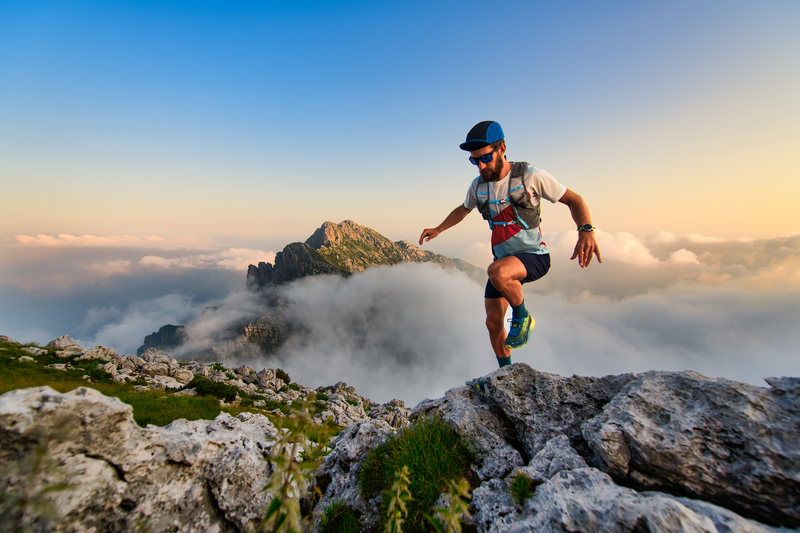
95% of researchers rate our articles as excellent or good
Learn more about the work of our research integrity team to safeguard the quality of each article we publish.
Find out more
ORIGINAL RESEARCH article
Front. Psychiatry , 11 December 2024
Sec. Autism
Volume 15 - 2024 | https://doi.org/10.3389/fpsyt.2024.1518180
Background: Eye tracking (ET) is emerging as a promising early and objective screening method for autism spectrum disorders (ASD), but it requires more reliable metrics with enhanced sensitivity and specificity for clinical use.
Methods: This study introduces a suite of novel ET metrics: Area of Interest (AOI) Switch Counts (ASC), Favorable AOI Shifts (FAS) along self-determined pathways, and AOI Vacancy Counts (AVC), applied to toddlers and preschoolers diagnosed with ASD. The correlation between these new ET metrics and Autism Diagnostic Observation Schedule, Second Edition (ADOS-2) scores via linear regression and sensitivity and specificity of the cut-off scores were assessed to predict diagnosis.
Results: Our findings indicate significantly lower FAS and ASC and higher AVC (P<0.05) in children with ASD compared to their non-ASD counterparts within this high-risk cohort; the significance was not seen in total fixation time neither pupil size (p > 0.05). Furthermore, FAS was negatively correlated with ADOS-2 total scores and social affect (SA) subscale (p < 0.05). Among these new ET metrics, AVC yielded the best sensitivity 88-100% and specificity 80-88% with cut off score 0.305-0.306, followed by FAS and ASC to separate ASD from non-ASD for diagnosis.
Conclusions: This study confirms the utility of innovative ET metrics—FAS, AVC, and ASC—which exhibit markedly improved sensitivity and specificity, enhancing ASD screening and diagnostic processes.
Early diagnosis and intervention are pivotal in determining long-term outcomes for individuals with Autism Spectrum Disorder (ASD). The prognostic implications underscore the necessity for developing readily accessible and effective early detection tools (1–4). Current diagnostic frameworks such as the Diagnostic and Statistical Manual of Mental Disorders, Fifth Edition (DSM-5) (5), and the Autism Diagnostic Observation Schedule, Second Edition (ADOS-2) (6),provide structured criteria for early diagnosis. Nevertheless, these conventional methods are often elaborate, time-consuming, and resource-intensive. This complexity can delay diagnostic and therapeutic interventions, particularly in underserved populations (7, 8). Despite recent advances in diagnostic methodologies, the mean age of diagnosis persists at four to five years (4). Furthermore, disparities in diagnosis times are evident, with ethno-racial minorities and non-English speaking children diagnosed significantly later than their white counterparts (9). Addressing these disparities is critical, underscoring the urgent need for innovative diagnostic tools that facilitate earlier detection and intervention in high-risk populations.
Amidst the subjective limitations of standard assessments, recent research has shifted towards objective biomarkers for ASD diagnosis. Eye tracking (ET) technology has gained prominence as a promising diagnostic tool due to its inherent objectivity and rapid assessment capabilities (10–14). Traditionally utilized in human perception studies and extensively in ASD research, ET technology quantifies eye positions, movements (11, 15), and pupil size dynamics (16–18) to delineate zones of user interest. Distinctive eye movement patterns and gaze behaviors in ASD, such as challenges in interpreting gaze cues, a preference for systematically arranged images over faces, and a lack of right hemispheric dominance for facial processing, have been well-documented (19–22). Essential metrics in ASD ET research include Total Gaze Count (TGC) and Total Fixation Time (TFT), which respectively measure the frequency of gazes and the duration of eye fixation within designated Areas of Interest (AOIs) (11–14). Recent investigations have highlighted significant reductions in TFT across most AOIs for ASD subjects compared to controls in developmental cohorts, reinforcing the diagnostic potential of these parameters (13).
Despite the promising aspects of ET, variability in its protocols and paradigms has historically limited its utility as a consistent diagnostic tool (23, 24). It is important to note that traditionally used ET metrics such as TFT have limited sensitivity and specialty and are unable to identify subtle changes and recent studies have reported newly introduced metrics that can achieve higher accuracies for diagnosis (25). Thus, the present study aims to introduce a set of novel ET metrics such as Area of Interest Switch Counts (ASC), Favored Area of Interest Shifts (FAS), and Area of Interest Vacancy Counts (AVC) with the hypothesis that AVC and the adjunct metrics are able to provide improved accuracy and specificity in the discriminability between ASD and non-ASD cohorts. These metrics are designed to quantify dynamic shifts between AOIs and are hypothesized to reflect fundamental ASD-related deficits such as joint attention, social referencing, and theory of mind. Integrating these new metrics with established diagnostic assessments like ADOS-2, we aim to significantly enhance the specificity and sensitivity of ET for ASD diagnosis. Preliminary findings have facilitated the identification of optimal cutoff scores, providing foundational proof-of-concept and methodologies poised to refine ASD diagnostic approaches through advanced ET metrics.
This study involved thirty-nine individuals aged 18 to 84 months, identified as high-risk for ASD by clinicians or caregivers in Massachusetts and its surrounding states. High-risk status was confirmed via telephone screening prior to enrollment. Inclusion criteria required participants to meet one or more of the following: (1) having at least one sibling with a clinical ASD diagnosis; (2) caregiver or clinician concerns regarding the child’s development in social interaction, play, or other behaviors; (3) scoring in the positive range on the Modified Checklist for Autism in Toddlers (M-CHAT). Participants with major congenital or genetic disorders, or behavioral issues likely to cause significant stress during testing were excluded. Those previously diagnosed with ASD were included without disclosing their diagnosis to the examiner. Subjects were categorized into ASD and non-ASD groups based on DSM-5 criteria, evaluated by two field experts.
The study was conducted according to the guidelines of the Declaration of Helsinki and approved by the Institutional Review Board of Massachusetts General Hospital 2017P001667, 13 July 2018). The secondary use of research samples/data was approved by the Institutional Review Board of Massachusetts General Hospital (2020P004102; January 7, 2021). Written informed consent was obtained from parents or guardians of all subjects involved in the study.
Eye tracking data were collected using a Tobii X3-120 eye tracker, with the screen resolution set to 1024 × 768 pixels, a sampling frequency of 250 Hz, and a spatial resolution of 0.03 degrees. Participants were seated in a dark, soundproof room, 65 cm from a 22-inch widescreen LCD monitor, with their vision centered on the display. Data inclusion required successful completion of a five-point calibration and the full experiment, retaining only data from compliant participants.
Eye tracking stimuli consisted of two videos previously used in related research (13, 14). The first video (25 seconds) featured a woman and a tablet, alternating attention between the two based on the tablet’s activity (turning on/off), testing joint attention capabilities. The second video (10 seconds) displayed a woman silently mouthing the alphabet, focusing on the eyes and mouth as separate areas of interest (AOIs) to examine social communication and early language development cues (18). These two videos were specifically designed and selected to test ASD-related core deficits such as social referencing and contextual understanding of social environments.
ADOS-2, the gold standard in ASD diagnostics, involves several modules selected based on the participant’s age and language development, assessing social interactions, communication, and behaviors (6, 26, 27). It ends with a diagnostic algorithm tailored to maximize diagnostic sensitivity and specificity. Each module’s outcomes are quantified into a calibrated severity score (CSS) from 1 to 10 (28). In the present study, the ADOS-2 modules T, 1, and 2 were utilized and their administration involved two formally trained professionals for ADOS-2; additionally, three trained professionals, each with 1-2 years of specialized experience, performed the eye tracking data collection. The overall evaluation process takes approximately one hour.
Eye tracking raw data was processed using Tobii Pro software. Consistent with prior studies, data segments with less than 25% screen-looking time were excluded, as were participants with fewer than 50% valid trials (29–31). Comparisons of TGC, ASC, FAS, and AVC between ASD and non-ASD groups utilized the Wilcoxon rank-sum test, while discriminant analysis evaluated the ability of AOIs to categorize subjects by ASD severity. Detailed AOI shift analysis within and across different attention time blocks for video 1 was performed. Correlations between TGC, ASC, FAS, AVC, and ADOS-2 total/sub-scores were examined using R (version 4.4.1) to determine the sensitivity and specificity of ET metrics in predicting ASD diagnosis, identifying optimal cutoff scores for effective separation of ASD and non-ASD groups. The optimal ADOS-2 cutoffs were determined independently by two domain experts independently, both of which reported a consistent cutoff of 5 as the optimal value.
For this study cohort, 39 high-risk individuals for ASD were included in data analysis. The participants included 22 ASD individuals and 17 non-ASD individuals. Among them 25 males and 14 females; 15 White (42.8%), 11 Asian (31.4%), and 9 (25.7%) subjects of other races. Table 1 shows the demographic and clinical features of all the participants. There were no significant differences in age or gender between ASD and non-ASD in the two groups; however, their ADOS-2 total, and sub-scores were significantly different as expected (Table 1).
The difference of FAS and AVC between ASD and non-ASD individuals. Video 1 (25s) contains a woman (AOI-1 is her face) on the left side of the screen and a tablet (AOI-2) on the right side of the screen (Figure 1A). The video elapsed a total of 25s divided into four blocks of time 1-2-3-4 as described above in the protocol (Figure 1B): Block 1 is when the tablet is on with pictures moving, meant to draw subjects’ attention to watch; Block 2 is when the woman suddenly turns off the tablet, and we expect subjects to turn and look at the woman’s face, wondering what is going on at this point; Block 3 is when the woman turned on the tablet again; and Block 4 is when the woman turns off the tablet again. The attention shifts were expected during tablet on-off-on-off. The blue bars represent the TGC of non-ASD group, the red bars represent the TGC of ASD group; green colored areas are FAS pathway which are expected normally subjects would do following the sequence of tablet-face-tablet-face vs the opposite. Pink colored areas are unfavored attention shift (UAS) showed on Figure 1B.
Figure 1. (A) Video 1, AOI 1 (face) and AOI 2 (tablet); (B) mean gaze numbers in each second (totally 25s). Blue bars: non-ASD group; Red bars: ASD group. Green areas: Favored AOI Shift (FAS). Pink areas: Unfavored AOI shift (UAS).
The TGC was analyzed for both ASD and non-ASD groups in two AOIs cross the different time blocks (Table 2). We found that non-ASD individuals showed significant TGC differences cross time blocks 1→2, 2→3 and 3→4 for both AOI areas. Instead, ASD children had no TGC difference during 1→2 and 2→3 shifts, and only started to show difference during 3→4 shift for both AOI areas; meanwhile the difference between both subject groups showed significance 1→2 and 2→3 but not 3→4 (Table 2). When we further investigated FAS pathway and AVC which is subject’s gaze counts on neither AOIs, we found that ASD group had significantly reduced TGC along FAS (p < 0.00001) and significantly increased in AVC (p < 0.00001) across different time blocks relative to non-ASD group (Table 3).
Table 2. The comparison of significance of total gaze counts cross time blocks in ASD vs non-ASD groups.
Video 2 (10 seconds) consisted of a woman sitting and mouthing the alphabet without sound. We defined two important AOIs: AOI-1 was defined as the eye area and AOI-2 was defined as the mouth area (Figure 2); We studied their TGC in these AOIs, and ASC which are the switches between these two AOIs. Red dots represent the ASD group and blue dots represent non-ASD group. Figure 2B shows TGC and Figure 2C showed TFT for both groups. We can see the different density distribution pattern between the ASD and the non-ASD groups. The ASD group has a more diverse and scattered distribution.
Figure 2. Video 2 shows a woman mouthing the alphabet without sound (A) AOI-1 for both eye areas (green areas), and AOI-2 for nose and mouth area (yellow areas). (B) distribution of total gaze counts (TGG). (C) distribution of total fixation time (TFT). Blue dots: non-ASD group, red dots: ASD group.
When we investigated the detailed TGC, ASC, and AVC, we found that the ASD group had significantly less ASC between AOI 1 and 2 (p = 0.0452), and significantly more AVC (p = 0.000017) vs the non-ASD group, while TGC was found to be significantly smaller in the ASD group than the non-ASD group for AOI-1 area (p = 0.00379), not AOI-2 area (p = 0.6537). We also compared with the old EP metrics TFT and pupil size, they all showed no significant difference between two groups (p > 0.05), demonstrating that AVC and ASC had significantly higher sensitivity than the old metrics (Table 4).
We conducted a correlation study with regression analysis between the significant ET index and ASD severity based on ADOS-2 scores. We found that FAS-UAS for video 1 negatively correlated with ADOS-2 total scores (r = -0.373, p = 0.01948), SA scores (r = -0.33, p = 0.0412) and RRB scores (r = -0.25, p = 0.124). When we use ADOS-2 total CSS cut off score 5 and FAS-UAS cut off score 641.1, we got sensitivity 91%, specificity 72% (Figure 3).
Figure 3. Video 1 (Face and Tablet) FAS-UAS vs ADOS-2 total scores and the linear regression fit. Linear fit: gaze = -72.841*ADOS-2 total scores + 1005.3. Correlation = -0.373. If ADOS-2 total scores cutoff = 5, the gaze cutoff was calculated as: gaze cutoff = 641.1 (below would be diagnosed), specificity = 0.91, sensitivity = 0.72, p = 0.01948.
When we compared ASD (red dots) vs non-ASD (blue dots) group, we found that AVC had the best sensitivity and specificity among all the ET metrics (new or old), the results were consistent in both video 1 (sensitivity 88%, specificity 88%, p < 0.00001, cut-off score 0.305) showed in Figure 4, and video 2 (sensitivity 100%, specificity 80%, p < 0.000045, cut-off score 0.306) in Figure 5. ASC for video 2 had sensitivity 71%, specificity 64%, p = 0.04523, and cut off score 4.5.
Figure 4. Video 1 (Face and Tablet) VAC vs time unit distributions for non-ASD (round) and ASD (triangle). Results based on the cutoff effect: cutoff = 0.305 (above would be diagnosed), Specificity = 0.88, Sensitivity = 0.88, p < 0.00001.
Figure 5. Video 2 (Speaking) VAC vs time unit distributions for non-ASD (round) and ASD (triangle). Results based on the cutoff effect: cutoff = 0.306 (above would be diagnosed), specificity = 1.00, sensitivity = 0.80, p = 0.000045.
Previous investigations into ET as a diagnostic tool for ASD have shown promising results, such as notable reductions in TFT of AOIs among ASD subjects (13, 19). However, the diversity in ET protocols and paradigms has often limited its reliability as a diagnostic instrument (23). For example, the traditionally used ET metrics such as TFT have limited sensitivity and specialty in differentiating between ASD and non-ASD individuals. Nonetheless, ongoing research have introduced metrics that achieves higher accuracies for the diagnosis of ASD (25). Given these advancements, our study aims to explore alternatives to the previous efforts through the discovery of a novel set of ET metrics—FAS, ASC, and AVC—designed to enhance diagnostic accuracy by capturing subtle behavioral markers fundamental to ASD.
The introduction of FAS versus UAS aims to differentiate between typical and atypical attention shifts, reflecting an individual’s ability to prioritize relevant stimuli dynamically. This approach underscores cognitive flexibility, a critical aspect often impaired in ASD. ASC and AVC, respectively, quantify transitions between competitive targets and the absence of gaze on expected targets, providing a nuanced understanding of attentional engagement and disengagement in ASD individuals.
Our findings indicate these new metrics are more sensitive and specific than traditional measures like TFT and pupil size, particularly in distinguishing ASD from non-ASD in a cohort of high-risk individuals. Furthermore, our findings justify an enhanced ability for the metrics in capturing ASD core symptom differences between those with and without ASD relative to previous findings. For instance, our analysis revealed that ASD participants exhibited significantly fewer FAS and heightened UAS during tasks designed to test joint attention (JA), a fundamental social communicative skill that is typically disrupted in ASD (32). Notably, the correlation of these metrics with ADOS-2 scores suggests that the severity of social affect impairments is inversely related to the engagement in favored gaze patterns.
The AVC metric emerged as particularly insightful, revealing that ASD individuals frequently failed to engage with designated AOIs—indicative of the inattentive phenomenon often reported anecdotally by caregivers of ASD individuals. The statistical robustness of AVC (sensitivity of 88-100% and specificity of 80-88% across various tests) supports its potential utility in clinical settings, emphasizing its role in detecting divergent attention patterns.
This study not only reaffirms the utility of ET in ASD diagnosis but also introduces new avenues for understanding the neural and cognitive underpinnings of the disorder. The reduced tendency of ASD individuals to shift attention as expected may reflect broader deficits in theory of mind and social cognition, potentially linked to underlying neural abnormalities in networks involving the cerebellum and prefrontal cortex (33, 34).
While our results are promising, the specificity of the participant cohort—high-risk individuals rather than a broader demographic including non-ASD individuals—necessitates cautious interpretation. Future research should aim to validate these findings across more diverse populations and clinical settings, enhancing the generalizability of the metrics. Other limitations should be noted, such as limited sample size and lack of ethnic diversity, which may limit the generalizability of our findings to a broader and more diverse population.
Despite the mentioned limitations, our study positions ET not only as a feasible diagnostic tool for early ASD screening but also highlights its potential to provide deeper insights into the distinct neurodevelopmental trajectories associated with the disorder. The development of ET metrics like FAS, ASC, and AVC marks a significant advance in the objective measurement of core ASD features, paving the way for more targeted interventions and therapies tailored to individual neurodevelopmental profiles. As we continue to refine these metrics and explore their clinical implications, large-scale studies will be essential for establishing their efficacy and integrating them into routine clinical practice for ASD screening and formal clinical diagnosis.
The raw data supporting the conclusions of this article will be made available by the authors, without undue reservation.
The studies involving humans were approved by Massachusetts General Hospital. The studies were conducted in accordance with the local legislation and institutional requirements. Written informed consent for participation in this study was provided by the participants’ legal guardians/next of kin. Written informed consent was obtained from the individual(s) for the publication of any potentially identifiable images or data included in this article.
RW: Data curation, Formal analysis, Investigation, Methodology, Software, Validation, Visualization, Writing – original draft, Writing – review & editing. KK: Project administration, Supervision, Writing – original draft, Writing – review & editing. KL: Visualization, Writing – original draft, Writing – review & editing. X-JK: Conceptualization, Funding acquisition, Investigation, Methodology, Project administration, Resources, Supervision, Writing – original draft, Writing – review & editing.
The author(s) declare financial support was received for the research, authorship, and/or publication of this article. Financial support for this research was provided by Massachusetts General Hospital (grant numbers 230361 and 233263).
The authors declare that the research was conducted in the absence of any commercial or financial relationships that could be construed as a potential conflict of interest.
The author(s) declare that no Generative AI was used in the creation of this manuscript.
All claims expressed in this article are solely those of the authors and do not necessarily represent those of their affiliated organizations, or those of the publisher, the editors and the reviewers. Any product that may be evaluated in this article, or claim that may be made by its manufacturer, is not guaranteed or endorsed by the publisher.
1. Towle PO, Patrick PA. Autism spectrum disorder screening instruments for very young children: A systematic review. Autism Res Treat. (2016) 2016:4624829. doi: 10.1155/2016/4624829
2. Dow D, Guthrie W, Stronach ST, Wetherby AM. Psychometric analysis of the Systematic Observation of Red Flags for autism spectrum disorder in toddlers. Autism. (2017) 21:301–9. doi: 10.1177/1362361316636760
3. Kantzer A-K, Fernell E, Westerlund J, Hagberg B, Gillberg C, Miniscalco C. Young children who screen positive for autism: Stability, change and “comorbidity” over two years. Res Dev Disabil. (2018) 72:297–307. doi: 10.1016/j.ridd.2016.10.004
4. Zwaigenbaum L, Penner M. Autism spectrum disorder: advances in diagnosis and evaluation. BMJ. (2018) 361:k1674. doi: 10.1136/bmj.k1674
5. American-Psychiatric-Association. Diagnostic and statistical manual of mental disorders: DSM-5. 5th edition. Washington, DC: American Psychiatric Association (2013).
6. Lord C, Risi S, Lambrecht L, Cook EH, Leventhal BL, DiLavore PC, et al. The Autism Diagnostic Observation Schedule—Generic: A standard measure of social and communication deficits associated with the spectrum of autism. JADD. (2000) 30:205–23.
7. Monteiro SA, Dempsey J, Broton S, Berry L, Goin-Kochel RP, Voigt RG. Early intervention before autism diagnosis in children referred to a regional autism clinic. J Dev Behav Pediatr. (2016) 37:15–9. doi: 10.1097/DBP.0000000000000241
8. Rotholz DA, Kinsman AM, Lacy KK, Charles J. Improving early identification and intervention for children at risk for autism spectrum disorder. Pediatrics. (2017) 139:e20161061. doi: 10.1542/peds.2016-1061
9. Stahmer AC, Vejnoska S, Iadarola S, Straiton D, Segovia FR, Luelmo P, et al. Caregiver voices: Cross-cultural input on improving access to autism services. J Racial Ethn. Health Disparities. (2019) 6:752–73. doi: 10.1007/s40615-019-00575-y
10. Gliga T, Bedford R, Charman T, Johnson MH, Team B. Enhanced visual search in infancy predicts emerging autism symptoms. Curr Biol. (2015) 25:1727–30. doi: 10.1016/j.cub.2015.05.011
11. Helminen TM, Leppänen JM, Eriksson K, Luoma A, Hietanen JK, Kylliüainen A. Atypical physiological orienting to direct gaze in low-functioning children with autism spectrum disorder. Autism Res. (2017) 10:810–20. doi: 10.1002/aur.2017.10.issue-5
12. Kong X-J, Wang BK, Park J, Kong J. Introduction of a new video-based eye tracking paradigm for early detection of ASD. N.A.J. Med Sci. (2017) 10:133–5. doi: 10.7156/najms.2017.1004133
13. Kong X-J, Wei Z, Sun B, Tu Y, Huang Y, Cheng M, et al. Different eye tracking patterns in autism spectrum disorder in toddler and preschool children. Front Psychiatry. (2022) 13:899521. doi: 10.3389/fpsyt.2022.899521
14. Wan G, Kong X, Sun B, Yu S, Tu Y, Park J, et al. Applying eye tracking to identify autism spectrum disorder in children. J Autism Dev Disord. (2019) 49:209–15. doi: 10.1007/s10803-018-3690-y
15. Black MH, Chen NTM, Iyer KK, Lipp OV, Bölte S, Falkmer M, et al. Mechanisms of facial emotion recognition in autism spectrum disorders: Insights from eye tracking and electroencephalography. Neurosci Biobehav Rev. (2017) 80:488–515. doi: 10.1016/j.neubiorev.2017.06.016
16. Lynch GTF, James SM, VanDam M. Pupillary response and phenotype in ASD: Latency to constriction discriminates ASD from typically developing adolescents. Autism Res. (2018) 11:364–75. doi: 10.1002/aur.2018.11.issue-2
17. Nyström P, Gliga T, Jobs EN, Gredebüack G, Charman T, Johnson MH, et al. Enhanced pupillary light reflex in infancy is associated with autism diagnosis in toddlerhood. Nat Commun. (2018) 9:1678. doi: 10.1038/s41467-018-03985-4
18. Artoni P, Piffer A, Vinci V, LeBlanc J, Nelson CA, Hensch TK, et al. Deep learning of spontaneous arousal fluctuations detects early cholinergic defects across neurodevelopmental mouse models and patients. Proc Natl Acad Sci U. S. A. (2020) 117:23298–303. doi: 10.1073/pnas.1820847116
19. Papagiannopoulou EA, Chitty KM, Hermens DF, Hickie IB, Lagopoulos J. A systematic review and meta-analysis of eye-tracking studies in children with autism spectrum disorders. Soc Neurosci. (2014) 9:610–32. doi: 10.1080/17470919.2014.934966
20. Fujioka T, Inohara K, Okamoto Y, Masuya Y, Ishitobi M, Saito DN, et al. Gazefinder as a clinical supplementary tool for discriminating between autism spectrum disorder and typical development in male adolescents and adults. Mol Autism. (2016) 7:19. doi: 10.1186/s13229-016-0083-y
21. Frazier TW, Klingemier EW, Parikh S, Speer L, Strauss MS, Eng C, et al. Development and validation of objective and quantitative eye tracking-based measures of autism risk and symptom levels. J Am Acad Child Adolesc. Psychiatry. (2018) 57:858–66. doi: 10.1016/j.jaac.2018.06.023
22. Strathearn L, Kim S, Bastian DA, Jung J, Iyengar U, Martinez S, et al. Visual systemizing preference in children with autism: A randomized controlled trial of intranasal oxytocin. Dev Psychopathol. (2018) 30:511–21. doi: 10.1017/S0954579417001018
23. Mastergeorge AM, Kahathuduwa C, Blume J. Eye-tracking in infants and young children at risk for autism spectrum disorder: A systematic review of visual stimuli in experimental paradigms. J Autism Dev Disord. (2021) 51:2578–99. doi: 10.1007/s10803-020-04731-w
24. Jeyarani RA, Senthilkumar R. Eye tracking biomarkers for autism spectrum disorder detection using machine learning and deep learning techniques: review. Res Autism Spectr. Disord. (2023) 108:102228. doi: 10.1016/j.rasd.2023.102228
25. Lencastre P, Lotfigolian M, Lind PG. Identifying autism gaze patterns in five-second data records. Diagnostics. (2024) 14:1047. doi: 10.3390/diagnostics14101047
26. Luyster R, Gotham K, Guthrie W, Coffing M, Petrak R, Pierce K, et al. The Autism Diagnostic Observation Schedule-toddler module: a new module of a standardized diagnostic measure for autism spectrum disorders. J Autism Dev Disord. (2009) 39:1305–20. doi: 10.1007/s10803-009-0746-z
27. Falkmer T, Anderson K, Falkmer M, Horlin C. Diagnostic procedures in autism spectrum disorders: a systematic literature review. Eur Child Adolesc. Psychiatry. (2013) 22:329–40. doi: 10.1007/s00787-013-0375-0
28. Hus V, Gotham K, Lord C. Standardizing ADOS domain scores: separating severity of social affect and restricted and repetitive behaviors. J Autism Dev Disord. (2014) 44:2400–12. doi: 10.1007/s10803-012-1719-1
29. Pierce K, Conant D, Hazin R, Stoner R, Desmond J. Preference for geometric patterns early in life as a risk factor for autism. Arch Gen Psychiatry. (2011) 68:101–9. doi: 10.1001/archgenpsychiatry.2010.113
30. Chawarska K, Ye S, Shic F, Chen L. Multilevel differences in spontaneous social attention in toddlers with autism spectrum disorder. Child Dev. (2016) 87:543–57. doi: 10.1111/cdev.2016.87.issue-2
31. Wang Q, Hu Y, Shi D, Zhang Y, Zou X, Li S, et al. Children with autism spectrum disorder prefer looking at repetitive movements in a preferential looking paradigm. J Autism Dev Disord. (2018) 48:2821–31. doi: 10.1007/s10803-018-3546-5
32. Mundy P. A review of joint attention and social-cognitive brain systems in typical development and autism spectrum disorder. Eur J Neurosci. (2018) 47:497–514. doi: 10.1111/ejn.2018.47.issue-6
33. Kelly E, Meng F, Fujita H, Morgado F, Kazemi Y, Rice LC, et al. Regulation of autism-relevant behaviors by cerebellar-prefrontal cortical circuits. Nat Neurosci. (2020) 23:1102–10. doi: 10.1038/s41593-020-0665-z
34. Clausi S, Olivito G, Siciliano L, Lupo M, Laghi F, Baiocco R, et al. The cerebellum is linked to theory of mind alterations in autism. A direct clinical and MRI comparison between individuals with autism and cerebellar neurodegenerative pathologies. Autism Res. (2021) 14:2300–13. doi: 10.1002/aur.v14.11
Keywords: autism spectrum disorder (ASD), eye tracking (ET), area of interest (AOI), gaze abnormality, early diagnosis
Citation: Wang RK, Kwong K, Liu K and Kong X-J (2024) New eye tracking metrics system: the value in early diagnosis of autism spectrum disorder. Front. Psychiatry 15:1518180. doi: 10.3389/fpsyt.2024.1518180
Received: 30 October 2024; Accepted: 25 November 2024;
Published: 11 December 2024.
Edited by:
Cheol E. Han, Korea University, Republic of KoreaReviewed by:
Matthias Dose, kbo Kliniken des Bezirks Oberbayern, GermanyCopyright © 2024 Wang, Kwong, Liu and Kong. This is an open-access article distributed under the terms of the Creative Commons Attribution License (CC BY). The use, distribution or reproduction in other forums is permitted, provided the original author(s) and the copyright owner(s) are credited and that the original publication in this journal is cited, in accordance with accepted academic practice. No use, distribution or reproduction is permitted which does not comply with these terms.
*Correspondence: Xue-Jun Kong, eGtvbmcxQG1naC5oYXJ2YXJkLmVkdQ==
Disclaimer: All claims expressed in this article are solely those of the authors and do not necessarily represent those of their affiliated organizations, or those of the publisher, the editors and the reviewers. Any product that may be evaluated in this article or claim that may be made by its manufacturer is not guaranteed or endorsed by the publisher.
Research integrity at Frontiers
Learn more about the work of our research integrity team to safeguard the quality of each article we publish.