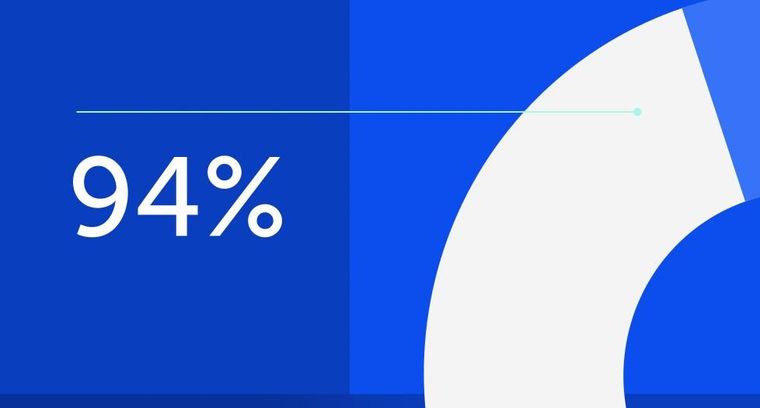
94% of researchers rate our articles as excellent or good
Learn more about the work of our research integrity team to safeguard the quality of each article we publish.
Find out more
ORIGINAL RESEARCH article
Front. Psychiatry, 20 December 2024
Sec. Molecular Psychiatry
Volume 15 - 2024 | https://doi.org/10.3389/fpsyt.2024.1499395
This article is part of the Research TopicThe Individual and Joint Contributions of Molecular and Environmental Factors on Gene Expression and Psychopathology DevelopmentView all 6 articles
Introduction: Research has yet to examine the interplay between indices of environmental risk and resilience processes and genetic predisposition for epigenetic aging in predicting early adolescent depressive symptoms. In the current study we examine whether adverse life events and parental acceptance moderate polygenic predisposition for GrimAge epigenetic aging in predicting trajectories of depressive symptoms across early adolescence.
Method: Using data from the Adolescent Brain Development Study (ABCD, N = 11,875), we created polygenic scores for GrimAge, and examined whether exposure to adverse life events and parental acceptance moderated the relation between genetic risk and depressive symptom trajectories from age 10/11 to 12/13 using growth mixture modelling. We examined models separately in European American (EA), African American (AA), and Latinx (LX) subgroups of ABCD.
Results: In the EA and AA subgroups, adverse life events moderated polygenic scores for GrimAge such that there was increased likelihood of membership in a higher vs. lower depression trajectory.
Discussion: We extend literature by identifying genetic contributions to epigenetic aging as a depression diathesis in adolescence. Findings also highlight the detrimental role of adverse life events in exacerbating genetic risk for the development of depression in adolescence.
Developmental science is replete with examples of environmental stress-induced changes to the epigenome that then influence protein expression and downstream physiology, emotion, cognition, and behavior, including mental health symptoms and disorders. Seldom studied are the genetic influences on epigenetic variation, such as histone acetylation and methylation. Some alleles, for example, are more likely to be methylated than others, necessitating the study of genetic influences on epigenetic variation. Epigenetic clocks capture combinations of DNA methylation patterns (1). Biological age indexed by epigenetic clocks are more reliable measures of aging than chronological age (2), and importantly, variation in these clocks predicts mental health problems such as depression (3, 4). Accumulating evidence indicates that epigenetic clocks are heritable (5, 6). The second-generation epigenetic clock GrimAge is currently the best predictor of health span and lifespan (7). A recent Genome Wide Association Study (GWAS) (6) generated summary effect sizes for SNP prediction of GrimAge, providing the statistics needed for forming a polygenic risk score representing genomic influences on GrimAge. Polygenic scores aggregate variation across thousands of genetic variants across the genome, providing a cumulative measure of genetic predisposition (8). In the current study, we present a novel examination of whether polygenic predisposition for GrimAge epigenetic aging is moderated by adverse life events, as a risk factor, and parent acceptance, as a resilience factor, in predicting early adolescent depression.
Developmental psychopathology theory emphasizes the cumulative salience of multiple levels of risk and resilience within the social environment over the course of psychopathology (9, 10). Separate from this, the diathesis-stress theory of depression proposes that preexisting risk factors, such as genetic predisposition, can be exacerbated by risky environments, increasing likelihood of depression (11, 12). Conversely, within a resilience framework, positive environmental influences may buffer genetic predisposition for depression (13). As an extension and integration of these theories, the gene-environment cascade theoretical framework posits that that the interplay between genetic predisposition and the environment can have cascading effects that alter trajectories of mental health over time (14). Leveraging this framework, we expand traditional main effect genetic association models to include risk and resilience processes and developmental outcomes. Furthermore, we account for the known heterogeneity in depression by allowing for developmental subgroups of depression using growth mixture modeling.
Adverse life events refers to experiences or exposure to traumatic or stressful events. A robust literature including a recent meta-analysis indicates that adverse life events are associated with greater incidence of major depressive disorder before the age of 18 (15). The associations between adverse life events and increases in depression and depressive symptoms in childhood and adolescence have also been corroborated in large international and national samples (16, 17). Thus, adverse life events represent a compelling index of environmental risk during childhood. Moreover, there is ample evidence that risk for depression results from the interplay between adverse life events and genetic and epigenetic predispositions.
A recent review concluded that the interplay between genetic predisposition and adverse life events in childhood represents a classic example of gene-environment interaction (GxE) in mental disorder (18). This is supported by an umbrella review indicating that childhood risk for depression is consistent with a diathesis-stress framework resulting, in part, from adverse environmental exposure and genetic and/or epigenetic predispositions (19). Moreover, this review and its cited literature notes that variability in DNA methylation results from both genetic and environmental variation (20). This aligns with another review of biological factors in depression indicating that one route by which adverse life events in childhood contribute to depression is via the interplay between genetic and epigenetic systems (21). Thus, there is theoretical and research evidence indicating that genetic predisposition for epigenetic variation may be moderated by adverse life events in predicting depressive symptoms in early adolescence.
Parent acceptance encompasses warm, involved, and supportive behaviors a parent expresses towards their child. Four meta-analysis support associations between greater parental warmth and lower childhood/adolescent depression (22–25). Compared to GxE research on adverse life events, less research has examined parent acceptance as moderating genetic predisposition in predicting depression. One study found an interaction between polygenic predisposition for genetic sensitivity and parental warmth associated with depressive symptoms, but the polygenic index was based on only a few candidate genes (26). Using a twin model, parent nurturance moderated heritability for psychological resilience (27). More commonly, GxE studies have examined single candidate genes, negative aspects of parenting, and externalizing outcomes (28). This prompts the need for greater examination of positive parenting as a resilience factor operating as a buffer between genetic risk and pathways to early adolescent depression.
The current study examined whether genetic predisposition for GrimAge epigenetic aging was moderated by adverse life events and parent acceptance in predicting depressive symptom trajectories across early adolescence. Due to known heterogeneity in depression development, we used a person-centered approach to uncover depressive symptom classes across early adolescence separately within EA, AA, and LX subgroups. Person-centered approaches identify distinct patterns of behavior for subgroups of individuals whereas variable-centered approaches collapse variance in a behavior across individuals which can miss heterogeneity in the group (29). Growth Mixture Modelling (GMM) is one person-centered approach that identifies subgroups based on patterns of individual-level trajectories on a specific trait or behavior (30). We examined trajectories and genetic effects separately in EA, AA, and LX subgroups because there can be underlying variation in genetic ancestry across race and ethnicity (e.g., allele frequency, linkage disequilibrium patterns) which necessitate examining effects separately within each racial/ethnic group (31).
We hypothesized that genetic predisposition for GrimAge would be associated with greater likelihood of membership in trajectories with higher depressive symptoms. We hypothesized that adverse life events would moderate the effect of polygenic predisposition for GrimAge such that the association between genetic risk and membership in higher vs. lower depressive symptom trajectories would be stronger among youth experiencing more adverse life events. Conversely, we hypothesized that the association between genetic risk and membership in higher depressive symptom vs. lower trajectories would be weaker among youth who report higher levels of parental acceptance.
The Adolescent Brain Cognitive Development (ABCD; N = 11,875) Study is a large longitudinal study of youth in the United States with annual assessments on behavioral, social, and neurocognitive functioning starting at age 9/10 with four waves of data available to age 12/13. Youth in ABCD are 47.8% female and racially/ethnically diverse (52.1% non-Hispanic White, 15.0% non-Hispanic Black, 20.3% Hispanic/Latinx, 2.1% Asian, and 10.5% other [e.g., multiracial]). At age 9/10, median combined family income was $75k to $100k, with approximately 20% of the sample reporting earning $35k or less. The current study included data from the age 10/11, 11/12, and 12/13 assessments. Youth were included if they had genetic, depressive symptom, and environmental data and were White/European American (EA, n = 6,043; 47% female), Black/African American (AA, n = 1,640; 50% female), or Hispanic/Latinx (LX, n = 2,283; 48% female).
Youth in ABCD were primarily recruited using a probability sampling of schools located within 21 national study sites (32). Parents provided written informed consent for their own and their child’s participation and youth provided assent. Baseline data collection for age 9/10 began in September, 2016, with annual follow ups. Youth in ABCD are assessed in an array of domains encompassing psychosocial and family functioning, physical health, contextual and cultural environment, brain imaging, and whole genome genotyping. Data are released through the NIMH Data Archive. Data used in the present study came from ABCD data release 5.0.
Saliva samples were collected from youth at the age 9/10 assessment (33), which were genotyped by the Rutgers University Cell and DNA Repository (RUCDR). DNA from saliva samples was genotyped on the Smokescreen Genotyping Array (34). RUCDR performed DNA quality controls based on calling signals and variant call rates, and the quality-controlled genotyping ABCD data contains 11,099 unique individuals with 516,598 genetic variants. Imputation was performed via the TOPMed imputation server using mixed ancestry and Engle v2.4 phasing. Single nucleotide polymorphisms (SNPs) with a genotyping rate < 0.95 or that violated Hardy–Weinberg equilibrium (p < 10-6) or with minor allele frequency < 0.01 were excluded from analysis.
We created the PGS based on summary statistics from McCartney et al. (6), the largest published trans-ancestral genome-wide association study (GWAS) on DNA methylation biomarkers of aging. In McCartney et al., GWAS were performed on multiple indices of DNA methylation in approximately 40,000 individuals separately for European American and Black/African American samples (European American N = 34,710; Black/African American N = 6,195). We leveraged summary statistics to create a PGS characterizing genetic predisposition for GrimAge (Grim-PGS) in ABCD after filtering and matching discovery, target, and reference samples. We formed Grim-PGS using the PRS-CSx method, which uses a Bayesian regression and continuous shrinkage method (35, 36). Using GWAS summary statistics, PRS-CSx estimates posterior effect sizes for SNPs which are inferred under coupled continuous shrinkage priors across multiple populations, yielding more accurate effect size estimation. Grim-PGS scores were calculated using joint modelling across EA and AA GWAS summary statistics via coupled shrinkage priors (36). Final Grim-PGS were based on posterior PRS-CSx weights and created using the score procedure in PLINK 1.9 (37). Given that no GWAS on DNA methylation exist in Latinx samples, we used Grim-PGS meta-analyzed across EA and AA GWAS generated by PRS-CSx, which have shown enhanced portability across populations (36). An overview on the underlying principes of polygenic score creation can be found in Kachuri et al. (2024) (38).
To account for potential population stratification, within each ancestry, the first 10 genetic ancestry principal components were extracted based on ancestry informative markers, which were residualized from the Grim-PGS for each ancestral group. Final Grim-PGS were standardized for ease of interpretation.
Youth reported on their significant life events using the Adverse Life Events scale (39–43). This scale captures youth experience of adverse life events on 25 items (e.g., “Saw crime or accident”, “Mother or father lost job”; yes (1) or no (0)). Each item was followed by youth’s perception of the event (“Was this a good or bad experience?”; mostly good (1) or mostly bad (2)). Total number of negative adverse life events were summed and youth experiencing greater than 10 events were recoded as 11 to help address sparsity of datapoints (<1%), limit the influence of extreme values, and in-line with past research on adverse experiences (44, 45).
Youth reported on parenting using the Child Report of Behavior Inventory (46, 47). Five items from the acceptance subscale capture youth perception of their parent’s warmth, acceptance, and responsiveness (e.g., “Is able to make me feel better when I am upset”). Response options ranged from 1 (not at all) to 3 (very much). Items were coded to reflect greater acceptance and mean composited (Cronbach’s alpha = .72).
Parents reported on their child’s behavior using the Child Behavior Check List (48). The DSM-oriented depression subscale captures depressive symptoms during the past 6 months based on 13 items (e.g., “Unhappy, sad, or depressed” and “There is very little s/he enjoys”) on a three-point scale; not true (0) to very true/often true (2). Items were coded to reflect greater depressive symptoms and mean composited within study wave (Cronbach’s alphas ranged from .74 to .79).
Extant research indicates that both depression and epigenetic aging can vary by sex, pubertal status, and income (49–51). Therefore, we controlled for sex recoded to binary for analyses (1 = male, 2 = female), income (1 = less than $5000 to 10 = $200,000 and greater), and pubertal status (1 = prepuberty to 5 = postpuberty) and examined Grim-PGS by covariate interactions (52).
There are potential differences in polygenic score functioning across racial and ethnic groups based on genetic ancestry (31). Therefore, we conducted all analyses separately by racial/ethnic subgroup. Using Growth Mixture Modelling (GMM), we examined trajectories of depressive symptoms across ages 10-11, 11-12, and 12-13 separately for EA, AA, and LX subgroups in Mplus v.8.8 including intercept, slope, and quadratic terms. We did not include wave 9/10 depressive symptoms in GMM as youth report of adverse life events was not available until age 10/11. We examined iterative GMM solutions starting with 1 class and increasing to 5 classes. We examined model fit based on Akaike Information Criterion (AIC), Bayesian Information Criterion (BIC), adjusted BIC (aBIC), Vuong-Lo-Mendell-Rubin Likelihood Ratio Test (VLMR), Bootstrap Likelihood Ratio Test (BLRT), entropy, class sample proportions, and theoretical interpretability (53). Full information maximum likelihood was used to handle missing data based upon the missing at random (MAR) assumption.
Once we identified the optimal class solution, we examined associations between our predictors and covariates with depression trajectories separately within the EA, AA, and LX groups, using the R3step method (54). The R3step method estimates latent classes based on indicators (e.g., depression), creates a most likely latent class variable based on the posterior distribution, and regresses the predictor(s) on class membership. The R3step method tests for likelihood of trajectory membership across pairwise class comparisons which we examined using the lowest depression trajectory as the reference group.
Within the optimal class solution, we tested two models within each subgroup. The first model examined associations between the Grim-PGS, adverse life events, the Grim-PGS by adverse life events interaction term, and covariates with depression trajectories. The second model examined associations between the Grim-PGS, parent acceptance, the Grim-PGS by parent acceptance interaction term, and covariates with depression trajectories. Based on methodological recommendations, within all models we examined for Grim-PGS by covariate interactions, and non-significant interactions were trimmed from final models (52).
Descriptive statistics and correlations can be found in Table 1. There were relatively low levels of adverse life events and relatively high levels of parent acceptance across EA, AA, and LX subgroups. Depressive symptoms were also low in all subgroups. There were significant racial/ethnic differences in youth’s experience of adverse life events, with the highest levels in the AA subgroup and lowest levels in the EA subgroup. Parental acceptance was highest in the EA subgroup and lowest in the AA subgroup. Depressive symptoms were lower in the AA subgroup compared to both the EA and LX subgroups. Within the EA subgroup the Grim-PGS was associated with lower parent acceptance and greater depression at age 10/11. Within all subgroups, adverse life events were associated with higher youth depression, and parent acceptance was associated with lower youth depression.
Table 1. Means, standard deviations, and bivariate correlations between study variables by racial/ethnic group.
We conducted growth mixture modelling separately within the EA, AA, and LX subgroups, increasing the number of classes iteratively. Initial models had better fit without the quadratic term in all subgroups, so we excluded the quadratic slope from our iterative model testing. Model fit indices can be found in Table 2. For the EA subgroup, a four-class model was optimal based on lower AIC, BIC, and aBIC values compared to the 3-class model and significant VLMR and BLRT values compared to the 5-class model. Average levels of depressive symptoms can be found in Figure 1. We classified the four classes as high-decreasing (n = 138; 2.3%), increasing (n = 201; 3.3%), moderate (n = 633; 10.5%), and low (n = 5,071; 83.9%).
For the AA subgroup, a three-class solution was optimal based on lower AIC, BIC, and aBIC values compared to the 2-class solution and significant VLMR and BLRT values compared to the 4-class solution. Average levels of depressive symptoms can be found in Figure 2. We classified the three classes as high-decreasing (n = 40; 2.4%), moderate (n = 183; 11.2%), and low (n = 1,417; 86.4%).
For the LX subgroup, a two-class solution was optimal based on lower AIC, BIC, and aBIC values compared to the 1-class solution and significant VLMR and BLRT values compared to the 3-class solution. Average levels of depressive symptoms can be found in Figure 3. We classified the two classes as high-decreasing (n = 140; 6.1%) and low (n = 2143; 93.9%).
Associations between Grim-PGS, adverse life events, and parental acceptance and depressive symptom trajectories can be found in Tables 3–5. Within the EA subgroup (see Table 3), adverse life events were associated with greater likelihood of membership in the high-decreasing, increasing, and moderate depression trajectories compared to the low depression trajectory. The Grim-PGS by adverse life events interaction was associated with greater likelihood of being in the moderate vs. low trajectory. Follow up analyses indicated that the association between Grim-PRS and membership in the moderate vs. low trajectory was stronger among youth who reported experiencing greater adverse life events (+.5SD; OR = 1.35, 95% CI [1.05, 1.74]) than among youth who experienced fewer adverse life events (-.5SD, OR = .90, 95% CI [.48, 1.71]). Parental acceptance was associated with lower likelihood of following the high, increasing, and moderate trajectories compared to the low trajectory. There were no significant interaction effects between Grim-PGS and parental acceptance in predicting trajectories of depressive symptoms.
Table 3. Associations between Grim-PGS, adverse life events, parental acceptance, and depressive symptom trajectories among European American youth.
Table 4. Associations between Grim-PGS, adverse life events, and depressive symptom trajectories among African American youth.
Within the AA subgroup (see Table 4), there were no significant main effects of adverse life events or parental acceptance on depression trajectories. However, similar to the finding among EA youth, the Grim-PGS by adverse life event interaction was also associated with greater likelihood of being in the moderate vs. low trajectory. Specifically, the association between Grim-PRS and membership in the moderate vs. low trajectory was stronger among youth who reported experiencing more adverse life events (+.5SD; OR = 1.44, 95% CI [1.05, 1.90]) than among youth who experienced fewer adverse life events (-.5SD; OR = 1.38, 95% CI [.27, 7.17]).
Among LX youth (see Table 5), parental acceptance was associated with lower likelihood of membership in the high trajectory of depressive symptoms relative to the low trajectory. No main effect of adverse life events nor GXE effects were detected.
Table 5. Associations between Grim-PGS, adverse life events, and depressive symptom trajectories among Latinx youth.
The goal of the current study was to examine whether environmental risk (i.e., adverse life events) and resilience factors (i.e., parental acceptance), moderate the influence of a genetic predisposition for epigenetic aging on trajectories of depression across early adolescence. In both the EA and AA subgroups we found adverse life events, but not parental acceptance, moderated polygenic scores for GrimAge such that there was increased likelihood of membership in a higher vs. lower depression trajectory for those high on both polygenic predisposition and adverse life events. This novel finding highlights how early life adversity may have long-term impacts on youth mental health. This finding is in-line with a Diathesis Stress Theory perspective, that adverse life events sometimes exacerbate genetic predispositions leading to psychopathology later in life. Findings have important implications for basic science and future research endeavors.
Using a growth mixture modelling approach, we identified heterogeneity in depressive symptom trajectories within EA, AA, and LX subgroups from age 10/11 to 12/13. The trajectories we identified largely replicate those found in our previous study in ABCD that included four waves of data and focused on substance use intent and perceived harm (55). In the EA subgroup we identified high-decreasing, increasing, moderate, and low trajectories. Three of these trajectories were also identified in the AA subgroup; high-decreasing, moderate, and low. In the LX subgroup we also identified high-decreasing and low trajectories. This supports previous work finding heterogeneity in depressive symptom trajectories and illustrates that there are important differences across race/ethnicity, such as an increasing group present only in the EA subgroup (55–58). It should be noted that differences in trajectories across racial/ethnic groups may be due to sample size and statistical power. Future studies should examine depressive symptom trajectories in large samples of racially and ethnically diverse youth to further elucidate developmental differences in youth’s depression.
Contrary to our hypothesis, we did not find direct effects of polygenic scores for GrimAge on depressive symptom trajectories in any subgroup. However, in zero-order correlations for the EA subgroup we did find a positive association between Grim-PGS and depressive symptoms at age 10/11. The lack of direct Grim-PGS associations with depressive symptoms may result from examining multiple trajectories of depressive symptoms, contributing to a loss in power. Alternatively, there are several intervening biological mechanisms between genetic predisposition for epigenetic aging and depression which may attenuate any direct association. Finally, as evidenced by our findings, it may be that genetic predisposition for GrimAge is only associated with depression when it is combined with exposure to adverse life events.
In the EA subgroup we found direct effects of adverse life events contributing to greater likelihood of depressive symptom trajectory membership in all trajectories compared to the low trajectory, which aligns with previous literature (15). As hypothesized, we found that adverse life events moderated the effect of polygenic predisposition for GrimAge contributing to greater likelihood of membership in the moderate vs. low depressive symptom trajectories in the EA and AA subgroups. This aligns with the Diathesis Stress Theory and past research indicating that adverse life events moderate genetic predispositions in predicting depressive symptoms and depression (18, 20). Recent reviews suggest that adverse life events may be associated with depression via genetic predispositions and epigenetic profiles embedded in serotonin, HPA axis, and oxytocin systems (19, 21, 59). Evidence also indicates that GrimAge is associated with brain aging and health, which could serve as one mechanism underlying effects on depression (4, 60, 61). Our findings extend prior work by examining polygenic predisposition for GrimAge epigenetic aging and by demonstrating associations with depressive symptom trajectories across early adolescence.
Of note, we did not find interactive effects with other trajectories with elevated depressive symptoms (e.g., high-decreasing, increasing). These trajectories may have other etiologic underpinnings. Alternatively, it may be that these subgroups were too small so we had limited power to detect interactive effects. Limited power may also explain the lack of any interaction effects in the LX subgroup. The lack of an ethnically aligned polygenic score also likely contributed to a lack of findings in this subgroup.
We did not find support for our hypothesis that parental acceptance, under Resilience Theory, would buffer the negative influence of polygenic predisposition for GrimAge on membership in higher vs. lower depressive symptom trajectories. It may be that the Diathesis Stress Theory fits better with depression and depressive symptoms, and parental acceptance has buffering effects on genetic predisposition for other child outcomes. However, in the EA and LX groups we did find direct effects of parental acceptance on higher likelihood of membership in the low depressive trajectory compared to higher depressive trajectories. These effects align with past research finding greater parental warmth and acceptance associated with lower childhood and adolescent depression (22–25). The lack of an interactive effect with parental acceptance may be due to the relatively large direct effects. Also, there is heterogeneity in the severity, timing, and type of adverse life events that youth experience. Accumulating research findings suggest that there may be sensitive periods in development during which different types or more severe adverse experiences can affect brain development, systemic inflammation, and adolescent depression (62–64). Therefore, the timing, severity, and type of adverse experiences may also affect epigenetic aging (65, 66).
In light of many strengths, including the large longitudinal sample, the focus on genetic influences on epigenetic aging, and the Bayesian methods that allowed us to form racially aligned polygenic scores, there were also several key weaknesses. First, as no GWAS on epigenetic aging exists in Latinx samples we were unable to create ethnically aligned polygenic scores for the LX group. Future genetically informed research should aim to include more ethnically diverse samples to ensure the equitable benefit of research findings. Second, modelling multiple trajectories in each racial/ethnic subgroup allowed use to examine for specificity of effects on distinct depressive symptom trajectories. However, this likely led to a loss of power in comparisons involving the smaller trajectories. Finally, we were only able to model trajectories using three waves of data because of the assessment timing of adverse life events. Future research examining effects on trajectories across developmental periods will help to illuminate distal effects of key risk and resilience factors.
We extended the literature by creating polygenic scores for GrimAge representing epigenetic aging. We found evidence of moderation by adverse life events in predicting membership in moderate vs. low depressive symptom trajectories across early adolescence. These findings highlight the detrimental role of adverse life events in exacerbating genetic risk for GrimAge epigenetic aging. Future research should identify developmental mechanisms and environmental contexts that facilitate epigenetic aging as a risk factor for depression across the lifespan. For instance, research could examine pathways from adverse life experiences, genetic-epigenetic effects, biological stress pathways, and depression across different developmental periods and in diverse sociocultural samples (67). Greater specificity in the mechanisms underlying this pathway can help inform prevention and intervention efforts. In the current study, findings underscore the need for continued preventive interventions for at-risk youth and support systems for youth exposed to adverse life events. In-line with the current findings, interventions that target individual and social mechanisms in youth exposed to adverse life events may buffer genetic influences on future mental health issues under a Diathesis-Stress Framework (68).
Publicly available datasets were analyzed in this study. This data can be found here: National Institute for Mental Health Data Archive: https://nda.nih.gov/.
KE: Conceptualization, Data curation, Formal analysis, Methodology, Writing – original draft, Writing – review & editing. JS: Conceptualization, Funding acquisition, Methodology, Writing – original draft, Writing – review & editing. WQ: Data curation, Methodology, Project administration, Writing – original draft, Writing – review & editing. KL-C: Conceptualization, Funding acquisition, Investigation, Writing – original draft, Writing – review & editing.
The author(s) declare financial support was received for the research, authorship, and/or publication of this article. This work was supported by the National Institute on Alcohol Abuse and Alcoholism (R01AA031281; JS), National Institute on Drug Abuse (R01DA053740, KL-C) and National Institute of Child Health and Human Development (R01HD086085, KL-C).
Data used in the preparation of this article were obtained from the Adolescent Brain Cognitive DevelopmentSM (ABCD) Study (https://abcdstudy.org), held in the NIMH Data Archive (NDA). This is a multisite, longitudinal study designed to recruit more than 10,000 children age 9-10 and follow them over 10 years into early adulthood. The ABCD Study® is supported by the National Institutes of Health and additional federal partners under award numbers U01DA041048, U01DA050989, U01DA051016, U01DA041022, U01DA051018, U01DA051037, U01DA050987, U01DA041174, U01DA041106, U01DA041117, U01DA041028, U01DA041134, U01DA050988, U01DA051039, U01DA041156, U01DA041025, U01DA041120, U01DA051038, U01DA041148, U01DA041093, U01DA041089, U24DA041123, U24DA041147. A full list of supporters is available at https://abcdstudy.org/federal-partners.html. A listing of participating sites and a complete listing of the study investigators can be found at https://abcdstudy.org/consortium_members/. ABCD consortium investigators designed and implemented the study and/or provided data but did not necessarily participate in the analysis or writing of this report. This manuscript reflects the views of the authors and may not reflect the opinions or views of the NIH or ABCD consortium investigators.
The authors declare that the research was conducted in the absence of any commercial or financial relationships that could be construed as a potential conflict of interest.
The author(s) declared that they were an editorial board member of Frontiers, at the time of submission. This had no impact on the peer review process and the final decision.
All claims expressed in this article are solely those of the authors and do not necessarily represent those of their affiliated organizations, or those of the publisher, the editors and the reviewers. Any product that may be evaluated in this article, or claim that may be made by its manufacturer, is not guaranteed or endorsed by the publisher.
1. Duan R, Fu Q, Sun Y, Li Q. Epigenetic clock: A promising biomarker and practical tool in aging. Ageing Res Rev. (2022) 81:101743. doi: 10.1016/j.arr.2022.101743
2. Biemans Y, Bach D, Behrouzi P, Horvath S, Kramer CS, Liu S, et al. Identifying the relation between food groups and biological ageing: A data-driven approach. Age Ageing. (2024) 53:ii20–ii9. doi: 10.1093/ageing/afae038
3. Han LK, Aghajani M, Clark SL, Chan RF, Hattab MW, Shabalin AA, et al. Epigenetic aging in major depressive disorder. Am J Psychiatry. (2018) 175:774–82. doi: 10.1176/appi.ajp.2018.17060595
4. Protsenko E, Yang R, Nier B, Reus V, Hammamieh R, Rampersaud R, et al. Grimage,” an epigenetic predictor of mortality, is accelerated in major depressive disorder. Trans Psychiatry. (2021) 11:193. doi: 10.1038/s41398-021-01302-0
5. Gibson J, Russ TC, Clarke T-K, Howard DM, Hillary RF, Evans KL, et al. A meta-analysis of genome-wide association studies of epigenetic age acceleration. PloS Genet. (2019) 15:e1008104. doi: 10.1371/journal.pgen.1008104
6. McCartney DL, Min JL, Richmond RC, Lu AT, Sobczyk MK, Davies G, et al. Genome-wide association studies identify 137 genetic loci for DNA methylation biomarkers of aging. Genome Biol. (2021) 22:1–25. doi: 10.1186/s13059-021-02398-9
7. Brown RL, Alegria KE, Hamlat E, Tomiyama AJ, Laraia B, Crimmins EM, et al. Psychosocial disadvantage during childhood and midlife health: NIMHD social epigenomics program. JAMA network Open. (2024) 7:e2421841–e. doi: 10.1001/jamanetworkopen.2024.21841
8. Allegrini AG, Baldwin JR, Barkhuizen W, Pingault JB. Research review: A guide to computing and implementing polygenic scores in developmental research. J Child Psychol Psychiatry. (2022) 63:1111–24. doi: 10.1111/jcpp.v63.10
9. Masten AS, Kalstabakken AW. Developmental perspectives on psychopathology in children and adolescents. In: Butcher JN, Kendall PC, editors. Apa Handbook of Psychopathology: Child and Adolescent Psychopathology. Washington, DC: American Psychological Association (2018).
10. Cicchetti D. Development and psychopathology. In: Developmental Psychopathology: Theory and Method, 2nd Ed, vol. 1. John Wiley & Sons, Inc, Hoboken, NJ, US (2006). p. 1–23.
11. Colodro-Conde L, Couvy-Duchesne B, Zhu G, Coventry WL, Byrne EM, Gordon S, et al. A direct test of the diathesis–stress model for depression. Mol Psychiatry. (2018) 23:1590–6. doi: 10.1038/mp.2017.130
12. Arnau-Soler A, Adams MJ, Clarke T-K, MacIntyre DJ, Milburn K, Navrady L, et al. A validation of the diathesis-stress model for depression in generation Scotland. Trans Psychiatry. (2019) 9:25. doi: 10.1038/s41398-018-0356-7
13. Davis MC, Luecken L, Lemery-Chalfant K. Resilience in common life: introduction to the special issue. J Pers. (2009) 77:1637–44. doi: 10.1111/j.1467-6494.2009.00595.x
14. Elam KK, Lemery-Chalfant K, Chassin L. A gene-environment cascade theoretical framework of developmental psychopathology. J Psychopathol Clin Sci. (2023) 132:287. doi: 10.1017/S1474746419000071
15. LeMoult J, Humphreys KL, Tracy A, Hoffmeister J-A, Ip E, Gotlib IH. Meta-analysis: exposure to early life stress and risk for depression in childhood and adolescence. J Am Acad Child Adolesc Psychiatry. (2020) 59:842–55. doi: 10.1016/j.jaac.2019.10.011
16. Askeland KG, Bøe T, Breivik K, La Greca AM, Sivertsen B, Hysing M. Life events and adolescent depressive symptoms: protective factors associated with resilience. PloS One. (2020) 15:e0234109. doi: 10.1371/journal.pone.0234109
17. Elmore AL, Crouch E. The association of adverse childhood experiences with anxiety and depression for children and youth, 8 to 17 years of age. Acad Pediatr. (2020) 20:600–8. doi: 10.1016/j.acap.2020.02.012
18. Martins J, Yusupov N, Binder EB, Brückl TM, Czamara D. Early adversity as the prototype gene× Environment interaction in mental disorders? Pharmacol Biochem Behav. (2022) 215:173371. doi: 10.1016/j.pbb.2022.173371
19. Singh MK, Gorelik AJ, Stave C, Gotlib IH. Genetics, epigenetics, and neurobiology of childhood-onset depression: an umbrella review. Mol Psychiatry. (2024) 29:553–65. doi: 10.1038/s41380-023-02347-x
20. Czamara D, Tissink E, Tuhkanen J, Martins J, Awaloff Y, Drake AJ, et al. Combined effects of genotype and childhood adversity shape variability of DNA methylation across age. Trans Psychiatry. (2021) 11:88. doi: 10.1038/s41398-020-01147-z
21. Silva RC, Maffioletti E, Gennarelli M, Baune BT, Minelli A. Biological correlates of early life stressful events in major depressive disorder. Psychoneuroendocrinology. (2021) 125:105103. doi: 10.1016/j.psyneuen.2020.105103
22. McLeod BD, Weisz JR, Wood JJ. Examining the association between parenting and childhood depression: A meta-analysis. Clin Psychol Rev. (2007) 27:986–1003. doi: 10.1016/j.cpr.2007.03.001
23. Yap MBH, Pilkington PD, Ryan SM, Jorm AF. Parental factors associated with depression and anxiety in young people: A systematic review and meta-analysis. J Affect Disord. (2014) 156:8–23. doi: 10.1016/j.jad.2013.11.007
24. Pinquart M. Associations of parenting dimensions and styles with externalizing problems of children and adolescents: an updated meta-analysis. Dev Psychol. (2017) 53:873. doi: 10.1037/dev0000295
25. Manuele S, Yap M, Lin S, Pozzi E, Whittle S. Associations between paternal versus maternal parenting behaviors and child and adolescent internalizing problems: A systematic review and meta-analysis. Clin Psychol Rev. (2023) 105:102339. doi: 10.1016/j.cpr.2023.102339
26. Stocker CM, Masarik AS, Widaman KF, Reeb BT, Boardman JD, Smolen A, et al. Parenting and adolescents’ Psychological adjustment: longitudinal moderation by adolescents’ Genetic sensitivity. Dev Psychopathol. (2017) 29:1289–304. doi: 10.1017/S0954579416001310
27. Vazquez AY, Shewark EA, Hyde LW, Klump KL, Burt SA. Parental nurturance moderates the etiology of youth resilience. Behav Genet. (2024) 54:137–49. doi: 10.1007/s10519-023-10150-1
28. Assary E, Vincent JP, Keers R, Pluess M. Gene-environment interaction and psychiatric disorders: review and future directions. Semin Cell Dev Biol. (2018) 77:133–43. Elsevier. doi: 10.1016/j.semcdb.2017.10.016
29. van der Gaag MA. A person-centered approach in developmental science: why this is the future and how to get there. Infant Child Dev. (2023) 32:e2478. doi: 10.1002/icd.v32.6
30. Muthén B, Muthén LK. Integrating person-centered and variable-centered analyses: growth mixture modeling with latent trajectory classes. Alcoholism: Clin Exp Res. (2000) 24:882–91. doi: 10.1111/j.1530-0277.2000.tb02070.x
31. Martin AR, Gignoux CR, Walters RK, Wojcik GL, Neale BM, Gravel S, et al. Human demographic history impacts genetic risk prediction across diverse populations. Am J Hum Genet. (2017) 100:635–49. doi: 10.1016/j.ajhg.2017.03.004
32. Garavan H, Bartsch H, Conway K, Decastro A, Goldstein R, Heeringa S, et al. Recruiting the ABCD sample: design considerations and procedures. Dev Cogn Neurosci. (2018) 32:16–22. doi: 10.1016/j.dcn.2018.04.004
33. Uban KA, Horton MK, Jacobus J, Heyser C, Thompson WK, Tapert SF, et al. Biospecimens and the ABCD study: rationale, methods of collection, measurement and early data. Dev Cogn Neurosci. (2018) 32:97–106. doi: 10.1016/j.dcn.2018.03.005
34. Baurley JW, Edlund CK, Pardamean CI, Conti DV, Bergen AW. Smokescreen: A targeted genotyping array for addiction research. BMC Genomics. (2016) 17:1–12. doi: 10.1186/s12864-016-2495-7
35. Ge T, Chen C-Y, Ni Y, Feng Y-CA, Smoller JW. Polygenic prediction via Bayesian regression and continuous shrinkage priors. Nat Commun. (2019) 10:1776. doi: 10.1038/s41467-019-09718-5
36. Ruan Y, Lin Y-F, Feng Y-CA, Chen C-Y, Lam M, Guo Z, et al. Improving polygenic prediction in ancestrally diverse populations. Nat Genet. (2022) 54:573–80. doi: 10.1038/s41588-022-01054-7
37. Chang CC, Chow CC, Tellier LC, Vattikuti S, Purcell SM, Lee JJ. Second-generation plink: rising to the challenge of larger and richer datasets. Gigascience. (2015) 4:s13742–015-0047-8. doi: 10.1186/s13742-015-0047-8
38. Kachuri L, Chatterjee N, Hirbo J, Schaid DJ, Martin I, Kullo IJ, et al. Principles and methods for transferring polygenic risk scores across global populations. Nat Rev Genet. (2024) 25:8–25. doi: 10.1038/s41576-023-00637-2
39. Brieant AE, Sisk LM, Gee DG. Associations among negative life events, changes in cortico-limbic connectivity, and psychopathology in the ABCD study. Dev Cogn Neurosci. (2021) 52:101022. doi: 10.1016/j.dcn.2021.101022
40. Wethington E. Life events scale. Stress: Concepts, cognition, emotion and behavior. (2016) Academic Press, 103–8. doi: 10.1016/B978-0-12-800951-2.00012-1
41. Grant KE, Compas BE, Thurm AE, McMahon SD, Gipson PY. Stressors and child and adolescent psychopathology: measurement issues and prospective effects. J Clin Child Adolesc Psychol. (2004) 33:412–25. doi: 10.1207/s15374424jccp3302_23
42. Hoffman EA, Clark DB, Orendain N, Hudziak J, Squeglia LM, Dowling GJ. Stress exposures, neurodevelopment and health measures in the abcd study. Neurobiol Stress. (2019) 10:100157. doi: 10.1016/j.ynstr.2019.100157
43. Tiet QQ, Bird HR, Davies M, Hoven C, Cohen P, Jensen PS, et al. Adverse life events and resilience. J Am Acad Child Adolesc Psychiatry. (1998) 37:1191–200. doi: 10.1097/00004583-199811000-00020
44. Zhao C, Li X. Living under the shadow: adverse childhood experiences and entrepreneurial behaviors in Chinese adults. J Business Res. (2022) 138:239–55. doi: 10.1016/j.jbusres.2021.09.016
45. Hebous S, Ruf M. Evaluating the effects of ace systems on multinational debt financing and investment. J Public Economics. (2017) 156:131–49. doi: 10.1016/j.jpubeco.2017.02.011
46. Schaefer ES. Children's reports of parental behavior: an inventory. Child Dev. (1965) 36:413–24. doi: 10.2307/1126465
47. Schludermann E, Schludermann S. Children’s Report on Parent Behavior (Crpbi-108, Crpbi-30) for Older Children and Adolescents. Winnipeg, MB, Canada: University of Manitoba (1988).
48. Achenbach TM, Dumenci L, Rescorla LA. Ratings of Relations between Dsm-Iv Diagnostic Categories and Items of the CBCL/6-18, TRF, and YSR. Burlington, VT: University of Vermont (2001) p. 1–9.
49. Shen B, Mode NA, Hooten NN, Pacheco NL, Ezike N, Zonderman AB, et al. Association of race and poverty status with DNA methylation–based age. JAMA Network Open. (2023) 6:e236340–e. doi: 10.1001/jamanetworkopen.2023.6340
50. Goering M, Tiwari HK, Patki A, Espinoza CN, Knight DC, Mrug S. Examining health behaviors as mechanisms linking earlier pubertal timing with accelerated epigenetic aging in late adolescence. J Youth Adolescence. (2024), 1–19. doi: 10.1007/s10964-024-02096-2
51. Thapar A, Collishaw S, Pine DS, Thapar AK. Depression in adolescence. Lancet. (2012) 379:1056–67. doi: 10.1016/S0140-6736(11)60871-4
52. Keller MC. Gene× Environment interaction studies have not properly controlled for potential confounders: the problem and the (Simple) solution. Biol Psychiatry. (2014) 75:18–24. doi: 10.1016/j.biopsych.2013.09.006
53. Jung T, Wickrama KA. An introduction to latent class growth analysis and growth mixture modeling. Soc Pers Psychol compass. (2008) 2:302–17. doi: 10.1111/j.1751-9004.2007.00054.x
54. Asparouhov T, Muthén B. Auxiliary variables in mixture modeling: three-step approaches using M plus. Struct equation modeling: A Multidiscip J. (2014) 21:329–41. doi: 10.1080/10705511.2014.915181
55. Elam KK, Su J, Kutzner J, Trevino A. Individual trajectories of depressive symptoms within racially-ethnically diverse youth: associations with polygenic risk for depression and substance use intent and perceived harm. Behav Genet. (2024) 54:86–100. doi: 10.1007/s10519-023-10167-6
56. Musliner KL, Munk-Olsen T, Eaton WW, Zandi PP. Heterogeneity in long-term trajectories of depressive symptoms: patterns, predictors and outcomes. J Affect Disord. (2016) 192:199–211. doi: 10.1016/j.jad.2015.12.030
57. Shore L, Toumbourou JW, Lewis AJ, Kremer P. Longitudinal trajectories of child and adolescent depressive symptoms and their predictors–a systematic review and meta-analysis. Child Adolesc Ment Health. (2018) 23:107–20. doi: 10.1111/camh.2018.23.issue-2
58. Xiang Q, Chen K, Peng L, Luo J, Jiang J, Chen Y, et al. Prediction of the trajectories of depressive symptoms among children in the adolescent brain cognitive development (ABCD) study using machine learning approach. J Affect Disord. (2022) 310:162–71. doi: 10.1016/j.jad.2022.05.020
59. Lipsky RK, McDonald CC, Souders MC, Carpio CC, Teitelman AM. Adverse childhood experiences, the serotonergic system, and depressive and anxiety disorders in adulthood: A systematic literature review. Neurosci Biobehav Rev. (2022) 134:104495. doi: 10.1016/j.neubiorev.2021.12.018
60. Peterson JA, Strath LJ, Nodarse CL, Rani A, Huo Z, Meng L, et al. Epigenetic aging mediates the association between pain impact and brain aging in middle to older age individuals with knee pain. Epigenetics. (2022) 17:2178–87. doi: 10.1080/15592294.2022.2111752
61. Hillary RF, Stevenson AJ, Cox SR, McCartney DL, Harris SE, Seeboth A, et al. An epigenetic predictor of death captures multi-modal measures of brain health. Mol Psychiatry. (2021) 26:3806–16. doi: 10.1038/s41380-019-0616-9
62. Iob E, Lacey R, Giunchiglia V, Steptoe A. Adverse childhood experiences and severity levels of inflammation and depression from childhood to young adulthood: A longitudinal cohort study. Mol Psychiatry. (2022) 27:2255–63. doi: 10.1038/s41380-022-01478-x
63. Farooq B, Russell AE, Howe LD, Herbert A, Smith AD, Fisher HL, et al. The relationship between type, timing and duration of exposure to adverse childhood experiences and adolescent self-harm and depression: findings from three UK prospective population-based cohorts. J Child Psychol Psychiatry. (2024) 65:1369–87. doi: 10.1111/jcpp.v65.10
64. Sicorello M, Thome J, Herzog J, Schmahl C. Differential effects of early adversity and posttraumatic stress disorder on amygdala reactivity: the role of developmental timing. Biol Psychiatry: Cogn Neurosci Neuroimaging. (2021) 6:1044–51. doi: 10.1016/j.bpsc.2020.10.009
65. Strath LJ, Peterson JA, Meng L, Rani A, Huo Z, Foster TC, et al. Socioeconomic status, knee pain, and epigenetic aging in community-dwelling middle-to-older age adults. J Pain. (2024) 25:293–301. doi: 10.1016/j.jpain.2023.06.002
66. Jackson P, Spector AL, Strath LJ, Antoine LH, Li P, Goodin BR, et al. Epigenetic age acceleration mediates the relationship between neighborhood deprivation and pain severity in adults with or at risk for knee osteoarthritis pain. Soc Sci Med. (2023) 331:116088. doi: 10.1016/j.socscimed.2023.116088
67. Iob E, Lacey R, Steptoe A. Adverse childhood experiences and depressive symptoms in later life: longitudinal mediation effects of inflammation. Brain Behavior Immun. (2020) 90:97–107. doi: 10.1016/j.bbi.2020.07.045
Keywords: polygenic, epigenetics, depression, adverse life events, parent acceptance, early adolescence
Citation: Elam KK, Su J, Qin WA and Lemery-Chalfant K (2024) Polygenic risk for epigenetic aging and adverse life experiences interact to predict growth in adolescent depression in a racially/ethnically diverse sample. Front. Psychiatry 15:1499395. doi: 10.3389/fpsyt.2024.1499395
Received: 20 September 2024; Accepted: 21 November 2024;
Published: 20 December 2024.
Edited by:
Rodrigo G. Arzate-Mejía, Medical Faculty of the University of Zurich, SwitzerlandReviewed by:
Pippa Ann Thomson, Medical Research Council Institute of Genetics and Molecular Medicine (MRC), United KingdomCopyright © 2024 Elam, Su, Qin and Lemery-Chalfant. This is an open-access article distributed under the terms of the Creative Commons Attribution License (CC BY). The use, distribution or reproduction in other forums is permitted, provided the original author(s) and the copyright owner(s) are credited and that the original publication in this journal is cited, in accordance with accepted academic practice. No use, distribution or reproduction is permitted which does not comply with these terms.
*Correspondence: Kit K. Elam, a2l0ZWxhbUBpdS5lZHU=
Disclaimer: All claims expressed in this article are solely those of the authors and do not necessarily represent those of their affiliated organizations, or those of the publisher, the editors and the reviewers. Any product that may be evaluated in this article or claim that may be made by its manufacturer is not guaranteed or endorsed by the publisher.
Research integrity at Frontiers
Learn more about the work of our research integrity team to safeguard the quality of each article we publish.