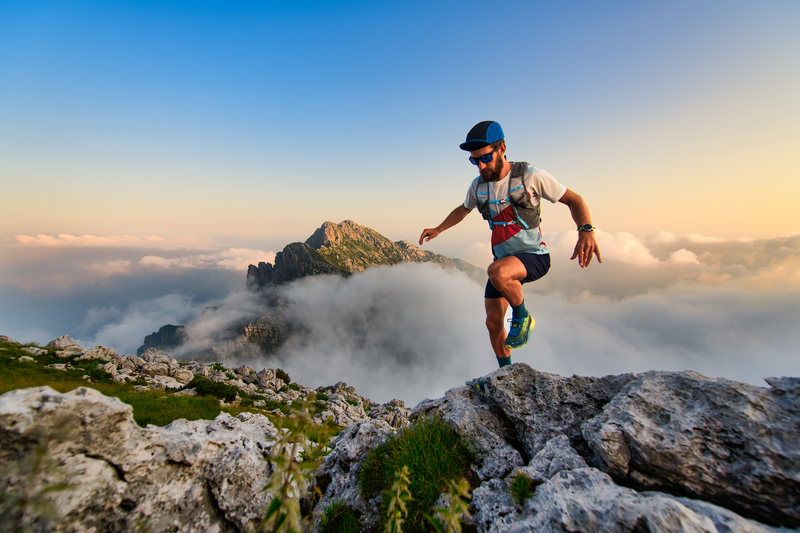
94% of researchers rate our articles as excellent or good
Learn more about the work of our research integrity team to safeguard the quality of each article we publish.
Find out more
ORIGINAL RESEARCH article
Front. Psychiatry , 23 January 2025
Sec. Anxiety and Stress Disorders
Volume 16 - 2025 | https://doi.org/10.3389/fpsyt.2025.1508756
Background: Social anxiety (SA) and problematic smartphone use (PSU) have become increasingly common among college students in recent decades, with research indicating a mutual increase in risk. This study aim to deepen the understanding of how SA and PSU are interconnected at the symptom-level within this demographic using network analysis.
Methods: We recruited 1,197 college students from four institutions in Shaanxi Province, China. Symptoms of SA and PSU were assessed through self-report questionnaires. A regularized Gaussian graphical model was used to estimate the relationships between these symptoms. We calculated Bridge Expected Influence (BEI) to identify key symptoms contributing to their co-occurrence. Additionally, a network comparison test was conducted to examine potential gender differences in the BEI values of the SA-PSU network.
Results: Distinct relationships were observed between SA and PSU symptoms. Notably, the connections between ‘Get embarrassed very easily’ (SA3) and ‘shyness in new situations’ (PSU1), as well as between SA3 and ‘Escape or relieve negative moods’ (PSU8), showed the strongest inter-construct connections. SA3 and PSU8 were identified as the key symptoms contributing to the co-occurrence, with the highest BEI. Network comparison tests between males and females revealed no significant differences in global expected influence, between-community edges weights, and BEI.
Conclusion: The key bridging symptoms this study identified supports the existing theories about the co-occurrence of SA and PSU, and contributes to understanding the underlying mechanisms. Our findings suggest that interventions targeting negative emotions in daily interactions could be effective in reducing PSU.
Social anxiety (SA) is defined as a chronic emotional disorder characterized by an irrational fear or anxiety in social situations where there is potential for scrutiny or negative evaluation by others (1). Its prevalence in Chinese college students is about 12-14%, and topped out at 33% worldwide according to the present studies. Problematic smartphone use (PSU) is defined as excessive use of smartphones phones with marked functional impairment and distress (2, 3). SA and PSU have both become increasingly prevalent and among college students, with a notable rise in recent studies, and they may have serious negative impacts on individual mental health, social functioning and academic development (4–9). Meanwhile, the concrete symptoms and subtypes of SA and PSU have been classified more specifically as the increased attention and exploration (10, 11). The concrete manifestation of SA involve a set of cognitive, emotional and behavioral characteristics (12). For instance, the performance subtype SA has been widely regard as a distinct subtype whose physiological responses to performance situations were extremely strong. Individuals with performance subtype of SA primarily manifest anxious when speaking to group and troubled when being watched, while individuals with other forms of SA may manifest shyness in new situations, nervous because large groups and hard to talk to strangers (13).
In recent years, there has been increasing empirical studies pointed out the positive correlation between SA and PSU (14–18). SA has been treated as the antecedent of PSU, which might cause a range of other negative consequences, such as emotional symptoms (e.g., depression and anxiety) and addiction-like symptoms (e.g., preoccupation, withdrawal and tolerance) (19–21). PSU was associated with similar behavioral and emotional problems, and could further worsen the SA symptoms in turn (20, 22–24).
Meanwhile, theoretical studies proposed several potential theoretical models to explain the interaction between SA and PSU (25, 26). These models are based on the premise that PSU serves as a compensatory mechanism for coping with underlying psychopathology instead of pathological in and of itself inherently (27, 28). For instance, based on the Compensatory Internet Use Theory (CIUT, 28), the excessive electronics use is probably meant to alleviate their negative emotion driven by stressful job and life. In addition, the excessive reassurance seeking has been regarded as the first pathway of integrative Pathways Model, which treated PSU as a maladaptive coping strategy for negative emotions and events (27, 29). Under this theory, people with high level of SA might treat PSU as a way to distract themselves from negative emotions caused by social interaction. In other words, the PSU is driven by negative reinforcement (30, 31). Moreover, the Pathways Model proposed other two pathways leading to the manifestation of PSU, impulsiveness and extraversion, corresponding to individuals with poor impulse control and a constant desire for communication and external stimulation, respectively (29, 30). This impulsiveness pathway is promoted by low self-control or impulse control, which results in increased addictive-like PSU symptoms, such as preoccupation, tolerance and unsuccessful control (30). Impulsivity is a traditional and predictive dimension of most of behavioral addictions (e.g., PSU, 32). It is suggested that the extraversion pathway is linked to a wide range of risky smartphone-related behaviors, which is corresponding to the consequence of reward and excitement-driven smartphone usage patterns, but some studies failed to found the significant positive relationship between extraversion and PSU (30, 33). Several studies which centered around PSU and SA as well as other anxiety disorders have also provided empirical support for these theories (24–26). However, these studies primarily explain the inter-connections leading to PSU through a single pathway, rather than exploring the multifaceted nature of these relationships from a more integrated direction.
Although numerous studies have provided empirical evidence on the association between SA and PSU, most of studies relied on aggregate scores (14–17, 34, 35). Both PSU and SA are heterogeneous syndromes, composed of distinct symptoms (1, 35–37). The aggregate scoring approach may conceal the unique contributions of individual SA and PSU symptoms, thereby limiting mechanistic insights into their co-occurrence. Shifting focus from the disorder level to the symptom level can yield more nuanced pathological information about the co-occurrence between PSU and SA (38–43).
Network analysis offers a symptom-level-oriented approach and achieves significant methodological benefits over traditional statistical models, such as intuitive visualization and unique indices (44, 45). It effectively visualizes the network structure between symptom communities with nodes and edges. As a symptom-level analysis, it also examines the direct relationships between each PSU symptom and specific SA symptoms, providing deeper theoretical and clinical insights. Furthermore, the bridge expected influence (BEI) index could identify key bridge symptoms that activate the other community in general and lead to the co-occurrence of SA and PSU (39). This is crucial for identifying potential intervention targets for managing their co-occurrence (41). It is worth mentioning that the lifetime prevalence of SA and clinical severity of SA is significantly higher among females than males (46, 47), while males are more prone to PSU than females (48). Male and female college students exhibit distinct preferences and usage patterns when it comes to smartphone functionality, with males more inclined towards entertainment uses (e.g., gaming and watching anime), while females more inclined towards social applications of smartphones (49). Therefore, a deeper inspection of the gender differences of the inter-connections between SA and PSU may help in targeted alleviation of its comorbidity, and the network comparison tests could be an appropriate method on this purpose.
Recent studies by Zhou and Shen (18) and Tao et al. (24) have examined the correlation and cross-lagged effects between SA and PSU symptoms among high school students using network analysis. Both studies identified ‘withdrawal’ as a bridge symptom linking SA and PSU. Tao et al. (24) also noted the potential impact of ‘prolonged online learning’ and the moderating role of ‘the fear of missing out’ in this process. However, the risk period for the onset of SA can extend to the early 40s (21). The significant impairments associated with SA and PSU in college students highlighted the need to understand the mechanisms in specific age groups (23, 50, 51). Compared to high school students, college students undergo substantial changes in their social networks and face increased social evaluation as they transition into social life (52, 53). Additionally, upon entering college, young adults often have unrestricted access to mobile phones, more free time, and less parental supervision. This freedom might make the virtual world a more secure environment for those who are more susceptible to anxiety and embarrassment in social interactions (20). Extending such research to include college students is thus crucial for developing better understanding and strategies to improve their mental health.
To address the previously mentioned issues, this study employed network analysis to explore the unique connections between SA and PSU symptoms in college students. Our aims are: 1) to identify the inter-connections between SA and PSU symptoms in this demographic; 2) to identify bridge nodes in this co-occurrence; and 3) to explore potential sex differences in the network characteristics.
The study recruited 1,432 college students via Wenjuanxing, an online tool, and used the convenience sampling approach. Invitations were distributed through WeChat. After excluding 235 responses due to incomplete demographic information (n = 51) and failure to pass two mandatory attention checks (n = 184), a final sample of 1,197 participants was determined. This group comprised 860 females, aged 18 to 22, with a mean age of 18.68 years (SD = 0.85). All participants provided informed consent at the beginning of the survey. The data collection and analysis procedures were approved by the Ethics Committee of the First Affiliated Hospital of the Air Force Medical University (Approval No. KY20234188-1, dated 21 May 2022) and adhered to the Declaration of Helsinki.
SA symptoms were assessed using the SA subscale of the Self-Consciousness Scale (SASS-CS; 54). This subscale includes six items, one of which is reverse coded for scoring. Participants rated each item on a 5-point scale from 1 (strongly disagree) to 5 (totally agree), where higher scores indicate more severe SA symptoms. The Chinese version of the SASS-CS has demonstrated acceptable reliability and validity (51, 55), and confirmed acceptable internal consistency (α = 0.85) in the current study.
PSU symptoms were assessed using the Problematic Smartphone Use Scale (PSUS) developed by Hussain et al. (56). This modified version includes nine items that comprehensively cover the addictive types of PSU symptoms (e.g. preoccupation, withdrawal, tolerance, and escapism/avoidance). Participants rated each item on a 5-point scale, from 1 (never) to 5 (very often). Consistent with previous studies (36, 57), the scale demonstrated good internal consistency (α = 0.85) in the current study.
The current structure of the SA-PSU network was estimated using a combination of the Extended Bayesian Information Criterion Graphical Least Absolute Shrinkage and Selection Operator (EBICglasso) and the Gaussian Graphical Model (GGM), with a gamma value set at 0.5 (58). In this network, edges signify the partial (Spearman) correlations between node pairs, adjusted for other nodes influence (59). The network was constructed and visualized using the Fruchterman-Reingold algorithm, via the R-package qgraph (60, 61). The bridge expected influence (BEI) was calculated via the R-package networktools to evaluate each node’s bridging effect. Higher positive BEI values suggest stronger ability to activate another community, whereas higher negative values indicate a greater ability to inactivate another community (41).
To evaluate the accuracy of edge weights, we calculated 95% confidence intervals (CIs) of edge weights using 1,000 bootstrap samples, and conducted bootstrapped difference tests. We assessed the stability of the BEI estimation by calculating the Correlation Stability (CS) coefficient through a case-dropping methodology, using 1,000 bootstrap samples as well. According to Epskamp et al. (58), a CS coefficient above 0.5 is considered optimal. We conducted these analyses using the R-package bootnet.
To explore potential sex differences within the network, we conducted the network comparison test using the R-package Network Comparison Test, with 1,000 permutations (62). We focused on gender differences in three key network characteristics: global expected influence (the sum of all edges in male and female networks), weights of between-community edges and node BEI. We applied the Bonferroni-Holm method to adjust the significance levels in order to account for multiple comparisons and thereby correct for family-wise errors. (van Borkulo et al., 2023). Moreover, due to the disparity in the number of male and female participants, a subsample matching the number of male participants was randomly drawn from all female participants to undergo the same network comparison procedures, thereby validating the stability of the current network comparison results.
Figure 1A represents the final network structure of SA and PSU symptoms. A total of 17 between-community edges (31.48%) were generated out of 54 potential between-community edges, with edge weights ranging from -0.05 to 0.09. Supplementary Table S1 (in the Supplementary Materials) shows all edge weights within the final network. ‘Troubled when being watched’ (SA2) is positively linked with three PSU symptoms and the strongest positive edge was between SA2 and ‘Escape or relieve negative moods’ (PSU8) (weight = 0.06). ‘Get embarrassed very easily’ (SA3) is positively linked with five PSU symptoms, among which two strongest positive edges were between SA3 and ‘Preoccupation’ (PSU1) (weight = 0.09) and symptom PSU8 (weight = 0.08). ‘Hard to talk to strangers’ (SA4) is negatively linked with symptom PSU1 (weight = -0.05) and positively linked with ‘Negative consequences’ (PSU9) (weight = 0.04). The bootstrapped 95% confidence intervals for the edge weights are shown in Supplementary Figure S1, while the bootstrapped difference test for edge weights is displayed in Supplementary Figure S2 (both in the Supplementary Materials 1).
Figure 1. (A) The network structure of social anxiety and problematic smartphone use. Blue edges represent positive connections and red edges represent negative connections. (B) Bridge expected influence plot.
Table 1 and Figure 1B present each node’s BEI. The nodes SA3 and PSU8 exhibited the highest positive BEI values, at 0.20 and 0.14, respectively. Conversely, ‘Deception’ and SA4 displayed negative BEI, both at -0.02. The CS-coefficient of BEI was 0.67, surpassing the threshold of 0.50 (Supplementary Figure S3 in the Supplementary Material 1). Additionally, the bootstrapped difference test, detailed in Supplementary Figure S4 (in the Supplementary Material 1), highlighted significant variations in BEI among the nodes. Notably, the BEI of SA3 was greater than those of all other nodes, while PSU8 significantly differed from all other nodes within the PSU community, except for PSU1.
Table 1. Abbreviations, mean scores, standard deviations and raw value of bridge expected influences for each variable selected in the present network.
Figure 2A shows the network structure for male participants, and Figure 2B shows the corresponding structure for females. Network comparison tests between males and females revealed no significant differences in global expected influence ([S] = 0.10; males = 6.51, females = 6.61; p = 0.30), network invariance ([M] = 0.15; p = 0.46), and BEI. Figure 2C shows the BEI values for female and male networks. Supplementary Figure S5 and Supplementary Figure S6 (in the Supplementary Material 1) show the robustness test results of female and male network. The CS-coefficients of edges in both female and male networks are 0.75, indicating the stabilities of edge weights are adequate. The CS-coefficients of BEI are 0.59 in female network and 0.44 in male network. Additionally, the network comparison tests on the extracted smaller subsample also showed the same results, indicating the results based on original data set was stable (in the Supplementary Material 2).
Figure 2. Network structure of social anxiety symptoms and problematic smartphone use symptoms for male (A) and female (B) participants. Blue edges represent positive connections and red edges represent negative connections. (C) Bridge expected influence plots for male and female participants.
This study investigated the connections between SA and PSU symptoms in college students using network analysis. Our findings revealed distinct connections between SA and PSU symptoms, with the strongest links observed between SA3 and PSU1; as well as between SA3 and PSU8. Additionally, SA3 demonstrated the strongest positive bridging effect on the PSU symptoms community, while symptom PSU8 showed the strongest positive bridging effect on the SA symptoms community. Gender had minimal impact on the network characteristics.
We observed that SA2 and SA3 were positively related to the same PSU symptom PSU8. It supported previous theories about the mechanisms underlying PSU. This correlation can be explained by negative reinforcement and the excessive reassurance pathway of the Pathways Model: college students with high SA may use smartphones as a coping strategy to mitigate negative emotions from interpersonal interactions (29, 63). Recent studies suggested that frequent cell phone use might be a habitual coping style for avoiding anxiety, and that individuals with SA tended to exhibit more rigid regulation patterns (18, 64). The fear and anxiety about missing out on rewarding events may directly trigger the increase of smartphone use frequency (65, 66). Our findings aligned with these prior research results and underscored the importance of adopting more appropriate and flexible emotional regulation strategies rather than the avoidance coping strategies like PSU in individuals with high levels of SA.
In addition, SA3 positively linked with the PSU1, which could be explained by the excessive reassurance pathway and the impulsiveness pathway of the Pathway Model (27, 29). The more often individuals feel embarrassed in a social situation, the more likely they are to be preoccupied with smartphone use. The imbalance between the effort they put in (i.e., keep calm and restrain negative emotions) and the unsatisfactory outcome they received frequently in social interaction would cause negative emotional overreaction (i.e., embarrassment, anxiety, frustration and other negative emotions), which leads to a decreased performance of self-control (i.e., engage in preoccupation with smartphone use) (67, 68). Similarly, the positive edges between SA symptoms (i.e., characterized by being nervous in large groups, shyness in new situations) and PSU1 supported the same pathway. Previous studies have also provided numerous evidences of the positive symptom-level associations between SA and PSU (17, 18), while the current study further supported that those college students with high level of SA could be more likely to achieve their aim of social avoidance and relieve irritability (e.g., embarrassment, tension and shyness) through excessive focus on their smartphones. It might be helpful to improve the self-control of SA individuals in smartphone usage by realizing the real motivation of their behavior. Furthermore, by revealing a nuanced connection that specific SA symptoms linked to PSU symptoms (i.e., Preoccupation) differently, we highlighted the specific symptom that might drive the SA-PSU association. The current findings built upon this knowledge revealed a nuanced connection: different SA subtype (i.e., performance subtype of SA) may be more closely linked to specific PSU symptoms.
Intriguingly, we found that SA4 was negatively related to PSU1 but positively associated with PSU9. One possible explanation for this could be: difficulty in talking to strangers may reduce smartphone preoccupation as individuals who are less comfortable engaging with new people might not use social media extensively to build and expand their networks, resulting in less overall screen time. However, this same discomfort can lead to negative consequences. The reluctance to initiate conversations, both online and in-person, can result in negative consequences (e.g., missed opportunities and limited personal and professional growth) due to limited social and professional connectivity.
The node bridge centrality of the SA-PSU network may cast light on the prominent role of specific SA symptoms in the development and maintenance of PSU. Since that BEI of SA3 is significantly greater than other nodes in the SA symptoms community, it was identified as bridge nodes for the SA-PSU association. It suggested that SA3 had significantly stronger associations with the majority PSU symptoms than other SA symptoms. Furthermore, in the PSU symptoms community, PSU8 was identified as the bridge node, which indicated that PSU8 might be susceptible to the SA symptoms community. The bridge nodes for the SA-PSU association reported in the current study were different from several studies reported recently (i.e., withdrawal or productivity loss), which might due to the age difference of participants (24, 35). These results might emphasize the need of targeted interventions for different age groups. For instance, the acceptance and commitment therapy or stand-alone virtual reality exposure therapy (69, 70) might be more valid for reducing co-occurrence of SA and PSU among college students, as the coping-motivated smartphone use are more likely in college students as previously stated.
Although prior studies have suggested that SA and PSU may be affected by sex independently (46, 71, 72), the sex differences have seldom been focused or reported in the studies of their comorbidity (20, 34, 35, 73). Therefore, the sex differences have been explored in the current study specifically, and our results indicated no significant sex differences on the SA-PSU connection, or the bridge centrality in college students. Thus, the comparison of network between sexes underscored more caution in attributing sex differences to these connections, given the current scarcity of robust evidence. Future investigations should aim to consolidate these findings by gathering additional evidence, thereby enabling the formulation of more robust and convincing conclusions. This will not only enrich our theoretical knowledge but also pave the way for more targeted and effective clinical interventions across diverse populations.
The study emphasized the importance of targeted interventions based on the complex connections between PSU and SA among college students. It was suggested that the co-occurrence mechanisms of PSU and SA among college students, as distinct from its among adolescent, necessitated tailored interventions that specifically target different ages (18, 24). Specifically, college students who have the most difficulty in regulating the negative emotions in interpersonal interactions exhibit a greater tendency of PSU problems. Therefore, a more appropriate way to regulate emotions is crucial coping strategies. Furthermore, this study highlighted the significance of SA3 as a significant predictor of symptom communities, underscoring the cultivation of self-regulation flexibility and self-consciousness in colleges and universities (74). To address SA and manage PSU more effectively, and to promote the physical and mental health of college students, interventions for the improvement of emotion regulation ability and adaptive strategies are warranted.
The current study has several limitations that warrant further consideration. Firstly, the findings were based on data from college students, potentially limiting their clinical applicability. Future research is required to examine these findings in clinical samples. Secondly, the cross-sectional design of this study limited our ability to establish the direction of associations between SA and PSU. The discussion, which considered SA as a risk factor for PSU, was primarily based on theoretical grounds (27, 28, 30). Longitudinal studies are necessary to determine the directionality of the observed relationships. Finally, our results may suggested that the interaction between PSU and SA might vary depending on the purpose of smartphone use. It is essential to distinguish between social and non-social PSU when exploring comorbidity issues.
In the current study, we used a symptom-level network approach to explore the relationships between SA and PSU among college students. Our findings not only supported existing theoretical models (e.g. the Pathways Model) but also provided novel insights (e.g. negative reinforcement, smartphone use for social purpose) into the mechanisms behind the co-occurrence of these two constructs. Notably, the bridge centrality results emphasized the significant roles of SA3 and PSU8 in linking SA and PSU among college students. These findings could guide tailored campus prevention and intervention for SA and PSU among this specific age group. For instance, teaching students to cope flexibly with negative moods in social contexts could effectively prevent the worsening of PSU.
The raw data supporting the conclusions of this article will be made available by the authors, without undue reservation.
The studies involving humans were approved by the Medical Ethics Committee of the First Affiliated Hospital of the Air Force Medical University. The studies were conducted in accordance with the local legislation and institutional requirements. The participants provided their written informed consent to participate in this study.
WX: Conceptualization, Funding acquisition, Writing – original draft, Writing – review & editing. XW (2nd Author): Writing – original draft, Writing – review & editing. TF: Writing – original draft. JX: Data curation, Writing – original draft. CL: Writing – review & editing. XW (6th Author): Data curation, Writing – original draft. HW: Data curation, Writing – original draft. LR: Conceptualization, Methodology, Visualization, Writing – review & editing. XL: Supervision, Writing – review & editing.
The author(s) declare financial support was received for the research, authorship, and/or publication of this article. WX involvement in this research was supported by the research start-up fund for postdoctoral in the Air Force Medical University (grant number: qd20230218).
We would like to thank all participants involved in this study.
The authors declare that the research was conducted in the absence of any commercial or financial relationships that could be construed as a potential conflict of interest.
The author(s) declare that no Generative AI was used in the creation of this manuscript.
All claims expressed in this article are solely those of the authors and do not necessarily represent those of their affiliated organizations, or those of the publisher, the editors and the reviewers. Any product that may be evaluated in this article, or claim that may be made by its manufacturer, is not guaranteed or endorsed by the publisher.
The Supplementary Material for this article can be found online at: https://www.frontiersin.org/articles/10.3389/fpsyt.2025.1508756/full#supplementary-material
1. American Psychiatric Association. Diagnostic and statistical manual of mental disorders. 5th Ed. American Psychiatric Association: Washington Dc (2013).
2. Panova T, Carbonell X. Is smartphone addiction really an addiction? J Behav Addict. (2018) 7:252–9. doi: 10.1556/2006.7.2018.49
3. Winkler A, Jeromin F, Doering BK, Barke A. Problematic smartphone use has detrimental effects on mental health and somatic symptoms in A heterogeneous sample of german adults. Comput In Hum Behav. (2020) 113:106500. doi: 10.1016/j.chb.2020.106500
4. Crisafulli MJ, Flori JN, Dunn ME, Dvorak RD. College student alcohol use: understanding the role of alcohol expectancies, social anxiety, social connectedness, and need to belong. Psychiatry. (2023) 87:21–35. doi: 10.1080/00332747.2023.2286845
5. Dougherty EN, Johnson NK, Badillo K, Haedt-Matt AA. Sleep reactivity is associated with social anxiety and disordered-eating behaviors in college students. J Of Ach. (2023) 71:2280–5. doi: 10.1080/07448481.2021.1967359
6. Meng SQ, Cheng JL, Li YY, Yang XQ, Zheng JW, Chang XW, et al. Global prevalence of digital addiction in general population: A systematic review and meta-analysis. Clin Psychol Rev. (2022) 92:102128. doi: 10.1016/j.cpr.2022.102128
7. Oka T, Hamamura T, Miyake Y, Kobayashi N, Honjo M, Kawato M, et al. Prevalence and risk factors of internet gaming disorder and problematic internet use before and during the covid-19 pandemic: A large online survey of Japanese adults. J Psychiatr Res. (2021) 142:218–25. doi: 10.1016/j.jpsychires.2021.07.054
8. Wacks Y, Weinstein AM. Excessive smartphone use is associated with health problems in adolescents and young adults. Front Psychiatry. (2021) 12:669042. doi: 10.3389/fpsyt.2021.669042
9. Zhang Y, Qi Y, Ma Y. The associations between social support and problematic mobile phone use among children and adolescents: A three-level meta-analysis. J Youth Adolesc. (2024). doi: 10.1007/s10964-024-02055-x
10. Cooper D, Anderson T. Interpersonal subtypes within social anxiety: the identification of distinct social features. J Pers Assess. (2019) 101:64–72. doi: 10.1080/00223891.2017.1373116
11. Costache ME, Frick A, Mansson K, Engman J, Faria V, Hjorth O, et al. Higher- and lower-order personality traits and cluster subtypes in social anxiety disorder. PloS One. (2020) 15:E0232187. doi: 10.1371/journal.pone.0232187
12. Leichsenring F, Leweke F. Social anxiety disorder. N Engl J Med. (2017) 376:2255–64. doi: 10.1056/NEJMcp1614701
13. Šipka D, Brodbeck J, Schulz A, Stolz T, Berger T. Factor structure of the social phobia scale (Sps) and the social interaction anxiety scale (Sias) in A clinical sample recruited from the community. BMC Psychiatry. (2023) 23:646. doi: 10.1186/s12888-023-05142-8
14. Coyne SM, Stockdale L, Summers K. Problematic cell phone use, depression, anxiety, and self-regulation: evidence from A three year longitudinal study from adolescence to emerging adulthood. Comput In Hum Behav. (2019) 96:78–84. doi: 10.1016/j.chb.2019.02.014
15. Kong F, Qin J, Huang B, Zhang H, Lei L. The effect of social anxiety on mobile phone dependence among chinese adolescents: A moderated mediation model. Children And Youth Serv Rev. (2020) 108:104517. doi: 10.1016/j.childyouth.2019.104517
16. Pera A. The psychology of addictive smartphone behavior in young adults: problematic use, social anxiety, and depressive stress. Front Psychiatry. (2020) 11:573473. doi: 10.3389/fpsyt.2020.573473
17. Xiao Z, Huang J. The relation between college students’ Social anxiety and mobile phone addiction: the mediating role of regulatory emotional self-efficacy and subjective well-being. Front Psychol. (2022) 13:861527. doi: 10.3389/fpsyg.2022.861527
18. Zhou X, Shen X. Unveiling the relationship between social anxiety, loneliness, motivations, and problematic smartphone use: A network approach. Compr Psychiatry. (2024) 130:152451. doi: 10.1016/j.comppsych.2024.152451
19. Hyett MP, Mcevoy PM. Social anxiety disorder: looking back and moving forward. Psychol Med. (2018) 48:1937–44. doi: 10.1017/S0033291717003816
20. Ran G, Li J, Zhang Q, Niu X. The association between social anxiety and mobile phone addiction: A three-level meta-analysis. Comput In Hum Behav. (2022) 130:107198. doi: 10.1016/j.chb.2022.107198
21. Stein DJ, Lim CCW, Roest AM, De Jonge P, Aguilar-Gaxiola S, Al-Hamzawi A, et al. The cross-national epidemiology of social anxiety disorder: data from the world mental health survey initiative. BMC Med. (2017) 15:143. doi: 10.1186/s12916-017-0889-2
22. Busch PA, Mccarthy S. Antecedents and consequences of problematic smartphone use: A systematic literature review of an emerging research area. Comput In Hum Behav. (2021) 114:106414. doi: 10.1016/j.chb.2020.106414
23. Tang Q, Zou X, Gui J, Wang S, Liu X, Liu G, et al. Effects of childhood trauma on the symptom-level relation between depression, anxiety, stress, and problematic smartphone use: A network analysis. J Affect Disord. (2024) 358:1–11. doi: 10.1016/j.jad.2024.05.018
24. Tao Y, Tang Q, Wang S, Zou X, Ma Z, Zhang L, et al. The impact of long-term online learning on social anxiety and problematic smartphone use symptoms among secondary school students with different levels of fear of missing out: evidence from A symptom network and longitudinal panel network analysis. J Behav Addict. (2024) 13:102–19. doi: 10.1556/2006.2023.00081
25. Long J, Liu TQ, Liao YH, Qi C, He HY, Chen SB, et al. Prevalence and correlates of problematic smartphone use in A large random sample of chinese undergraduates. BMC Psychiatry. (2016) 16:408. doi: 10.1186/s12888-016-1083-3
26. Zhitomirsky-Geffet M, Blau M. Cross-generational analysis of predictive factors of addictive behavior in smartphone usage. Comput In Hum Behav. (2016) 64:682–93. doi: 10.1016/j.chb.2016.07.061
27. Billieux J. Problematic use of the mobile phone: A literature review and A pathways model. Curr Psychiatry Rev. (2012) 8:299–307. doi: 10.2174/157340012803520522
28. Kardefelt-Winther D. A conceptual and methodological critique of internet addiction research: towards A model of compensatory internet use. Comput In Hum Behav. (2014) 31:351–4. doi: 10.1016/j.chb.2013.10.059
29. Billieux J, Philippot P, Schmid C, Maurage P, De Mol J, van der Linden M. Is dysfunctional use of the mobile phone A behavioural addiction? Confronting symptom-based versus process-based approaches. Clin Psychol Psychother. (2015) 22:460–8. doi: 10.1002/cpp.v22.5
30. Canale N, Moretta T, Pancani L, Buodo G, Vieno A, Dalmaso M, et al. A test of the pathway model of problematic smartphone use. J Behav Addict. (2021) 1:181–93. doi: 10.1556/2006.2020.00103
31. Elhai JD, Levine JC, Hall BJ. The relationship between anxiety symptom severity and problematic smartphone use: A review of the literature and conceptual frameworks. J Anxiety Disord. (2019) 62:45–52. doi: 10.1016/j.janxdis.2018.11.005
32. Mitchell L, Hussain Z. Predictors of problematic smartphone use: An examination of the Integrative Pathways Model and the role of age, gender, impulsiveness, excessive reassurance seeking, extraversion, and depression. Behav Sci (Basel, Switzerland). (2018) 8:74. doi: 10.3390/bs8080074
33. Stead H, Bibby PA. Personality, fear of missing out and problematic internet use and their relationship to subjective well-being. Comput Hum Behav. (2017) 76:534–40. doi: 10.1016/j.chb.2017.08.016
34. Annoni AM, Petrocchi S, Camerini AL, Marciano L. The relationship between social anxiety, smartphone use, dispositional trust, and problematic smartphone use: A moderated mediation model. Int J Environ Res Public Health. (2021) 18:2452. doi: 10.3390/ijerph18052452
35. Li S, Feng N, Cui L. Network analysis of social anxiety and problematic mobile phone use in chinese adolescents: A longitudinal study. Addict Behav. (2024) 155:108026. doi: 10.1016/j.addbeh.2024.108026
36. Liu C, Ren L, Rotaru K, Liu X, Li K, Yang W, et al. Bridging the links between big five personality traits and problematic smartphone use: A network analysis. J Behav Addict. (2023) 12:128–36. doi: 10.1556/2006.2022.00093
37. Wei X, An F, Liu C, Li K, Wu L, Ren L, et al. Escaping negative moods and concentration problems play bridge roles in the symptom network of problematic smartphone use and depression. Front Public Health. (2022) 10:981136. doi: 10.3389/fpubh.2022.981136
38. Borsboom D. A network theory of mental disorders. World Psychiatry. (2017) 16:5–13. doi: 10.1002/wps.20375
39. Cramer AO, Waldorp LJ, van der Maas HL, Borsboom D. Comorbidity: A network perspective. Behav Brain Sci. (2010) 33:137–50. doi: 10.1017/S0140525X09991567
40. Hofmann SG, Curtiss J, Mcnally RJ. A complex network perspective on clinical science. Perspect Psychol Sci. (2016) 11:597–605. doi: 10.1177/1745691616639283
41. Jones PJ, Ma R, Mcnally RJ. Bridge centrality: A network approach to understanding comorbidity. Multivariate Behav Res. (2021) 56:353–67. doi: 10.1080/00273171.2019.1614898
42. Mcnally RJ. Network analysis of psychopathology: controversies and challenges. Annu Rev Clin Psychol. (2021) 17:31–53. doi: 10.1146/annurev-clinpsy-081219-092850
43. Ren L, Wang Y, Wu L, Wei Z, Cui LB, Wei X, et al. Network structure of depression and anxiety symptoms in chinese female nursing students. BMC Psychiatry. (2021) 21:279. doi: 10.1186/s12888-021-03276-1
44. Bringmann LF, Eronen MI. Don’t blame the model: reconsidering the network approach to psychopathology. Psychol Rev. (2018) 125:606–15. doi: 10.1037/rev0000108
45. Fried EI, Cramer AOJ. Moving forward: challenges and directions for psychopathological network theory and methodology. Perspect Psychol Sci. (2017) 12:999–1020. doi: 10.1177/1745691617705892
46. Asher M, Asnaani A, Aderka IM. Gender differences in social anxiety disorder: A review. Clin Psychol Rev. (2017) 56:1–12. doi: 10.1016/j.cpr.2017.05.004
47. He X. Relationship between self-esteem, interpersonal trust, and social anxiety of sollege students. Occup Ther Int. (2022) 8088754.
48. Su W, Han X, Yu H, Wu Y, Potenza MN. Do men become addicted to internet gaming and women to social media? A meta-analysis examining genderrelated differences in specific internet addiction. Comput Hum Behav. (2020) 113:106480.1–11. doi: 10.1016/j.chb.2020.106480
49. Rio PD, Lazaro MI, Barco SD, Castano B. Mobile abuse in university students and profiles of victimization and aggression. Adicciones. (2017) 29:245–55. doi: 10.20882/adicciones.837
50. Ezenwaji CO, Aloh HE, Okeke PMD, Osilike CC, Ekwealor NE, Koledoye UL, et al. Managing social anxiety among undergraduate adult education and extra-mural studies students: an intervention study. Med (Baltimore). (2021) 100:E27596. doi: 10.1097/MD.0000000000027596
51. Jin Y, Xu S, Chen C, Wilson A, Gao D, Ji Y, et al. Symptom association between social anxiety disorder, appearance anxiety, and eating disorders among chinese university students: A network analysis to conceptualize comorbidity. Front Public Health. (2022) 10:1044081. doi: 10.3389/fpubh.2022.1044081
52. Lei J, Brosnan M, Ashwin C, Russell A. Evaluating the role of autistic traits, social anxiety, and social network changes during transition to first year of university in typically developing students and students on the autism spectrum. J Autism Dev Disord. (2020) 50:2832–51. doi: 10.1007/s10803-020-04391-w
53. Ramón-Arbués E, Gea-Caballero V, Granada-López JM, Juárez-Vela R, Pellicer-García B, Antón-Solanas I. The prevalence of depression, anxiety and stress and their associated factors in college students. Int J Environ Res Public Health. (2020) 17:7001. doi: 10.3390/ijerph17197001
54. Fenigstein A, Scheier MF, Buss AH. Public and private self-consciousness: assessment and theory. J Of Consulting And Clin Psychol. (1975) 43:522–7. doi: 10.1037/h0076760
55. Ren Y, Yang J, Liu L. Social anxiety and internet addiction among rural left-behind children: the mediating effect of loneliness. Iran J Public Health. (2017) 46:1659–68.
56. Hussain Z, Griffiths MD, Sheffield D. An investigation into problematic smartphone use: the role of narcissism, anxiety, and personality factors. J Behav Addict. (2017) 6:378–86. doi: 10.1556/2006.6.2017.052
57. Liu C, Ren L, Li K, Yang W, Li Y, Rotaru K, et al. Understanding the association between intolerance of uncertainty and problematic smartphone use: A network analysis. Front Psychiatry. (2022) 13:917833. doi: 10.3389/fpsyt.2022.917833
58. Epskamp S, Borsboom D, Fried EI. Estimating psychological networks and their accuracy: A tutorial paper. Behav Res Methods. (2018) 50:195–212. doi: 10.3758/s13428-017-0862-1
59. Isvoranu AM, Epskamp S. Which estimation method to choose in network psychometrics? Deriving guidelines for applied researchers. Psychol Methods. (2023) 28:925–46. doi: 10.1037/met0000439
60. Fruchterman T, Reingold E. Graph drawing by force-directed placement. Software Pract Exper. (1991) 21:1129–64. doi: 10.1002/spe.4380211102
61. Epskamp S, Cramer AOJ, Waldorp LJ, Schmittmann VD, Borsboom D. Qgraph: network visualizations of relationships in psychometric data. J Of Stat Software. (2012) 48:1–18. doi: 10.18637/jss.v048.i04
62. Van Borkulo CD, Van Bork R, Boschloo L, Kossakowski JJ, Tio P, Schoevers RA, et al. Comparing network structures on three aspects: A permutation test. Psychol Methods. (2023) 28:1273–85. doi: 10.1037/met0000476
63. Elhai JD, Gallinari EF, Rozgonjuk D, Yang H. Depression, anxiety and fear of missing out as correlates of social, non-social and problematic smartphone use. Addict Behav. (2020) 105:106335. doi: 10.1016/j.addbeh.2020.106335
64. Shahidin SH, Midin M, Sidi H, Choy CL, Nik Jaafar NR, Mohd Salleh Sahimi H, et al. The relationship between emotion regulation (Er) and problematic smartphone use (Psu): A systematic review and meta-analyses. Int J Environ Res Public Health. (2022) 19:15848. doi: 10.3390/ijerph192315848
65. Elhai JD, Yang H, Fang J, Bai X, Hall BJ. Depression and anxiety symptoms are related to problematic smartphone use severity in chinese young adults: fear of missing out as A mediator. Addict Behav. (2020) 101:105962. doi: 10.1016/j.addbeh.2019.04.020
66. Wolniewicz CA, Tiamiyu MF, Weeks JW, Elhai JD. Problematic smartphone use and relations with negative affect, fear of missing out, and fear of negative and positive evaluation. Psychiatry Res. (2018) 262:618–23. doi: 10.1016/j.psychres.2017.09.058
67. Li X, Li W, Liu M, Xiao W, Zhou H. How does shyness affect chinese college students’ Tendency to mobile phone addiction? Testing the mediating roles of social anxiety and self-control. Front Public Health. (2022) 10:902425. doi: 10.3389/fpubh.2022.902425
68. Shenhav A, Botvinick MM, Cohen JD. The expected value of control: an integrative theory of anterior cingulate cortex function. Neuron. (2013) 79:217–40. doi: 10.1016/j.neuron.2013.07.007
69. Piccirillo ML, Taylor Dryman M, Heimberg RG. Safety behaviors in adults with social anxiety: review and future directions. Behav Ther. (2016) 47:675–87. doi: 10.1016/j.beth.2015.11.005
70. Rejbrand C, Fure B, Sonnby K. Stand-alone virtual reality exposure therapy as A treatment for social anxiety symptoms: A systematic review and meta-analysis. Ups J Med Sci. (2023) 128:9289. doi: 10.48101/ujms.v128.9289
71. Claesdotter-Knutsson E, André F, Fridh M, Delfin C, Hakansson A, Lindström M. Gender-based differences and associated factors surrounding excessive smartphone use among adolescents: cross-sectional study. JMIR Pediatr Parent. (2021) 4:E30889. doi: 10.2196/30889
72. Wu YY, Chou WH. Smartphone addiction, gender and interpersonal attachment: A cross-sectional analytical survey in Taiwan. Digit Health. (2023) 9:20552076231177134. doi: 10.1177/20552076231177134
73. Li J, Zhan D, Zhou Y, Gao X. Loneliness and problematic mobile phone use among adolescents during the covid-19 pandemic: the roles of escape motivation and self-control. Addict Behav. (2021) 118:106857. doi: 10.1016/j.addbeh.2021.106857
Keywords: social anxiety, problematic smartphone use, network analysis, sex difference, college students
Citation: Xing W, Wang X, Feng T, Xie J, Liu C, Wang X, Wang H, Ren L and Liu X (2025) Network analysis of the association between social anxiety and problematic smartphone use in college students. Front. Psychiatry 16:1508756. doi: 10.3389/fpsyt.2025.1508756
Received: 09 October 2024; Accepted: 06 January 2025;
Published: 23 January 2025.
Edited by:
Andras Norbert Zsido, University of Pécs, HungaryReviewed by:
Yanqiang Tao, Beijing Normal University, ChinaCopyright © 2025 Xing, Wang, Feng, Xie, Liu, Wang, Wang, Ren and Liu. This is an open-access article distributed under the terms of the Creative Commons Attribution License (CC BY). The use, distribution or reproduction in other forums is permitted, provided the original author(s) and the copyright owner(s) are credited and that the original publication in this journal is cited, in accordance with accepted academic practice. No use, distribution or reproduction is permitted which does not comply with these terms.
*Correspondence: Lei Ren, cmxfZm1tdUAxNjMuY29t; Xufeng Liu, bHhmX2ZtbXVAMTYzLmNvbQ==
Disclaimer: All claims expressed in this article are solely those of the authors and do not necessarily represent those of their affiliated organizations, or those of the publisher, the editors and the reviewers. Any product that may be evaluated in this article or claim that may be made by its manufacturer is not guaranteed or endorsed by the publisher.
Research integrity at Frontiers
Learn more about the work of our research integrity team to safeguard the quality of each article we publish.