- 1School of Medicine, Jianghan University, Wuhan, Hubei, China
- 2Office of Psychosocial Services, Wuhan Mental Health Center, Wuhan, Hubei, China
- 3Department of Psychiatry, Wudong Hospital, Wuhan, Hubei, China
Background: The issue of psychological maladjustment, particularly Non-Suicidal Self-Injury (NSSI), is prevalent among vocational high school students. Therefore, timely identification of high-risk individuals is important in providing further intervention.
Methods: A survey was conducted among 2081 students from a vocational high school in Wuhan, China. The students were divided into two groups: those who had engaged in Non-Suicidal Self-Injury (NSSI) within the past two weeks and those who had not. Lasso regression and logistic regression were employed to identify significant risk factors associated with NSSI. Subsequently, a nomogram was developed to enhance the accuracy and efficiency of identifying individuals at high risk for NSSI. The performance of the model was assessed through various validation methods including Area Under the Curve (AUC), calibration curves, and Decision Curve Analysis (DCA).
Results: The significant predictors of NSSI encompassed gender, problem behavior, depressive mood, and borderline personality tendencies. Based on these predictors, a nomogram was constructed. The model’s accuracy was validated using AUC, calibration curves, and DCA, showing high accuracy.
Conclusion: A nomogram prediction tool for NSSI among vocational high school students was constructed, providing an accurate and quick method for predicting adolescent NSSI behavior.
1 Introduction
Non-Suicidal Self-Injury (NSSI) is an increasingly serious clinical and public health problem (1, 2), characterized by individuals repeatedly inflicting superficial but painful physical injuries without suicidal intent. The purpose is mostly to relieve negative emotions or reduce interpersonal stress (3). The onset of NSSI is usually in early adolescence (12 - 14 years). Recent studies on Chinese adolescents have reported a six-month prevalence rate of 22.7% for NSSI (4); Globally, studies estimate a 17% prevalence of non-suicidal self-injury (NSSI) among adolescents, with rates dropping to 6% in adults (5, pp. 1990–2015); this suggests that the lifetime prevalence of this behavior decreases with age. However, failure to intervene promptly can also lead to serious consequences such as cognitive impairment, poor interpersonal relationships, violent crime, and even suicide (6–10). Studies have shown that nearly half (49.29%) of the respondents who had engaged in NSSI behaviors had a history of suicide attempts (11, 12).
There are more risk factors associated with NSSI, which can be broadly categorized into the following seven groups: psychiatric disorders (depression, personality disorders, etc.), bullying, low mental health literacy, problematic behaviors (addictions, substance abuse, etc.), adverse childhood experiences, physical symptoms (disabilities, etc.), and females (13–17). Of these, depression and Borderline Personality Disorder (BPD) are strong correlates of NSSI (18, 19). Problematic behaviors such as addictions, substance abuse, and other behaviors are similarly high-risk factors for NSSI (20, 21).
Vocational education plays an important role in China’s education system, but social recognition of vocational education needs to be improved. Some of the students receiving vocational education may have some challenges and disturbances in terms of academic performance, discipline, character, and habits, which may also affect their self-perception, motivation, and social performance. In addition to the psychological conflicts that characterize adolescence, secondary students also have to face social opinion and employment pressure, which makes them more vulnerable to physical and mental health crises (22, 23). Some studies have shown that the detection rate of NSSI among Chinese secondary school students ranges from 28.9% to 57% (24), which is higher than that of general secondary school students (17%) (25).
Current research on NSSI prediction exhibits two critical limitations. First, while nomograms are widely utilized in clinical depression screening, no dedicated models exist for vocational education populations—particularly secondary vocational students who face unique career-planning pressures and social identity challenges. Second, traditional logistic regression methods often suffer from prediction bias due to multicollinearity in psychological scale data. This study introduces a novel approach by integrating Lasso regression with logistic regression. The methodology involves two phases: (1) Lasso regression automatically identifies key predictors, eliminating redundant variables; (2) logistic regression quantifies risk contributions to generate a visual nomogram. Enable schools, community agencies, and healthcare professionals to swiftly identify individuals at high risk of NSSI.
2 Methods
2.1 Study population
This large-scale cohort study enrolled all full-time students (N=2,160) aged 16-18 years from a vocational high school in Wuhan during September 2022. The inclusion criteria required: Complete responses on core psychosocial measures (NSSI, depressive symptoms, etc.); Guardian-approved informed consent. Exclusion criteria eliminated: Questionnaires with >20% missing items in key domains (n=51); Invalid responses showing response bias (e.g., identical scores across 90% items, n=28); Participants outside the 16-18 age range (n=0). The final analytical sample comprised 2,081 students (916 males, 1,165 females), representing 96.34% of initial participants. The study was approved by the Ethics Committee of Wuhan Mental Health Center (Ethics No. KY2021.11.01), and informed consent was obtained from the participating students and their guardians.
2.2 Measures
Demographic characteristics, school bullying, critical incidents, emotional problems (depression and anxiety), problem behaviors (smoking, drinking, gambling, substance abuse behaviors), and borderline personality disorder (BPD) were investigated.
Demographics: the subjects’ gender, grade level, whether they were an only child, parent’s education level and occupation, and family economic status were mainly included.
Depression: The PHQ-9 scale was used, which consists of nine questions divided into four levels ranging from “not at all” to “almost every day” on a 3-point scale. After completing the retest of the PHQ-9, Cronbach’s alpha coefficient of the total score was 0.85, and the PHQ-9 had good reliability in the assessment of depression in adolescents (26).
Anxiety: The GAD-7 was mainly used. The Generalized Anxiety Scale (GAS) was developed by Spitzer et al. and consists of 7 items based on a total score. The sensitivity of the Chinese version of the GAD-7 was 86.2% with a specificity of 95.5% and a Kappa value of 0.825, suggesting that the GAD-7 has a good validity scale validity (27).
BPD: The borderline subscale of PDQ-4+ was mainly used, and “yes” and “no” were used for scoring, “yes” was scored as 1, and “no” was scored as 0. The PDQ-4+ was mainly used for scoring. “The PDQ-4+ retest reliability coefficients ranged from 0.50 to 0.80 (P < 0.01) with split-half reliability indices ranging from 0.50 to 0.93 and alpha coefficients ranging from 0.56 to 0.78; this is suitable for use in screening scales (28).
Problem behaviors: alcohol, tobacco, gambling, and the presence of addictive or psychoactive substance use were included, with presence scored as “1” and absence scored as “0.”
Critical incident: the Primary Care Post-Traumatic Stress Disorder (PC-PTSD-5) scale was used: it assesses the five symptoms of PTSD: re-experiencing, numbness, avoidance, heightened alertness, and negative change, with good diagnostic accuracy and good reliability.
School bullying: The main investigation was whether the subject had suffered from school bullying in the past 12 months. The score for having experienced bullying in school is “1”, and the score for not having experienced bullying in school is “0”.
NSSI Behavior: The main investigation is whether the subject has experienced NSSI in the past 2 weeks, with NSSI behavior being scored as “1” and no NSSI behavior being scored as “0”.
2.3 Statistical analysis
Descriptive analyses were performed using SPSS 26.0 software and the process of differentiating between training and validation sets, lasso regression, logistic regression, construction of column line plots, and their internal validation were performed using R software (version 4.0.0). The data were randomly divided into training and validation sets with a ratio of 7:3. The training set was used for variable selection and model construction, while the validation set was used to evaluate the effectiveness of the model. Categorical variables were described by their frequencies and percentages. Continuous variables were represented by interquartile spacing because they did not fit the normal distribution (see Appendix A). The training set was analyzed using univariate logistic analysis to compare the relationship of different variables with the NSSI. All tests were two-tailed and p<0.05 was statistically significant.
Significant predictors of NSSI were screened using the Lasso regression model. Lasso regression reduces the correlation between variables and ensures that subsequently generated models are not overfitted (29). 16 Logistic regression models were then constructed to further remove confounders. Combining the above methods to screen for characteristic variables, the four optimal variables were selected to construct a column-line plot. The accuracy of the model is determined by calculating the area under the curve (AUC). Calibration curves provide a visual demonstration of the consistency of the model’s predicted probabilities with actual observations. Finally, the decision curve (DCA) is used to evaluate the value of the predictive model in clinical decision-making (30).
3 Results
3.1 Incidence and demographic characteristics of NSSI in adolescents
Collinearity diagnostics revealed no substantial multicollinearity (all VIFs < 2.0; see Appendix A). Of the 2,081 adolescents surveyed, the age group was concentrated in the 15-18 age group, with 916 males and 1,165 females; 957 were in the first year of high school, 868 in the second year, and 256 in the third year of high school. The total number of those who had experienced NSSI was 187, with an overall detection rate of 9.0% (187/2081); the NSSI detection rate for males (4.3%) was lower than that for females (12.7%), which was statistically different. Lower grades, poorer family finances, unclear marital status of parents, presence of problematic behaviors, experiencing a major event, having depression and anxiety, and BPD were all risk factors associated with self-injury. See Table 1 for details.
The dataset was randomly divided into training sets and validation sets in the ratio of 7:3, as detailed in Table 2, and there was no significant difference between the two groups (P>0.05). The training set totaled 1457 and the number of NSSIs was 136; the validation set was 624 and the number of NSSIs was 51.
3.2 Filter variables
Data analysis was performed on the training set, where categorical variables were analyzed using univariate analysis of variance and continuous variables were tested using non-parametric tests, and the final factors screened out as having a significant effect were gender, age, grade, family economic status, problem behavior, critical incidents, anxiety and depressive moods, and borderline tendencies (all p<0.05), as detailed in Table 3.
To avoid overfitting, the lasso regression analysis was continued, and the results of lasso regression are shown in Figure 1 and Table 4. Figure 1A shows the cross-validation graph, Figure 1B shows the coefficient graph, and Table 4 shows the specific values of lasso regression, the value is not 0 that is, the independent variables that have a significant effect on the dependent variable. The final factors screened out as having a significant effect were gender, problem behavior, critical incident, depression and anxiety, and BPD.
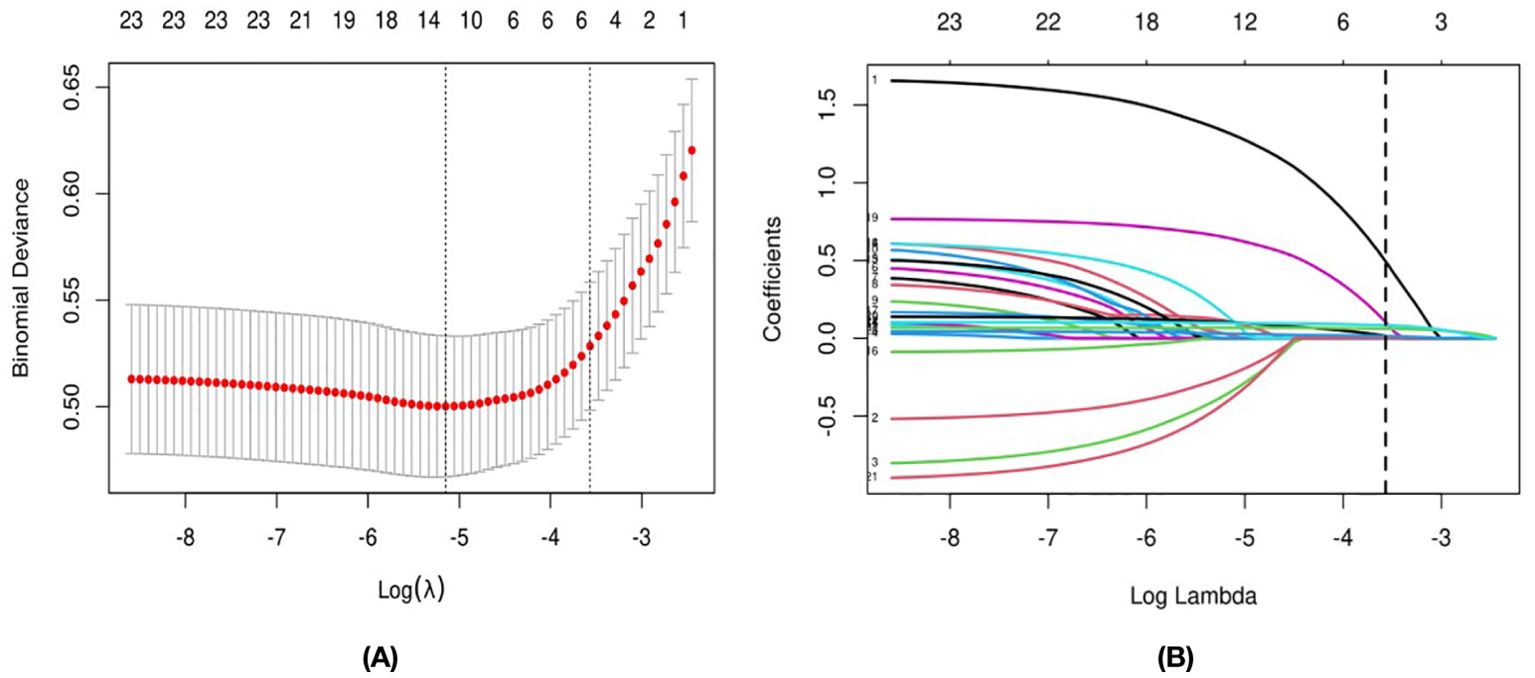
Figure 1. The Variable Filtering Process of the Lasso Regression: (A) for the Cross-Validation Graph, (B) for the Coefficient Graph.
To further exclude the influence of confounding factors on the results, multifactorial logistic regression was adopted, as detailed in Table 5. Eventually, through the above two screening methods, four NSSI-related risk factors were screened out, which were gender, problem behavior, depression score, and BPD.
Table 6 shows the logistic regression model after removing all overfitting factors and confounders, showing better results (all P < 0.05).
3.3 Establish nomogram
Four high-risk factors for NSSI were selected, and a nomogramical model was developed to predict the propensity for NSSI. Each factor had a corresponding score, and these 4 scores were added up to obtain a total score, which then corresponded to the following risk scales to obtain the probability of NSSI. The higher the total score, the higher the probability of NSSI. (See Figure 2).
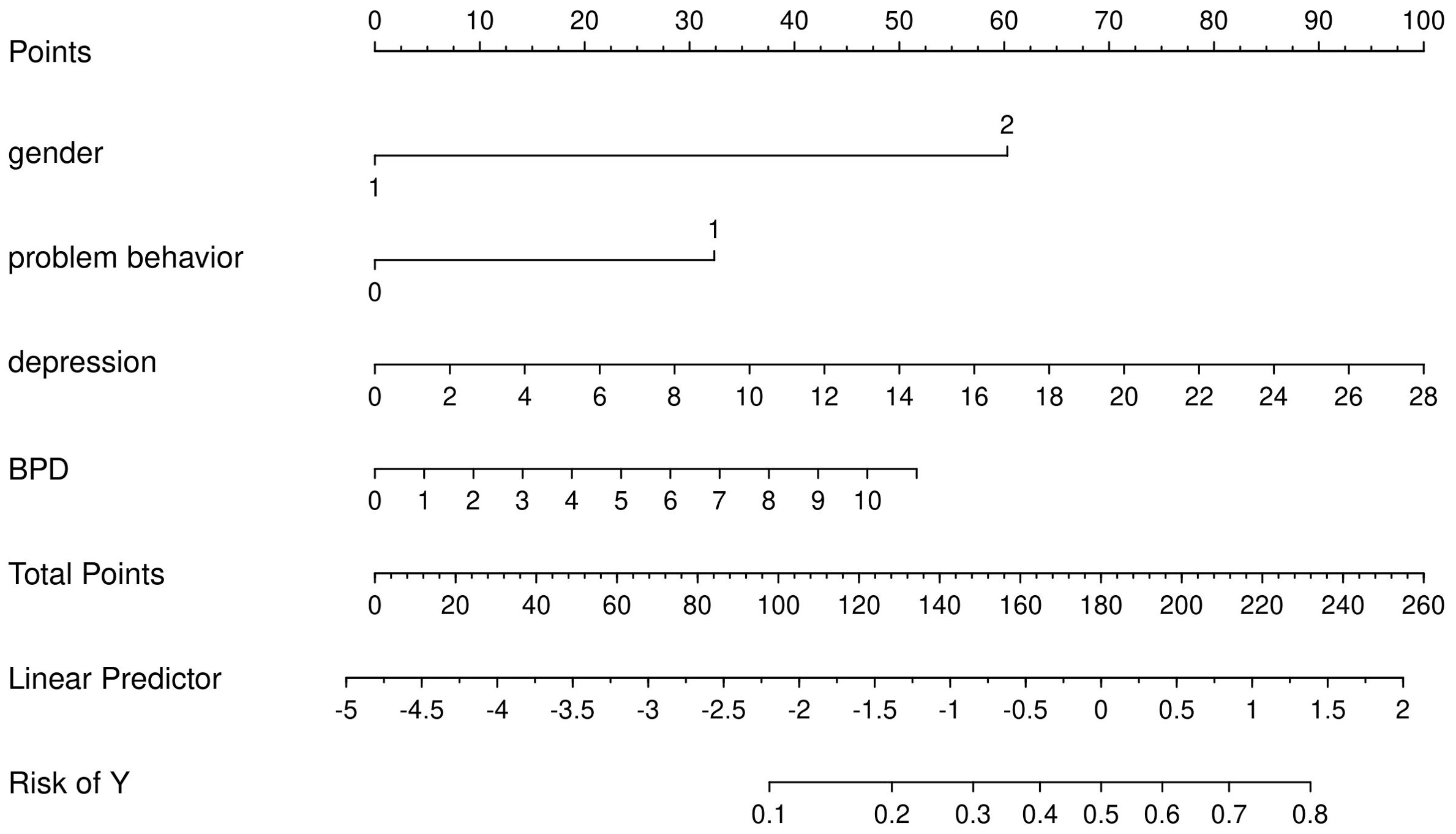
Figure 2. Proposed Nomogram for NSSI. (Gender: 1 = Male, 2 = Female; Problem Behavior: 0 = No, 1 = Yes; Depression: continuous score for depressive symptoms; BPD: continuous score for Borderline Personality Disorder traits).
3.4 Internal validation
The predictive model was evaluated using its area under the curve (AUC). The larger the value of AUC, the higher the accuracy of the model. The AUCs of the column-line plots for the training and validation sets are 0.820 and 0.750, respectively, as shown in Figure 3, indicating that the model is moderately accurate. The predictive ability of the model is fair.
The closer the Bias-corrected line or Apparent line is to the Ideal line, the higher the consistency between the predicted and actual values, using the Calibration curves to judge the model fit. As shown in Figure 4, both groups of Bias-corrected lines are closer to the Ideal line, which indicates a better model fit.
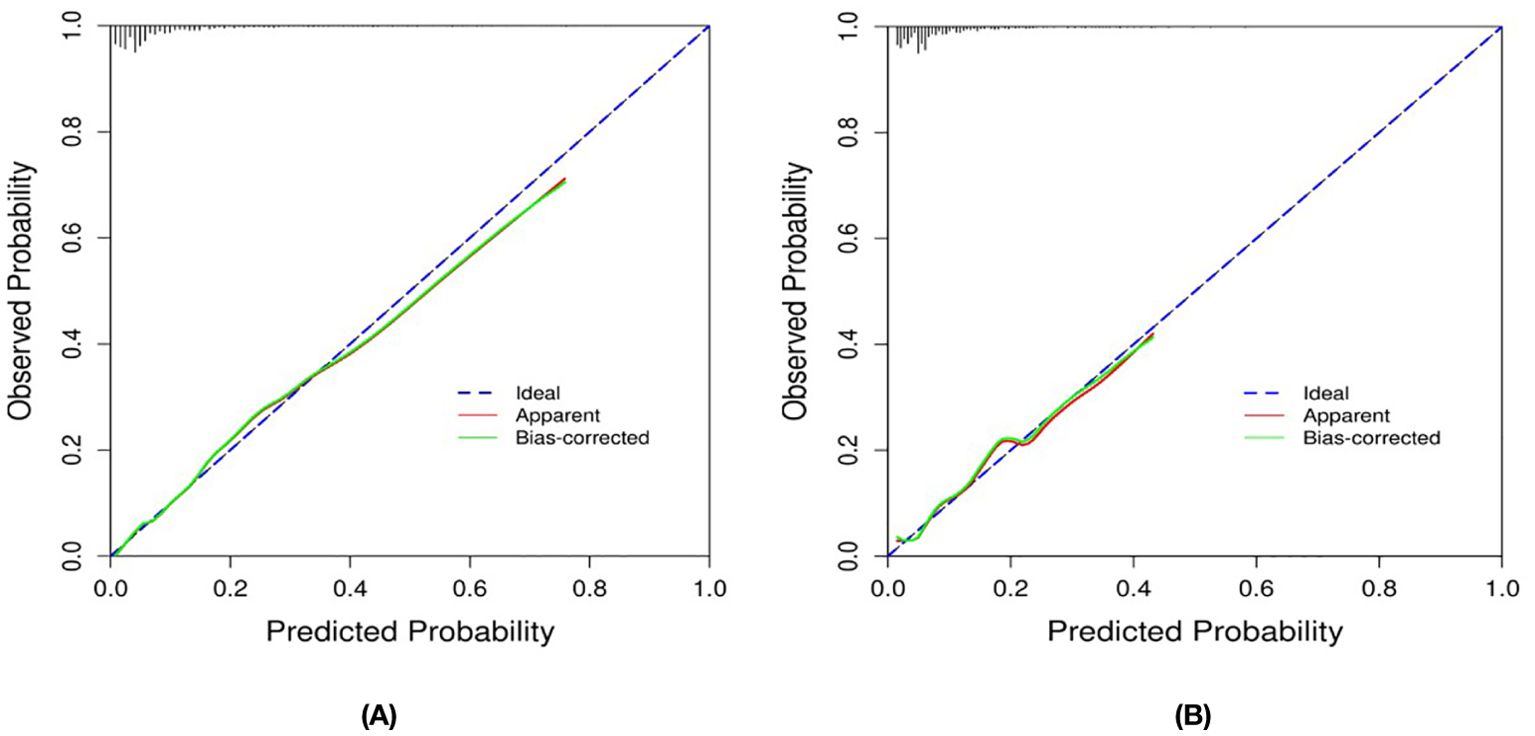
Figure 4. Calibration Curves of the Nomogram in the Study: (A) for the Training set; and (B) for the Internal Validation set.
The clinical benefits of the model were assessed using decision curve analysis (DCA). As detailed in Figure 5, when the model curve is above the “ALL” and “NONE” curves, the model is well-identified, calibrated, clinically applicable, and generic in this range.
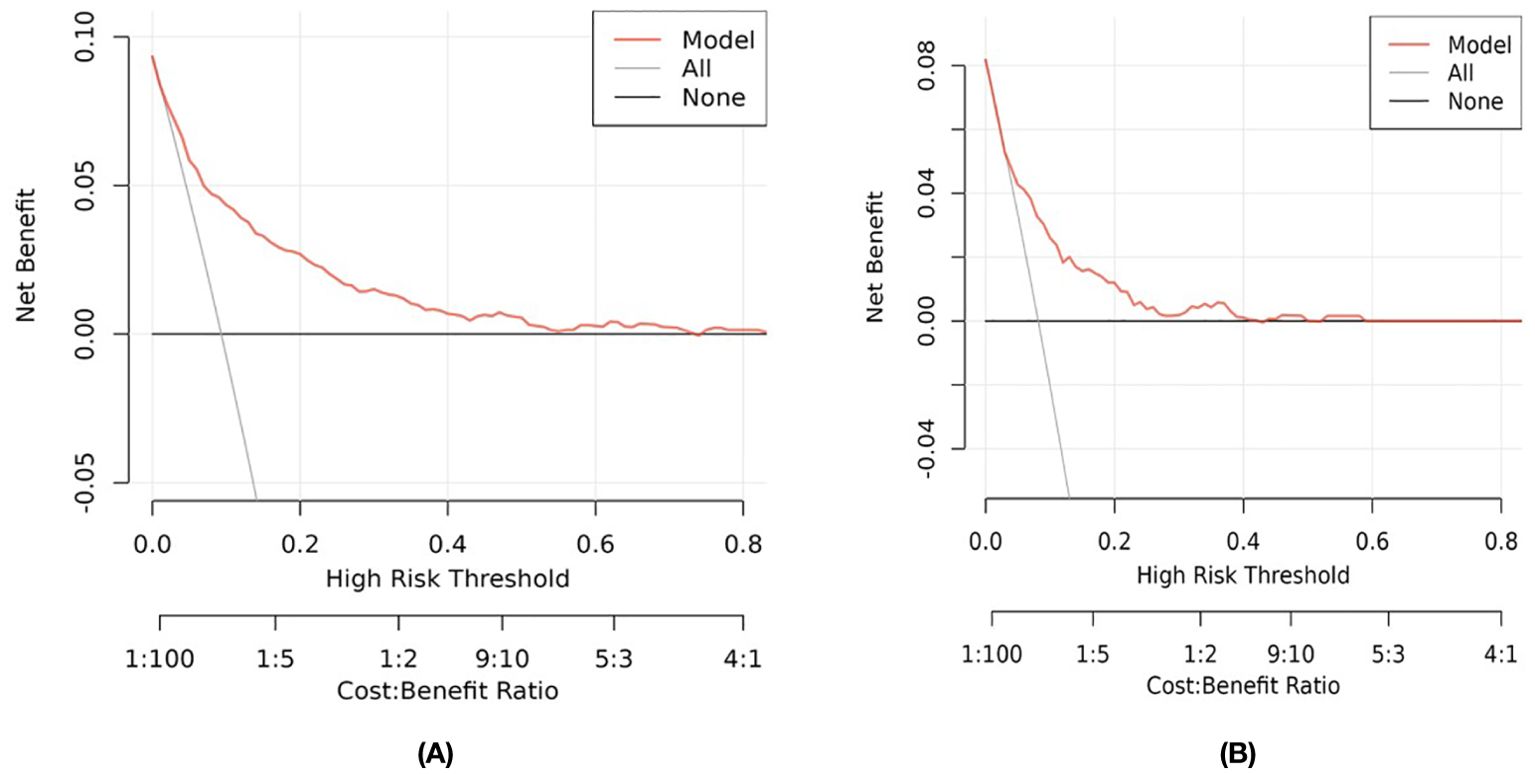
Figure 5. Decision Curve Analysis (DCA) for the Study’s Nomogram: (A) for the Training Set; and (B) for the Internal Validation Set.
4 Discussion
In this study, four major risk factors for NSSI were screened by Lasso regression and logistic regression analyses: females, problem behavior (addictive behaviors such as smoking, drinking, and gambling), depressed mood, and BPD, which is similar to the findings of previous studies (18, 31–37), The importance of these factors in predicting the risk of NSSI was further validated. To make the study results more intuitive and easier to understand, we visualized these risk factors by constructing a nomogram. The nomogram not only visualizes the weight and influence of each factor but also helps educators and clinicians to identify high-risk individuals more accurately.
Compared with men, women are more likely to experience NSSI (15, 38). This phenomenon may be related to physiological mechanisms, as women are affected by luteinizing hormones and estrogen, and their ability to recover from stress is slower than men’s, which increases the risk of NSSI (39, 40); In addition, females tend to ruminate more than males, and are more sensitive to changes in negative emotions, which makes it easier for them to detoxify their negative emotions or regulate their interpersonal relationships through NSSI (41, 42). Meanwhile, research findings suggest that the more severe the depressive mood, the more likely NSSI will occur (43–45), The behavior of NSSI in depressed patients may be associated with genetic and cognitive risk factors such as reduced serotonin transmission (46–48). Problem behavior may negatively impact adolescents’ physical and mental health, disrupting normal developmental trajectories and leading to an increase in mood symptoms, thereby increasing the incidence of NSSI (17, 49). Research has shown that BPD characteristics such as emotion regulation, self-punishment, and resistance to dissociation are strongly associated with NSSI behavior (50), and can indirectly increase the frequency and risk of NSSI by increasing the emotion regulation effect following NSSI (37). During the NSSI, BPD people, who are no longer sensitive to the painful experience of NSSI and whose emotional experience is no longer intense, may be at risk for NSSI because they are no longer sensitive to the painful experience of NSSI (51–54).
5 Strengths and limitations
First, this study avoids overfitting the model through lasso regression and logistic regression. Second, the study focuses on this special and often neglected group of students in Chinese secondary vocational schools, filling a gap in attention to their mental health. Finally, the constructed column-line diagram model visualized the risk factors of NSSI, which made the prediction model more intuitive and practical, and helped people to assess the risk of NSSI in individuals quickly.
However, the study also has shortcomings. First, the sample was limited to students of a vocational high school in Wuhan, which lacked generalization. Second, self-reports may be underreported or exaggerated, and more reliable survey methods are needed. Finally, the study was cross-sectional in design and did not focus on developmental factors, which is a limitation of the study of NSSI development.
6 Conclusion
This study confirms that gender, depressive symptoms, problematic behaviors, and BPD serve as core predictors of NSSI among vocational school students. The visual predictive model constructed from these factors offers practical pathways for tiered intervention in campus mental health practices. Educators can rapidly identify high-risk individuals through scale-based risk evaluation during routine academic management. Concurrently, psychological counselors may design stepwise intervention plans according to specific risk factor profiles, such as the “depression-behavioral issues” subtype or “personality trait-dominant” subtype.
Future investigations should prioritize multicenter controlled trials to assess cross-cultural applicability while strengthening comparative analyses with existing models, including traditional psychological assessment tools and emerging computational approaches. Such efforts will enhance the ecological validity and implementation precision of risk prediction systems within vocational education settings, while addressing inherent limitations of self-reported data.
Data availability statement
The raw data supporting the conclusions of this article will be made available by the authors, without undue reservation.
Ethics statement
The studies involving humans were approved by Wuhan Mental Health Center, Wuhan, Hubei Province. The studies were conducted in accordance with the local legislation and institutional requirements. Written informed consent for participation in this study was provided by the participants’ legal guardians/next of kin.
Author contributions
YL: Methodology, Software, Validation, Visualization, Writing – original draft. TW: Data curation, Investigation, Writing – review & editing. SY: Data curation, Investigation, Methodology, Writing – review & editing. YZ: Data curation, Investigation, Writing – review & editing. LL: Data curation, Investigation, Project administration, Supervision, Writing – review & editing.
Funding
The author(s) declare that no financial support was received for the research and/or publication of this article.
Conflict of interest
The authors declare that the research was conducted in the absence of any commercial or financial relationships that could be construed as a potential conflict of interest.
Generative AI statement
The author(s) declare that no Generative AI was used in the creation of this manuscript.
Publisher’s note
All claims expressed in this article are solely those of the authors and do not necessarily represent those of their affiliated organizations, or those of the publisher, the editors and the reviewers. Any product that may be evaluated in this article, or claim that may be made by its manufacturer, is not guaranteed or endorsed by the publisher.
Supplementary material
The Supplementary Material for this article can be found online at: https://www.frontiersin.org/articles/10.3389/fpsyt.2025.1539884/full#supplementary-material
References
1. Fox KR, Millner AJ, Mukerji CE, Nock MK. Examining the role of sex in self-injurious thoughts and behaviors. Clin Psychol Rev. (2018) 66:3–11. doi: 10.1016/j.cpr.2017.09.009
2. Simone AC, Hamza CA. Examining the disclosure of nonsuicidal self-injury to informal and formal sources: A review of the literature. Clin Psychol Rev. (2020) 82:101907. doi: 10.1016/j.cpr.2020.101907
3. Barnhill JW. DSM-5® Clinical cases. (DSM-5): Washington, DC, USA: American Psychiatric Publishing (2013). doi: 10.1176/appi.books.9781585624836
4. Wei C, Wang Y, Ma T, Zou Q, Xu Q, Lu H, et al. Gratitude buffers the effects of stressful life events and deviant peer affiliation on adolescents’ non-suicidal self-injury. Front Psychol. (2022) 13:939974. doi: 10.3389/fpsyg.2022.939974
5. Gillies D, Christou MA, Dixon AC, Featherston OJ, Rapti I, Garcia-Anguita A, et al. Prevalence and characteristics of self-harm in adolescents: meta-analyses of community-based studies 1990-2015. J Am Acad Child Adolesc Psychiatry. (2018) 57:733–41. doi: 10.1016/j.jaac.2018.06.018
6. Hepp J, Carpenter RW, Störkel LM, Schmitz SE, Schmahl C, Niedtfeld I. A systematic review of daily life studies on non-suicidal self-injury based on the four-function model. Clin Psychol Rev. (2020) 82:101888. doi: 10.1016/j.cpr.2020.101888
7. Mars B, Heron J, Crane C, Hawton K, Lewis G, Macleod J, et al. Clinical and social outcomes of adolescent self harm: Population based birth cohort study. BMJ (Clinical Res Ed.). (2014) 349:g5954. doi: 10.1136/bmj.g5954
8. Oppenheimer CW, Glenn CR, Miller AB. Future directions in suicide and self-injury revisited: integrating a developmental psychopathology perspective. J Clin Child Adolesc Psychology: Off J Soc Clin Child Adolesc Psychology Am psychol Association Division 53. (2022) 51:242–60. doi: 10.1080/15374416.2022.2051526
9. Preyde M, Vanderkooy J, Msw PC, Heintzman J, Msw AW, Barrick K. The psychosocial characteristics associated with NSSI and suicide attempt of youth admitted to an in-patient psychiatric unit. Journal of the Canadian Academy of Child and Adolescent Psychiatry = Journal De l’Academie Canadienne De Psychiatrie De L’enfant Et De L’adolescent (2014) 23(2):100–10.
10. Wilkinson PO, Qiu T, Neufeld S, Jones PB, Goodyer IM. Sporadic and recurrent non-suicidal self-injury before age 14 and incident onset of psychiatric disorders by 17 years: Prospective cohort study. Br J Psychiatry: J Ment Sci. (2018) 212:222–6. doi: 10.1192/bjp.2017.45
11. Liu RT. The epidemiology of non-suicidal self-injury: Lifetime prevalence, sociodemographic and clinical correlates, and treatment use in a nationally representative sample of adults in England. psychol Med. (2023) 53:274–82. doi: 10.1017/S003329172100146X
12. Zetterqvist M. The DSM-5 diagnosis of nonsuicidal self-injury disorder: A review of the empirical literature. Child Adolesc Psychiatry Ment Health. (2015) 9:31–1. doi: 10.1186/s13034-015-0062-7
13. Andrews T, Martin G, Hasking P, Page A. Predictors of onset for non-suicidal self-injury within a school-based sample of adolescents. Prev Science: Off J Soc Prev Res. (2014) 15:850–9. doi: 10.1007/s11121-013-0412-8
14. Bellis MA, Hughes K, Leckenby N, Jones L, Baban A, Kachaeva M, et al. Adverse childhood experiences and associations with health-harming behaviours in young adults: Surveys in eight eastern European countries. Bull World Health Organ. (2014) 92:641–55. doi: 10.2471/BLT.13.129247
15. Fox KR, Franklin JC, Ribeiro JD, Kleiman EM, Bentley KH, Nock MK. Meta-analysis of risk factors for nonsuicidal self-injury. Clin Psychol Rev. (2015) 42:156–67. doi: 10.1016/j.cpr.2015.09.002
16. Hawton K, Saunders KEA, O’Connor RC. Self-harm and suicide in adolescents. Lancet (London England). (2012) 379:2373–82. doi: 10.1016/S0140-6736(12)60322-5
17. Wang Y-J, Li X, Ng CH, Xu D-W, Hu S, Yuan T-F. Risk factors for non-suicidal self-injury (NSSI) in adolescents: A meta-analysis. eClinicalMedicine. (2022) 46:101350. doi: 10.1016/j.eclinm.2022.101350
18. Peters EM, John A, Baetz M, Balbuena L. Examining the role of borderline personality traits in the relationship between major depression and nonsuicidal self-injury. Compr Psychiatry. (2018) 86:96–101. doi: 10.1016/j.comppsych.2018.07.008
19. Zanarini MC, Frankenburg FR, Ridolfi ME, Jager-Hyman S, Hennen J, Gunderson JG. Reported childhood onset of self-mutilation among borderline patients. J Pers Disord. (2006) 20:9–15. doi: 10.1521/pedi.2006.20.1.9
20. Carpenter RW, Trela CJ, Lane SP, Wood PK, Piasecki TM, Trull TJ. Elevated rate of alcohol consumption in borderline personality disorder patients in daily life. Psychopharmacology. (2017) 234:3395–406. doi: 10.1007/s00213-017-4727-1
21. Himelein-Wachowiak M, Giorgi S, Kwarteng A, Schriefer D, Smitterberg C, Yadeta K, et al. Getting “clean” from nonsuicidal self-injury: Experiences of addiction on the subreddit r/selfharm. J Behav Addict. (2022) 11:128–39. doi: 10.1556/2006.2022.00005
22. Denton E-G, Álvarez K. The global prevalence of nonsuicidal self-injury among adolescents. JAMA Network Open. (2024) 7:e2415406. doi: 10.1001/jamanetworkopen.2024.15406
23. Iswanti DI, Lai L-L, Saifudin IM, Moh. Y, Kandar K, Dewi RK, et al. The predictor of non-suicidal self-injury behavior among adolescents: A cross-sectional study. Jurnal Ners. (2024) 19:125–33. doi: 10.20473/jn.v19i2.54610
24. Wang MY, Zhang Y, Zhang SS, Wang LQ. Differences in self-injury behaviors between higher vocational students and secondary vocational students and their relationship with social support. J Shenyang Institute Eng (Social Sci Edition). (2016) 12:557–62. doi: 10.13888/j.cnki.jsie(ss).2016.04.02
25. Zhang YF. Non-suicidal self-injury behavior in secondary vocational school students: A study on the mediating effect model and intervention. Southwest University, Chongqing, China (2023). doi: 10.27684/d.cnki.gxndx.2023.003425
26. Hu XC, Zhang YL, Liang W, Zhang HM, Yang SC. Validation of the reliability and validity of the Patient Health Questionnaire Depression Scale (PHQ-9) in adolescents. Sichuan Ment Health. (2014) 27:357–60. doi: 10.3969/j.issn.1007-3256.2014.04.021
27. Su WL, Luo LX, Yan N, Zhang YC, Jiang GQ, Yuan G. Investigation of the Generalized Anxiety Disorder Scale (GAD-7) among children and adolescents in Chongqing and its influencing factors. J Psychiatry. (2022) 35:158–62. doi: 10.3969/j.issn.2095-9346.202202.009
28. Yang YP, Shen DY, Wang JY, Yang J. A study on the reliability and validity of the Personality Disorder Diagnostic Questionnaire (PDQ-4+) in China. Chin J Clin Psychol. (2002) 3:165–8. doi: 10.16128/j.cnki.1005-3611.2002.03.002
29. Friedman J, Hastie T, Tibshirani R. Regularization paths for generalized linear models via coordinate descent. J Stat Software. (2010) 33:1–22. doi: 10.18637/jss.v033.i01
30. Vickers AJ, Elkin EB. Decision curve analysis: A novel method for evaluating prediction models. Med Decision Making: Int J Soc Med Decision Making. (2006) 26:565–74. doi: 10.1177/0272989X06295361
31. Baker AL, Kavanagh DJ, Kay-Lambkin FJ, Hunt SA, Lewin TJ, Carr VJ, et al. Randomized controlled trial of MICBT for co-existing alcohol misuse and depression: Outcomes to 36-months. J Subst Abuse Treat. (2014) 46:281–90. doi: 10.1016/j.jsat.2013.10.001
32. Buelens T, Costantini G, Luyckx K, Claes L. Comorbidity between non-suicidal self-injury disorder and borderline personality disorder in adolescents: A graphical network approach. Front Psychiatry. (2020) 11:580922. doi: 10.3389/fpsyt.2020.580922
33. Cipriano A, Cella S, Cotrufo P. Nonsuicidal self-injury: A systematic review. Front Psychol. (2017) 8:1946. doi: 10.3389/fpsyg.2017.01946
34. Foulds JA, Douglas Sellman J, Adamson SJ, Boden JM, Mulder RT, Joyce PR. Depression outcome in alcohol dependent patients: An evaluation of the role of independent and substance-induced depression and other predictors. J Affect Disord. (2015) 174:503–10. doi: 10.1016/j.jad.2014.11.052
35. Giletta M, Scholte RHJ, Engels RCME, Ciairano S, Prinstein MJ. Adolescent non-suicidal self-injury: A cross-national study of community samples from Italy, the Netherlands and the United States. Psychiatry Res. (2012) 197:66–72. doi: 10.1016/j.psychres.2012.02.009
36. Larsson B, Sund AM. Prevalence, course, incidence, and 1-year prediction of deliberate self-harm and suicide attempts in early Norwegian school adolescents. Suicide Life-Threatening Behav. (2008) 38:152–65. doi: 10.1521/suli.2008.38.2.152
37. Spitzen TL, Tull MT, Baer MM, Dixon-Gordon KL, Chapman AL, Gratz KL. Predicting engagement in nonsuicidal self-injury (NSSI) over the course of 12 months: The roles of borderline personality disorder pathology and emotional consequences of NSSI. J Affect Disord. (2020) 277:631–9. doi: 10.1016/j.jad.2020.08.049
38. Gromatsky MA, He S, Perlman G, Klein DN, Kotov R, Waszczuk MA. Prospective prediction of first onset of nonsuicidal self-injury in adolescent females. J Am Acad Child Adolesc Psychiatry. (2020) 59:1049–57. doi: 10.1016/j.jaac.2019.08.006
39. Jeong J-Y, Kim D-H. Gender differences in the prevalence of and factors related to non-suicidal self-injury among middle and high school students in South Korea. Int J Environ Res Public Health. (2021) 18:5965. doi: 10.3390/ijerph18115965
40. Liang Y, Chen J, Xiong Y, Wang Q, Ren P. Profiles and transitions of non-suicidal self-injury and depressive symptoms among adolescent males and females: predictive role of bullying victimization. J Youth Adolescence. (2023) 52:1705–20. doi: 10.1007/s10964-023-01779-6
41. Gratz KL, Chapman AL, Dixon-Gordon KL, Tull MT. Exploring the association of deliberate self-harm with emotional relief using a novel Implicit Association Test. Pers Disord. (2016) 7:91–102. doi: 10.1037/per0000138
42. Siener S, Kerns KA. Emotion regulation and depressive symptoms in preadolescence. Child Psychiatry Hum Dev. (2012) 43:414–30. doi: 10.1007/s10578-011-0274-x
43. Brunner R, Kaess M, Parzer P, Fischer G, Carli V, Hoven CW, et al. Life-time prevalence and psychosocial correlates of adolescent direct self-injurious behavior: A comparative study of findings in 11 European countries. J Child Psychol Psychiatry. (2014) 55:337–48. doi: 10.1111/jcpp.12166
44. Cox LJ, Stanley BH, Melhem NM, Oquendo MA, Birmaher B, Burke A, et al. A longitudinal study of nonsuicidal self-injury in offspring at high risk for mood disorder. J Clin Psychiatry. (2012) 73:821–8. doi: 10.4088/JCP.11m07250
45. Jiang Y, Ren Y, Liu T, You J. Rejection sensitivity and adolescent non-suicidal self-injury: Mediation through depressive symptoms and moderation by fear of self-compassion. Psychol Psychother. (2021) 94 Suppl 2:481–96. doi: 10.1111/papt.12293
46. Guerry JD, Prinstein MJ. Longitudinal prediction of adolescent nonsuicidal self-injury: Examination of a cognitive vulnerability-stress model. J Clin Child Adolesc Psychology: Off J Soc Clin Child Adolesc Psychology Am psychol Association Division. (2010) 53(1):77–89. doi: 10.1080/15374410903401195
47. Mendelson T, Kubzansky LD, Datta GD, Buka SL. Relation of female gender and low socioeconomic status to internalizing symptoms among adolescents: A case of double jeopardy? Soc Sci Med. (2008) 66:1284–96. doi: 10.1016/j.socscimed.2007.11.033
48. Seo D, Ahluwalia A, Potenza MN, Sinha R. Gender differences in neural correlates of stress-induced anxiety. J Neurosci Res. (2017) 95:115–25. doi: 10.1002/jnr.23926
49. Xie B, Fan B, Wang W, Li W, Lu C, Guo L. Sex differences in the associations of nonmedical use of prescription drugs with self-injurious thoughts and behaviors among adolescents: A large-scale study in China. J Affect Disord. (2021) 285:29–36. doi: 10.1016/j.jad.2021.02.034
50. Vega D, Torrubia R, Soto À, Ribas J, Soler J, Pascual JC, et al. Exploring the relationship between non suicidal self-injury and borderline personality traits in young adults. Psychiatry Res. (2017) 256:403–11. doi: 10.1016/j.psychres.2017.07.008
51. Andrewes HE, Hulbert C, Cotton SM, Betts J, Chanen AM. Ecological momentary assessment of nonsuicidal self-injury in youth with borderline personality disorder. Pers Disord. (2017) 8:357–65. doi: 10.1037/per0000205
52. Selby EA, Harnedy LE, Hiner M, Kim J. Developmental and momentary dynamics in the onset and maintenance of nonsuicidal self-injurious behavior and borderline personality disorder. Curr Psychiatry Rep. (2022) 24:897–909. doi: 10.1007/s11920-022-01396-3
53. Stepp SD, Lazarus SA, Byrd AL. A systematic review of risk factors prospectively associated with borderline personality disorder: Taking stock and moving forward. Pers Disord. (2016) 7:316–23. doi: 10.1037/per0000186
Keywords: machine learning, neural networks, data analysis, bioinformatics, clinical applications
Citation: Liu Y, Wu T, Yan S, Zhou Y and Liu L (2025) A predictive model of non-suicidal self-injury - a study based on the construction and validation of a nomogram. Front. Psychiatry 16:1539884. doi: 10.3389/fpsyt.2025.1539884
Received: 05 December 2024; Accepted: 11 March 2025;
Published: 04 April 2025.
Edited by:
Albert Kimtai Tele, VU Amsterdam, NetherlandsReviewed by:
Santiago Resett, Conicet- Universidad Austral, ArgentinaIsaiah Gitonga, Maynooth University, Ireland
Copyright © 2025 Liu, Wu, Yan, Zhou and Liu. This is an open-access article distributed under the terms of the Creative Commons Attribution License (CC BY). The use, distribution or reproduction in other forums is permitted, provided the original author(s) and the copyright owner(s) are credited and that the original publication in this journal is cited, in accordance with accepted academic practice. No use, distribution or reproduction is permitted which does not comply with these terms.
*Correspondence: Lianzhong Liu, bGx6d3Vkb25nQDE2My5jb20=; Yang Zhou, MzA3ODU4NzMyQHFxLmNvbQ==