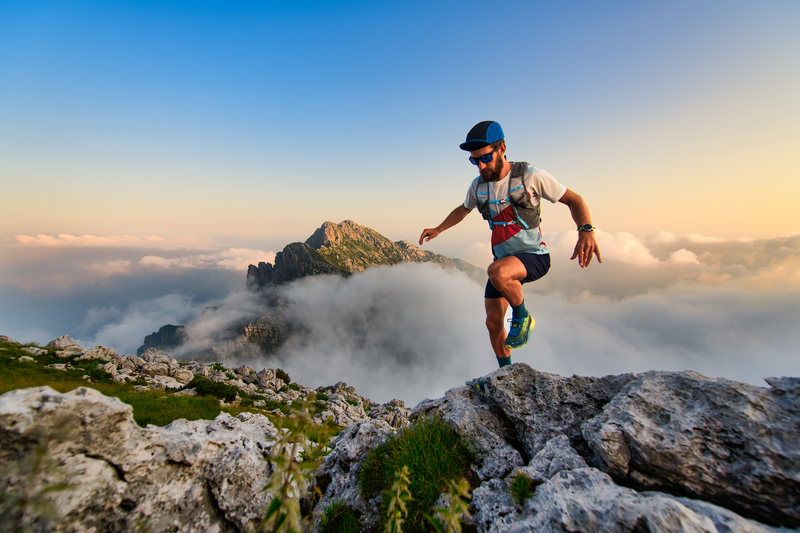
95% of researchers rate our articles as excellent or good
Learn more about the work of our research integrity team to safeguard the quality of each article we publish.
Find out more
STUDY PROTOCOL article
Front. Psychiatry , 07 January 2025
Sec. Schizophrenia
Volume 15 - 2024 | https://doi.org/10.3389/fpsyt.2024.1497565
This article is part of the Research Topic Underlying Neurobiological, Genetic, and Behavioral Mechanisms in Schizophrenia and Autism Spectrum Disorder View all 10 articles
The application of personalized medicine in patients with first-episode psychosis (FEP) requires tools for classifying patients according to their response to treatment, considering both treatment efficacy and toxicity. However, several limitations have hindered its translation into clinical practice. Here, we describe the rationale, aims and methodology of Applied Pharmacogenetics to Predict Response to Treatment of First Psychotic Episode (the FarmaPRED-PEP project), which aims to develop and validate predictive algorithms to classify FEP patients according to their response to antipsychotics, thereby allowing the most appropriate treatment strategy to be selected. These predictors will integrate, through machine learning techniques, pharmacogenetic (measured as polygenic risk scores) and epigenetic data together with clinical, sociodemographic, environmental, and neuroanatomical data. To do this, the FarmaPRED-PEP project will use data from two already recruited cohorts: the PEPS cohort from the “Genotype-Phenotype Interaction and Environment. Application to a Predictive Model in First Psychotic Episodes” study (the PEPs study from the Spanish abbreviation) (N=335) and the PAFIP cohort from “Clinical Program on Early Phases of Psychosis” (PAFIP from the Spanish abbreviation) (N = 350). These cohorts will be used to create the predictor, which will then be validated in a new cohort, the FarmaPRED cohort (N = 300). The FarmaPRED-PEP project has been designed to overcome several of the limitations identified in pharmacogenetic studies in psychiatry: (1) the sample size; (2) the phenotype heterogeneity and its definition; (3) the complexity of the phenotype and (4) the gender perspective. The global reach of the FarmaPRED-PEP project is to facilitate the effective deployment of precision medicine in national health systems.
Schizophrenia (SZ) is the most paradigmatic psychotic disorder. The course of the illness is often chronic and highly variable, causing a significant loss of quality of life for the patient and their family members (1). It also has a high cost for society, accounting for 10% of the global burden of mental disorders in Europe (2). There is great variability in the efficacy of antipsychotic drugs (APs) in the treatment of SZ as well as in the susceptibility of patients to the side effects of these drugs. On average, between 20% and 30% of patients do not respond appropriately to AP treatment and less than 40% achieve symptom remission (3), with a 70% treatment discontinuation rate. This leads to relapses that entail new admissions, thereby worsening the prognosis, negatively affecting the patient’s quality of life, and reducing life expectancy (4). Furthermore, approximately 30% of patients develop treatment-resistant SZ (TRS). It is essential to elucidate the underlying pathophysiology of TRS to identify biomarkers for its early detection and treatment. As an example, plasma levels of metabolites involved in the Kynurenine pathway, at the crossroad between neuroinflammation and glutamatergic neurotransmission, has been proposed as biomarker of TRS (5). However, the rates of long-term recovery for first-episode psychosis (FEP) are more favorable since about 51% of patients may achieve either symptomatic, functional, and personal recovery (6). Selecting the best compound for each patient is a challenging procedure (7), and its selection, unfortunately, largely relies on clinical experience and a trial-and-error strategy that exposes the patients to a higher risk of adverse reactions, prolongs the recovery time, and worsens the long-term response (8).
In this context, the search for biomarkers to select the most suitable AP for each patient in the early stages is a priority area in psychiatry (9). Pharmacogenetics (PGx) has become one of the main tools in this search for biomarkers (3). However, PGx results are characterized by a lack of replicability, thus limiting their translation into clinical practice. These studies have relied on the knowledge of pharmacokinetic and/or pharmacodynamic processes. The pharmacokinetic studies have shown the most promising results for clinical implementation as the genetic variability in metabolism-related genes explains a significant percentage of the variability in the plasma levels of some APs. Genotyping these genes can be very useful in improving APs efficacy and tolerability (10). In this regard, international guideline groups, such as the Dutch Pharmacogenetics Working Group (DPWG), have published pharmacogenetic guidelines for gene-drug interactions involving CYP2D6, CYP3A4, and CYP1A2 with antipsychotics (11), while the Clinical Pharmacogenetics Implementation Consortium (CPIC) is developing similar guidelines (https://cpicpgx.org/prioritization-of-cpic-guidelines/). Additionally, regulatory agencies like the Food and Drug Administration (FDA) have identified 41 pharmacogenomic biomarkers relevant to psychiatry in drug labeling (https://www.fda.gov/drugs/science-and-research-drugs/table-pharmacogenomic-biomarkers-drug-labeling). By contrast, the pharmacodynamic studies have been rarely replicated in independent populations, often explaining a small percentage of the observed variability that limits their predictive capacity (12).
The candidate gene approach is further limited by the lack of understanding of the mechanism of action of the APs. The pharmacological response to APs can be defined as a complex phenotype with polygenic inheritance involving multiple loci with small effects. Polygenic risk scores (PRS) are constructed by summing the multiple risk alleles associated with a phenotype and are weighted by the magnitude of their estimated effect in genome-wide association studies (GWAS) using large cohorts with sufficient statistical power (13). In psychiatry, these PRS have been calculated to estimate the genetic risk for various conditions, such as SZ, major depression, and bipolar disorder (14). Several studies have demonstrated that the PRS calculated for psychopathologies can be useful in pharmacogenomic studies (14–17) as predictors of the response to APs (18–22). Additionally, the PRS calculated for phenotypes related to the pharmacological response to APs, such as cognitive performance or metabolic alterations, can also be associated with the efficacy or toxicity of AP treatments (14, 23–25). The clinical application of PRS and their inclusion in healthcare systems are some of the most promising aspects in implementing PGx in clinical practice (13). However, PRS have limited precision in their predictive capacity, as there are many other sociodemographic, environmental, clinical, and pharmacological factors involved in this complex phenotype. Despite these limitations, there is evidence for the clinical applicability of PRS, mainly for patient identification and stratification (13, 17). In these examples, PRS have been incorporated into risk algorithms and are already used in clinical practice in some cases, increasing their predictive capacity. The integration of PRS into algorithms that include other risk factors (i.e., sociodemographic, environmental, clinical, and pharmacological) represents the future of personalized medicine.
PGx studies have been hindered by limitations such as difficulties in recruiting large cohorts, as they require detailed information and longitudinal follow-up to assess the response to pharmacological treatment. Additionally, PGx studies in SZ are constrained by disease-specific limitations (14) such as diagnostic heterogeneity (multiple and variable symptoms accompanied by neuropsychological and functional impairments) (26), multiple comorbidities, and pharmacological heterogeneity (multiple APs with different properties, variable doses, AP combinations, and concomitant medications) (27, 28). Furthermore, there is heterogeneity in the definition of response phenotypes that is usually defined as a percentage of improvement in overall symptomatology or a threshold value measured in cross-sectional studies, without the consideration of the different symptoms and dimensions of psychiatric disorders, their longitudinal evolution, or years of treatment. Accordingly, several authors proposed adding functioning and personal recovery measures to the list of outcome indicators for FEP, expanding it beyond symptomatologic remission (29).
The “Applied Pharmacogenetics to Predict Response to Treatment of First Psychotic Episode” (FarmaPRED-PEP from the Spanish abbreviation) project is a multicenter study designed to allow the development and validation of a predictive algorithm for the application of personalized medicine in patients with first-episode psychosis (FEP). The purpose of this predictor will be to classify FEP patients according to their response phenotype to APs, thereby allowing the most appropriate treatment strategy to be selected. This predictor will integrate, through machine learning techniques, pharmacogenetic (measured as polygenic risk scores) and epigenetic data with clinical, sociodemographic, environmental, and neuroanatomical data. To do this, the FarmaPRED-PEP project will use the data from two already recruited cohorts of patients with FEP and a longitudinal follow-up: the PEPS cohort from “Genotype-Phenotype Interaction and Environment. Application to a Predictive Model in First Psychotic Episodes” (the PEPs study from the Spanish abbreviation; PI08/0208) (N = 335) (30) and the PAFIP cohort from “Clinical Program on Early Phases of Psychosis” (PAFIP from the Spanish abbreviation)” (N = 350) (31). These cohorts will allow response phenotypes to be defined using longitudinal data, taking into account not only the symptomatological dimensions of the pathology, but also the neurocognitive dimensions and adverse effects. These cohorts will be used to create as well as internally validate the predictor. This predictor will be externally validated in a new prospective cohort of patients with FEP and a longitudinal follow-up, the FarmaPRED cohort (N = 300).
FarmaPRED-PEP is an observational, naturalistic, and longitudinal study examining clinical trajectories and the predictors of clinical response to APs in FEP cohorts (Figure 1).
The specific aims of the FarmaPRED-PEP study are to:
1. Define treatment response phenotypes to antipsychotics in two cohorts of patients with first-episode psychosis (N = 700) using statistical techniques applied to longitudinal data on symptomatology, neurocognition, and adverse effects, before validating these phenotypes in a new prospective cohort (N = 300).
2. Reach consensus on clinical recommendations for the defined treatment response phenotypes.
3. Develop and perform internal, external, and prospective validation of the predictive algorithms for the defined response phenotypes using machine learning techniques that integrate pharmacogenetic variables (measured as PRS) and epigenetic data along with clinical, sociodemographic, environmental, and neuroanatomical data.
4. Develop predictive algorithms for antipsychotic responses specifically adapted for each gender.
5. Develop a computer application that contains the predictive algorithms for the treatment response phenotypes and the clinical recommendations for each.
6. Study the feasibility of the clinical applicability of the predictive algorithms in coordination with healthcare systems.
7. Promote educational programs on personalized and precision medicine in psychiatry.
8. Explore strategies to promote access to genomic and health data, and their potential risks and benefits in psychiatric patients.
To achieve these objectives, the FarmaPRED-PEP study has established a national research network that is focused on recruiting FEP patients from 12 national study sites (Figure 2), with eight work packages having already been designed (Table 1). The teams from these sites are members of the Biomedical Research Networking Center in Mental Health (CIBERSAM from the Spanish abbreviation), a Spanish network focusing on translational research on the neuroscientific aspects related to health and mental illness (www.cibersam.es) (32).
The FarmaPRED-PEP study will take advance of two already recruited Spanish cohorts of FEP patients with longitudinal assessment and is currently recruiting a third cohort. The two cohorts previously recruited are: the PEPS cohort (N = 335) (30) and the PAFIP cohort (N = 350) (31). A complete description of these cohorts can be found elsewhere (30, 33).
The FarmaPRED-PEP research network is recruiting the FarmaPRED cohort. The inclusion criteria are: aged between 16 and 35 years at the time of first evaluation; a duration of positive symptomatology that does not exceed 12 months; a duration of AP treatment that does not exceed 3 months; fluency in Spanish; and signed informed consent for the study (for minors, by a legal guardian if the patient agrees to participate). The exclusion criteria are: a neurological disorder; traumatic brain injury with a loss of consciousness; intellectual disability, not only an IQ < 70, but also poor functioning; somatic pathology with a mental impact; toxic psychosis; or a refusal to undergo genetic testing.
The study was approved by the research ethics committees of all the participating clinical centers (HCB/2022/0079). Informed consent is to be obtained from all the participants. Informed consent has been designed according to the recommendations of the Spanish Precision Medicine Infrastructure Associated with Science and Technology (IMPaCT) (https://impact.isciii.es/) and the Carlos III Health Institute (Spain) to ensure proper data sharing and to promote open science.
Clinical trajectories, derived from longitudinal data that characterize AP response phenotypes, will serve as the primary clinical endpoint. These trajectories will utilize data collected at baseline, 3, 6, and 12 months and will be identified via clustering and latent variable analyses. Response phenotypes will be based on longitudinal changes across multiple domains, including psychotic symptomatology (positive, negative, and general symptoms), affective symptoms, and side effects. All measures used to define these trajectories are detailed below.
The diagnosis of a psychotic disorder will be established using semi-structured interviews based on the age of the patient: the Schedule for Affective Disorders and Schizophrenia for School-Age Children - Present and Lifetime Version (K-SADS-PL) (< 18 years old) (34) and the Mini-International Neuropsychiatric Interview (MINI) (> 18 years old) (35). To retrospectively characterize and date the initial symptoms of a psychotic illness, the Symptom Onset in Schizophrenia (SOS) inventory will be used (36).
At baseline, a complete personal and family history will be taken, including a history of drug use. Environmental factors and stressors will also be recorded using several measures that include the Lewis-Murray Obstetric Complications Scale (37), the List of Threatening Experiences Questionnaire (38) and the Childhood Trauma Questionnaire (39), with information on urbanicity and the socioeconomic status also being collected using the Hollingshead-Redlich index. The Premorbid Adjustment Scale (PAS) (40) will be used to assess premorbid adjustment, one of the most studied factors in relation to the prognosis of psychotic disorders. In each evaluation, weight, height, the body mass index (BMI), blood pressure, and the abdominal perimeter will also be recorded, with the aim of monitoring the physical health indicators.
In each evaluation, information on the drugs prescribed will be recorded, including the type of drug, the duration of treatment, and dosage. Information on psychological treatment will also be collected. To assess adverse drug reactions, several measures will be included: the Udvalg für Kliniske Undersogelser (UKU) side effect rating scale (41), the Simpson-Angus scale, and general blood tests (total cholesterol, LDL, HDL, triglycerides, glucose, and prolactin). AP plasma levels will be measured at each visit. The analysis of plasma levels together with the complete genotyping of the cytochromes and transporters will allow the identification of genuine non-responders, differentiating them from non-adherers to treatment. Adherence will be measured using the Morisky Green Levine Medication Adherence Scale (42).
At baseline and in each evaluation, a comprehensive assessment of psychopathology will be performed that will include well-established measures such as the Positive and Negative Syndrome Scale (PANSS) (43), the Brief Negative Symptom Scale (BNSS) (44), the Young Mania Rating Scale (YMRS) (45), and the Montgomery—Asberg Depression Rating Scale (MADRS) (46). The assessment of global functioning will include the Clinical Global Impression (CGI) Scale (47), the Global Assessment of Functioning (GAF) Scale and Functional Assessment Staging (FAST) (48).
The neuropsychological assessment battery will be applied in the three-month evaluation to ensure that the patient is clinically stable. The neuropsychological assessment battery will be repeated in the one-year follow-up visit. The study will use the MATRICS Consensus Cognitive Battery (MCCB) (49) consisting of 10 individually administered tests that measure cognitive performance in seven domains: speed of processing (BACS: symbol coding; category fluency: animal naming; Trail Making Test - Part A), attention/vigilance (CPT-IP), working memory (WMS®-III: Spatial Span; Letter-Number Span), verbal learning (HVLT-R™), visual learning (BVMT-R™), reasoning and problem solving (NAB®: Mazes), and social cognition (MSCEIT™: Managing Emotions). The MCCB will be complemented by several tests to facilitate the harmonization of the cognitive data among the cohorts. These tests include: the Vocabulary and Matrix Reasoning subtests of the Wechsler Adult Intelligence Scale – Fourth Edition (WAIS-IV) (50) to measure current IQ; the Backward Digit Span subtest of the WAIS-IV (50) to measure working memory; and the Trail Making Test - Part B (51) to measure executive functions. Additionally, at baseline, the Cognitive Reserve Assessment Scale in Health (CRASH) (52) will be used to measure cognitive reserve.
The multimodal neuroimaging protocol is designed for a 3T MRI scanner and includes structural imaging and resting-state functional MRI (rfMRI). Images will be collected at baseline or in the three-month evaluation to ensure that the patient is clinically stable. There are currently 11 MRI scanning sites with a variety of vendors and platforms. Data will be collected from each center and processed at one site. Upon arrival at the data analysis center, quality control (QC) will check the consistency of the data and adherence to the specified imaging protocol. Following QC, the data will be mathematically harmonized to eliminate residual site effects and will then be processed using robust analysis streams.
In all evaluations, blood samples will be collected in whole-blood EDTA tubes. The blood samples will be processed using a standardized protocol to obtain aliquots of plasma (for plasma AP assessment) and buffy coat (for DNA extraction). Samples will be stored in -80°C freezers. The relevant metadata will include the fasting duration, the time since the last AP dosage, and recent illnesses or inflammatory conditions. DNA will be extracted from buffy coat samples following established standard protocols. Normalized DNA concentrations will be sent to the Spanish National Genotyping Center (CeGen). Whole-genome genotyping will be undertaken using the Axiom™ Spain Biobank Array (developed in the University of Santiago de Compostela, Spain) (Thermo Fisher Scientific) and data will be analyzed to obtain polygenic risk scores (PRS) for schizophrenia and other psychopathologies as well as other conditions including cognition, personality traits, and metabolic or inflammatory traits. GWAS summary results will be downloaded from the Psychiatric Genomics Consortium and the Science Genetic Association Consortium. Standard pipeline analysis (previously described (25)) will be performed, allowing efficient quality control, relatedness testing, principal component analysis, and genotype imputation. The selected PRSs will be computed using the PRS continuous shrinkage (PRS-CS) software, a Bayesian-based method that infers an updated posterior SNP effect size by applying continuous shrinkage to the discovery GWAS summary statistics. The external linkage disequilibrium (LD) reference panel will be constructed using a publicly available subsample of the UK Biobank. The derived PRSs will be standardized to z-scores with mean 0 and a standard deviation of 1. The genotyping of specific pharmacogenetic genes (including cytochromes and transporters) will be conducted with the PharmacoScan array (Thermo Fisher Scientific). Only genes with meaningful impacts on AP metabolism, as per CPIC levels A–B for gene-drug interactions (https://cpicpgx.org/genes-drugs/), will be included. Genetic variants will be annotated using star (*) alleles, where applicable, via the PharmVar database (https://www.pharmvar.org/). For statistical analysis, genotype-to-phenotype translations will follow consensus recommendations (e.g., CYP2D6) (53) or, if absent, CPIC-defined phenotypes. DNA methylation will be assessed using the Illumina Infinium MethylationEPIC v2.0 kit (Illumina) and analyzed to calculate different epigenetic clocks (54) and methylation profile scores (MPS) (55) according to standard protocols that include quality control, beta-value computation, normalization, and singular value decomposition to identify and remove unwanted sources of variation (56, 57). We will use publicly available data from methylation-wide association studies as a discovery sample to calculate methylation profile scores using similar approaches to those used for the construction of PRS. We developed a code for computing MPS accessible via the following public repository on GitHub: https://github.com/agonse/methylscore.
Phenotypic data will be collected and managed using LibreClinica, an open-source electronic data capture (EDC) system for clinical trials based on the OpenClinica(R) (OC) 3 community edition. A web-based EDC has been designed specifically for the collection of data from all the measures and questionnaires. Real-time data validation and quality rules will be defined to ensure that the data are entered accurately and as completely as possible. Clinical raters will directly enter the questionnaire data using the corresponding data entry forms. Each data entry will then be carefully double-checked by an external project management team that will resolve any discrepancy or query. Standard operating procedures will be established to ensure proper data collection and comparability across the study sites.
The analyses will focus on two main goals: (1) the characterization of AP response phenotypes using longitudinal data and (2) the prediction of these phenotypes. Clinical trajectories utilizing data from baseline, 3-, 6-, and 12-month follow-ups will identify AP response phenotypes based on the longitudinal change in different psychotic symptom domains (positive, negative, and general) as well as in affective symptoms and side effects. Trajectory analyses will include all the information available from all the time-points and will apply state-of-the-art clustering and latent variable analyses. The PEPS cohort (N = 300) will be used to identify these AP response phenotypes and to perform their clinical characterization. Predictions will be based on the data collected at baseline and the cognition information collected in the 3-month follow-up. To ensure our ability to test the robustness of the prediction models in an unbiased manner, the PEPS cohort will be used to create the prediction model, while the PAFIP cohort (N = 350) will be used as an unseen independent validation sample. Explainable machine learning methods will be used. The most robust model will then be selected and fine-tuned by merging the PEPS and PAFIP cohorts before being externally validated using the FarmaPRED cohort.
We used a recently-described method to determine sample size for developing a clinical prediction model using a traditional likelihood-based approach (e.g., logistic regression) (58). Sample sizes were derived for the scenarios of low, medium or high prediction performance (Nagelkerke R-squared value of 0.25/0.40/0.55), with the number of parameters in the model being 10. The minimum expected frequency of the AP response cluster was 15% according to previous studies with the PEPS cohort (59). Using the R package ‘pmsampsize’, we estimated the sample sizes required for binary outcomes. For a binary outcome, there are three criteria for determining sample size: (1) a small overfitting defined by an expected shrinkage of predictor effects by 10% or less; (2) a small absolute difference of 0.05 in the model’s apparent and adjusted Nagelkerke R-squared value; and (3) a precise estimation (within +/- 0.05) of the average outcome risk in the population for a key time-point of interest for prediction. Each criterion may require a different sample size and the chosen sample size is the largest of the three. For this study, when the outcome is considered binary, the criterion with the largest required sample size will be criterion 1 for a low predictive performance and 2 for medium and high performances. Assuming a phenotype frequency of 15% and a maximum of 10 parameters to be included in the predictive model, the minimum sample size for a model is estimated to be between 290 and 310.
The FarmaPRED-PEP project has been designed to overcome several of the limitations identified in PGx studies in psychiatry, including:
1. Sample size. As commented previously, the recruitment of large cohorts with longitudinal follow-ups and deep phenotyping is challenging and difficult to achieve. Here, the aim of the study is to collect data from 1000 patients through the integration of three cohorts of FEP patients: the PEPS cohort, the PAFIP cohort, and the FarmaPRED cohort. The assessment instruments and measurement time-points to be used in the FarmaPRED cohort were decided via a working group discussion to facilitate the harmonization of clinical and neurocognitive data among the cohorts.
2. Phenotype heterogeneity and definition. One factor hindering the identification of predictors for AP response is the inconsistency in how AP response phenotypes are defined across various studies (28, 59). Many researchers adopt binary classifications to measure effectiveness (categorizing subjects as either responders or non-responders) or evaluate toxicity (determining whether an adverse effect is present or absent), although there is no consensus regarding the ideal cut-off values for these variables. Such binary classifications fail to capture the complex nature of AP responses, which are influenced by both the effectiveness of the treatment (encompassing multiple aspects such as clinical symptoms, neurocognitive performance, and quality of life) and the side effects involved. Additional challenges in predicting AP responses include the diverse progression of the disease itself. Predicting response outcomes using patients with chronic conditions introduces more variability and limits the applicability of the findings due to differences in illness duration, diagnostic criteria, and previous treatments. Conversely, patients experiencing their first episode of schizophrenia generally show less variation in their prior use of antipsychotics, making them more appropriate subjects for studying predictors of treatment outcomes (60, 61). The aim of the FarmaPRED-PEP study is to define the phenotype of response to APs using longitudinal data from over the course of one year after the FEP and considering that this response is a complex phenotype encompassing not only the remission of the various symptoms that characterize the pathology, but also the neurocognitive aspects and the emergence of adverse effects.
3. The complexity of the phenotype. The AP response is a complex phenotype with a polygenic basis that could be partially captured using PRS. However, as is the case with other risk factors used in healthcare (e.g., cholesterol levels), PRS have a predictive capacity with limited accuracy, meaning they cannot predict a clinical variable of interest with sufficient precision at the individual level. Even if the PRS captured all the genetic variability in a pharmacological response attributable to common genetic variants, their predictive capacity would still be imperfect, mainly for two reasons. Firstly, genetic factors are not the only risk factors that explain variability in a pharmacological response as there are many other sociodemographic, environmental, clinical, and pharmacological factors involved in this complex phenotype (62, 63). Secondly, PRS only account for the contribution of common genetic variants, each with a small effect, without considering the effects of rare genetic variants, which are less frequent but have a larger impact, or the effects attributable to epigenetic modifications. Although the inclusion of rare genetic variants, identifiable through massive sequencing techniques, is not expected to increase the predictive capacity of the PRS in the short term (13), the inclusion of epigenetics in pharmacogenomic studies is expected to help explain much of the missing heritability, that is, the heritability of a phenotype that is not captured by genetic variants (3). In this regard, variables such as epigenetic clocks have recently been shown to be related not only to schizophrenia, but also to the response to APs (56, 64, 65).
4. The gender perspective. The study assumes a gender-based approach as a cross-cutting axis to improve health interventions. Therefore, its objective is to develop predictive algorithms for the response to AP, especially tailored to each gender. The differences between women and men in the incidence of FEP are well known, but less studied in relation to the response to AP. In this sense, the study will not be limited to considering gender as a simple categorical variable for introduction into statistical models, but aims to conduct an in-depth study of the impact that gender has on pharmacological response in FEP and its interaction with other health determinants (e.g., age, socioeconomic status, educational level, etc.). Therefore, this study will promote the search, detection, and analysis of differences, as well as similarities, between men and women, both in terms of efficacy and in terms of the frequency and type of adverse effects. Thus, it aims to determine whether the improvement in psychotic symptomatology, but also in neurocognitive characteristics, as well as the frequency and severity of adverse effects, are exclusive to one of the two sexes, more prevalent in one of the two sexes, with different characteristics between both sexes, or even if they receive different responses from the system depending on whether they are men or women. To avoid some of the gender biases and common malpractices in health research, the study data will be collected and analyzed disaggregated by gender and will be presented in reports and publications derived in the same way.
Overcoming these limitations, the aim of the FarmaPRED-PEP study is to develop algorithms to predict AP response phenotypes using genetic and epigenetic data (measured as risk scores) together with clinical, sociodemographic, environmental, and neuroanatomical data.
The future of medicine is focused on offering a comprehensive approach to health, taking into account all the determining factors of health or illness. The generation of large amounts of health data has exceeded the capacity to manage all available information in real time; therefore, artificial intelligence can become a support tool for healthcare professionals, who, however, must always have the final say in decision-making. Machine learning systems, and more specifically deep learning ones, are capable of extracting patterns and generating conclusions from a large amount of data using complex formulas and associations, making them less intuitive. This fact limits the understanding and comprehension of the systems by healthcare professionals, making it difficult to integrate them into workflows and generating a lack of confidence in the results they can provide. Our project will be focus in explainable artificial intelligence methods that derive informative predictions that can be mapped back to individual features and biomarkers, as opposed to some deep learning and other “black-box” techniques that may not be appropriate for achieving interpretability.
The application of precision medicine in psychiatry has been defined as a multi-stage process (66), where PRS play a significant role in each stage, such as in: (1) predicting the risk of developing the disorder to design preventive strategies; (2) stratifying patients based on their own characteristics, both clinical and genetic, to identify the most effective treatment, treatment-resistant patients, and susceptibility to developing adverse effects; and (3) optimizing dosage regimens to ensure treatment efficacy and tolerability based on drug kinetics and patient genetic characteristics. The FarmaPRED-PEP study focuses on the second and third stages of this strategy.
Although the FarmaPRED study has been designed to address several limitations commonly found in pharmacogenomics (PGx) studies, certain constraints should be considered when interpreting future findings. Firstly, as a naturalistic study, treatment heterogeneity and the non-random selection of specific treatments could act as potential confounders. Secondly, while allowing prior antipsychotic (AP) treatment of less than three months aims to facilitate sample recruitment and ensure adequate statistical power, it may also introduce variability that could confound clinical trajectories. Thirdly, although treatment adherence will be monitored, no interventions to enhance adherence are planned; as a result, adherence variability may further confound the response phenotypes based on clinical trajectories. Finally, despite the implementation of rigorous data harmonization protocols to ensure comparability across the three cohorts involved in the study, uncontrolled differences between cohorts may still influence the findings.
The application of these strategies in personalized and precision medicine is especially relevant in patients with FEP, as recovery after FEP has become the primary goal of any treatment strategy (61), with their progress during the first year of treatment considered crucial for disease prognosis and relapse prevention. Although SZ is a potentially disabling and serious mental illness, an appropriate multidisciplinary approach to FEP can contribute to complete recovery (67). A critical period of 2 to 5 years after FEP has been defined, during which therapeutic interventions may be more successful in preventing relapses and achieving functional recovery in patients (68). Preventing relapses in the early stages of the disease has become a major challenge due to the critical impact that a relapse can have on functional prognosis (69). Non-adherence to APs and a lack of disease knowledge are very common in patients with FEP and are associated with an increased risk of relapse, leading to a worse disease course and functionality (70–72).
Psychotic disorders encompass schizophrenia, schizophreniform disorder, schizoaffective disorder, brief psychotic disorder, and substance-induced psychotic disorder. Additionally, other mental health conditions, such as major depressive disorder, bipolar disorder, obsessive-compulsive disorder (OCD), posttraumatic stress disorder (PTSD), and autism spectrum disorder, may also present with short- to medium-term psychotic symptoms. Given the genetic pleiotropy shared among these conditions -captured through polygenic risk scores- and the widespread use of APs to treat them, the findings of the FarmaPRED-PEP study have the potential to offer transdiagnostic insights that extend beyond first-episode psychosis (FEP) patients.
Pharmacogenomic studies and personalized and precision medicine in the field of psychotic disorders will need to address the challenges that must be faced to ensure their future applicability in clinical practice. These challenges have been defined by the International Consortium for Personalized Medicine (ICPerMed) (https://www.icpermed.eu/index.php) and include, among others, the management of genomic and health data in terms of ensuring confidentiality as well as access to citizens and researchers, the involvement of health authorities in promoting personalized medicine to facilitate its implementation in healthcare systems, the education and involvement of healthcare professionals to ensure knowledge, access, and application of personalized and precision medicine strategies, the multidisciplinary integration of researchers and clinicians to develop personalized medicine strategies, and the collection of data and their use for a more efficient patient-centered healthcare system. These challenges are also the pillars of IMPaCT (https://impact.isciii.es/) of the Carlos III Health Institute (Spain), the founder of the FarmaPRED-PEP study. The aims of our study are aligned with the mission of IMPaCT to facilitate the effective deployment of precision medicine in the Spanish National Health System, ensuring scientific and technical quality, equity, and efficiency in the use of available scientific resources to meet the needs of the citizenry.
The studies involving humans were approved by Hospital Clinic Barcelona HCB/2022/0079. The studies were conducted in accordance with the local legislation and institutional requirements. Written informed consent for participation in this study was provided by the participants’ legal guardians/next of kin.
SM: Conceptualization, Funding acquisition, Investigation, Methodology, Project administration, Writing – original draft, Writing – review & editing. LJ: Methodology, Writing – original draft, Writing – review & editing. MC: Conceptualization, Writing – original draft, Writing – review & editing. BC-F: Conceptualization, Writing – original draft, Writing – review & editing. JV-B: Conceptualization, Writing – original draft, Writing – review & editing. CS: Conceptualization, Writing – original draft, Writing – review & editing. AG-P: Conceptualization, Writing – original draft, Writing – review & editing. AI: Conceptualization, Writing – original draft, Writing – review & editing. JU: Conceptualization, Writing – original draft, Writing – review & editing. CR-L-A: Conceptualization, Writing – original draft, Writing – review & editing. AC: Conceptualization, Writing – original draft, Writing – review & editing. AS: Conceptualization, Writing – original draft, Writing – review & editing. MB: Conceptualization, Writing – original draft, Writing – review & editing.
The author(s) declare financial support was received for the research, authorship, and/or publication of this article. This study (PMP21/00085) was funded by Instituto de Salud Carlos III (ISCIII) and funded by the European Union (NextGenerationUE- Recovery and Resilence Facility); Centro de Investigación Biomédica en Red de Salud Mental (CIBERSAM); Catalan Government, the Secretariat of Universities and Research of the Department of Enterprise and Knowledge (2021 SGR 00672). The “Programa Asistencial de las Fases Iniciales de Psicosis” (PAFIP) was carried out at the Hospital Marqués de Valdecilla, University of Cantabria, Santander, Spain, under the following grant supports: Instituto de Salud Carlos III (Instituto de Salud Carlos III PI020499, PI050427, PI060507, PI14/00639 and PI14/00918); Plan Nacional de Drogas Research (2005-Orden sco/3246/2004); SENY Fundació (CI 2005–0308007); and Fundación Marqués de Valdecilla (API07/011); ClinicalTrials.gov Identifier: NCT03090490, NCT02916303, NCT02205437, NCT0253249, NCT02858102, NCT02220504, NCT02534363, NCT02526030. The PEPS cohort has been funded by the Ministerio de Economía y Competitividad (PI08/0208; PI11/00325; PI14/00612; PI20/00661; PI20/00661), Instituto de Salud Carlos III – Fondo Europeo de Desarrollo Regional. Unión Europea. Una manera de hacer Europa, Centro de Investigación Biomédica en Red de salud Mental, CIBERSAM.
The authors thank the Language Advisory Service at the University of Barcelona for manuscript revision.
The authors declare that the research was conducted in the absence of any commercial or financial relationships that could be construed as a potential conflict of interest.
The author(s) declared that they were an editorial board member of Frontiers, at the time of submission. This had no impact on the peer review process and the final decision.
All claims expressed in this article are solely those of the authors and do not necessarily represent those of their affiliated organizations, or those of the publisher, the editors and the reviewers. Any product that may be evaluated in this article, or claim that may be made by its manufacturer, is not guaranteed or endorsed by the publisher.
1. Ayesa-Arriola R, Ortíz-García de la Foz V, Martínez-García O, Setién-Suero E, Ramírez ML, Suárez-Pinilla P, et al. Dissecting the functional outcomes of first episode schizophrenia spectrum disorders: A 10-year follow-up study in the PAFIP cohort. Psychol Med. (2021) 51:264–77. doi: 10.1017/S0033291719003179
2. Perälä J, Suvisaari J, Saarni SI, Kuoppasalmi K, Isometsä E, Pirkola S, et al. Lifetime prevalence of psychotic and bipolar I disorders in a general population. Arch Gen Psychiatry. (2007) 64:19–28. doi: 10.1001/ARCHPSYC.64.1.19
3. Lisoway AJ, Chen CC, Zai CC, Tiwari AK, Kennedy JL. Toward personalized medicine in schizophrenia: Genetics and epigenetics of antipsychotic treatment. Schizophr Res. (2021) 232:112–24. doi: 10.1016/J.SCHRES.2021.05.010
4. Kahn RS, Fleischhacker WW, Boter H, Davidson M, Vergouwe Y, Keet IP, et al. Effectiveness of antipsychotic drugs in first-episode schizophrenia and schizophreniform disorder: an open randomised clinical trial. Lancet. (2008) 371:1085–97. doi: 10.1016/S0140-6736(08)60486-9
5. Sapienza J, Agostoni G, Dall’Acqua S, Sut S, Nasini S, Martini F, et al. The kynurenine pathway in treatment-resistant schizophrenia at the crossroads between pathophysiology and pharmacotherapy. Schizophr Res. (2024) 264:71–80. doi: 10.1016/J.SCHRES.2023.12.005
6. Peralta V, García De Jalón E, Moreno-Izco L, Peralta D, Janda L, Sánchez-Torres AM, et al. Long-term outcomes of first-admission psychosis: A naturalistic 21-year follow-up study of symptomatic, functional and personal recovery and their baseline predictors. Schizophr Bull. (2022) 48:631–42. doi: 10.1093/schbul/sbab145
7. Leucht S, Cipriani A, Spineli L, Mavridis D, Örey D, Richter F, et al. Comparative efficacy and tolerability of 15 antipsychotic drugs in schizophrenia: a multiple-treatments meta-analysis. Lancet. (2013) 382:951–62. doi: 10.1016/S0140-6736(13)60733-3
8. Perkins DO, Gu H, Weiden PJ, McEvoy JP, Hamer RM, Lieberman JA. Predictors of treatment discontinuation and medication nonadherence in patients recovering from a first episode of schizophrenia, schizophreniform disorder, or schizoaffective disorder: a randomized, double-blind, flexible-dose, multicenter study. J Clin Psychiatry. (2008) 69:106–13. doi: 10.4088/JCP.V69N0114
9. Bzdok D, Meyer-Lindenberg A. Machine learning for precision psychiatry: opportunities and challenges. Biol Psychiatry Cognit Neurosci Neuroimaging. (2018) 3:223–30. doi: 10.1016/J.BPSC.2017.11.007
10. de Leon J. Personalizing dosing of risperidone, paliperidone and clozapine using therapeutic drug monitoring and pharmacogenetics. Neuropharmacology. (2020) 168. doi: 10.1016/J.NEUROPHARM.2019.05.033
11. Beunk L, Nijenhuis M, Soree B, de Boer-Veger NJ, Buunk AM, Guchelaar HJ, et al. Dutch Pharmacogenetics Working Group (DPWG) guideline for the gene-drug interaction between CYP2D6, CYP3A4 and CYP1A2 and antipsychotics. Eur J Hum Genet. (2024) 32:278–85. doi: 10.1038/S41431-023-01347-3
12. Zhang JP, Malhotra AK. Recent progress in pharmacogenomics of antipsychotic drug response. Curr Psychiatry Rep. (2018) 20. doi: 10.1007/S11920-018-0886-Y
13. Wray NR, Lin T, Austin J, McGrath JJ, Hickie IB, Murray GK, et al. From basic science to clinical application of polygenic risk scores: A primer. JAMA Psychiatry. (2021) 78:101–9. doi: 10.1001/JAMAPSYCHIATRY.2020.3049
14. Murray GK, Lin T, Austin J, McGrath JJ, Hickie IB, Wray NR. Could polygenic risk scores be useful in psychiatry?: A review. JAMA Psychiatry. (2021) 78:210–9. doi: 10.1001/jamapsychiatry.2020.3042
15. Eeltink E, van der Horst MZ, Zinkstok JR, Aalfs CM, Luykx JJ. Polygenic risk scores for genetic counseling in psychiatry: Lessons learned from other fields of medicine. Neurosci Biobehav Rev. (2021) 121:119–27. doi: 10.1016/J.NEUBIOREV.2020.11.021
16. Fusar-Poli L, Rutten BPF, van Os J, Aguglia E, Guloksuz S. Polygenic risk scores for predicting outcomes and treatment response in psychiatry: hope or hype? Int Rev Psychiatry. (2022) 34:663–75. doi: 10.1080/09540261.2022.2101352
17. Lewis CM, Vassos E. Polygenic risk scores: from research tools to clinical instruments. Genome Med. (2020) 12. doi: 10.1186/S13073-020-00742-5
18. Ikeda M, Saito T, Kanazawa T, Iwata N. Polygenic risk score as clinical utility in psychiatry: a clinical viewpoint. J Hum Genet. (2021) 66:53–60. doi: 10.1038/S10038-020-0814-Y
19. Zhang JP, Robinson D, Yu J, Gallego J, Fleischhacker WW, Kahn RS, et al. Schizophrenia polygenic risk score as a predictor of antipsychotic efficacy in first-episode psychosis. Am J Psychiatry. (2019) 176:21–8. doi: 10.1176/APPI.AJP.2018.17121363
20. Sengupta SM, MacDonald K, Fathalli F, Yim A, Lepage M, Iyer S, et al. Polygenic Risk Score associated with specific symptom dimensions in first-episode psychosis. Schizophr Res. (2017) 184:116–21. doi: 10.1016/J.SCHRES.2016.11.039
21. Vassos E, Di Forti M, Coleman J, Iyegbe C, Prata D, Euesden J, et al. An examination of polygenic score risk prediction in individuals with first-episode psychosis. Biol Psychiatry. (2017) 81:470–7. doi: 10.1016/J.BIOPSYCH.2016.06.028
22. Pardiñas AF, Smart SE, Willcocks IR, Holmans PA, Dennison CA, Lynham AJ, et al. Interaction testing and polygenic risk scoring to estimate the association of common genetic variants with treatment resistance in schizophrenia. JAMA Psychiatry. (2022) 79:260–9. doi: 10.1001/jamapsychiatry.2021.3799
23. Segura ÀG, Martínez-Pinteño A, Gassó P, Rodríguez N, Bioque M, Cuesta MJ, et al. Metabolic polygenic risk scores effect on antipsychotic-induced metabolic dysregulation: A longitudinal study in a first episode psychosis cohort. Schizophr Res. (2022) 244:101–10. doi: 10.1016/J.SCHRES.2022.05.021
24. Segura AG, Mezquida G, Martínez-Pinteño A, Gassó P, Rodriguez N, Moreno-Izco L, et al. Link between cognitive polygenic risk scores and clinical progression after a first-psychotic episode. Psychol Med. (2022) 53:4634–47. doi: 10.1017/S0033291722001544
25. Segura ÀG, Prohens L, Gassó P, Rodríguez N, Garcia-Rizo C, Moreno-Izco L, et al. The polygenic basis of relapse after a first episode of schizophrenia. Eur Neuropsychopharmacol. (2023) 75:80–92. doi: 10.1016/J.EURONEURO.2023.06.003
26. Cuesta MJ, Gil-Berrozpe GJ, Sánchez-Torres AM, Moreno-Izco L, García de Jalón E, Peralta V. 20-Year trajectories of six psychopathological dimensions in patients with first-episode psychosis: Could they be predicted? Psychiatry Res. (2024) 331. doi: 10.1016/j.psychres.2023.115614
27. Mas S, Llerena A, Saíz J, Bernardo M, Lafuente A. Strengths and weaknesses of pharmacogenetic studies of antipsychotic drugs: The potential value of the PEPs study. Pharmacogenomics. (2012) 13:1773–82. doi: 10.2217/pgs.12.159
28. Mas S, Gassó P, Lafuente A. Applicability of gene expression and systems biology to develop pharmacogenetic predictors; Antipsychotic-induced extrapyramidal symptoms as an example. Pharmacogenomics. (2015) 16:1975–88. doi: 10.2217/pgs.15.134
29. García de Jalón E, Ariz MC, Aquerreta A, Aranguren L, Gutierrez G, Corrales A, et al. Effectiveness of the early intervention service for first-episode psychosis in Navarra (PEPsNa): Broadening the scope of outcome measures. Spanish J Psychiatry Ment Health. (2023) 16:192–203. doi: 10.1016/J.RPSM.2022.07.002
30. Bernardo M, Bioque M, Parellada M, Saiz Ruiz J, Cuesta MJ, Llerena A, et al. Assessing clinical and functional outcomes in a gene-environment interaction study in first episode of psychosis (PEPs). Rev Psiquiatr Salud Ment. (2013) 6:4–16. doi: 10.1016/J.RPSM.2012.11.001
31. Pelayo-Terán JM, Pérez-Iglesias R, Ramírez-Bonilla ML, González-Blanch C, Martínez-García O, Pardo-García G, et al. Epidemiological factors associated with treated incidence of first-episode non-affective psychosis in Cantabria: insights from the Clinical Programme on Early Phases of Psychosis. Early Interv Psychiatry. (2008) 2:178–87. doi: 10.1111/J.1751-7893.2008.00074.X
32. Salagre E, Arango C, Artigas F, Ayuso-Mateos JL, Bernardo M, Castro-Fornieles J, et al. CIBERSAM: Ten years of collaborative translational research in mental disorders. Rev Psiquiatr Salud Ment. (2019) 12:1–8. doi: 10.1016/J.RPSM.2018.10.001
33. Crespo-Facorro B, de la Foz VOG, Ayesa-Arriola R, Pérez-Iglesias R, Mata I, Suarez-Pinilla P, et al. Prediction of acute clinical response following a first episode of non affective psychosis: results of a cohort of 375 patients from the Spanish PAFIP study. Prog Neuropsychopharmacol Biol Psychiatry. (2013) 44:162–7. doi: 10.1016/J.PNPBP.2013.02.009
34. Kaufman J, Birmaher B, Brent D, Rao U, Flynn C, Moreci P, et al. Schedule for Affective Disorders and Schizophrenia for School-Age Children-Present and Lifetime Version (K-SADS-PL): initial reliability and validity data. J Am Acad Child Adolesc Psychiatry. (1997) 36:980–8. doi: 10.1097/00004583-199707000-00021
35. Sheehan DV, Lecrubier Y, Sheehan KH, Amorim P, Janavs J, Weiller E, et al. The Mini-International Neuropsychiatric Interview (M.I.N.I.): The development and validation of a structured diagnostic psychiatric interview for DSM-IV and ICD-10. J Clin Psychiatry. (1998) 59(Suppl 20):22–33.
36. Perkins DO, Leserman J, Jarskog LF, Graham K, Kazmer J, Lieberman JA. Characterizing and dating the onset of symptoms in psychotic illness: The Symptom Onset in Schizophrenia (SOS) inventory. Schizophr Res. (2000) 44:1–10. doi: 10.1016/S0920-9964(99)00161-9
37. Lewis S, Owen MJ, Murray R. Obstetric complications and schizophrenia: methodology and mechanisms. In: Schulz S, Tamminga H, editors. Schizophrenia. A scientific focus. Oxford University Press, New York (1989).
38. Brugha TS, Cragg D. The List of Threatening Experiences: the reliability and validity of a brief life events questionnaire. Acta Psychiatr Scand. (1990) 82:77–81. doi: 10.1111/J.1600-0447.1990.TB01360.X
39. Bernstein DP, Stein JA, Newcomb MD, Walker E, Pogge D, Ahluvalia T, et al. Development and validation of a brief screening version of the Childhood Trauma Questionnaire. Child Abuse Negl. (2003) 27:169–90. doi: 10.1016/S0145-2134(02)00541-0
40. Cannon-Spoor HE, Potkin SG, Jed Wyatt R. Measurement of premorbid adjustment in chronic schizophrenia. Schizophr Bull. (1982) 8:470–80. doi: 10.1093/SCHBUL/8.3.470
41. Lingjærde O, Ahlfors UG, Bech P, Dencker SJ, Elgen K. The UKU side effect rating scale. A new comprehensive rating scale for psychotropic drugs and a cross-sectional study of side effects in neuroleptic-treated patients. Acta Psychiatr Scand Suppl. (1987) 334:1–100. doi: 10.1111/J.1600-0447.1987.TB10566.X
42. Morisky DE, Green LW, Levine DM. Concurrent and predictive validity of a self-reported measure of medication adherence. Med Care. (1986) 24:67–74. doi: 10.1097/00005650-198601000-00007
43. Kay SR, Fiszbein A, Opler LA. The positive and negative syndrome scale (PANSS) for schizophrenia. Schizophr Bull. (1987) 13:261–76. doi: 10.1093/SCHBUL/13.2.261
44. Kirkpatrick B, Strauss GP, Nguyen L, Fischer BA, Daniel DG, Cienfuegos A, et al. The brief negative symptom scale: psychometric properties. Schizophr Bull. (2011) 37:300. doi: 10.1093/SCHBUL/SBQ059
45. Young RC, Biggs JT, Ziegler VE, Meyer DA. A rating scale for mania: reliability, validity and sensitivity. Br J Psychiatry. (1978) 133:429–35. doi: 10.1192/BJP.133.5.429
46. Montgomery SA, Asberg M. A new depression scale designed to be sensitive to change. Br J Psychiatry. (1979) 134:382–9. doi: 10.1192/BJP.134.4.382
47. Guy W. ECDEU Assessment Manual for Psuchopharmacology Revised: Clinical Global Impression Vol. 1. Rockville, Maryland: NIMH (1976).
48. Reisberg B. Functional assessment staging (FAST). Psychopharmacol Bull. (1988) 24. doi: 10.1016/j.schres.2011.10.015
49. August SM, Kiwanuka JN, McMahon RP, Gold JM. The MATRICS Consensus Cognitive Battery (MCCB): Clinical and cognitive correlates. Schizophr Res. (2012) 134:76–82. doi: 10.1016/j.schres.2011.10.015
50. Drozdick LW, Raiford SE, Wahlstrom D, Weiss LG. The wechsler adult intelligence scale—Fourth edition and the wechsler memory scale—Fourth edition. In: Contemporary intellectual assessment: Theories, tests, and issues, 4th ed. The Guilford Press, New York, NY, US (2018). p. 486–511.
51. Reitan RM, Wolfson D. Category test and trail making test as measures of frontal lobe functions. Clin Neuropsychol. (1995) 9:50–6. doi: 10.1080/13854049508402057
52. Amoretti S, Cabrera B, Torrent C, Bonnín CDM, Mezquida G, Garriga M, et al. Cognitive reserve assessment scale in health (Crash): Its validity and reliability. J Clin Med. (2019) 8. doi: 10.3390/jcm8050586
53. Caudle KE, Sangkuhl K, Whirl-Carrillo M, Swen JJ, Haidar CE, Klein TE, et al. Standardizing CYP2D6 genotype to phenotype translation: consensus recommendations from the clinical pharmacogenetics implementation consortium and dutch pharmacogenetics working group. Clin Transl Sci. (2020) 13:116–24. doi: 10.1111/CTS.12692
54. Raffington L. Utilizing epigenetics to study the shared nature of development and biological aging across the lifespan. NPJ Sci Learn. (2024) 9. doi: 10.1038/S41539-024-00239-5
55. Nabais MF, Gadd DA, Hannon E, Mill J, McRae AF, Wray NR. An overview of DNA methylation-derived trait score methods and applications. Genome Biol. (2023) 24. doi: 10.1186/S13059-023-02855-7
56. Segura ÀG, Prohens L, Mezquida G, Amoretti S, Bioque M, Ribeiro M, et al. Epigenetic clocks in relapse after a first episode of schizophrenia. Schizophr (Heidelberg Germany). (2022) 8. doi: 10.1038/S41537-022-00268-2
57. Segura AG, de la Serna E, Sugranyes G, Baeza I, Valli I, Díaz-Caneja C, et al. Epigenetic age deacceleration in youth at familial risk for schizophrenia and bipolar disorder. Transl Psychiatry. (2023) 13. doi: 10.1038/S41398-023-02463-W
58. Riley RD, Snell KIE, Ensor J, Burke DL, Harrell FE, Moons KGM, et al. Minimum sample size for developing a multivariable prediction model: PART II - binary and time-to-event outcomes. Stat Med. (2019) 38:1276–96. doi: 10.1002/SIM.7992
59. Mas S, Gassó P, Rodríguez N, Cabrera B, Mezquida G, Lobo A, et al. Personalized medicine begins with the phenotype: identifying antipsychotic response phenotypes in a first-episode psychosis cohort. Acta Psychiatr Scand. (2020) 141:541–52. doi: 10.1111/ACPS.13131
60. Hui CLM, Honer WG, Lee EHM, Chang WC, Chan SKW, Chen ESM, et al. Long-term effects of discontinuation from antipsychotic maintenance following first-episode schizophrenia and related disorders: a 10 year follow-up of a randomised, double-blind trial. Lancet Psychiatry. (2018) 5:432–42. doi: 10.1016/S2215-0366(18)30090-7
61. Bernardo M, Bioque M. What have we learned from research into first-episode psychosis? Rev Psiquiatr Salud Ment. (2014) 7:61–3. doi: 10.1016/J.RPSM.2014.03.001
62. Cuesta MJ, Papiol S, Ibañez B, García De Jalón E, Sánchez-Torres AM, Gil-Berrozpe GJ, et al. Effect of polygenic risk score, family load of schizophrenia and exposome risk score, and their interactions, on the long-term outcome of first-episode psychosis. Psychol Med. (2023) 53:6838–47. doi: 10.1017/S0033291723000351
63. Vassos E, Sham P, Kempton M, Trotta A, Stilo SA, Gayer-Anderson C, et al. The Maudsley environmental risk score for psychosis. Psychol Med. (2020) 50:2213–20. doi: 10.1017/S0033291719002319
64. Akbarian S. Epigenetic clocks in schizophrenia: promising biomarkers, foggy clockwork. Biol Psychiatry. (2020) 88:210–1. doi: 10.1016/J.BIOPSYCH.2020.04.004
65. Higgins-Chen AT, Boks MP, Vinkers CH, Kahn RS, Levine ME. Schizophrenia and epigenetic aging biomarkers: increased mortality, reduced cancer risk, and unique clozapine effects. Biol Psychiatry. (2020) 88:224–35. doi: 10.1016/J.BIOPSYCH.2020.01.025
66. Rees E, Owen MJ. Translating insights from neuropsychiatric genetics and genomics for precision psychiatry. Genome Med. (2020) 12. doi: 10.1186/S13073-020-00734-5
67. Crespo-Facorro B, Pelayo-Teran JM, Mayoral-van Son J. Current data on and clinical insights into the treatment of first episode nonaffective psychosis: A comprehensive review. Neurol Ther. (2016) 5:105–30. doi: 10.1007/S40120-016-0050-8
68. Birchwood M, Todd P, Jackson C. Early intervention in psychosis: The critical-period hypothesis. Int Clin Psychopharmacology. (1998) 172:53–9. doi: 10.1097/00004850-199804004-00006
69. Abdel-Baki A, Ouellet-Plamondon C, Malla A. Pharmacotherapy challenges in patients with first-episode psychosis. J Affect Disord. (2012) 138 Suppl. doi: 10.1016/J.JAD.2012.02.029
70. Mayoral-Van Son J, Ortiz-Garcia de la Foz V, Martinez-Garcia O, Moreno T, Parrilla-Escobar M, Valdizan EM, et al. Clinical outcome after antipsychotic treatment discontinuation in functionally recovered first-episode nonaffective psychosis individuals: a 3-year naturalistic follow-up study. J Clin Psychiatry. (2016) 77:492–500. doi: 10.4088/JCP.14M09540
71. Ayesa-Arriola R, Moríñigo JDL, David AS, Pérez-Iglesias R, Rodríguez-Sánchez JM, Crespo-Facorro B. Lack of insight 3 years after first-episode psychosis: an unchangeable illness trait determined from first presentation? Schizophr Res. (2014) 157:271–7. doi: 10.1016/J.SCHRES.2014.05.011
Keywords: personalized medicine, antipsychotic, prediction, psychosis, Pharmacogenetics
Citation: Mas S, Julià L, Cuesta MJ, Crespo-Facorro B, Vázquez-Bourgon J, Spuch C, Gonzalez-Pinto A, Ibañez A, Usall J, Romero-López-Alberca C, Catalan A, Mané A and Bernardo M (2025) Applied pharmacogenetics to predict response to treatment of first psychotic episode: study protocol. Front. Psychiatry 15:1497565. doi: 10.3389/fpsyt.2024.1497565
Received: 17 September 2024; Accepted: 10 December 2024;
Published: 07 January 2025.
Edited by:
Michal Assaf, Olin Neuropsychiatry Research Center (ONRC), United StatesReviewed by:
Jeffrey Bishop, University of Minnesota Twin Cities, United StatesCopyright © 2025 Mas, Julià, Cuesta, Crespo-Facorro, Vázquez-Bourgon, Spuch, Gonzalez-Pinto, Ibañez, Usall, Romero-López-Alberca, Catalan, Mané and Bernardo. This is an open-access article distributed under the terms of the Creative Commons Attribution License (CC BY). The use, distribution or reproduction in other forums is permitted, provided the original author(s) and the copyright owner(s) are credited and that the original publication in this journal is cited, in accordance with accepted academic practice. No use, distribution or reproduction is permitted which does not comply with these terms.
*Correspondence: Sergi Mas, c2VyZ2ltYXNoQHViLmVkdQ==
Disclaimer: All claims expressed in this article are solely those of the authors and do not necessarily represent those of their affiliated organizations, or those of the publisher, the editors and the reviewers. Any product that may be evaluated in this article or claim that may be made by its manufacturer is not guaranteed or endorsed by the publisher.
Research integrity at Frontiers
Learn more about the work of our research integrity team to safeguard the quality of each article we publish.