysis of covariance (ANCOVA) was used to compare QoL between subgroups with and without IA after controlling for variables that had significant differences in univariate analyses. A binary logistic regression analysis was performed to examine independent correlates of IA status. Having IA symptoms was the dependent variable, while those with significant group differences in univariate analyses were entered as independent variables. Two-tailed tests were used in all analyses, with the significance level set at 0.05.
A network analysis was performed using R program (30). In the network model, nodes represented individual IA symptoms and edges represented the correlations between symptoms. Thicker edges represented stronger correlations, with green and red edges indicating positive and negative correlations, respectively. The network structure of IA symptoms was constructed using the Graphical Gaussian Model (GGM) following nonparanormal transformation of the data. The graphic least absolute shrinkage and selection operator (LASSO) in combination with Extended Bayesian Information Criterion (EBIC) were used to estimate a regularized GGM (20). Network model was estimated using the R package “bootnet” and “qgraph” (31). The default gamma hyperparameter was set as 0.5, with “EBICglasso” as the default method (32).
In the network model, expected influence (EI) was used to determine central symptoms as a reliable centrality index (24). Predictability was assessed using the “mgm” package (33) to calculate the variance in a node that could be explained by neighboring nodes. In addition, the “flow” function in R package “qgraph” was used to identify IA symptoms that were directly associated with QoL (32).
To evaluate the robustness of the estimated network, centrality stability was examined using the correlation stability coefficient (CS-coefficient), which was required to be above 0.25, and preferably above 0.50 (31). Additionally, network property differences (i.e., node EIs and edges) were assessed based on bootstrapped difference. Differences were significant between two nodes or two edges if zero was not included in the 95% confidence interval (34) of 1,000-bootstrap. As for bootstrapped CIs of edge weights, larger CIs indicate poorer precision in the estimation of edges. Both case-drop and nonparametric bootstraps (1,000 iterations) were used to evaluate variability in the model.
Some studies (35, 36) found that males were more likely to have IA, but other studies (37, 38) showed that the prevalence of IA among males and females was similar. Hence, the Network Comparison Test (NCT) was performed to assess gender differences in the network structure (i.e., distributions of edge weights) and global strength (i.e., total absolute connectivity among the symptoms) with Bonferrori correction. NCT analysis was performed using the R package “NetworkComparisonTest” (version 2.2.1) (35, 36).
A total of 7,273 college students were invited to participate in this study. Finally, 6,514 students (1,774 males and 4,740 females) met the study criteria and completed the assessments, resulting in a participation rate of 89.56%. The socio-demographic characteristics are presented in Table 1.
The prevalence of IA (IAT total score ≥ 50) was 27.9% (95%CI: 26.8%-29.0%). Table 1 summarizes differences between subgroups with and without IA. College students with IA were more likely to live in urban areas (P=0.024), be in senior grade (P<0.001) and in non-health major (P<0.001), have a history of COVID infection (P=0.039), poor perceived health status (P<0.001), lower income (P<0.001), current drinking (P<0.001) and less exercise (P<0.001) compared to college students without IA.
After controlling for variables which had significant group differences in univariate analyses, the IA subgroup showed a lower QoL score (F (1, 6514) = 128.167, P<0.001). The logistic regression analysis revealed that college students with IA were more likely to live in urban areas (OR = 1.135; P = 0.032), be in senior grade (OR = 1.396; P = 0.017), have lower income (P<0.001), current drinking (OR = 1.413; P<0.001), and less exercise (P<0.001), but were not likely to be in health major (OR = 0.796; P<0.001), or have perceived good health status (OR = 0.516; P<0.001) (see Table 2).
Figure 1 presents the network structure of IA symptoms of college students. A total of 190 edges were estimated; among those, 96 edges had non-zero weights. IAT1 (“Stay online longer”) - IAT2 (“Neglect chores to spend more time online”) was the strongest edge, followed by IAT16 (“Request an extension for longer time spent online”) - IAT17 (“Failure to cut down the time spent online”), and IAT3 (“Prefer the excitement online”) - IAT19 (“Spend more time online over”).
On the right panel of Figure 1, the EI of 20 symptoms in the IAT network is plotted by order. IAT8 (“Academic efficiency declines”) had the highest EI value in the network model, followed by IAT16 (“Request an extension for longer time”), and IAT2 (“Neglect chores to spend more time online”). Descriptive information and network centrality indices of each IA symptom are shown in Supplementary Table S1. The mean predictability was 0.522, indicating that an average of 52.2% of the variance in each node could be accounted for by neighboring nodes in the model.
As presented in Figure 2, IAT4 (“Form new relationships with online users”) had the strongest association with QoL, followed by IAT14 (“Sleep loss”) and IAT3 (“Prefer the excitement online to the time with others”). The difference lied in that IAT4 (average edge weight=0.068) was positively associated with QoL, while IAT14 (average edge weight=-0.066) and IAT3 (average edge weight=-0.053) were negatively associated with QoL.
Figure 3 presents network reliability results. Bootstrapped difference tests for edge weights showed that most comparisons among edge weights were statistically significant. Supplementary Figure S1 presents the network stability results. The CS-coefficient of EI was 0.75 based on the case-dropping bootstrap procedure, indicating that the network model was stable. For the accuracy of the network, bootstrap 95% CIs for estimated edge weights showed a narrow range (see Supplementary Figure S2); most of the edge weights were non-zero, suggesting that most edges were primarily stable and accurate (see Supplementary Figure S3). The comparison of network model between male and female college students did not find significant gender differences in global strength (S = 0.201, p = 0.997), but there was group difference in network structure (M = 0.163, p = 0.001) (Supplementary Figure S4).
Figure 3. Estimation of node expected influence difference by bootstrapped difference test. Gray boxes indicate nodes that do not differ significantly from one-another, and black boxes represent nodes that do differ significantly from one-another in Expected Influence. Here, many nodes significantly differ from each other, meaning that the network stability can be interpreted as high.
To the best of our knowledge, this was the first study to examine the network structure of IA among college students in the post-pandemic era. The findings indicated that IA was common among Chinese college students. Even after adjusting for covariates, college students with IA still exhibited significantly lower QoL compared to those without IA. The network analysis results revealed that the most central symptoms were “Academic efficiency declines” (IAT8), followed by “Request an extension for longer time” (IAT16) and “Neglect chores to spend more time online” (IAT2). These symptoms were more likely to trigger or maintain IA symptoms in college students.
The prevalence of IA among Chinese college students in this study was 27.9% (95%CI: 26.8%-29.0%), which is significantly lower than the rate of 55.8% (95%CI: 53.7%-57.9%), reported among college students in China during the COVID-19 pandemic (6). One possible explanation for this difference could be the considerable lifestyle and social restrictions as a result of the COVID-19 pandemic. Strict public health measures, such as lockdowns and school closures, led to online learning, reduced outdoor physical activity, and increased social distancing among college students. Consequently, students might have spent more time using the Internet (17, 39). Another contributing factor could be the lower percentage of freshmen in our study compared to previous research (6). College freshmen often faced high levels of stress while preparing for their college entrance exam, transitioning to college life, and having reduced supervision and increased freedom, all of which might increase the risk of IA (40).
Consistent with previous findings (41), we found that senior grade students were prone to experiencing IA, which could be attributed to the high academic stress arising from demanding coursework, projects and theses as graduation approaches, as well as future employment concerns. Additionally, residing in urban areas and current alcohol consumption emerged as risk factors for IA, which however is not supported by previous research (42–44). Apart from sociocultural factors, our findings might be related to the greater availability of network devices and Wi-Fi access in cities, along with diminished self-control in those who consumed alcohol. Furthermore, we found that good economic and health status were protective factors against IA, which align with previous research (45, 46). Generally, individuals with high economic level and good physical well-being tended to adopt healthier lifestyles and have increased access to quality medical resources (47). Having a health-related major was also another protective factor, possibly because college students in such courses might have greater awareness of the risks associated with IA compared to those in other majors. Moreover, engaging in regular physical exercise emerged as another protective factor against IA, since physical activities could foster a broader social circle through group classes and sports (48).
We found that “Academic efficiency declines” (IAT8) was the most central symptom with the highest EI value in the network model of IA among college students, which is consistent with the results of previous research that showed excessive smartphone use might diminished work efficiency (49). Several reasons could account for the detrimental impact of IA on academic performance. First, students with IA tended to have excessive attention to online activities, which could hinder their focus on learning and reduce their cognitive and self-control capacity. Consequently, they might experience anxiety, depression, and other mental health problems, which could adversely impact on their academic performance. Additionally, when faced with stressful situations and academic pressures, individuals with IA might resort to online activities as a means of alleviating negative emotions and escaping their reality (50). Further, IA could disrupt healthy sleep patterns (51) among college students since they might spend extended periods online during nighttime, leading to irregular or insufficient sleep. Consequently, they could suffer from impaired concentration, drowsiness, and reduced energy levels during daytime, thereby further hampering their academic performance.
In this study, “Request an extension for longer time” (IAT16) emerged as one of the most central symptoms in the network model which supports past findings regarding the adverse impact of IA on work duties and domestic responsibilities (52). Owing to reduced self-control, college students might spend more time indulging in online activities, often as a means of coping with emotional difficulties (53). Using the Internet for comfort, distraction, or emotional support often led to a destructive cycle of negative emotions, which could contribute to internet addictive behavior (54). Furthermore, college students with high level of anxiety might spend more time online as a means of avoiding other responsibilities, such as academic studies or personal relationships (55). Such results are also consistent with past research suggesting that socially anxious individuals often preferred online communication over face-to-face interactions (56).
“Neglect chores to spend more time online” (IAT2) also was another central symptom in the network model of IA. Chores are tasks or work that are often tedious or unpleasant but require regular completion. Previous findings also linked excessive smartphone use (serving as a proxy for internet use) with reduced engagement in chores or other activities (57). When confronted with daily chores, college students often prioritized immediate gratification offered by the Internet over fulfilling their responsibilities. Previous research found that individuals who relied on the Internet for immediate gratification tended to prioritize internet usage when faced with various demands (58), thus providing temporary relief or distraction from their responsibilities or difficulties they might be encountering.
The flow network of QoL and IA symptoms revealed both positive and negative associations between internet use and QoL among college students. Notably, the symptom “Form new relationships with online users” (IAT4) had the highest positive association with QoL, indicating that establishing friendships online corresponds to QoL improvement in college students. Past research has found that internet use could in fact enhance social relationships and alleviate the severity of depression (59). Additionally, the Internet could provide young people with a wide range of communication and entertainment options (60). Therefore, the internet might also serve as a means for students to forge new friendships.
“Sleep loss” (IAT14) was another symptom which negatively correlated with QoL. Excessive use of the internet could pose a risk of overindulging in the virtual world and neglecting relationships and responsibilities in the real world, such as their personal life or college studies (51). Previous research demonstrated that increased internet usage was associated with reduced sleep duration (61), which in turn could affect QoL. Adolescents often used internet during nighttime to hide their online activities and avoided conflicts with their parents, resulting in decreased sleep duration and potential impacts on their overall health (62). Indeed, excessive use of the Internet to make friends and/or for other reasons might explain why the symptom of “Prefer the excitement online to the time with others” (IAT3) was negatively associated with QoL in this study.
Central symptoms identified within the network model of IA symptoms have potential clinical significance. By targeting these key symptoms, interventions could be implemented to address IA among college students and prevent at-risk students from developing problematic internet use (63). For instance, for the symptoms such as “Request an extension for longer time” and “Academic efficiency declines,” strategies such as enhancing offline peer-to-peer communication, improving family functioning and relationships, and educating families on monitoring internet use (64) could be used by college psychological counselors. Similarly, for the symptom “Neglect chores to spend more time online,”, another effective measure such as reality therapy could be employed. This approach could directly address issues related to self-control by guiding individuals to reflect on their behaviors and plan appropriate alternatives (65).
The strengths of this study included its large sample size, multi-center study design and use of network analysis. Some limitations should also be considered. First, this was a cross-sectional study, so causal directions between symptoms could not be determined. Second, IA was measured via validated questionnaire rather than face to face psychiatric interviews, hence certain response biases or recall biases could not be avoided. Third, for logistical reasons, particular associated factors of IA, such as the severity of physical diseases, and social support and stressful life events, were not recorded. Finally, data were collected from four participating universities in China, thus, the findings could not be generalizable to all college students nationwide.
In conclusion, IA was common among Chinese college students, which showed a negative impact on QoL. Psychosocial interventions that target the most central symptoms (e.g., “Academic efficiency decline”, “Request an extension for longer time”, and “Neglect chores to spend more time online”) should be developed and implemented for those in need. While communicating with new friends online appropriately might be associated with better QoL among college students, those who reported sleep loss due to excessive internet use tended to report poorer QoL. Future research should address such moderating factors.
The original contributions presented in the study are included in the article/Supplementary Material. Further inquiries can be directed to the corresponding authors.
This study was approved by the Ethics Committee of Beijing Anding Hospital, China. All eligible participants provided written informed consent.
L-YA: Data curation, Investigation, Methodology, Writing – original draft. M-YC: Data curation, Investigation, Methodology, Writing – original draft. Y-YJ: Data curation, Investigation, Methodology, Writing – original draft. H-TH: Data curation, Investigation, Methodology, Writing – original draft. SL: Data curation, Investigation, Methodology, Writing – review & editing. YF: Data curation, Investigation, Methodology, Writing – original draft. X-LZ: Data curation, Investigation, Methodology, Writing – original draft. ZS: Data curation, Investigation, Methodology, Writing – review & editing. TC: Data curation, Investigation, Methodology, Writing – review & editing. CN: Project administration, Supervision, Writing – review & editing. Y-TX: Project administration, Supervision, Writing – original draft. GW: Project administration, Supervision, Writing – review & editing.
The author(s) declare that financial support was received for the research and/or publication of this article. The study was supported by Beijing High Level Public Health Technology Talent Construction Project (Discipline Backbone-01-028), the Beijing Municipal Science & Technology Commission (No. Z181100001518005), and the Beijing Hospitals Authority Clinical Medicine Development of Special Funding Support (XMLX202128) and the University of Macau (MYRG-GRG2023-00141-FHS; CPG2025-00021-FHS).
The authors are grateful to all participants and clinicians involved in this study.
The authors declare that the research was conducted in the absence of any commercial or financial relationships that could be construed as a potential conflict of interest.
The author(s) declare that no Generative AI was used in the creation of this manuscript.
All claims expressed in this article are solely those of the authors and do not necessarily represent those of their affiliated organizations, or those of the publisher, the editors and the reviewers. Any product that may be evaluated in this article, or claim that may be made by its manufacturer, is not guaranteed or endorsed by the publisher.
The Supplementary Material for this article can be found online at: https://www.frontiersin.org/articles/10.3389/fpsyt.2025.1555372/full#supplementary-material
1. Berezovskaya IP, Shipunova OD, Kedich SI. Internet addiction and youth coping strategies, proceedings of the XI international scientific conference communicative strategies of the information society. Association for Computing Machinery, St. Petersburg, Russian Federation (2020). p. 23. Available online at: https://www.cac.gov.cn/2021-02/03/c_1613923423079314.htm.
2. China Internet Network Information Center. The 47th statistical reports on internet development. (2021).
3. Lozano-Blasco R, Robres AQ, Sánchez AS. Internet addiction in young adults: A meta-analysis and systematic review. Comput Hum Behav. (2022) 130:107201. doi: 10.1016/j.chb.2022.107201
4. Cheng C, Li AY. Internet addiction prevalence and quality of (real) life: a meta-analysis of 31 nations across seven world regions. Cyberpsychol Behav Soc Netw. (2014) 17:755–60. doi: 10.1089/cyber.2014.0317
5. Duc TQ, Chi VTQ, Huyen NTH, Quang PN, Thuy BT, Nguyen Di K. Growing propensity of internet addiction among Asian college students: meta-analysis of pooled prevalence from 39 studies with over 50,000 participants. Public Health. (2024) 227:250–8. doi: 10.1016/j.puhe.2023.11.040
6. Cai P, Wang J, Ye P, Feng X, Yang G, Huang C, et al. Physical exercise/sports ameliorate the internet addiction from college students during the pandemic of COVID-19 in China. Front Public Health. (2023) 11:1310213. doi: 10.3389/fpubh.2023.1310213
7. Albursan IS, Al Qudah MF, Al-Barashdi HS, Bakhiet SF, Darandari E, Al-Asqah SS, et al. Smartphone addiction among university students in light of the COVID-19 pandemic: prevalence, relationship to academic procrastination, quality of life, gender and educational stage. Int J Environ Res Public Health. (2022) 19:(16). doi: 10.3390/ijerph191610439
8. Gavurova B, Ivankova V, Rigelsky M, Mudarri T. Internet addiction in socio-demographic, academic, and psychological profile of college students during the COVID-19 pandemic in the Czech Republic and Slovakia. Front Public Health. (2022) 10:944085. doi: 10.3389/fpubh.2022.944085
9. Zhao Y, Jiang Z, Guo S, Wu P, Lu Q, Xu Y, et al. Association of symptoms of attention deficit and hyperactivity with problematic internet use among university students in wuhan, China during the COVID-19 pandemic. J Affect Disord. (2021) 286:220–7. doi: 10.1016/j.jad.2021.02.078
10. World Health Organization. The World Health Organization Quality of Life assessment (WHOQOL): position paper from the World Health Organization. Soc Sci Med. (1995) 41:1403–9. doi: 10.1016/0277-9536(95)00112-K
11. Gong R, Zhang Y, Long R, Zhu R, Li S, Liu X, et al. The impact of social network site addiction on depression in chinese medical students: A serial multiple mediator model involving loneliness and unmet interpersonal needs. Int J Environ Res Public Health. (2021) 18:8614. doi: 10.3390/ijerph18168614
12. Jahagirdar V, Rama K, Soppari P, Kumar MV. Mobile phones: vital addiction or lethal addiction? Mobile phone usage patterns and assessment of mobile addiction among undergraduate medical students in telangana. India. J Addict. (2021) 2021:8750650. doi: 10.1155/2021/8750650
13. Kuss DJ, Griffiths MD. Online social networking and addiction–a review of the psychological literature. Int J Environ Res Public Health. (2011) 8:3528–52. doi: 10.3390/ijerph8093528
14. Li HM, Zhong BL. Quality of life among college students and its associated factors: a narrative review. AME Med J. (2022) 7:38. doi: 10.21037/amj-22-96
15. Abdel-Salam DM, Alrowaili HI, Albedaiwi HK, Alessa AI, Alfayyadh HA. Prevalence of Internet addiction and its associated factors among female students at Jouf University, Saudi Arabia. J Egyptian Public Health Assoc. (2019) 94:1–8. doi: 10.1186/s42506-019-0009-6
16. Kolaib AMA, Alhazmi AHH, Kulaib MMA. Prevalence of internet addiction and its associated factors among medical students at Taiba University, Saudi Arabia. J Family Med primary Care. (2020) 9:4797–800. doi: 10.4103/jfmpc.jfmpc_655_20
17. Li YY, Sun Y, Meng SQ, Bao YP, Cheng JL, Chang XW, et al. Internet addiction increases in the general population during COVID-19: evidence from China. Am J Addict. (2021) 30:389–97. doi: 10.1111/ajad.13156
18. Hirota T, McElroy E, So R. Network analysis of internet addiction symptoms among a clinical sample of Japanese adolescents with autism spectrum disorder. J Autism Dev Disord. (2021) 51:2764–72. doi: 10.1007/s10803-020-04714-x
19. Schnabel RB, Hasenfuß G, Buchmann S, Kahl KG, Aeschbacher S, Osswald S, et al. Heart and brain interactions: Pathophysiology and management of cardio-psycho-neurological disorders. Herz. (2021) 46:138–49. doi: 10.1007/s00059-021-05022-5
20. Epskamp S, Borsboom D, Fried EI. Estimating psychological networks and their accuracy: A tutorial paper. Behav Res Methods. (2018) 50:195–212. doi: 10.3758/s13428-017-0862-1
21. Zhong BL, Yuan MD, Li F, Sun P. The psychological network of loneliness symptoms among chinese residents during the COVID-19 outbreak. Psychol Res Behav Manag. (2023) 16:3767–76. doi: 10.2147/PRBM.S424565
22. Burger J, Isvoranu AM, Lunansky G, Haslbeck JMB, Epskamp S, Hoekstra RHA, et al. Reporting standards for psychological network analyses in cross-sectional data. Psychol Methods. (2023) 28:806–24. doi: 10.1037/met0000471
23. Epskamp S, Kruis J, Marsman M. Estimating psychopathological networks: Be careful what you wish for. PloS One. (2017) 12:e0179891. doi: 10.1371/journal.pone.0179891
24. Robinaugh DJ, Millner AJ, McNally RJ. Identifying highly influential nodes in the complicated grief network. J Abnorm Psychol. (2016) 125:747–57. doi: 10.1037/abn0000181
25. Bai W, Cai H, Wu S, Zhang L, Feng KX, Li YC, et al. Internet addiction and its association with quality of life in patients with major depressive disorder: a network perspective. Transl Psychiatry. (2022) 12:138. doi: 10.1038/s41398-022-01893-2
26. Yang Y, Li Y, An Y, Zhao YJ, Zhang L, Cheung T, et al. Workplace violence against chinese frontline clinicians during the COVID-19 pandemic and its associations with demographic and clinical characteristics and quality of life: A structural equation modeling investigation. Front Psychiatry. (2021) 12:649989. doi: 10.3389/fpsyt.2021.649989
27. Li Y, Zhong B, Liu X, Zhang Y, Zhu J, Hao W. Reliability and validity of the Chinese version of self-rating Young’s diagnostic questionnaire of internet addiction: A preliminary study. Chin J Drug Depend (in Chinese). (2012) 21:390–4.
28. Young KS. Internet addiction: the emergence of a new clinical disorder. CyberPsychology Behav. (1998) 1:237–44 doi: 10.1089/cpb.1998.1.237
29. Xia P, Li N, Hau K-T, Liu C, Lu Y. Quality of life of Chinese urban community residents: a psychometric study of the mainland Chinese version of the WHOQOL-BREF. BMC Med Res Method. (2012) 12:1–11. doi: 10.1186/1471-2288-12-37
30. R Core Team. R: A language and environment for statistical computing. Vienna, Austria: R Foundation for Statistical Computing (2020).
31. Epskamp S, Borsboom D, Fried EI. Estimating psychological networks and their accuracy: a tutorial paper. Behav Res Methods. (2018) 50:195–212 doi: 10.3758/s13428-017-0862-1.
32. Epskamp S, Cramer AOJ, Waldorp LJ, Schmittmann VD, Borsboom D. Qgraph: Network visualizations of relationships in psychometric data. J Stat Software. (2012) 48:1–18. doi: 10.18637/jss.v048.i04
33. Haslbeck J, Waldorp LJ. How well do Network Models predict Observations? On the Importance of Predictability in Network Models. Behav Res. (2018) 50:853–61. doi: 10.3758/s13428-017-0910-x
34. Buneviciene I, Bunevicius A. Prevalence of internet addiction in healthcare professionals: Systematic review and meta-analysis. Int J Soc Psychiatry. (2021) 67:483–91. doi: 10.1177/0020764020959093
35. Salama B. Prevalence and associated factors of Internet addiction among undergraduate students at Al-Beheira Governorate, Egypt. Int J Public Health. (2020) 65:905–10. doi: 10.1007/s00038-020-01429-9
36. Shen Y, Wang L, Huang C, Guo J, De Leon SA, Lu J, et al. Sex differences in prevalence, risk factors and clinical correlates of internet addiction among chinese college students. J Affect Disord. (2020) 279:680–6. doi: 10.1016/j.jad.2020.10.054
37. Ibrahim AK, Fouad I, Kelly SJ, El Fawal B, Ahmed GK. Prevalence and determinants of Internet Addiction among medical students and its association with depression. J Affect Disord. (2022) 314:94–102. doi: 10.1016/j.jad.2022.07.007
38. Shen Y, Wang L, Huang C, Guo J, De Leon SA, Lu J, et al. Sex differences in prevalence, risk factors and clinical correlates of internet addiction among chinese college students. J Affect Disord. (2021) 279:680–6. doi: 10.1016/j.jad.2020.10.054
39. Ozturk FO, Ayaz-Alkaya S. Internet addiction and psychosocial problems among adolescents during the COVID-19 pandemic: A cross-sectional study. Arch Psychiatr Nurs. (2021) 35:595–601. doi: 10.1016/j.apnu.2021.08.007
40. Karacic S, Oreskovic S. Internet addiction through the phase of adolescence: A questionnaire study. JMIR Ment Health. (2017) 4:e11. doi: 10.2196/mental.5537
41. AlSaif HI, Alhozaimi ZA, Alrashed AS, Alanazi KS, Alshibani MG, Almigbal TH, et al. Is there an association between increased stress and smartphone addiction? Insights from a study on medical students from Saudi Arabia during the COVID-19 pandemic. Medicina (Kaunas). (2023) 59:(8). doi: 10.3390/medicina59081501
42. Chatterjee S, Kar SK. Smartphone addiction and quality of sleep among Indian medical students. Psychiatry. (2021) 84:182–91. doi: 10.1080/00332747.2021.1907870
43. Latifeh Y, Alkhatib Y, Hmidouch M, Swed S, Hafez W, Sawaf B, et al. Prevalence of internet addiction among Syrian undergraduate medical students. Med (Baltimore). (2022) 101:e32261. doi: 10.1097/MD.0000000000032261
44. Shehata WM, Abdeldaim DE. Internet addiction among medical and non-medical students during COVID-19 pandemic, Tanta University, Egypt. Environ Sci pollut Res Int. (2021) 28:59945–52. doi: 10.1007/s11356-021-14961-9
45. Salameh P, Hajj A, Badro DA, Abou Selwan C, Aoun R, Sacre H. Mental health outcomes of the COVID-19 pandemic and a collapsing economy: perspectives from a developing country. Psychiatry Res. (2020) 294:113520. doi: 10.1016/j.psychres.2020.113520
46. Wang C, Chudzicka-Czupała A, Tee ML, Núñez MIL, Tripp C, Fardin MA, et al. A chain mediation model on COVID-19 symptoms and mental health outcomes in Americans, Asians and Europeans. Sci Rep. (2021) 11:6481. doi: 10.1038/s41598-021-85943-7
47. Roy K, Chaudhuri A. Influence of socioeconomic status, wealth and financial empowerment on gender differences in health and healthcare utilization in later life: evidence from India. Soc Sci Med. (2008) 66:1951–62. doi: 10.1016/j.socscimed.2008.01.015
48. Chen BC, Chen MY, Wu YF, Wu YT. The relationship of social media addiction with internet use and perceived health: the moderating effects of regular exercise intervention. Front Public Health. (2022) 10:854532. doi: 10.3389/fpubh.2022.854532
49. Samaha M, Hawi NS. Relationships among smartphone addiction, stress, academic performance, and satisfaction with life. Comput Hum Behav. (2016) 57:321–5. doi: 10.1016/j.chb.2015.12.045
50. Chen Z, Poon K-T, Cheng C. Deficits in recognizing disgust facial expressions and Internet addiction: Perceived stress as a mediator. Psychiatry Res. (2017) 254:211–7. doi: 10.1016/j.psychres.2017.04.057
51. Pitichat T. Smartphones in the workplace: Changing organizational behavior, transforming the future. LUX: A J Transdisciplinary Writing Res Claremont Graduate Univ. (2013) 3:13. doi: 10.5642/lux.201303.13
52. Ineme ME, Ineme KM, Akpabio GA, Osinowo HO. Predictive roles of depression and demographic factors in Internet addiction: a cross-sectional study of students in a Nigerian university. Int J Cyber Criminology. (2017) 11:10–23. doi: 10.5281/zenodo.495776
53. Javaeed A, Zafar MB, Iqbal M, Ghauri SK. Correlation between Internet addiction, depression, anxiety and stress among undergraduate medical students in Azad Kashmir. Pak J Med Sci. (2019) 35:506–9. doi: 10.12669/pjms.35.2.169
54. Loleska S, Pop-Jordanova N. Is smartphone addiction in the younger population a public health problem? PRILOZI. (2021) 42:29–36. doi: 10.2478/prilozi-2021-0032
55. Glaser P, Liu J, Hakim M, Vilar R, Zhang R. Is social media use for networking positive or negative? Offline social capital and internet addiction as mediators for the relationship between social media use and mental health. New Z J Psychol. (2018) 47:11–7.
56. Ratan ZA, Parrish AM, Zaman SB, Alotaibi MS, Hosseinzadeh H. Smartphone addiction and associated health outcomes in adult populations: A systematic review. Int J Environ Res Public Health. (2021) 18:(22). doi: 10.3390/ijerph182212257
57. Mehrotra A, Müller SR, Harari GM, Gosling SD, Mascolo C, Musolesi M, et al. Understanding the role of places and activities on mobile phone interaction and usage patterns. Proc ACM Interact Mob. Wearable Ubiquitous Technol. (2017) 1:84. doi: 10.1145/3131901
58. Balikuddembe JK, Reinhardt JD. Can digitization of health care help low-resourced countries provide better community-based rehabilitation services? Phys Ther. (2020) 100:217–24. doi: 10.1093/ptj/pzz162
59. Jun HJ, Kim M-Y. What accounts for the relationship between internet use and suicidal ideation of Korean older adults? A mediation analysis. Journals Gerontology Ser B: psychol Sci Soc Sci. (2017) 72:846–55. doi: 10.1093/geronb/gbw163
60. Bremer J. The internet and children: advantages and disadvantages. Child Adolesc Psychiatr Clinics North America. (2005) 14:405–28. doi: 10.1016/j.chc.2005.02.003
61. Barthorpe A, Winstone L, Mars B, Moran P. Is social media screen time really associated with poor adolescent mental health? A time Use diary study. J Affect Disord. (2020) 274:864–70. doi: 10.1016/j.jad.2020.05.106
62. Lemola S, Perkinson-Gloor N, Brand S, Dewald-Kaufmann JF, Grob A. Adolescents’ electronic media use at night, sleep disturbance, and depressive symptoms in the smartphone age. J Youth Adolesc. (2015) 44:405–18. doi: 10.1007/s10964-014-0176-x
63. Zhao Y, Qu D, Chen S, Chi X. Network analysis of internet addiction and depression among Chinese college students during the COVID-19 pandemic: A longitudinal study. Comput Hum Behav. (2023) 138:107424. doi: 10.1016/j.chb.2022.107424
64. Yen JY, Ko CH, Yen CF, Wu HY, Yang MJ. The comorbid psychiatric symptoms of Internet addiction: attention deficit and hyperactivity disorder (ADHD), depression, social phobia, and hostility. J Adolesc Health. (2007) 41:93–8. doi: 10.1016/j.jadohealth.2007.02.002
Keywords: college students, internet addiction, network analysis, prevalence, quality of life
Citation: A L-Y, Chen M-Y, Jiang Y-Y, Huang H-T, Liu S, Feng Y, Zhang X-L, Su Z, Cheung T, Ng CH, Xiang Y-T and Wang G (2025) Internet addiction and its association with quality of life in college students: a network perspective. Front. Psychiatry 16:1555372. doi: 10.3389/fpsyt.2025.1555372
Received: 04 January 2025; Accepted: 03 March 2025;
Published: 02 April 2025.
Edited by:
Wei Hao, Central South University, ChinaReviewed by:
Jiansong Zhou, Central South University, ChinaCopyright © 2025 A, Chen, Jiang, Huang, Liu, Feng, Zhang, Su, Cheung, Ng, Xiang and Wang. This is an open-access article distributed under the terms of the Creative Commons Attribution License (CC BY). The use, distribution or reproduction in other forums is permitted, provided the original author(s) and the copyright owner(s) are credited and that the original publication in this journal is cited, in accordance with accepted academic practice. No use, distribution or reproduction is permitted which does not comply with these terms.
*Correspondence: Yu-Tao Xiang, eHl1dGx5QGdtYWlsLmNvbQ==; Chee H. Ng, Y25nQHVuaW1lbGIuZWR1LmF1; Gang Wang, Z2FuZ3dhbmdkb2NAY2NtdS5lZHUuY24=
†These authors have contributed equally to this work
Disclaimer: All claims expressed in this article are solely those of the authors and do not necessarily represent those of their affiliated organizations, or those of the publisher, the editors and the reviewers. Any product that may be evaluated in this article or claim that may be made by its manufacturer is not guaranteed or endorsed by the publisher.
Research integrity at Frontiers
Learn more about the work of our research integrity team to safeguard the quality of each article we publish.