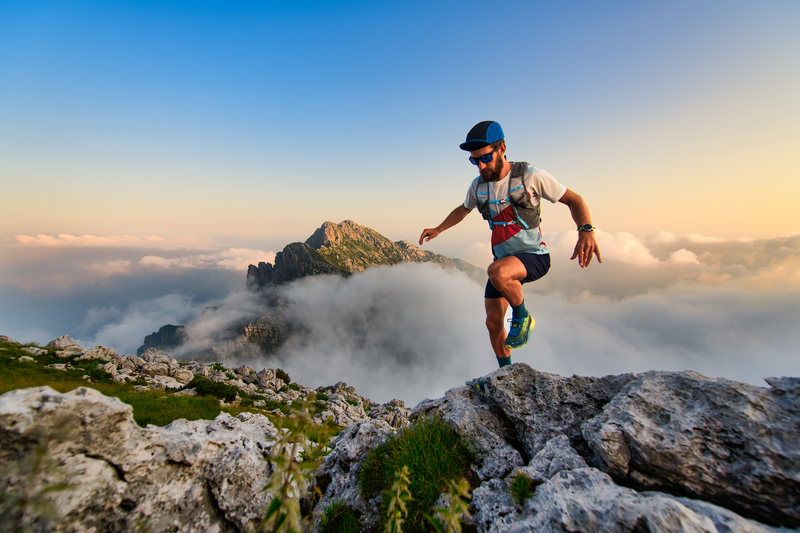
95% of researchers rate our articles as excellent or good
Learn more about the work of our research integrity team to safeguard the quality of each article we publish.
Find out more
REVIEW article
Front. Psychiatry , 16 October 2024
Sec. Autism
Volume 15 - 2024 | https://doi.org/10.3389/fpsyt.2024.1474003
This article is part of the Research Topic Neuroimaging Advances in Understanding Neurodevelopmental Disorders: Bridging Neuropathology and Treatment View all articles
Background: A growing body of literature classifies autism spectrum disorder (ASD) as a heterogeneous, complex neurodevelopmental disorder that often is identified prior to three years of age. We aim to provide a narrative review of key structural and functional properties that differentiate the neuroimaging profile of autistic youth from their typically developing (TD) peers across different neuroimaging modalities.
Methods: Relevant studies were identified by searching for key terms in PubMed, with the most recent search conducted on September 1, 2023. Original research papers were included if they applied at least one of seven neuroimaging modalities (structural MRI, functional MRI, DTI, MRS, fNIRS, MEG, EEG) to compare autistic children or those with a family history of ASD to TD youth or those without ASD family history; included only participants <18 years; and were published from 2013 to 2023.
Results: In total, 172 papers were considered for qualitative synthesis. When comparing ASD to TD groups, structural MRI-based papers (n = 26) indicated larger subcortical gray matter volume in ASD groups. DTI-based papers (n = 14) reported higher mean and radial diffusivity in ASD participants. Functional MRI-based papers (n = 41) reported a substantial number of between-network functional connectivity findings in both directions. MRS-based papers (n = 19) demonstrated higher metabolite markers of excitatory neurotransmission and lower inhibitory markers in ASD groups. fNIRS-based papers (n = 20) reported lower oxygenated hemoglobin signals in ASD. Converging findings in MEG- (n = 20) and EEG-based (n = 32) papers indicated lower event-related potential and field amplitudes in ASD groups. Findings in the anterior cingulate cortex, insula, prefrontal cortex, amygdala, thalamus, cerebellum, corpus callosum, and default mode network appeared numerous times across modalities and provided opportunities for multimodal qualitative analysis.
Conclusions: Comparing across neuroimaging modalities, we found significant differences between the ASD and TD neuroimaging profile in addition to substantial heterogeneity. Inconsistent results are frequently seen within imaging modalities, comparable study populations and research designs. Still, converging patterns across imaging modalities support various existing theories on ASD.
The prevalence of autism spectrum disorder (ASD) has risen drastically from 6.7 to 27.6 per 1,000 in children in the United States over the past twenty years; thus, investigating its neurobiological underpinnings is critically important (1, 2). ASD is a neurodevelopmental condition characterized by social communication deficits and restrictive interests or repetitive behaviors (3). Its clinical presentation is highly heterogeneous, and different symptom profiles are associated with varying levels of severity and impairment (4, 5). Notably, factors such as age, IQ, sex/gender, race, and ethnicity can greatly affect clinical presentation, others’ perception of the ASD phenotype, and subsequent diagnosis (6–9). As such, it is likely that more than the estimated 1 in 23 male children and 1 in 88 female children in the United States are affected (2, 10). Various factors likely contribute to increasing prevalence of ASD diagnoses, including change of diagnostic criteria, increased awareness of parents and health care providers, and improved recognition of ASD symptoms in girls. Still, these factors do not fully explain the rising incidence of ASD, and care should be taken to not dismiss the notion that the actual number of autistic individuals is increasing, rather than just the number of diagnoses (11, 12).
As the community of individuals affected by ASD continues to grow, it is essential to understand the neurobiological and environmental processes that contribute to autistic people’s highly diverse experiences. While no single neurobiological mechanism or cause has been identified for ASD, numerous genetic and epigenetic processes are implicated in its etiology (13, 14). The genetic heritability of the disorder is well established, with twin studies estimating the proportion of the phenotype variance due to genetic factors to be between 60-90% (15, 16). This should provide a target in the effort to characterize the genetic contribution to the etiology of ASD. However, autistic individuals have substantial genotypic as well as phenotypic variability (17). Developmental processes such as executive function (18, 19) and myelination (20) have heterogeneous trajectories from childhood into young adulthood. Understanding how genetic differences lead to various neurobiological and behavioral presentations will allow clinicians to better understand and support autistic youth.
We aimed to conduct this research through a neurodiversity framework, acknowledging the work that autistic scholars are doing to decrease stigma and increase acceptance in the autism research space (21–23). There is an ongoing discussion in the autism research and advocacy communities about whether person-first language (i.e., “youth with autism”) or identity-first language (i.e., “autistic youth”) should be used when discussing people on the autism spectrum (24, 25). We have chosen to use identity-first (also known as disability-first) language in this review because it currently represents the most accepted language in the autism community (24, 26–28). However, we recognize that all language has the possibility to offend or exclude some individuals, and that language changes over time. Similarly, research priorities in the autism field are changing. Participatory research with ASD stakeholders has highlighted the imbalance between existing research, largely in the biomedical and neuroscience spheres, and the need to investigate issues like stigma and support needs (29–31). Despite the wealth of existing literature on the neurobiology of ASD, it has proven difficult to translate these findings into a causal framework or into real-world change in the lives of autistic people.
Autism research in the 1980s and 1990s focused largely on behavioral phenotyping, genetics, and identifying cognitive features of ASD (32–34). Since the turn of the century, neuroimaging tools have been instrumental in the quest to uncover biological mechanisms underlying the disorder. Structural and functional magnetic resonance imaging (sMRI and fMRI, respectively) techniques, as well as electroencephalography (EEG) and magnetoencephalography (MEG), have steadily advanced and become more accessible to researchers. Novel neuroimaging methods including magnetic resonance spectroscopy (MRS), diffusion tensor imaging (DTI), and functional near-infrared spectroscopy (fNIRS) have been increasingly adopted in ASD research. These technological advancements have led to a large body of literature examining differences between the brain in those with and without ASD. Currently, prominent theories for the etiology of ASD include the excitation/inhibition (35, 36), maternal-immune (37), cerebral connectivity (38), dopamine (39), and mitochondrial dysfunction (40) hypotheses. While neuroimaging research has improved our collective understanding of ASD, the results from these studies have been mixed. For example, there is research supporting increased excitation, increased inhibition, and no excitation/inhibition imbalance in autistic participants (41). These inconsistencies may be due in part to discrepancies in research methods: e.g., many researchers opt to include participants across wide age ranges, making it difficult to identify specific neurodevelopmental patterns. Study populations also differ in the inclusion and consideration of autistic participants with more severe symptoms, greater support needs, intellectual disability, language impairment, and co-occurring disorders. Moreover, hypothesis vs. data-driven approaches in neuroimaging research yield heterogeneous results, and many researchers may be hesitant to report null findings.
Analyses using a single neuroimaging technique may not be sufficient to capture the complex neural correlates underlying such a heterogeneous condition. Comparing similar constructs of brain structure or function (e.g., connectivity) across different neuroimaging modalities (e.g., fMRI, DTI, EEG, and MEG) could provide stronger evidence for specific phenotypic findings. There have been both empirical studies (42–45) and reviews (46–48) describing multimodal investigation into the neural correlates of ASD. However, there have not been any large-scale analyses exploring whether there are consistent brain findings in autistic youth across the vast range of modern neuroimaging modalities. In this literature review, we aim to examine MRI, DTI, MRS, fNIRS, MEG, and EEG findings from the past decade to determine what structural, functional, and chemical differences exist between autistic and neurotypical youth. Moreover, we intend to compare findings across modalities to identify converging patterns and highlight inconsistencies in ASD neuroimaging research.
Relevant studies were identified by searching PubMed with the terms detailed in Supplementary Table 1. The most recent search was conducted on September 1, 2023. We used the PICOTS framework (population, intervention, comparison, outcome, timing) to formulate search terms. The population included autistic youth across the autism spectrum; the intervention included neuroimaging modalities; the comparison included typically developing youth; the outcome included structural, functional and chemical brain differences between autistic and neurotypical youth; the timing included articles published between January 1, 2013, and September 1, 2023. A separate search was completed for each imaging modality to ensure that a maximum number of papers would be included (Supplementary Table 1).
Articles were initially included based on the following criteria: empirical research with cross-sectional or longitudinal design; publication in 2013 or later; population with an ASD diagnosis, ASD traits, or family history of ASD and typically developing comparison group; study population mean age under 25 years old; use of at least one neuroimaging modality (computed tomography (CT), DTI, EEG, MEG, fMRI, sMRI, MRS, fNIRS, positron emission tomography (PET), single-photon emission computed tomography (SPECT), ultrasound); article published in peer-reviewed journal; full text available in English.
Articles were excluded based on the following criteria: reviews, meta-analyses, case studies/series, qualitative studies, theses, editorials, clinical trials, and book chapters; unavailable full texts, abstract-only texts, papers published before 2013, papers not published in English; animal research; articles unrelated to autism or imaging, study populations not containing participants with ASD or not containing a TD group; studies of clinical groups with known ASD causes or where the focus is on specific diagnoses (e.g. Fragile X, tuberous sclerosis); does not use a neuroimaging modality listed in inclusion criteria (e.g., electromyography (EMG), postmortem); mean age of study population over 25 years-of-age.
Study selection involved four separate stages as shown in Figure 1. Duplicates were removed prior to title/abstract screening. The work associated with screening, reading, and synthesis was distributed among seven authors (ARH, SNV, BG, ML, MAMP, LOS, MO) at all stages. Team members met weekly to review progress, discuss challenges, and cross-check results and inconsistencies.
Records included in primary evaluation were required to meet all inclusion/exclusion criteria. A breakdown of records included and excluded at each stage in each neuroimaging modality can be found in Supplementary Table 2. During primary analyses, the authors reached consensus that additional inclusion/exclusion criteria needed to be implemented to address the heterogeneity of samples and outcomes across papers. Articles that included any participants over the age of 18 were subsequently excluded to focus the literature review on a pediatric population. We based the sample size cutoff on the central limit theorem and only included papers with N > 25 for both the case (ASD) and the control (typically developing youth) groups. This sample size has a greater probability of approximating a normal distribution for the continuous imaging metrics and decreases the risk of including false positives (49, 50). This criterium was not applied to MRS and fNIRS studies, since sample size for these modalities is generally smaller than N = 25, and this exclusion criterium would prohibit any meaningful analysis for these specific modalities. Several articles used data from the Autism Brain Imaging Data Exchange (ABIDE), which resulted in substantial overlap in study populations. Further, not all studies that used ABIDE data specified which ABIDE research sites were included. To limit overlap between study populations in our literature review, only the 2014 ABIDE summary paper was included; all other studies using data from ABIDE were excluded (51). Ultimately, 172 full-text articles were included for the qualitative synthesis.
The following data were extracted from each paper: sample size; participants’ age, sex/gender, IQ; ASD measurement tools; study design; country; neuroimaging outcomes comparing ASD and TD groups. Since most articles did not specify how they determined the sex or gender of participants, describe their framework for considering sex differences, and/or did not include female participants, we did not include sex/gender differences in our qualitative analysis (52). We chose to include articles that compared participants with and without a family history of ASD (as opposed to a formal ASD diagnosis) to encompass autistic youth who may be too young to receive a formal diagnosis. Additionally, two papers that did not include participants formally diagnosed with ASD were included to account for the full spectrum of subclinical autism traits. Outcomes specific to behavioral symptoms and within-group comparisons were not included.
We performed a qualitative synthesis to summarize the evidence, since heterogeneous methods, samples, and data reporting across included studies did not allow for quantitative analyses. Key findings from each paper were summarized into Supplementary Tables 3–9, which were originally organized by modality and ordered based on the average age of the sample. If a paper reported outcomes relevant to two or more modalities, those outcomes were listed under each relevant modality. Team members discussed key findings and brain regions that emerged as relevant across multiple modalities. One team member (SNV) summarized key findings for relevant brain regions: findings relevant to the regions that were most frequently identified in each of Supplementary Tables 3–9 were included. These findings were organized by region, then modality, and data was extracted for total sample size (ASD and TD groups), mean age (weighted mean between ASD and TD groups), and neuroimaging outcome.
Key outcomes for MEG and EEG papers followed a different framework since encephalography does not provide adequate spatial specificity for region-specific evaluation. MEG and EEG findings were organized into tables based on domain (e.g. event-related potentials, frequency/power). Team members then discussed concordant findings between MEG and EEG studies, as well as commonalities between these studies and those using MR-based neuroimaging methods.
To safeguard consistency during qualitative synthesis, two separate team members read each paper. The primary reader synthesized key findings of the paper. The second reader cross-validated the synthesized outcomes with the original findings reported in the paper. In case of disagreement between the primary and a second reader, a third reader (IFV) reviewed the paper and determined the final outcome.
Since we did not perform a formal systematic or scoping review, we did not perform a formal quality and bias assessment of each paper. However, we identified areas of potential and major limitations during reading and consensus meetings, and we will discuss these limitations in Section 4.1 below.
Box and violin plots were created using R Statistical Software (v4.4.0, R Core Team 2024) with the following packages: magrittr v2.0.3 (53), tibble v3.2.1 (54), vctrs v0.6.5 (55), ggplot2 v3.5.1 (56), tidyr v1.3.1 (57), and plyr v1.8.9 (58). We summarized all findings from 172 papers into three-dimensional brain figures and bubble plots. To visualize recurring themes in neuroimaging literature by modality, bubble plots were generated to identify mentions of specific brain regions and metrics/domains measured across studies. Findings were organized by neuroimaging modality. Each finding was registered as an entry including sample size, brain region, and the associated metric/domain. Findings were manually assigned directional values (-1, -0.5, 0, 0.5, and 1) indicating negative, partial negative, neutral, partial positive, or positive directionality relative to participants with ASD. For example, language indicating ‘ASD group has more’, ‘ASD group has higher’, or ‘ASD group has faster’ corresponded to positive values, while terms like ‘less’, ‘lower’, and ‘slower’ were assigned negative values. Findings without group differences were assigned values of 0. Partial directionality was assigned to findings involving sex-related or ASD-subtype group differences. Additionally, findings were weighted by sample size. Eight bubble plots were generated to depict the aggregated directionality and sample size weights for findings categorized by brain region and related metric/domain. The “whole brain” row in each bubble plot represents outcomes in papers that performed whole-brain analysis for a given metric, not the sum of our own analyses for that metric. We have chosen not to display numeric values for aggregated directionality because of the qualitative nature of this review, and the numeric values do not reflect statistical significance. Future studies that combine the raw data from each modality and each study and perform meta-analyses would be well suited to provide quantitative findings. Bubble plots were created in RStudio, using packages forcats v1.0.0 (59) and ggplot2 v3.5.1 (56).
To create the three-dimensional brain figures, we documented the directionality of differences between ASD and TD groups findings per imaging modality, associated brain regions, and sample size. Next, we created two values for every brain region found in each modality: directionality and opacity. Cumulative directionality for each brain region was calculated by combining all papers that found a significant difference or null finding between ASD and TD group: a positive direction indicated that ASD group had a higher value of a given neuroimaging metric compared to TD groups; a negative direction indicated that the ASD group had a lower value of a given neuroimaging metric compared to TD groups; neutral indicated a null finding. For example: several papers with conflicting findings on regional volume may result in a cumulative null as directionality score. Opacity ranges from 0 to 1 and is calculated by summing the sample sizes of all papers contributing to a region’s directionality score and dividing this number by the largest combined sample size found in that region. Thus, the brain region which has the largest overall sample size will have an opacity of 1, being fully visible, while regions that do not have any findings will have a sample size of 0, thus being invisible in the figure. We then applied these directionality and opacity scores to the brain regions included in the Desikan-Killiany atlas (60). All brain figures were created in ITK-SNAP Version 4.2.0. The directionality score dictates the red/green/blue (RGB) value of the region, ranging from blue (negative) to red (positive) (61). In summary, the color is determined by the directionality and the opacity by sample size. For DTI, we applied the same process but used the ITT Human Brain Atlas (v.5.0) WM atlas. In this atlas, every white matter voxel is assigned a label indicating the two most likely gray matter regions that are connected by the white matter in the voxel (62, 63). For fMRI, we included a functional connectivity figure, which was visualized using the BrainNet Viewer Version 1.7 (64). We presented functional connectivity via a node network. The nodes represented brain regions (i.e. amygdala, anterior cingulate cortex, posterior cingulate cortex, prefrontal cortex, dorsolateral prefrontal cortex, temporal lobe central gyri, occipital lobe, parietal lobe, thalamus, cerebellum, and frontal lobe). The edges represented the functional connectivity findings between these regions.
Results are presented in the following order: study selection process (Figure 1), characteristics of selected papers (Table 1, Figure 2, Section 3.1), within-modality findings in Section 3.2, including global and brain map/bubble plot-based findings, followed by region-specific findings across modalities in Section 3.3. Global findings reference Supplementary Tables 3–9, brain maps and bubble plot-based findings reference Figures 3–8, and region-specific findings reference Tables 2–4. An additional brain map depicting subcortical regions relevant in each modality is available in Supplementary Figure 1.
Figure 2. Age and IQ violin plots. (A) Box plots depict mean age (black diamond) and median age (black dash) for ASD (left, blue) and TD (right, red) participants across all publications within a modality. Violin plots convey distribution of mean ages across studies within a neuroimaging modality. (B) Box and violin plots depict mean, median, and distribution of mean IQ for studies within each neuroimaging modality.
Figure 3. Patterns of sMRI findings. (A) Bubble plot and (B) brain map depicting regional patterns of structural MRI findings. Red indicates positive findings (e.g. larger volume), while blue indicates negative findings (e.g. smaller volume). Bubble size in (A) and opacity in (B) represent the number of participants associated with each finding.
Figure 4. Patterns of DTI findings. (A) Bubble plot and (B) brain map depicting regional patterns of DTI findings. Red indicates positive findings (e.g. higher diffusivity), while blue indicates negative findings (e.g. lower fractional diffusivity). Diffusivity directionality signage was inversed for brain maps to reflect white matter integrity (i.e. higher mean diffusivity values and lower fractional anisotropy values are both mapped as blue). Bubble size in (A) and opacity in (B) represent the number of participants associated with each finding.
Figure 5. Patterns of fMRI findings. (A) Bubble plot and (B) brain map depicting intra-regional patterns of functional MRI findings. Red indicates positive findings (e.g. increased local functional connectivity), while blue indicates negative findings (e.g. decreased local functional connectivity). (C) Bubble plot and (D) brain map depicting inter-regional patterns of functional MRI findings. Pink indicates positive findings (e.g. increased long-range functional connectivity), while blue indicates negative findings (e.g. decreased long-range functional connectivity). Bubble size in (A, C) and opacity in (B, D) represent the number of participants associated with each finding.
Figure 6. Patterns of MRS findings. (A) Bubble plot and (B) brain map depicting regional patterns of MRS findings. Red indicates positive findings (e.g. increased metabolites associated with excitation), while blue indicates negative findings (e.g. increased metabolites associated with inhibition). Bubble size in (A) and opacity in (B) represent the number of participants associated with each finding.
Figure 7. Patterns of fNIRS findings. (A) Bubble plot and (B) brain map depicting regional patterns of fNIRS findings. Red indicates positive findings (e.g. increased oxy-Hb), while blue indicates negative findings (e.g. increased deoxy-Hb). Bubble size in (A) and opacity in (B) represent the number of participants associated with each finding.
Figure 8. Patterns of MEG and EEG findings. (A) Bubble plots depicting regional patterns of (A) MEG and (B) EEG findings. Red indicates positive findings (e.g. increased total power), while blue indicates negative findings (e.g. decreased total power). Bubble size in represents the number of participants associated with each finding.
Figure 1 shows the flowchart of the study selection process. Of the 2,794 initial records identified, 989 duplicates were excluded. The remaining 1,805 records were screened, with 797 being excluded based on title and abstract. The remaining 1,008 full-text records were assessed for eligibility, resulting in exclusion of 316 additional records, leaving 692 full-text records included in a primary evaluation. Following application of the secondary set of exclusion criteria, 520 records were excluded, and 172 final papers including 26 sMRI (65–90), 14 DTI (91–104), 41 fMRI (51, 105–144), 19 MRS (145–163), 20 fNIRS (164–183), 20 MEG (184–203), and 32 EEG (204–235) articles were considered in qualitative analysis.
Characteristics of the included articles are presented in Table 1. The majority of studies followed a cross-sectional design (n = 152); a small number (n = 20) had longitudinal designs. The age distribution was right-skewed, with 32% of the selection having a mean age within 0-5 years (n = 55), and 48% of the selection having a mean age within 6-10 years old (n = 48). Approximately 38% of the articles had mean age fall between 11-15 years old (n = 66), and only 2% fell between 15-18 years old (n = 3). Distributions of mean age across modalities are presented in Figure 2A. Distributions of mean age between ASD and TD groups were similar across all seven modalities, as would be expected given frequent age-matching of groups. DTI, MEG, and sMRI showed distributions with mean ages in early childhood and early adolescence. EEG, MRS, and fMRI had mean ages of around 10, 12, and 13 years, respectively. The distribution of fNIRS papers ranged from 0 to 16 years. Aside from several outliers, the fMRI group distributions most closely approximated normality, with a peak in early adolescence.
Female participants were included in 82% of the studies (n = 141), 16% of studies included male participants only (n = 28), and 3 papers did not report sex. When both male and female participants were included, the percentage of female participants fell between 9.8% and 76.0%, with a mean value of 23.7%. IQ distribution was not reported in 53 studies (31%). IQ thresholds were used as exclusion criteria in 21 studies (12%), using thresholds of greater than either 75 or 80. About one quarter of studies (22%) matched ASD and TD groups on IQ. The ASD group had a lower average IQ score in 20% of papers that did not IQ-match participants. Distributions of mean IQ scores across modalities are presented in Figure 2B. Across imaging modalities, mean IQ values were lower for ASD groups than for TD groups. ASD groups showed wider distributions of mean IQ, and more outliers, with mean IQ values occasionally falling below 80. sMRI is the only modality with an ASD distribution containing mean IQ values below 60, and very few mean IQ values in any distribution outside sMRI fell below 80.
Most papers (n = 157) featured ASD/TD case-control study arms. Some articles compared infants with a family-history of ASD to infants without ASD family history to compare high- vs. low-likelihood cases (n = 13). Two papers contained only a TD group, where the authors assessed ASD traits in school-aged children. ASD traits in school-aged children may still reflect part of the autism spectrum, despite being undiagnosed or subclinical.
Nearly 60% of studies were carried out in North America (n = 100), while 25% were carried out in Asia (n = 44) or 12% in Europe (n = 20). Most studies used fMRI (n = 41), EEG (n = 32) or sMRI (n = 26) modalities. Less frequent modalities by number of papers included fNIRS (n = 20), MEG (n = 20), MRS (n =19), and DTI was the least frequent modality (n = 14). Sample sizes in MRS, MEG and fNIRS papers were small (n < 50 per modality), following our inclusion criteria. Most other modalities had a sample size between 51-100 participants (45% of studies). There were no CT, PET, SPECT, or ultrasound studies that met inclusion criteria for the qualitative analysis. We hypothesize that the modern non-invasive neuroimaging modalities included in this review are favored in the literature because they do not require radiation exposure and have improved spatial resolution.
We identified differences between the ASD and TD neuroimaging profiles within each of the seven neuroimaging modalities. Structural MRI-based papers (n = 26, Supplementary Table 3; Figure 3) demonstrated larger overall subcortical gray matter volume in ASD groups. However, there were positive and negative differences in cortical gray matter volumes, i.e. both larger and smaller volumes in ASD groups compared to TD across various brain regions (65, 70, 78, 89). The amygdala, thalamus, hippocampus, and corpus callosum were the most prominent regions assessed in sMRI papers. Evaluation of cortical thickness, structural covariance, cerebrospinal fluid volume, gyrification, intracranial volume, surface area, and total brain volume did not reveal any consistent patterns (Figure 3).
DTI-based papers (n = 14, Supplementary Table 4; Figure 4) reported overall higher mean and radial diffusivity in ASD groups compared to TD groups (94, 96, 99, 102, 104, 108, 113, 133), and mixed directionality of fractional anisotropy (FA) findings (91, 92, 98, 100, 102, 103, 198). A small number of papers indicated higher global axial diffusivity in ASD (92, 93, 95, 99). Several DTI studies focused specifically on the corpus callosum, superior longitudinal fasciculus, and cingulum (91–93, 96, 102, 104). The corpus callosum (CC) appeared most often in DTI studies, with ASD groups showing overall higher axial diffusivity, notably higher FA values, lower mean diffusivity, and mixed radial diffusivity findings compared to TD groups (Figure 4) in this structure. Overall, findings suggest lower white matter microstructural integrity in the ASD group (Figure 4B).
Functional MRI-based papers (n = 41, Supplementary Table 5; Figure 5) also demonstrated substantial variability in findings. Within-network, regional connectivity and task-based functional activity patterns were highly mixed (Figures 5A, B), showing both increased and decreased connectivity or activation in various brain regions, without a clear pattern (51, 106, 113, 115, 116, 118, 123, 125–127, 131, 132, 135, 140–142). Studies with larger sample sizes indicate that ASD participants have higher within-region functional connectivity in subcortical regions and lower within-region connectivity in the default mode network and ventral attention network (Figure 5A) compared to TD groups. Between-network (Figures 5C, D) findings were also highly mixed (51, 105–108, 111–114, 116, 118, 120, 121, 124, 126, 128–131, 134–137, 141, 163). There was an abundance of between-network connectivity findings in the amygdala, thalamus, default mode network, insula, and prefrontal cortex (105–108, 111, 113, 114, 118, 121, 126, 128, 134, 136, 137, 163). Figures 5C, D shows higher between-region functional connectivity across these brain regions. Between-network connectivity involving the amygdala and primary visual cortex (V1) is mostly decreased in ASD groups, whereas between-network connectivity involving the imitation network, language regions, paracingulate cortex, and thalamus is mostly increased in ASD groups compared to TD groups.
MRS-based papers (n = 19, Supplementary Table 6; Figure 6) overall reported higher metabolite markers of excitatory neurotransmission (145–147, 149, 150, 154–157, 159), lower metabolite markers of inhibitory transmission (156, 161, 162, 198), and lower markers of overall brain health in ASD groups (145, 147, 151–153, 158, 160). ASD groups appear to have lower markers of neuronal health such as n-acetylaspartate (NAA), choline-containing compounds, and creatine (Figure 6A). The anterior cingulate cortex (ACC) figured the most prominently in MRS papers (146, 152, 155–157, 160). Contrary to global findings, findings specified for region and MRS metric suggested that markers of excitation and inhibition were mixed and heterogenous across brain regions (Figure 6).
fNIRS-based papers (n = 20, Supplementary Table 7; Figure 7) demonstrated overall lower oxygenated hemoglobin (oxy-Hb) levels in ASD groups (164–168, 175–177, 179, 181), with one report indicating increased rightward lateralization (180). The ASD groups demonstrate a notably lower oxygenated hemoglobin levels in the dorsolateral prefrontal cortex (DLPFC), prefrontal cortex (PFC), and various temporal regions compared to TD groups (167, 168, 172–174, 177, 178). Lower oxy-Hb across brain regions indicates decreased task-related activation in the ASD group overall (Figure 7).
The lack of spatial specificity in these waveform-based imaging modalities prevented us from producing brain maps for MEG or EEG. MEG-based papers (n = 20, Supplementary Table 9; Figure 8A) reported decreased auditory ERP amplitude in ASD (188, 202). A small number of MEG studies suggested shorter ERF latency and higher total power (188, 196, 197, 201, 203). Contrary to global findings suggesting higher overall MEG power, region and domain-specific power/frequency findings in MEG papers are mixed; with most cortical regions showing higher power, while investigations of frontal regions and the whole brain noted lower activity and power (Figure 8A). Additionally, there are mixed region-specific connectivity and ERF findings. However, there is consensus that ASD participants have lower amplitude to auditory-evoked fields.
Consistently with MEG, EEG-based papers (n = 32, Supplementary Table 8; Figure 8B) reported decreased auditory and visual ERP amplitude and decreased alpha band power (208, 210, 218, 225–229, 232). ASD groups demonstrated higher whole-brain EEG connectivity (Figure 8B). Likewise, a decrease is seen in EEG frequency/power in diffuse anterior, central, and posterior regions of the brain. In addition, Figure 8B indicated mixed findings for EEG frequency/power in the whole brain. Lower auditory ERP amplitude is also observed.
Across modalities, notable patterns emerged when assessing all findings specific to cortical gray matter regions (Table 2). In the anterior cingulate cortex, ASD groups demonstrated higher markers of neuronal excitation (glutamate, Glu; and glutamine, Gln) (146, 157, 159) and higher functional connectivity within the thalamus and ventrolateral prefrontal cortex compared to TD groups (136). The insula in ASD showed altered functional connectivity with various cortical regions in resting state and task-based paradigms (107, 118, 121). The right insula specifically showed increased white matter volume and decreased cortical thickness (66, 102), as well as lower mean diffusivity in the insular cortex (102). Findings for the prefrontal cortex showed altered resting state functional connectivity with various cortical regions (108, 111, 113, 134, 136, 163). This region also exhibited lower oxy-Hb signal during administration of task involving social stimuli (167, 168). Studies reported less gray matter in the left anterior PFC (78) and lower markers of neuronal health in the medial PFC (145).
When assessing all findings specific to subcortical gray matter regions, the amygdala, thalamus and cerebellum were described more frequently than other regions (Table 3). Findings for the amygdala demonstrated larger volume in ASD groups compared to TD groups, even in early infancy (79, 85, 86). Additionally, amygdala-specific findings suggested decreased regional functional connectivity and between-network connectivity with several cortical structures implicated in emotional processing, including the medial prefrontal cortex, striatum, thalamus, cingulate cortex, and cerebellum, as well as the inferior occipital gyrus (114, 133, 134). One study found lower NAA levels in the amygdala in ASD participants compared to TD participants (158). The thalamus showed increased functional connectivity with subregions of the auditory cortex, Heschl’s gyrus, primary sensory cortex and prefrontal cortex (128, 129, 131). The cerebellum demonstrated decreased resting-state functional connectivity with amygdala, and locally, as indicated by decreased regional homogeneity in the right anterior cerebellum (134, 143). The cerebellum likewise demonstrated decreased GABA/Cr ratio and a negative correlation between GABA/Cr ratio and functional connectivity with the thalamus (156, 163). Only one study reported volume differences in the cerebellum across age in ASD compared to TD groups (87).
The region-specific findings for white matter structures and functional networks are presented in Table 4. Findings for the corpus callosum in ASD demonstrated both higher and lower fractional anisotropy, and a various age- and sex- associated differences in volume, fractional anisotropy, and axial diffusivity (91–93, 102, 104). Volumetric findings for the corpus callosum were mixed: both larger volumes and higher rates of hypoplastic corpus callosum were reported (69, 90). Findings for the default mode network showed significantly altered connectivity in both directions with the salience network (105, 137), as well as larger volume in default mode network structures (144).
The aim of this literature review was to determine patterns of differences in brain structure, function and neurochemistry between autistic and typically developing youth, and to assess converging patterns and inconsistencies within and across multiple neuroimaging modalities. In our literature synthesis of 172 papers, we found considerable heterogeneity in reported findings across all neuroimaging modalities but were able to identify some consistent patterns of difference between ASD and TD groups.
ASD groups had larger overall subcortical gray matter volume compared to TD, as well as higher mean and radial diffusivity. Literature further indicates that ASD youth had higher metabolite markers of excitatory neurotransmission, lower metabolite markers of inhibitory neurotransmission, and lower markers of overall brain health. EEG and MEG studies showed that ASD youth had decreased auditory ERP amplitude, and visual ERP amplitude in EEG-studies only. The anterior cingulate cortex, insula, prefrontal cortex, amygdala, thalamus, cerebellum, corpus callosum, and default mode network appeared consistently as regions of interest across all seven neuroimaging modalities.
Our findings corroborate a substantial body of existing literature (236–238) suggesting that the neurobiology of ASD is highly heterogeneous. Findings across every modality were notably inconsistent, as demonstrated by mixed findings in the bubble plots and brain maps. Differences in a neuroimaging metric (e.g. connectivity, activation, volume) between groups could rarely be defined in one direction. These issues were particularly salient in functional MRI papers, where patterns in connectivity, resting-state activation, and task-based activation were different in nearly every publication. A similar picture arose for structural MRI, with a wide variety of regions and metrics reported across studies.
These inconsistencies within imaging modalities may be explained by multiple factors. Firstly, there was considerable heterogeneity in the study populations, as illustrated by differences in the mean age and age ranges within and across neuroimaging modalities. While our secondary exclusion criteria (limiting participants to under 18-year-olds and case/control arms to N>25) theoretically decreased the heterogeneity of our samples and contributed to a lower risk of spurious findings due to very small sample sizes, enforcing a larger sample size potentially increased the heterogeneity of our findings by adding heterogeneity into ASD symptomatology. However, the innate heterogeneity of ASD will be present regardless of sample size, and we believe the importance of reducing the risk of spurious findings to be greater. Comparisons between specific age groups were complicated by large variations in analytical choices adopted by research groups. Issues related to the handling of sex/gender and IQ were common: a substantial proportion of studies had only male participants or a minority of female participants, and many studies did not report in IQ or excluded participants with IQ scores below 75 or 80 points. IQ measures were not evenly distributed between ASD and TD groups, indicating differences in selection into study samples. Since differences in cognitive performance have been shown to be related to autism and the broader autism phenotype (239), not matching on IQ introduces a considerable confound. Another potential method of controlling for cognitive performance-linked elements of ASD could be to match participants on parental measures of cognitive performance, or education level as a proxy for parental IQ when participant IQ cannot be measured. Further, race and ethnicity were rarely considered in the analyses, nor reported in the manuscripts. Not considering important factors such as ethnicity, sex, and IQ in study designs and analyses could introduce selection and confounding bias into analyses on neurobiological phenotypes, which may result in different findings reported in many of the studies.
Moreover, a decade of neuroimaging research has contributed significantly to the growth of analytical methods for complex neuroimaging data: the field has moved from mostly region of interest and hypothesis-driven studies to focus more on whole brain, hypothesis-free analyses. Both study types have the potential to introduce bias and inconsistency into reported findings. There are clear advantages statistically in the pre-hoc selection of brain regions of interest in the analysis of large quantities of neuroimaging data (240), though it is evident that such selection frequently depends on a priori assumptions about brain and behavior which can lead to potential bias in theoretically favored regions (241), at the expense of theoretically disfavored regions. Alternatively, whole-brain approaches, while not depending on an a priori selection of brain regions, have been known to unreliably delimit brain regions compared to ROI techniques (242, 243), and apply inconsistent corrections for multiple comparisons (244).
Methods for DTI tractography have improved (245), EEG and MEG technology has advanced (245), and there is increased use of MRS, MEG and NIRS modalities. The advancement of technology and analytical methods across the past decade adds another layer of variability into reported findings (246–248). That said, if robust differences were to be present between ASD and TD groups at the level of resolution of our current neuroimaging methods, emerging technologies and methods should result in an increase in the signal to noise ratio, with the result of strengthening and supporting earlier findings.
Inconsistency in image processing and acquisition within modalities adds another layer of variability into the reported findings. There is a broad range of methodological choices in this respect which might drive variability in results including the length of scans, any inter-operator variability in data collection, the much-debated use of global signal regression in image preprocessing, the type of artifact removal, the selection of image processing software, and any variability in image processing pipeline. It is nearly impossible to find two neuroimaging studies exactly alike along these domains, and each unique decision made in image collection, preprocessing, and analysis stands to drive variability in results across studies.
Alternatively, the differences in neuroimaging profiles described in this review may reflect actual biological differences in brain structure and function of autistic youth, rather than result from variability in methods. Observations within modalities also uncovered compelling consistencies. Structural imaging findings revealed that autistic participants have larger subcortical gray matter volumes and higher mean and radial diffusivity compared to typically developing peers (65, 70, 94, 96, 99, 102, 104, 108, 113, 133). Functional MRI findings hinted at patterns of lower between-network connectivity in areas related to the amygdala and V1 (114, 121, 134) but increased between-network connectivity in networks related to the imitation network, language regions, paracingulate and thalamus (106, 113, 116, 127, 129, 131). Additionally, autistic youth have higher metabolite markers of excitatory neurotransmission (145–147, 149, 150, 154–157, 159), lower metabolite markers of inhibitory neurotransmission (156, 161, 162, 198), lower markers of overall brain health and metabolism (145, 147, 151–153, 158, 160), decreased EEG-based visual ERP amplitude (218, 227, 228), and both EEG- and MEG-based decrease in auditory ERP/ERF amplitude (188, 202, 208, 210, 211, 232). These findings align with several currently existing theories on the causes of ASD, including the excitation/inhibition theory, which posits that a combination of genetic and environmental variables contribute to increased glutamatergic and/or decreased GABAergic transmission across the brain (35, 36). We also found evidence to support existing theories (249–251) that autistic youth have atypical connectivity patterns, although we were not able to identify consistent patterns of directionality. Our findings further indicated that gross structural changes or activity patterns are not likely to contribute to the neurobiological phenotype of ASD, but rather altered microstructural connectivity is more likely to be part of the phenotype of ASD (252, 253).
These findings might be taken together to motivate investigation into the relationship between genetics, cerebral microstructure, neuronal health, excitation/inhibition, and functional connectivity in autistic youth. Employing multimodal approaches to explore these concepts could provide complementary insights into both structural and functional findings. Additionally, longitudinal multimodal research may clarify whether the structural and functional differences observed in autistic youth are unidirectional or bidirectional. Whether the differences in neuroimaging profiles between autistic and neurotypical participants in this body of research translates to actual differences in neurobiological and behavioral phenotypes across the autism spectrum cannot be easily determined without considering the myriad biological, environmental, stochastic, and confounding factors at play.
Several brain regions appeared numerous times in the literature and displayed consistent differences between autistic and typically developing youth. These regions include the anterior cingulate cortex, insula, prefrontal cortex, amygdala, thalamus, cerebellum, corpus callosum, and default mode network. We found that autistic participants have altered neurochemical expression and functional connectivity in the ACC, which is consistent with post-mortem (254), fMRI (255), and multi-modal imaging (256) research that connects altered ACC cytoarchitecture and signaling with ASD diagnosis, autism symptoms and altered connectivity. Atypical connectivity patterns are also at play in the insula and prefrontal cortex. Impaired insular function could affect reward processing; additionally, abnormal insula activity has been implicated in social deficits related to ASD (257). The medial prefrontal cortex, a key hub in the default mode network, also plays an important role in cognitive functions like working memory, planning, inhibition, and social behavior (258, 259). Structural and functional abnormalities in other prefrontal subregions including the dorsolateral prefrontal cortex (260) and orbitofrontal cortex (261) are often associated with repetitive behaviors and social impairment in autistic individuals. Similarly, altered connectivity between the cortex and subcortical regions like the amygdala and thalamus are closely linked with ASD symptoms. Our findings that amygdala volume and connectivity are altered in autistic participants is consistent with over 20 years of research supporting “the amygdala theory of autism,” (262) which posits that structural and functional amygdala abnormalities explain social deficits in ASD. However, it is unlikely that changes to this region alone account for such a broad array of symptoms and severity (263). In the thalamus, changes in connectivity to other brain regions could affect sensory processing and attention, which likely contribute to core ASD symptoms (264). Cerebellar differences are also likely to affect key ASD features, such as motor activity, language ability, and higher cognitive functions (265). Our findings that autistic youth have structural alterations in the corpus callosum is consistent with research indicating that CC abnormalities are related to ASD symptoms; however, the directionality of these volumetric differences is inconsistent (266). Additional longitudinal research is necessary to tease apart how structural and functional abnormalities develop in autistic youth.
It is important to consider these region-specific findings may not be sensitive nor specific to ASD. The brain regions that we describe are also frequently found in multiple mental health and neurological conditions. Thus, these may reflect more general neurocognitive and social functions that are not necessarily specific to ASD. Autistic youth are at higher risk for internalizing psychopathology (267, 268), sleep problems (269), and attention problems (270) than their neurotypical peers; co-occurring psychopathology in autistic participants could contribute to the heterogeneity of findings. Further, some neuroimaging modalities are more prone to detect signal in certain brain regions: examples include fNIRS, where cap placement and hair interference can influence the accurate measurement in specific brain regions (i.e., the frontal and temporal compared to the parietal and occipital lobes) (271), or MRS, where researchers carefully specify a confined region of interest prior to scanning, often based on clear hypotheses (272, 273).
The converging patterns in neuroimaging profiles based on our literature review may be helpful in fine-tuning existing theories, but it is inappropriate to assume that all autistic youth have similar neuroimaging phenotypes, or that neuroimaging findings are sufficient for making an ASD diagnosis. The heterogeneity in the behavioral phenotype of ASD likely plays a major role in the heterogeneity of the findings within and between studies.
This literature review aimed to provide a birds-eye view of the wealth of neuroimaging research literature investigating neurobiological mechanisms of ASD and to identify consistencies across different modalities. A major strength of this study is that we included seven different imaging modalities and were able to carry out complementing qualitative syntheses of the literature. Region-specific brain maps and bubble plots provided a more quantitative counterpart to the region-specific findings. We expected that the weight of the findings across different neuroimaging modalities would reinforce each other and have some convergence. Our study has a number of limitations that should be carefully considered in the interpretation of our findings. First, our literature review does not follow the validated structure of a systematic or scoping review, and we did not conduct a formal quality assessment on the articles we included in analysis. Still, we followed recommendations for systematic gathering of literature and synthesizing the evidence rigorously. Second, publication bias operates at multiple levels: statistically significant positive and negative (excluding null) findings tend to be reported in the literature itself. Furthermore, in order to organize findings across such a scope of literature, we elected to largely exclude statistically insignificant and null findings. Notably, we applied a qualitative assessment to summarize the evidence. This is in itself not a limitation, but we will note that effect estimates and pooled statistical significance underlying the synthesis of the literature are not reflected in this review.
Finally, reconciling region-of-interest and whole brain approaches in neuroimaging literature is a challenge for the ASD field as well. There is a noteworthy bias with respect to the selection of imaging modality and specific regions-of-interest from which to extract neuroimaging signal. Selection of a neuroimaging modality and regions of interest should always be justified by theoretical assumptions and previous literature. It is a further, more general limitation and source of variability in any neuroimaging research that regions-of-interest, whether established by segmentation or parcellation, cytoarchitectonics, historical neuroanatomy studies, brain atlas or otherwise; these specified regions are not defined consistently across studies.
Our work reveals crucial implications for future research in ASD. The heterogeneity we observed, especially in the MRI-based studies, was substantial enough to motivate a closer look at sources of potentially avoidable variability in research. Such sources include differences in sample selection and demographics, ASD diagnostic criteria, cognitive ability and IQ, comorbid symptoms or diagnoses, image processing, and the inconsistent use of sedation in the MRI scanning of children with ASD.
Aside from these research implications, our work has potential clinical implications. Findings on the variability of structural measures in ASD compared to TD across the brain are relevant to both the relationship between the structural and electrical levels of analysis in the brain, and to the application of the novel therapy Repetitive Transcranial Magnetic Stimulation (rTMS). rTMS is a noninvasive electrical impulse-based therapy with established use in major depression (274, 275), gaining in popularity and clinical approval in other mental health disorders (276). In relation to EEG, and MEG, principles of electrophysics suggest that structural characteristics of underlying brain tissue at the scalp modulate the dynamic electrical field from underlying neuronal activity (277). Targets selected for rTMS would thus depend on structural properties of the brain, because these targets depend on wave properties of the underlying electrical field. Therefore, variable findings on structural differences in ASD suggest that substantial variability exists in the brain structure and electrical activity profiles of ASD, and this variability may be leveraged for personalized targeting in rTMS.
Ongoing work exists in the application of rTMS to reduce the symptomatology of ASD when these symptoms are burdensome or harmful to the individuals living with these symptoms (278, 279). This therapy requires specific brain targets, which most frequently are selected on theory and literature. There is potential for identified regions in this review, particularly those which potentially relate to the neurobiology of ASD across structural, functional, neurochemical domains to inform the selection of targets for rTMS therapies. Still, it is crucial to consider that we have not linked the brain regions in this literature review to any ASD symptomatology. Current ongoing research is exploring the relationship between brain regions and ASD symptomatology at the individual level by precision rTMS neuromodulation.
Future studies may build on our initial review to perform meta-analyses on more targeted questions that allow pooling of the effect estimates, which was not possible within the multimodal scope of this current review. The most rigorous approach would be to obtain raw data from all the studies and perform meta-analyses across modalities.
Furthermore, we did not involve ASD stakeholders in the making of this literature review. ASD and neurodiversity stakeholders in research and wider communities have in past years increasingly called for inclusion of stakeholders in research, to guide the questions that are asked in research and safeguard the needs of autistic and neurodiverse persons in research. We tried to incorporate perspectives from previously published work by autistic scholars and ASD stakeholders as much as possible but recognize that this does not equate the inclusion of original voices. A more comprehensive inclusion of input and commentary from those within the ASD community should be considered an essential element of future research examining the neurobiology of ASD.
It is evident that the approaches to quantify both diagnosis and severity of ASD were not consistent across studies. ADOS and ADI-R serve as the “gold standard” for diagnosis and assessment, tending to produce high levels of inter-rater reliability and agreement with clinician diagnosis (280–282). Nevertheless, it is quite clear that this model is not always followed rigorously, and in many cases not followed at all. In future work a clearer selection of papers based on ASD diagnostic metric and method is needed. Similarly, it is essential to note that we opted to include 15 articles in our primary analysis that did not divide participants into formally diagnosed case/control groups. This was a decision meant to permit a synthesis which captures both participants too young for diagnosis (family history), and a broader spectrum of the behavioral phenotype of ASD, potentially capturing subclinical levels of autism (ASD traits). In future work, a more careful evaluation of ASD diagnostic metric and method, and relative symptom level is required to develop a more sophisticated understanding of the ASD brain phenotype in relationship to the ASD behavioral phenotype. Moreover, future work could investigate the relationship between neuroimaging outcomes and behavioral features such as sensory sensitivity, social behavior, and motor symptoms. We did not address these phenotypic traits in our qualitative analysis, but a deeper understanding of the relationship between brain and behavior would undoubtedly strengthen our understanding of ASD.
The expansion of the investigation to include literature across the lifespan is needed to develop a broader understanding of the ASD brain phenotype. Specifically, within-subject longitudinal research that tracks the neurodevelopment of ASD well beyond our age cutoff of 18 years would be the optimal approach for investigating the neurobiology of ASD across the lifespan. Considering prenatal development by longitudinal or cross-sectional study would also offer insight into a crucially formative developmental phase, which we did not consider in this analysis.
ASD is a neurodevelopmental disorder marked by heterogeneity in its neuroimaging profile: inconsistent results are frequently seen within imaging modalities, comparable study populations and research designs. Despite variability, converging features do emerge, especially when considering brain region, age, imaging modality, and imaging modality metric. Within modalities, consistent differences between the ASD and TD imaging profile were found at both the global and region-specific levels.
Considering the rich neurodiversity in the ASD community, it is not surprising to find rich diversity in the range of neurobiological phenotypes associated with autism. Heterogeneity remains the rule, though multimodal methods show promise for elucidating the complexities associated with ASD phenotypes. In any case, multimodal neuroimaging is positioned to produce interesting and novel discoveries in the coming decade of investigations to advance our collective understanding of ASD, behavioral phenotypes, and neurodevelopment more broadly.
AH: Conceptualization, Data curation, Formal analysis, Investigation, Methodology, Writing – original draft, Writing – review & editing. SV: Data curation, Formal analysis, Investigation, Methodology, Writing – original draft, Writing – review & editing. BG: Conceptualization, Data curation, Formal analysis, Investigation, Methodology, Visualization, Writing – review & editing. ML: Conceptualization, Data curation, Formal analysis, Investigation, Methodology, Writing – review & editing. MM: Conceptualization, Data curation, Formal analysis, Investigation, Methodology, Visualization, Writing – review & editing. LS: Conceptualization, Data curation, Formal analysis, Investigation, Methodology, Visualization, Writing – review & editing. KM: Writing – review & editing. MO: Conceptualization, Investigation, Methodology, Writing – review & editing. SM: Writing – review & editing. SF: Writing – review & editing. LO: Writing – review & editing. TW: Resources, Supervision, Writing – review & editing, Conceptualization, Funding acquisition, Project administration. IV: Conceptualization, Data curation, Investigation, Methodology, Project administration, Supervision, Writing – original draft, Writing – review & editing.
The author(s) declare financial support was received for the research, authorship, and/or publication of this article. AH, SV, BG, ML, MM, LS, KM, MO, TW, and IV are supported by the NIMH Intramural Research Program (ZIAMH002986-01). SM, SF, and LO are supported by the NIMH Intramural Research Program (ZIAMH002955).
This research was carried out with the support of the Intramural Research Program of the NIH, National Institute of Mental Health. The authors would like to thank the participants involved in the surveyed literature, without whose participation this work would not be possible. The authors would also like to give special thanks to colleagues Neda Sadeghi and Marine Bouyssi-Kobar, whose generous feedback was of great use in the preparation of the manuscript, especially with regard to the visual representation of our findings.
The authors declare that the research was conducted in the absence of any commercial or financial relationships that could be construed as a potential conflict of interest.
The author(s) declared that they were an editorial board member of Frontiers, at the time of submission. This had no impact on the peer review process and the final decision.
All claims expressed in this article are solely those of the authors and do not necessarily represent those of their affiliated organizations, or those of the publisher, the editors and the reviewers. Any product that may be evaluated in this article, or claim that may be made by its manufacturer, is not guaranteed or endorsed by the publisher.
The opinions expressed in this article are the authors’ own and do not reflect the views of the National Institutes of Health, the Department of Health and Human Services, or the United States government.
The Supplementary Material for this article can be found online at: https://www.frontiersin.org/articles/10.3389/fpsyt.2024.1474003/full#supplementary-material
1. Interagency Autism Coordinating Committee (IACC). 2021-2023 IACC strategic plan for autism research, services, and policy. (2023). Retrieved from the U.S. Department of Health and Human Services Interagency Autism Coordinating Committee. Available Online at: https://iacc.hhs.gov/publications/strategic-plan/2023/.
2. Maenner MJ, Warren Z, Williams AR, Amoakohene E, Bakian AV, Bilder DA, et al. Prevalence and characteristics of autism spectrum disorder among children aged 8 years — Autism and developmental disabilities monitoring network, 11 sites, United States, 2020. MMWR Surveillance Summaries. (2023) 72:1–14. doi: 10.15585/mmwr.ss7202a1
3. American Psychiatric Association. Diagnostic and statistical manual of mental disorders. 5th ed. (2013). doi: 10.1176/appi.books.9780890425596
4. Wozniak RH, Leezenbaum NB, Northrup JB, West KL, Iverson JM. The development of autism spectrum disorders: variability and causal complexity. Wiley Interdiscip Rev Cognit Sci. (2017) 8. doi: 10.1002/wcs.2017.8.issue-1-2
5. Waizbard-Bartov E, Fein D, Lord C, Amaral DG. Autism severity and its relationship to disability. Autism Res. (2023) 16:685–96. doi: 10.1002/aur.v16.4
6. Hull L, Mandy W, Petrides K. Behavioural and cognitive sex/gender differences in autism spectrum condition and typically developing males and females. Autism. (2017) 21:706–27. doi: 10.1177/1362361316669087
7. Harrison AJ, Long KA, Tommet DC, Jones RN. Examining the role of race, ethnicity, and gender on social and behavioral ratings within the autism diagnostic observation schedule. J Autism Dev Disord. (2017) 47:2770–82. doi: 10.1007/s10803-017-3176-3
8. Tek S, Landa RJ. Differences in autism symptoms between minority and non-minority toddlers. J Autism Dev Disord. (2012) 42:1967–73. doi: 10.1007/s10803-012-1445-8
9. Beggiato A, Peyre H, Maruani A, Scheid I, Rastam M, Amsellem F, et al. Gender differences in autism spectrum disorders: Divergence among specific core symptoms. Autism Res. (2017) 10:680–9. doi: 10.1002/aur.2017.10.issue-4
10. Loomes R, Hull L, Mandy WPL. What is the male-to-female ratio in autism spectrum disorder? A systematic review and meta-analysis. J Am Acad Child Adolesc Psychiatry. (2017) 56:466–74. doi: 10.1016/j.jaac.2017.03.013
11. Salari N, Rasoulpoor S, Rasoulpoor S, Shohaimi S, Jafarpour S, Abdoli N, et al. The global prevalence of autism spectrum disorder: a comprehensive systematic review and meta-analysis. Ital J Pediatr. (2022) 48. doi: 10.1186/s13052-022-01310-w
12. Solmi M, Song M, Yon DK, Lee SW, Fombonne E, Kim MS, et al. Incidence, prevalence, and global burden of autism spectrum disorder from 1990 to 2019 across 204 countries. Mol Psychiatry. (2022) 27:4172–80. doi: 10.1038/s41380-022-01630-7
13. Yoon SH, Choi J, Lee WJ, Do JT. Genetic and epigenetic etiology underlying autism spectrum disorder. J Clin Med. (2020) 9. doi: 10.3390/jcm9040966
14. Lord C, Brugha TS, Charman T, Cusack J, Dumas G, Frazier T, et al. Autism spectrum disorder. Nat Rev Dis Primers. (2020) 6. doi: 10.1038/s41572-019-0138-4
15. Sandin S, Lichtenstein P, Kuja-Halkola R, Hultman C, Larsson H, Reichenberg A. The heritability of autism spectrum disorder. JAMA. (2017) 318:1182. doi: 10.1001/jama.2017.12141
16. Tick B, Bolton P, Happé F, Rutter M, Rijsdijk F. Heritability of autism spectrum disorders: a meta-analysis of twin studies. J Child Psychol Psychiatry. (2016) 57:585–95. doi: 10.1111/jcpp.2016.57.issue-5
17. Warrier V, Zhang X, Reed P, Havdahl A, Moore TM, Cliquet F, et al. Genetic correlates of phenotypic heterogeneity in autism. Nat Genet. (2022) 54:1293–304. doi: 10.1038/s41588-022-01072-5
18. Yeung MK, Bai J, Mak KL. Longitudinal changes in executive function in autism spectrum disorder: A systematic review and meta-analyses. Autism Res. (2024), 1-19. doi: 10.1002/aur.3196
19. Kouklari E-C, Tsermentseli S, Monks CP. Everyday executive function and adaptive skills in children and adolescents with autism spectrum disorder: Cross-sectional developmental trajectories. Autism Dev Lang Impairments. (2018) 3:239694151880077. doi: 10.1177/2396941518800775
20. Galvez-Contreras AY, Zarate-Lopez D, Torres-Chavez AL, Gonzalez-Perez O. Role of oligodendrocytes and myelin in the pathophysiology of autism spectrum disorder. Brain Sci. (2020) 10. doi: 10.3390/brainsci10120951
21. Chapman R. The reality of autism: On the metaphysics of disorder and diversity. Philos Psychol. (2020) 33:799–819. doi: 10.1080/09515089.2020.1751103
22. Kapp SK, Gillespie-Lynch K, Sherman LE, Hutman T. Deficit, difference, or both? Autism and neurodiversity. Dev Psychol. (2013) 49:59–71. doi: 10.1037/a0028353
23. Milton DE. Autistic expertise: a critical reflection on the production of knowledge in autism studies. Autism. (2014) 18:794–802. doi: 10.1177/1362361314525281
24. Kenny L, Hattersley C, Molins B, Buckley C, Povey C, Pellicano E. Which terms should be used to describe autism? Perspectives from the UK autism community. Autism. (2016) 20:442–62. doi: 10.1177/1362361315588200
25. Botha M, Hanlon J, Williams GL. Does language matter? Identity-first versus person-first language use in autism research: A response to Vivanti. J Autism Dev Disord. (2023) 53:870–8. doi: 10.1007/s10803-020-04858-w
26. Bottema-Beutel K, Kapp SK, Lester JN, Sasson NJ, Hand BN. Avoiding ableist language: suggestions for autism researchers. Autism Adulthood. (2021) 3:18–29. doi: 10.1089/aut.2020.0014
27. Bury SM, Jellett R, Spoor JR, Hedley D. It defines who I am" or "It's something I have": what language do [Autistic] Australian adults [on the autism spectrum] prefer? J Autism Dev Disord. (2023) 53:677–87. doi: 10.1007/s10803-020-04425-3
28. Gernsbacher MA. Editorial Perspective: The use of person-first language in scholarly writing may accentuate stigma. J Child Psychol Psychiatry. (2017) 58:859–61. doi: 10.1111/jcpp.2017.58.issue-7
29. Pellicano E, Dinsmore A, Charman T. What should autism research focus upon? Community views and priorities from the United Kingdom. Autism. (2014) 18:756–70. doi: 10.1177/1362361314529627
30. Roche L, Adams D, Clark M. Research priorities of the autism community: A systematic review of key stakeholder perspectives. Autism. (2021) 25:336–48. doi: 10.1177/1362361320967790
31. Cage E, Crompton CJ, Dantas S, Strachan K, Birch R, Robinson M, et al. What are the autism research priorities of autistic adults in Scotland? Autism. (2024) 28(9):2179–90. doi: 10.1177/13623613231222656
32. Baron-Cohen S. Autism: a specific cognitive disorder of “mind-blindness. Int Rev Psychiatry. (1990) 2:81–90. doi: 10.3109/09540269009028274
33. Ozonoff S, Pennington BF, Rogers SJ. Executive function deficits in high-functioning autistic individuals: relationship to theory of mind. J Child Psychol Psychiatry. (1991) 32:1081–105. doi: 10.1111/j.1469-7610.1991.tb00351.x
34. Lovaas IO, Smith T. A comprehensive behavioral theory of autistic children: paradigm for research and treatment. J Behav Ther Exp Psychiat. (1989) 20:17–29. doi: 10.1016/0005-7916(89)90004-9
35. Rubenstein JLR, Merzenich MM. Model of autism: increased ratio of excitation/inhibition in key neural systems. Genes Brain Behavior. (2003) 2:255–67. doi: 10.1034/j.1601-183X.2003.00037.x
36. Uzunova G, Pallanti S, Hollander E. Excitatory/inhibitory imbalance in autism spectrum disorders: Implications for interventions and therapeutics. World J Biol Psychiatry. (2016) 17:174–86. doi: 10.3109/15622975.2015.1085597
37. Bilbo SD, Block CL, Bolton JL, Hanamsagar R, Tran PK. Beyond infection - Maternal immune activation by environmental factors, microglial development, and relevance for autism spectrum disorders. Exp Neurol. (2018) 299:241–51. doi: 10.1016/j.expneurol.2017.07.002
38. Vasa RA, Mostofsky SH, Ewen JB. The disrupted connectivity hypothesis of autism spectrum disorders: time for the next phase in research. Biol Psychiatry: Cogn Neurosci Neuroimaging. (2016) 1:245–52. doi: 10.1016/j.bpsc.2016.02.003
39. Pavăl D. A dopamine hypothesis of autism spectrum disorder. Dev Neurosci. (2017) 39:355–60. doi: 10.1159/000478725
40. Siddiqui MF, Elwell C, Johnson MH. Mitochondrial dysfunction in autism spectrum disorders. Autism Open Access. (2016) 6. doi: 10.4172/2165-7890.1000190
41. Dickinson A, Jones M, Milne E. Measuring neural excitation and inhibition in autism: Different approaches, different findings and different interpretations. Brain Res. (2016) 1648:277–89. doi: 10.1016/j.brainres.2016.07.011
42. Cociu BA, Das S, Billeci L, Jamal W, Maharatna K, Calderoni S, et al. Multimodal functional and structural brain connectivity analysis in autism: A preliminary integrated approach with EEG, fMRI, and DTI. IEEE Trans Cogn Dev Systems. (2018) 10:213–26. doi: 10.1109/TCDS.2017.2680408
43. Libero LE, Deramus TP, Lahti AC, Deshpande G, Kana RK. Multimodal neuroimaging based classification of autism spectrum disorder using anatomical, neurochemical, and white matter correlates. Cortex. (2015) 66:46–59. doi: 10.1016/j.cortex.2015.02.008
44. Mueller S, Keeser D, Samson AC, Kirsch V, Blautzik J, Grothe M, et al. Convergent findings of altered functional and structural brain connectivity in individuals with high functioning autism: A multimodal MRI study. PloS One. (2013) 8:e67329. doi: 10.1371/journal.pone.0067329
45. Oblong LM, Llera A, Mei T, Haak K, Isakoglou C, Floris DL, et al. Linking functional and structural brain organisation with behaviour in autism: a multimodal EU-AIMS Longitudinal European Autism Project (LEAP) study. Mol Autism. (2023) 14. doi: 10.1186/s13229-023-00564-3
46. Mash LE, Reiter MA, Linke AC, Townsend J, Müller RA. Multimodal approaches to functional connectivity in autism spectrum disorders: An integrative perspective. Dev Neurobiol. (2018) 78:456–73. doi: 10.1002/dneu.22570
47. Xu M, Calhoun V, Jiang R, Yan W, Sui J. Brain imaging-based machine learning in autism spectrum disorder: methods and applications. J Neurosci Methods. (2021) 361:109271. doi: 10.1016/j.jneumeth.2021.109271
48. Yerys BE, Herrington JD. Multimodal imaging in autism: an early review of comprehensive neural circuit characterization. Curr Psychiatry Rep. (2014) 16. doi: 10.1007/s11920-014-0496-2
49. Szucs D, Ioannidis JP. Sample size evolution in neuroimaging research: An evaluation of highly-cited studies (1990-2012) and of latest practices (2017-2018) in high-impact journals. Neuroimage. (2020) 221:117164. doi: 10.1016/j.neuroimage.2020.117164
50. Kwak SG, Kim JH. Central limit theorem: the cornerstone of modern statistics. Korean J Anesthesiol. (2017) 70:144. doi: 10.4097/kjae.2017.70.2.144
51. Di Martino A, Yan CG, Li Q, Denio E, Castellanos FX, Alaerts K, et al. The autism brain imaging data exchange: towards a large-scale evaluation of the intrinsic brain architecture in autism. Mol Psychiatry. (2014) 19:659–67. doi: 10.1038/mp.2013.78
52. Eliot L, Beery AK, Jacobs EG, LeBlanc HF, Maney DL, McCarthy MM. Why and how to account for sex and gender in brain and behavioral research. J Neurosci. (2023) 43:6344–56. doi: 10.1523/JNEUROSCI.0020-23.2023
58. Wickham H. The split-apply-combine strategy for data analysis. J Stat Software. (2011) 40:1–29. doi: 10.18637/jss.v040.i01
60. Desikan RS, Segonne F, Fischl B, Quinn BT, Dickerson BC, Blacker D, et al. An automated labeling system for subdividing the human cerebral cortex on MRI scans into gyral based regions of interest. Neuroimage. (2006) 31:968–80. doi: 10.1016/j.neuroimage.2006.01.021
61. Yushkevich PA, Piven J, Hazlett HC, Smith RG, Ho S, Gee JC, et al. User-guided 3D active contour segmentation of anatomical structures: Significantly improved efficiency and reliability. NeuroImage. (2006) 31:1116–28. doi: 10.1016/j.neuroimage.2006.01.015
62. Zhang S, Arfanakis K. Evaluation of standardized and study-specific diffusion tensor imaging templates of the adult human brain: Template characteristics, spatial normalization accuracy, and detection of small inter-group FA differences. Neuroimage. (2018) 172:40–50. doi: 10.1016/j.neuroimage.2018.01.046
63. Qi X, Arfanakis K. Regionconnect: Rapidly extracting standardized brain connectivity information in voxel-wise neuroimaging studies. Neuroimage. (2021) 225:117462. doi: 10.1016/j.neuroimage.2020.117462
64. Xia M, Wang J, He Y. BrainNet viewer: A network visualization tool for human brain connectomics. PLoS One. (2013) 8:e68910. doi: 10.1371/journal.pone.0068910
65. Bai C, Wang Y, Zhang Y, Wang X, Chen Z, Yu W, et al. Abnormal gray matter volume and functional connectivity patterns in social cognition-related brain regions of young children with autism spectrum disorder. Autism Res. (2023) 16:1124–37. doi: 10.1002/aur.v16.6
66. Baribeau DA, Dupuis A, Paton TA, Hammill C, Scherer SW, Schachar RJ, et al. Structural neuroimaging correlates of social deficits are similar in autism spectrum disorder and attention-deficit/hyperactivity disorder: analysis from the POND Network. Transl Psychiatry. (2019) 9:72. doi: 10.1038/s41398-019-0382-0
67. D'Mello AM, Crocetti D, Mostofsky SH, Stoodley CJ. Cerebellar gray matter and lobular volumes correlate with core autism symptoms. NeuroImage Clin. (2015) 7:631–9. doi: 10.1016/j.nicl.2015.02.007
68. Duan X, Wang R, Xiao J, Li Y, Huang X, Guo X, et al. Subcortical structural covariance in young children with autism spectrum disorder. Prog Neuropsychopharmacol Biol Psychiatry. (2020) 99:109874. doi: 10.1016/j.pnpbp.2020.109874
69. Erbetta A, Bulgheroni S, Contarino VE, Chiapparini L, Esposito S, Annunziata S, et al. Low-functioning autism and nonsyndromic intellectual disability: magnetic resonance imaging (MRI) findings. J Child Neurol. (2015) 30:1658–63. doi: 10.1177/0883073815578523
70. Foster NE, Doyle-Thomas KA, Tryfon A, Ouimet T, Anagnostou E, Evans AC, et al. Structural gray matter differences during childhood development in autism spectrum disorder: A multimetric approach. Pediatr Neurol. (2015) 53:350–9. doi: 10.1016/j.pediatrneurol.2015.06.013
71. Giuliano A, Saviozzi I, Brambilla P, Muratori F, Retico A, Calderoni S. The effect of age, sex and clinical features on the volume of Corpus Callosum in pre-schoolers with Autism Spectrum Disorder: a case-control study. Eur J Neurosci. (2018) 47:568–78. doi: 10.1111/ejn.2018.47.issue-6
72. Lee JK, Andrews DS, Ozonoff S, Solomon M, Rogers S, Amaral DG, et al. Longitudinal evaluation of cerebral growth across childhood in boys and girls with autism spectrum disorder. Biol Psychiatry. (2021) 90:286–94. doi: 10.1016/j.biopsych.2020.10.014
73. Lee JK, Andrews DS, Ozturk A, Solomon M, Rogers S, Amaral DG, et al. Altered development of amygdala-connected brain regions in males and females with autism. J Neurosci. (2022) 42:6145–55. doi: 10.1523/JNEUROSCI.0053-22.2022
74. Libero LE, Schaer M, Li DD, Amaral DG, Nordahl CW. A longitudinal study of local gyrification index in young boys with autism spectrum disorder. Cereb Cortex. (2019) 29:2575–87. doi: 10.1093/cercor/bhy126
75. Mensen VT, Wierenga LM, van Dijk S, Rijks Y, Oranje B, Mandl RC, et al. Development of cortical thickness and surface area in autism spectrum disorder. NeuroImage Clin. (2017) 13:215–22. doi: 10.1016/j.nicl.2016.12.003
76. Mizuno Y, Kagitani-Shimono K, Jung M, Makita K, Takiguchi S, Fujisawa TX, et al. Structural brain abnormalities in children and adolescents with comorbid autism spectrum disorder and attention-deficit/hyperactivity disorder. Transl Psychiatry. (2019) 9:332. doi: 10.1038/s41398-019-0679-z
77. Ni HC, Lin HY, Chen YC, Tseng WI, Gau SS. Boys with autism spectrum disorder have distinct cortical folding patterns underpinning impaired self-regulation: a surface-based morphometry study. Brain Imaging Behav. (2020) 14:2464–76. doi: 10.1007/s11682-019-00199-0
78. Ni HC, Lin HY, Tseng WI, Chiu YN, Wu YY, Tsai WC, et al. Neural correlates of impaired self-regulation in male youths with autism spectrum disorder: A voxel-based morphometry study. Prog Neuropsychopharmacol Biol Psychiatry. (2018) 82:233–41. doi: 10.1016/j.pnpbp.2017.11.008
79. Nordahl CW, Iosif AM, Young GS, Hechtman A, Heath B, Lee JK, et al. High psychopathology subgroup in young children with autism: associations with biological sex and amygdala volume. J Am Acad Child Adolesc Psychiatry. (2020) 59:1353–63.e2. doi: 10.1016/j.jaac.2019.11.022
80. Pote I, Wang S, Sethna V, Blasi A, Daly E, Kuklisova-Murgasova M, et al. Familial risk of autism alters subcortical and cerebellar brain anatomy in infants and predicts the emergence of repetitive behaviors in early childhood. Autism Res. (2019) 12:614–27. doi: 10.1002/aur.2019.12.issue-4
81. Reinhardt VP, Iosif AM, Libero L, Heath B, Rogers SJ, Ferrer E, et al. Understanding hippocampal development in young children with autism spectrum disorder. J Am Acad Child Adolesc Psychiatry. (2020) 59:1069–79. doi: 10.1016/j.jaac.2019.08.008
82. Sharda M, Foster NEV, Tryfon A, Doyle-Thomas KAR, Ouimet T, Anagnostou E, et al. Language ability predicts cortical structure and covariance in boys with autism spectrum disorder. Cereb Cortex. (2017) 27:1849–62. doi: 10.1093/cercor/bhw024
83. Sharp TH, Elsabbagh M, Pickles A, Bedford R. The subcortical correlates of autistic traits in school-age children: a population-based neuroimaging study. Mol Autism. (2023) 14:6. doi: 10.1186/s13229-023-00538-5
84. Shen MD, Kim SH, McKinstry RC, Gu H, Hazlett HC, Nordahl CW, et al. Increased extra-axial cerebrospinal fluid in high-risk infants who later develop autism. Biol Psychiatry. (2017) 82:186–93. doi: 10.1016/j.biopsych.2017.02.1095
85. Shen MD, Swanson MR, Wolff JJ, Elison JT, Girault JB, Kim SH, et al. Subcortical brain development in autism and fragile X syndrome: evidence for dynamic, age- and disorder-specific trajectories in infancy. Am J Psychiatry. (2022) 179:562–72. doi: 10.1176/appi.ajp.21090896
86. Shiohama T, Ortug A, Warren JLA, Valli B, Levman J, Faja SK, et al. Small nucleus accumbens and large cerebral ventricles in infants and toddlers prior to receiving diagnoses of autism spectrum disorder. Cereb Cortex. (2022) 32:1200–11. doi: 10.1093/cercor/bhab283
87. Sussman D, Leung RC, Vogan VM, Lee W, Trelle S, Lin S, et al. The autism puzzle: Diffuse but not pervasive neuroanatomical abnormalities in children with ASD. NeuroImage Clin. (2015) 8:170–9. doi: 10.1016/j.nicl.2015.04.008
88. Wolff JJ, Gerig G, Lewis JD, Soda T, Styner MA, Vachet C, et al. Altered corpus callosum morphology associated with autism over the first 2 years of life. Brain. (2015) 138:2046–58. doi: 10.1093/brain/awv118
89. Yang DY, Beam D, Pelphrey KA, Abdullahi S, Jou RJ. Cortical morphological markers in children with autism: a structural magnetic resonance imaging study of thickness, area, volume, and gyrification. Mol Autism. (2016) 7:11. doi: 10.1186/s13229-016-0076-x
90. Zhang Y, Qin B, Wang L, Zhang K, Song C, Chen J, et al. Corpus callosum volumes in children with autism spectrum disorders: sex-associated differences. J Autism Dev Disord. (2023) 53:2421–9. doi: 10.1007/s10803-022-05538-7
91. Andrews DS, Lee JK, Harvey DJ, Waizbard-Bartov E, Solomon M, Rogers SJ, et al. A longitudinal study of white matter development in relation to changes in autism severity across early childhood. Biol Psychiatry. (2021) 89:424–32. doi: 10.1016/j.biopsych.2020.10.013
92. Andrews DS, Lee JK, Solomon M, Rogers SJ, Amaral DG, Nordahl CW. A diffusion-weighted imaging tract-based spatial statistics study of autism spectrum disorder in preschool-aged children. J Neurodev Disord. (2019) 11:32. doi: 10.1186/s11689-019-9291-z
93. Aoki Y, Yoncheva YN, Chen B, Nath T, Sharp D, Lazar M, et al. Association of white matter structure with autism spectrum disorder and attention-deficit/hyperactivity disorder. JAMA Psychiatry. (2017) 74:1120–8. doi: 10.1001/jamapsychiatry.2017.2573
94. Carper RA, Treiber JM, DeJesus SY, Müller RA. Reduced hemispheric asymmetry of white matter microstructure in autism spectrum disorder. J Am Acad Child Adolesc Psychiatry. (2016) 55:1073–80. doi: 10.1016/j.jaac.2016.09.491
95. Duerden EG, Card D, Roberts SW, Mak-Fan KM, Chakravarty MM, Lerch JP, et al. Self-injurious behaviours are associated with alterations in the somatosensory system in children with autism spectrum disorder. Brain Struct Funct. (2014) 219:1251–61. doi: 10.1007/s00429-013-0562-2
96. Hau J, Aljawad S, Baggett N, Fishman I, Carper RA, Müller RA. The cingulum and cingulate U-fibers in children and adolescents with autism spectrum disorders. Hum Brain Mapp. (2019) 40:3153–64. doi: 10.1002/hbm.v40.11
97. Irimia A, Torgerson CM, Jacokes ZJ, Van Horn JD. The connectomes of males and females with autism spectrum disorder have significantly different white matter connectivity densities. Sci Rep. (2017) 7:46401. doi: 10.1038/srep46401
98. Li Y, Fang H, Zheng W, Qian L, Xiao Y, Wu Q, et al. A fiber tractography study of social-emotional related fiber tracts in children and adolescents with autism spectrum disorder. Neurosci Bull. (2017) 33:722–30. doi: 10.1007/s12264-017-0155-9
99. Roberts TP, Heiken K, Zarnow D, Dell J, Nagae L, Blaskey L, et al. Left hemisphere diffusivity of the arcuate fasciculus: influences of autism spectrum disorder and language impairment. AJNR Am J Neuroradiol. (2014) 35:587–92. doi: 10.3174/ajnr.A3754
100. Solders SK, Carper RA, Müller RA. White matter compromise in autism? Differentiating motion confounds from true differences in diffusion tensor imaging. Autism Res. (2017) 10:1606–20. doi: 10.1002/aur.2017.10.issue-10
101. Solso S, Xu R, Proudfoot J, Hagler DJ Jr., Campbell K, Venkatraman V, et al. Diffusion tensor imaging provides evidence of possible axonal overconnectivity in frontal lobes in autism spectrum disorder toddlers. Biol Psychiatry. (2016) 79:676–84. doi: 10.1016/j.biopsych.2015.06.029
102. Xiao Z, Qiu T, Ke X, Xiao X, Xiao T, Liang F, et al. Autism spectrum disorder as early neurodevelopmental disorder: evidence from the brain imaging abnormalities in 2-3 years old toddlers. J Autism Dev Disord. (2014) 44:1633–40. doi: 10.1007/s10803-014-2033-x
103. Yu Q, Peng Y, Kang H, Peng Q, Ouyang M, Slinger M, et al. Differential white matter maturation from birth to 8 years of age. Cereb Cortex. (2020) 30:2673–89. doi: 10.1093/cercor/bhz268
104. Zhang M, Hu X, Jiao J, Yuan D, Li S, Luo T, et al. Brain white matter microstructure abnormalities in children with optimal outcome from autism: a four-year follow-up study. Sci Rep. (2022) 12:20151. doi: 10.1038/s41598-022-21085-8
105. Abbott AE, Nair A, Keown CL, Datko M, Jahedi A, Fishman I, et al. Patterns of atypical functional connectivity and behavioral links in autism differ between default, salience, and executive networks. Cereb Cortex. (2016) 26:4034–45. doi: 10.1093/cercor/bhv191
106. Bos DJ, van Raalten TR, Oranje B, Smits AR, Kobussen NA, Belle J, et al. Developmental differences in higher-order resting-state networks in Autism Spectrum Disorder. NeuroImage Clin. (2014) 4:820–7. doi: 10.1016/j.nicl.2014.05.007
107. Butera C, Kaplan J, Kilroy E, Harrison L, Jayashankar A, Loureiro F, et al. The relationship between alexithymia, interoception, and neural functional connectivity during facial expression processing in autism spectrum disorder. Neuropsychologia. (2023) 180:108469. doi: 10.1016/j.neuropsychologia.2023.108469
108. Carper RA, Solders S, Treiber JM, Fishman I, Müller RA. Corticospinal tract anatomy and functional connectivity of primary motor cortex in autism. J Am Acad Child Adolesc Psychiatry. (2015) 54:859–67. doi: 10.1016/j.jaac.2015.07.007
109. Dajani DR, Burrows CA, Odriozola P, Baez A, Nebel MB, Mostofsky SH, et al. Investigating functional brain network integrity using a traditional and novel categorical scheme for neurodevelopmental disorders. NeuroImage Clin. (2019) 21:101678. doi: 10.1016/j.nicl.2019.101678
110. Di Martino A, Zuo XN, Kelly C, Grzadzinski R, Mennes M, Schvarcz A, et al. Shared and distinct intrinsic functional network centrality in autism and attention-deficit/hyperactivity disorder. Biol Psychiatry. (2013) 74:623–32. doi: 10.1016/j.biopsych.2013.02.011
111. Doyle-Thomas KA, Lee W, Foster NE, Tryfon A, Ouimet T, Hyde KL, et al. Atypical functional brain connectivity during rest in autism spectrum disorders. Ann Neurol. (2015) 77:866–76. doi: 10.1002/ana.24391
112. Fan LY, Booth JR, Liu M, Chou TL, Gau SS. Developmental differences in neural connectivity for semantic processing in youths with autism. J Child Psychol Psychiatry. (2021) 62:1090–9. doi: 10.1111/jcpp.13373
113. Fishman I, Datko M, Cabrera Y, Carper RA, Müller RA. Reduced integration and differentiation of the imitation network in autism: A combined functional connectivity magnetic resonance imaging and diffusion-weighted imaging study. Ann Neurol. (2015) 78:958–69. doi: 10.1002/ana.24533
114. Fishman I, Linke AC, Hau J, Carper RA, Müller RA. Atypical functional connectivity of amygdala related to reduced symptom severity in children with autism. J Am Acad Child Adolesc Psychiatry. (2018) 57:764–74.e3. doi: 10.1016/j.jaac.2018.06.015
115. Floris DL, Barber AD, Nebel MB, Martinelli M, Lai MC, Crocetti D, et al. Atypical lateralization of motor circuit functional connectivity in children with autism is associated with motor deficits. Mol Autism. (2016) 7:35. doi: 10.1186/s13229-016-0096-6
116. Gao Y, Linke A, Jao Keehn RJ, Punyamurthula S, Jahedi A, Gates K, et al. The language network in autism: Atypical functional connectivity with default mode and visual regions. Autism Res. (2019) 12:1344–55. doi: 10.1002/aur.v12.9
117. Gooskens B, Bos DJ, Mensen VT, Shook DA, Bruchhage MMK, Naaijen J, et al. No evidence of differences in cognitive control in children with autism spectrum disorder or obsessive-compulsive disorder: An fMRI study. Dev Cognit Neurosci. (2019) 36:100602. doi: 10.1016/j.dcn.2018.11.004
118. Green SA, Hernandez L, Bookheimer SY, Dapretto M. Salience network connectivity in autism is related to brain and behavioral markers of sensory overresponsivity. J Am Acad Child Adolesc Psychiatry. (2016) 55:618–26.e1. doi: 10.1016/j.jaac.2016.04.013
119. He C, Chen Y, Jian T, Chen H, Guo X, Wang J, et al. Dynamic functional connectivity analysis reveals decreased variability of the default-mode network in developing autistic brain. Autism Res. (2018) 11:1479–93. doi: 10.1002/aur.2018.11.issue-11
120. He C, Cortes JM, Kang X, Cao J, Chen H, Guo X, et al. Individual-based morphological brain network organization and its association with autistic symptoms in young children with autism spectrum disorder. Hum Brain Mapp. (2021) 42:3282–94. doi: 10.1002/hbm.v42.10
121. Jao Keehn RJ, Pueschel EB, Gao Y, Jahedi A, Alemu K, Carper R, et al. Underconnectivity between visual and salience networks and links with sensory abnormalities in autism spectrum disorders. J Am Acad Child Adolesc Psychiatry. (2021) 60:274–85. doi: 10.1016/j.jaac.2020.02.007
122. Jung J, Zbozinek TD, Cummings KK, Wilhelm FH, Dapretto M, Craske MG, et al. Associations between physiological and neural measures of sensory reactivity in youth with autism. J Child Psychol Psychiatry. (2021) 62:1183–94. doi: 10.1111/jcpp.v62.10
123. Keown CL, Shih P, Nair A, Peterson N, Mulvey ME, Müller RA. Local functional overconnectivity in posterior brain regions is associated with symptom severity in autism spectrum disorders. Cell Rep. (2013) 5:567–72. doi: 10.1016/j.celrep.2013.10.003
124. Khan AJ, Nair A, Keown CL, Datko MC, Lincoln AJ, Müller RA. Cerebro-cerebellar resting-state functional connectivity in children and adolescents with autism spectrum disorder. Biol Psychiatry. (2015) 78:625–34. doi: 10.1016/j.biopsych.2015.03.024
125. Kilroy E, Harrison L, Butera C, Jayashankar A, Cermak S, Kaplan J, et al. Unique deficit in embodied simulation in autism: An fMRI study comparing autism and developmental coordination disorder. Hum Brain Mapp. (2021) 42:1532–46. doi: 10.1002/hbm.25312
126. Lawrence KE, Hernandez LM, Bowman HC, Padgaonkar NT, Fuster E, Jack A, et al. Sex differences in functional connectivity of the salience, default mode, and central executive networks in youth with ASD. Cereb Cortex. (2020) 30:5107–20. doi: 10.1093/cercor/bhaa105
127. Lin HY, Ni HC, Tseng WI, Gau SS. Characterizing intrinsic functional connectivity in relation to impaired self-regulation in intellectually able male youth with autism spectrum disorder. Autism. (2020) 24:1201–16. doi: 10.1177/1362361319888104
128. Linke AC, Chen B, Olson L, Ibarra C, Fong C, Reynolds S, et al. Sleep problems in preschoolers with autism spectrum disorder are associated with sensory sensitivities and thalamocortical overconnectivity. Biol Psychiatry Cognit Neurosci Neuroimaging. (2023) 8:21–31. doi: 10.1016/j.bpsc.2021.07.008
129. Linke AC, Jao Keehn RJ, Pueschel EB, Fishman I, Müller RA. Children with ASD show links between aberrant sound processing, social symptoms, and atypical auditory interhemispheric and thalamocortical functional connectivity. Dev Cognit Neurosci. (2018) 29:117–26. doi: 10.1016/j.dcn.2017.01.007
130. Liu J, Okada NJ, Cummings KK, Jung J, Patterson G, Bookheimer SY, et al. Emerging atypicalities in functional connectivity of language-related networks in young infants at high familial risk for ASD. Dev Cognit Neurosci. (2020) 45:100814. doi: 10.1016/j.dcn.2020.100814
131. Mash LE, Linke AC, Olson LA, Fishman I, Liu TT, Müller RA. Transient states of network connectivity are atypical in autism: A dynamic functional connectivity study. Hum Brain Mapp. (2019) 40:2377–89. doi: 10.1002/hbm.24529
132. McNaughton KA, Kirby LA, Warnell KR, Alkire D, Merchant JS, Moraczewski D, et al. Social-interactive reward elicits similar neural response in autism and typical development and predicts future social experiences. Dev Cognit Neurosci. (2023) 59:101197. doi: 10.1016/j.dcn.2023.101197
133. Nair A, Carper RA, Abbott AE, Chen CP, Solders S, Nakutin S, et al. Regional specificity of aberrant thalamocortical connectivity in autism. Hum Brain Mapp. (2015) 36:4497–511. doi: 10.1002/hbm.v36.11
134. Shen MD, Li DD, Keown CL, Lee A, Johnson RT, Angkustsiri K, et al. Functional connectivity of the amygdala is disrupted in preschool-aged children with autism spectrum disorder. J Am Acad Child Adolesc Psychiatry. (2016) 55:817–24. doi: 10.1016/j.jaac.2016.05.020
135. Shi F, Wang L, Peng Z, Wee CY, Shen D. Altered modular organization of structural cortical networks in children with autism. PloS One. (2013) 8:e63131. doi: 10.1371/journal.pone.0063131
136. Solomon M, Yoon JH, Ragland JD, Niendam TA, Lesh TA, Fairbrother W, et al. The development of the neural substrates of cognitive control in adolescents with autism spectrum disorders. Biol Psychiatry. (2014) 76:412–21. doi: 10.1016/j.biopsych.2013.08.036
137. Supekar K, Ryali S, Mistry P, Menon V. Aberrant dynamics of cognitive control and motor circuits predict distinct restricted and repetitive behaviors in children with autism. Nat Commun. (2021) 12:3537. doi: 10.1038/s41467-021-23822-5
138. Vogan VM, Francis KE, Morgan BR, Smith ML, Taylor MJ. Load matters: neural correlates of verbal working memory in children with autism spectrum disorder. J Neurodev Disord. (2018) 10:19. doi: 10.1186/s11689-018-9236-y
139. Wang Y, Hu D, Wu Z, Wang L, Huang W, Li G. Developmental abnormalities of structural covariance networks of cortical thickness and surface area in autistic infants within the first 2 years. Cereb Cortex. (2022) 32:3786–98. doi: 10.1093/cercor/bhab448
140. Xiao Y, Wen TH, Kupis L, Eyler LT, Goel D, Vaux K, et al. Neural responses to affective speech, including motherese, map onto clinical and social eye tracking profiles in toddlers with ASD. Nat Hum Behav. (2022) 6:443–54. doi: 10.1038/s41562-021-01237-y
141. Yerys BE, Herrington JD, Satterthwaite TD, Guy L, Schultz RT, Bassett DS. Globally weaker and topologically different: resting-state connectivity in youth with autism. Mol Autism. (2017) 8:39. doi: 10.1186/s13229-017-0156-6
142. Yerys BE, Tunç B, Satterthwaite TD, Antezana L, Mosner MG, Bertollo JR, et al. Functional connectivity of frontoparietal and salience/ventral attention networks have independent associations with co-occurring attention-deficit/hyperactivity disorder symptoms in children with autism. Biol Psychiatry Cognit Neurosci Neuroimaging. (2019) 4:343–51. doi: 10.1016/j.bpsc.2018.12.012
143. Zhao X, Zhu S, Cao Y, Cheng P, Lin Y, Sun Z, et al. Regional homogeneity of adolescents with high-functioning autism spectrum disorder and its association with symptom severity. Brain Behav. (2022) 12:e2693. doi: 10.1002/brb3.v12.8
144. Zielinski BA, Andrews DS, Lee JK, Solomon M, Rogers SJ, Heath B, et al. Sex-dependent structure of socioemotional salience, executive control, and default mode networks in preschool-aged children with autism. Neuroimage. (2022) 257:119252. doi: 10.1016/j.neuroimage.2022.119252
145. Carvalho Pereira A, Violante IR, Mouga S, Oliveira G, Castelo-Branco M. Medial frontal lobe neurochemistry in autism spectrum disorder is marked by reduced N-acetylaspartate and unchanged gamma-aminobutyric acid and glutamate + Glutamine levels. J Autism Dev Disord. (2018) 48:1467–82. doi: 10.1007/s10803-017-3406-8
146. Cochran DM, Sikoglu EM, Hodge SM, Edden RA, Foley A, Kennedy DN, et al. Relationship among glutamine, γ-aminobutyric acid, and social cognition in autism spectrum disorders. J Child Adolesc Psychopharmacol. (2015) 25:314–22. doi: 10.1089/cap.2014.0112
147. Corrigan NM, Shaw DW, Estes AM, Richards TL, Munson J, Friedman SD, et al. Atypical developmental patterns of brain chemistry in children with autism spectrum disorder. JAMA Psychiatry. (2013) 70:964–74. doi: 10.1001/jamapsychiatry.2013.1388
148. DeMayo MM, Harris AD, Song YJC, Pokorski I, Thapa R, Patel S, et al. Age-related parietal GABA alterations in children with autism spectrum disorder. Autism Res. (2021) 14:859–72. doi: 10.1002/aur.v14.5
149. Doyle-Thomas KA, Card D, Soorya LV, Wang AT, Fan J, Anagnostou E. Metabolic mapping of deep brain structures and associations with symptomatology in autism spectrum disorders. Res Autism Spectr Disord. (2014) 8:44–51. doi: 10.1016/j.rasd.2013.10.003
150. Drenthen GS, Barendse EM, Aldenkamp AP, van Veenendaal TM, Puts NA, Edden RA, et al. Altered neurotransmitter metabolism in adolescents with high-functioning autism. Psychiatry Res Neuroimaging. (2016) 256:44–9. doi: 10.1016/j.pscychresns.2016.09.007
151. Edmondson DA, Xia P, McNally Keehn R, Dydak U, Keehn B. A magnetic resonance spectroscopy study of superior visual search abilities in children with autism spectrum disorder. Autism Res. (2020) 13:550–62. doi: 10.1002/aur.v13.4
152. Goji A, Ito H, Mori K, Harada M, Hisaoka S, Toda Y, et al. Assessment of anterior cingulate cortex (ACC) and left cerebellar metabolism in Asperger's syndrome with proton magnetic resonance spectroscopy (MRS). PloS One. (2017) 12:e0169288. doi: 10.1371/journal.pone.0169288
153. Hardan AY, Fung LK, Frazier T, Berquist SW, Minshew NJ, Keshavan MS, et al. A proton spectroscopy study of white matter in children with autism. Prog Neuropsychopharmacol Biol Psychiatry. (2016) 66:48–53. doi: 10.1016/j.pnpbp.2015.11.005
154. He JL, Oeltzschner G, Mikkelsen M, Deronda A, Harris AD, Crocetti D, et al. Region-specific elevations of glutamate + glutamine correlate with the sensory symptoms of autism spectrum disorders. Transl Psychiatry. (2021) 11:411. doi: 10.1038/s41398-021-01525-1
155. Hollestein V, Buitelaar JK, Brandeis D, Banaschewski T, Kaiser A, Hohmann S, et al. Developmental changes in fronto-striatal glutamate and their association with functioning during inhibitory control in autism spectrum disorder and obsessive compulsive disorder. NeuroImage Clin. (2021) 30:102622. doi: 10.1016/j.nicl.2021.102622
156. Ito H, Mori K, Harada M, Hisaoka S, Toda Y, Mori T, et al. A proton magnetic resonance spectroscopic study in autism spectrum disorder using a 3-tesla clinical magnetic resonance imaging (MRI) system: the anterior cingulate cortex and the left cerebellum. J Child Neurol. (2017) 32:731–9. doi: 10.1177/0883073817702981
157. Joshi G, Biederman J, Wozniak J, Goldin RL, Crowley D, Furtak S, et al. Magnetic resonance spectroscopy study of the glutamatergic system in adolescent males with high-functioning autistic disorder: a pilot study at 4T. Eur Arch Psychiatry Clin Neurosci. (2013) 263:379–84. doi: 10.1007/s00406-012-0369-9
158. Mori K, Toda Y, Ito H, Mori T, Goji A, Fujii E, et al. A proton magnetic resonance spectroscopic study in autism spectrum disorders: amygdala and orbito-frontal cortex. Brain Dev. (2013) 35:139–45. doi: 10.1016/j.braindev.2012.09.016
159. Naaijen J, Zwiers MP, Amiri H, Williams SCR, Durston S, Oranje B, et al. Fronto-striatal glutamate in autism spectrum disorder and obsessive compulsive disorder. Neuropsychopharmacology. (2017) 42:2456–65. doi: 10.1038/npp.2016.260
160. Naaijen J, Zwiers MP, Forde NJ, Williams SC, Durston S, Brandeis D, et al. Striatal structure and its association with N-Acetylaspartate and glutamate in autism spectrum disorder and obsessive compulsive disorder. Eur Neuropsychopharmacol. (2018) 28:118–29. doi: 10.1016/j.euroneuro.2017.11.010
161. Puts NAJ, Wodka EL, Harris AD, Crocetti D, Tommerdahl M, Mostofsky SH, et al. Reduced GABA and altered somatosensory function in children with autism spectrum disorder. Autism Res. (2017) 10:608–19. doi: 10.1002/aur.2017.10.issue-4
162. Rojas DC, Singel D, Steinmetz S, Hepburn S, Brown MS. Decreased left perisylvian GABA concentration in children with autism and unaffected siblings. Neuroimage. (2014) 86:28–34. doi: 10.1016/j.neuroimage.2013.01.045
163. Wood ET, Cummings KK, Jung J, Patterson G, Okada N, Guo J, et al. Sensory over-responsivity is related to GABAergic inhibition in thalamocortical circuits. Transl Psychiatry. (2021) 11:39. doi: 10.1038/s41398-020-01154-0
164. Bhat AN, McDonald NM, Eilbott JE, Pelphrey KA. Exploring cortical activation and connectivity in infants with and without familial risk for autism during naturalistic social interactions: A preliminary study. Infant Behav Dev. (2019) 57:101337. doi: 10.1016/j.infbeh.2019.101337
165. Braukmann R, Lloyd-Fox S, Blasi A, Johnson MH, Bekkering H, Buitelaar JK, et al. Diminished socially selective neural processing in 5-month-old infants at high familial risk of autism. Eur J Neurosci. (2018) 47:720–8. doi: 10.1111/ejn.2018.47.issue-6
166. Edwards LA, Wagner JB, Tager-Flusberg H, Nelson CA. Differences in neural correlates of speech perception in 3 month olds at high and low risk for autism spectrum disorder. J Autism Dev Disord. (2017) 47:3125–38. doi: 10.1007/s10803-017-3222-1
167. Hou S, Liu N, Zou J, Yin X, Liu X, Zhang S, et al. Young children with autism show atypical prefrontal cortical responses to humanoid robots: An fNIRS study. Int J Psychophysiol. (2022) 181:23–32. doi: 10.1016/j.ijpsycho.2022.08.008
168. Iwanaga R, Tanaka G, Nakane H, Honda S, Imamura A, Ozawa H. Usefulness of near-infrared spectroscopy to detect brain dysfunction in children with autism spectrum disorder when inferring the mental state of others. Psychiatry Clin Neurosci. (2013) 67:203–9. doi: 10.1111/pcn.2013.67.issue-4
169. Jia H, Li Y, Yu D. Attenuation of long-range temporal correlations of neuronal oscillations in young children with autism spectrum disorder. NeuroImage Clin. (2018) 20:424–32. doi: 10.1016/j.nicl.2018.08.012
170. Jia H, Li Y, Yu D. Normalized spatial complexity analysis of neural signals. Sci Rep. (2018) 8:7912. doi: 10.1038/s41598-018-26329-0
171. Jung CE, Strother L, Feil-Seifer DJ, Hutsler JJ. Atypical asymmetry for processing human and robot faces in autism revealed by fNIRS. PloS One. (2016) 11:e0158804. doi: 10.1371/journal.pone.0158804
172. Kikuchi M, Yoshimura Y, Shitamichi K, Ueno S, Hiraishi H, Munesue T, et al. Anterior prefrontal hemodynamic connectivity in conscious 3- to 7-year-old children with typical development and autism spectrum disorder. PloS One. (2013) 8:e56087. doi: 10.1371/journal.pone.0056087
173. Li Y, Yu D. Weak network efficiency in young children with Autism Spectrum Disorder: Evidence from a functional near-infrared spectroscopy study. Brain Cogn. (2016) 108:47–55. doi: 10.1016/j.bandc.2016.07.006
174. Lin F, Hu Y, Huang W, Wu X, Sun H, Li J. Resting-state coupling between HbO and Hb measured by fNIRS in autism spectrum disorder. J Biophotonics. (2023) 16:e202200265. doi: 10.1002/jbio.202200265
175. Mori K, Toda Y, Ito H, Mori T, Mori K, Goji A, et al. Neuroimaging in autism spectrum disorders: 1H-MRS and NIRS study. J Med Invest. (2015) 62:29–36. doi: 10.2152/jmi.62.29
176. Pecukonis M, Perdue KL, Wong J, Tager-Flusberg H, Nelson CA. Exploring the relation between brain response to speech at 6-months and language outcomes at 24-months in infants at high and low risk for autism spectrum disorder: A preliminary functional near-infrared spectroscopy study. Dev Cognit Neurosci. (2021) 47:100897. doi: 10.1016/j.dcn.2020.100897
177. Schudlo LC, Anagnostou E, Chau T, Doyle-Thomas K. Investigating sensory response to physical discomfort in children with autism spectrum disorder using near-infrared spectroscopy. PloS One. (2021) 16:e0257029. doi: 10.1371/journal.pone.0257029
178. Sun H, Lin F, Wu X, Zhang T, Li J. Normalized mutual information of fNIRS signals as a measure for accessing typical and atypical brain activity. J Biophotonics. (2023) 16:e202200369. doi: 10.1002/jbio.202200369
179. Yasumura A, Kokubo N, Yamamoto H, Yasumura Y, Nakagawa E, Kaga M, et al. Neurobehavioral and hemodynamic evaluation of Stroop and reverse Stroop interference in children with attention-deficit/hyperactivity disorder. Brain Dev. (2014) 36:97–106. doi: 10.1016/j.braindev.2013.01.005
180. Yeung MK, Lee TL, Chan AS. Right-lateralized frontal activation underlies successful updating of verbal working memory in adolescents with high-functioning autism spectrum disorder. Biol Psychol. (2019) 148:107743. doi: 10.1016/j.biopsycho.2019.107743
181. Yeung MK, Lee TL, Chan AS. Frontal lobe dysfunction underlies the differential word retrieval impairment in adolescents with high-functioning autism. Autism Res. (2019) 12:600–13. doi: 10.1002/aur.2019.12.issue-4
182. Zhang F, Moerman F, Niu H, Warreyn P, Roeyers H. Atypical brain network development of infants at elevated likelihood for autism spectrum disorder during the first year of life. Autism Res. (2022) 15:2223–37. doi: 10.1002/aur.v15.12
183. Zhang T, Huang W, Wu X, Sun W, Lin F, Sun H, et al. Altered complexity in resting-state fNIRS signal in autism: a multiscale entropy approach. Physiol Meas. (2021) 42. doi: 10.1088/1361-6579/ac184d
184. Alho J, Khan S, Mamashli F, Perrachione TK, Losh A, McGuiggan NM, et al. Atypical cortical processing of bottom-up speech binding cues in children with autism spectrum disorders. NeuroImage Clin. (2023) 37:103336. doi: 10.1016/j.nicl.2023.103336
185. Duan F, Watanabe K, Yoshimura Y, Kikuchi M, Minabe Y, Aihara K. Detection of atypical network development patterns in children with autism spectrum disorder using magnetoencephalography. PloS One. (2017) 12:e0184422. doi: 10.1371/journal.pone.0184422
186. Edgar JC, Dipiero M, McBride E, Green HL, Berman J, Ku M, et al. Abnormal maturation of the resting-state peak alpha frequency in children with autism spectrum disorder. Hum Brain Mapp. (2019) 40:3288–98. doi: 10.1002/hbm.v40.11
187. Edgar JC, Franzen RE, McNamee M, Green HL, Shen G, DiPiero M, et al. A comparison of resting-state eyes-closed and dark-room alpha-band activity in children. Psychophysiology. (2023) 60:e14285. doi: 10.1111/psyp.14285
188. Edgar JC, Khan SY, Blaskey L, Chow VY, Rey M, Gaetz W, et al. Neuromagnetic oscillations predict evoked-response latency delays and core language deficits in autism spectrum disorders. J Autism Dev Disord. (2015) 45:395–405. doi: 10.1007/s10803-013-1904-x
189. Green HL, Dipiero M, Koppers S, Berman JI, Bloy L, Liu S, et al. Peak alpha frequency and thalamic structure in children with typical development and autism spectrum disorder. J Autism Dev Disord. (2022) 52:103–12. doi: 10.1007/s10803-021-04926-9
190. Green HL, Shen G, Franzen RE, McNamee M, Berman JI, Mowad TG, et al. Differential maturation of auditory cortex activity in young children with autism and typical development. J Autism Dev Disord. (2023) 53(10):4076–89. doi: 10.1007/s10803-022-05696-8
191. Hiraishi H, Kikuchi M, Yoshimura Y, Kitagawa S, Hasegawa C, Munesue T, et al. Unusual developmental pattern of brain lateralization in young boys with autism spectrum disorder: Power analysis with child-sized magnetoencephalography. Psychiatry Clin Neurosci. (2015) 69:153–60. doi: 10.1111/pcn.2015.69.issue-3
192. Kikuchi M, Shitamichi K, Yoshimura Y, Ueno S, Hiraishi H, Hirosawa T, et al. Altered brain connectivity in 3-to 7-year-old children with autism spectrum disorder. NeuroImage Clin. (2013) 2:394–401. doi: 10.1016/j.nicl.2013.03.003
193. Kikuchi M, Yoshimura Y, Hiraishi H, Munesue T, Hashimoto T, Tsubokawa T, et al. Reduced long-range functional connectivity in young children with autism spectrum disorder. Soc Cognit Affect Neurosci. (2015) 10:248–54. doi: 10.1093/scan/nsu049
194. Manyukhina VO, Prokofyev AO, Galuta IA, Goiaeva DE, Obukhova TS, Schneiderman JF, et al. Globally elevated excitation-inhibition ratio in children with autism spectrum disorder and below-average intelligence. Mol Autism. (2022) 13:20. doi: 10.1186/s13229-022-00498-2
195. Orekhova EV, Manyukhina VO, Galuta IA, Prokofyev AO, Goiaeva DE, Obukhova TS, et al. Gamma oscillations point to the role of primary visual cortex in atypical motion processing in autism. PloS One. (2023) 18:e0281531. doi: 10.1371/journal.pone.0281531
196. Port RG, Dipiero MA, Ku M, Liu S, Blaskey L, Kuschner ES, et al. Children with autism spectrum disorder demonstrate regionally specific altered resting-state phase-amplitude coupling. Brain Connect. (2019) 9:425–36. doi: 10.1089/brain.2018.0653
197. Roberts TP, Lanza MR, Dell J, Qasmieh S, Hines K, Blaskey L, et al. Maturational differences in thalamocortical white matter microstructure and auditory evoked response latencies in autism spectrum disorders. Brain Res. (2013) 1537:79–85. doi: 10.1016/j.brainres.2013.09.011
198. Roberts TPL, Bloy L, Ku M, Blaskey L, Jackel CR, Edgar JC, et al. A multimodal study of the contributions of conduction velocity to the auditory evoked neuromagnetic response: anomalies in autism spectrum disorder. Autism Res. (2020) 13:1730–45. doi: 10.1002/aur.v13.10
199. Stroganova TA, Komarov KS, Sysoeva OV, Goiaeva DE, Obukhova TS, Ovsiannikova TM, et al. Left hemispheric deficit in the sustained neuromagnetic response to periodic click trains in children with ASD. Mol Autism. (2020) 11:100. doi: 10.1186/s13229-020-00408-4
200. Takahashi T, Yoshimura Y, Hiraishi H, Hasegawa C, Munesue T, Higashida H, et al. Enhanced brain signal variability in children with autism spectrum disorder during early childhood. Hum Brain Mapp. (2016) 37:1038–50. doi: 10.1002/hbm.23089
201. Yoshimura Y, Ikeda T, Hasegawa C, An KM, Tanaka S, Yaoi K, et al. Shorter P1m response in children with autism spectrum disorder without intellectual disabilities. Int J Mol Sci. (2021) 22. doi: 10.3390/ijms22052611
202. Yoshimura Y, Kikuchi M, Hayashi N, Hiraishi H, Hasegawa C, Takahashi T, et al. Altered human voice processing in the frontal cortex and a developmental language delay in 3- to 5-year-old children with autism spectrum disorder. Sci Rep. (2017) 7:17116. doi: 10.1038/s41598-017-17058-x
203. Yoshimura Y, Kikuchi M, Hiraishi H, Hasegawa C, Takahashi T, Remijn GB, et al. Atypical development of the central auditory system in young children with Autism spectrum disorder. Autism Res. (2016) 9:1216–26. doi: 10.1002/aur.2016.9.issue-11
204. Askari E, Setarehdan SK, Sheikhani A, Mohammadi MR, Teshnehlab M. Modeling the connections of brain regions in children with autism using cellular neural networks and electroencephalography analysis. Artif Intell Med. (2018) 89:40–50. doi: 10.1016/j.artmed.2018.05.003
205. Bochet A, Sperdin HF, Rihs TA, Kojovic N, Franchini M, Jan RK, et al. Early alterations of large-scale brain networks temporal dynamics in young children with autism. Commun Biol. (2021) 4:968. doi: 10.1038/s42003-021-02494-3
206. Carter Leno V, Pickles A, van Noordt S, Huberty S, Desjardins J, Webb SJ, et al. 12-Month peak alpha frequency is a correlate but not a longitudinal predictor of non-verbal cognitive abilities in infants at low and high risk for autism spectrum disorder. Dev Cognit Neurosci. (2021) 48:100938. doi: 10.1016/j.dcn.2021.100938
207. Clawson A, South M, Baldwin SA, Larson MJ. Electrophysiological endophenotypes and the error-related negativity (ERN) in autism spectrum disorder: A family study. J Autism Dev Disord. (2017) 47:1436–52. doi: 10.1007/s10803-017-3066-8
208. Cotter M, Reisli S, Francisco AA, Wakim KM, Oakes L, Crosse MJ, et al. Neurophysiological measures of auditory sensory processing are associated with adaptive behavior in children with Autism Spectrum Disorder. J Neurodev Disord. (2023) 15:11. doi: 10.1186/s11689-023-09480-2
209. Dickinson A, DiStefano C, Senturk D, Jeste SS. Peak alpha frequency is a neural marker of cognitive function across the autism spectrum. Eur J Neurosci. (2018) 47:643–51. doi: 10.1111/ejn.2018.47.issue-6
210. Donkers FC, Carlson M, Schipul SE, Belger A, Baranek GT. Auditory event-related potentials and associations with sensory patterns in children with autism spectrum disorder, developmental delay, and typical development. Autism. (2020) 24:1093–110. doi: 10.1177/1362361319893196
211. Dwyer P, De Meo-Monteil R, Saron CD, Rivera SM. Effects of age on loudness-dependent auditory ERPs in young autistic and typically-developing children. Neuropsychologia. (2021) 156:107837. doi: 10.1016/j.neuropsychologia.2021.107837
212. Espenhahn S, Godfrey KJ, Kaur S, Ross M, Nath N, Dmitrieva O, et al. Tactile cortical responses and association with tactile reactivity in young children on the autism spectrum. Mol Autism. (2021) 12:26. doi: 10.1186/s13229-021-00435-9
213. Faja S, Clarkson T, Webb SJ. Neural and behavioral suppression of interfering flankers by children with and without autism spectrum disorder. Neuropsychologia. (2016) 93:251–61. doi: 10.1016/j.neuropsychologia.2016.10.017
214. Haartsen R, Charman T, Pasco G, Johnson MH, Jones EJH. Modulation of EEG theta by naturalistic social content is not altered in infants with family history of autism. Sci Rep. (2022) 12:20758. doi: 10.1038/s41598-022-24870-7
215. Han J, Zeng K, Kang J, Tong Z, Cai E, Chen H, et al. Development of brain network in children with autism from early childhood to late childhood. Neuroscience. (2017) 367:134–46. doi: 10.1016/j.neuroscience.2017.10.015
216. Hornung T, Chan WH, Müller RA, Townsend J, Keehn B. Dopaminergic hypo-activity and reduced theta-band power in autism spectrum disorder: A resting-state EEG study. Int J Psychophysiol. (2019) 146:101–6. doi: 10.1016/j.ijpsycho.2019.08.012
217. Keehn B, Vogel-Farley V, Tager-Flusberg H, Nelson CA. Atypical hemispheric specialization for faces in infants at risk for autism spectrum disorder. Autism Res. (2015) 8:187–98. doi: 10.1002/aur.1438
218. Knight EJ, Freedman EG, Myers EJ, Berruti AS, Oakes LA, Cao CZ, et al. Severely attenuated visual feedback processing in children on the autism spectrum. J Neurosci. (2023) 43:2424–38. doi: 10.1523/JNEUROSCI.1192-22.2023
219. Kolesnik A, Begum Ali J, Gliga T, Guiraud J, Charman T, Johnson MH, et al. Increased cortical reactivity to repeated tones at 8 months in infants with later ASD. Transl Psychiatry. (2019) 9:46. doi: 10.1038/s41398-019-0393-x
220. Kozhushko NJ, Nagornova ZV, Evdokimov SA, Shemyakina NV, Ponomarev VA, Tereshchenko EP, et al. Specificity of spontaneous EEG associated with different levels of cognitive and communicative dysfunctions in children. Int J Psychophysiol. (2018) 128:22–30. doi: 10.1016/j.ijpsycho.2018.03.013
221. Liu S, Tang F, Dou H, Zhang W. The relationship between autistic traits and empathy in adolescents: An ERP study. Neurosci Lett. (2023) 802:137173. doi: 10.1016/j.neulet.2023.137173
222. Madsen GF, Bilenberg N, Jepsen JR, Glenthøj B, Cantio C, Oranje B. Normal P50 gating in children with autism, yet attenuated P50 amplitude in the Asperger subcategory. Autism Res. (2015) 8:371–8. doi: 10.1002/aur.2015.8.issue-4
223. Magnuson JR, Iarocci G, Doesburg SM, Moreno S. Increased intra-subject variability of reaction times and single-trial event-related potential components in children with autism spectrum disorder. Autism Res. (2020) 13:221–9. doi: 10.1002/aur.v13.2
224. Manning C, Hassall CD, Hunt LT, Norcia AM, Wagenmakers EJ, Evans NJ, et al. Behavioural and neural indices of perceptual decision-making in autistic children during visual motion tasks. Sci Rep. (2022) 12:6072. doi: 10.1038/s41598-022-09885-4
225. Mash LE, Keehn B, Linke AC, Liu TT, Helm JL, Haist F, et al. Atypical relationships between spontaneous EEG and fMRI activity in autism. Brain Connect. (2020) 10:18–28. doi: 10.1089/brain.2019.0693
226. Neuhaus E, Lowry SJ, Santhosh M, Kresse A, Edwards LA, Keller J, et al. Resting state EEG in youth with ASD: age, sex, and relation to phenotype. J Neurodev Disord. (2021) 13:33. doi: 10.1186/s11689-021-09390-1
227. Norcia AM, Lee A, Meredith WJ, Kohler PJ, Pei F, Ghassan SA, et al. A case-control study of visual, auditory and audio-visual sensory interactions in children with autism spectrum disorder. J Vis. (2021) 21:5. doi: 10.1167/jov.21.4.5
228. Parker TC, Crowley MJ, Naples AJ, Rolison MJ, Wu J, Trapani JA, et al. The N170 event-related potential reflects delayed neural response to faces when visual attention is directed to the eyes in youths with ASD. Autism Res. (2021) 14:1347–56. doi: 10.1002/aur.v14.7
229. Pierce S, Kadlaskar G, Edmondson DA, McNally Keehn R, Dydak U, Keehn B. Associations between sensory processing and electrophysiological and neurochemical measures in children with ASD: an EEG-MRS study. J Neurodev Disord. (2021) 13:5. doi: 10.1186/s11689-020-09351-0
230. Pillai AS, McAuliffe D, Lakshmanan BM, Mostofsky SH, Crone NE, Ewen JB. Altered task-related modulation of long-range connectivity in children with autism. Autism Res. (2018) 11:245–57. doi: 10.1002/aur.2018.11.issue-2
231. Portnova GV, Maslennikova AV. Atypical EEG responses to nonverbal emotionally charged stimuli in children with ASD. Behav Neurol. (2020) 2020:2807946. doi: 10.1155/2020/2807946
232. Vlaskamp C, Oranje B, Madsen GF, Møllegaard Jepsen JR, Durston S, Cantio C, et al. Auditory processing in autism spectrum disorder: Mismatch negativity deficits. Autism Res. (2017) 10:1857–65. doi: 10.1002/aur.2017.10.issue-11
233. Wakim KM, Foxe JJ, Molholm S. Cued motor processing in autism and typical development: A high-density electrical mapping study of response-locked neural activity in children and adolescents. Eur J Neurosci. (2023) 58:2766–86. doi: 10.1111/ejn.16063
234. Yeung MK, Han YMY, Sze SL, Chan AS. Abnormal frontal theta oscillations underlie the cognitive flexibility deficits in children with high-functioning autism spectrum disorders. Neuropsychology. (2016) 30:281–95. doi: 10.1037/neu0000231
235. Zhang Y, Zhang S, Chen B, Jiang L, Li Y, Dong L, et al. Predicting the symptom severity in autism spectrum disorder based on EEG metrics. IEEE Trans Neural Syst Rehabil Eng. (2022) 30:1898–907. doi: 10.1109/TNSRE.2022.3188564
236. Mottron L, Bzdok D. Autism spectrum heterogeneity: fact or artifact? Mol Psychiatry. (2020) 25:3178–85. doi: 10.1038/s41380-020-0748-y
237. Martinez-Murcia FJ, Lai MC, Górriz JM, Ramírez J, Young AM, Deoni SC, et al. On the brain structure heterogeneity of autism: Parsing out acquisition site effects with significance-weighted principal component analysis. Hum Brain Mapp. (2017) 38:1208–23. doi: 10.1002/hbm.23449
238. Wolfers T, Floris DL, Dinga R, Van Rooij D, Isakoglou C, Kia SM, et al. From pattern classification to stratification: towards conceptualizing the heterogeneity of Autism Spectrum Disorder. Neurosci Biobehav Rev. (2019) 104:240–54. doi: 10.1016/j.neubiorev.2019.07.010
239. Hyseni F, Blanken LME, Muetzel R, Verhulst FC, Tiemeier H, White T. Autistic traits and neuropsychological performance in 6- to-10-year-old children: a population-based study. Child Neuropsychol. (2019) 25:352–69. doi: 10.1080/09297049.2018.1465543
240. Radua J, Mataix-Cols D. Meta-analytic methods for neuroimaging data explained. Biol Mood Anxiety Disord. (2012) 2:6. doi: 10.1186/2045-5380-2-6
241. Ioannidis JP. Excess significance bias in the literature on brain volume abnormalities. Arch Gen Psychiatry. (2011) 68:773–80. doi: 10.1001/archgenpsychiatry.2011.28
242. Uchida RR, Del-Ben CM, Araújo D, Busatto-Filho G, Duran FLS, Crippa JAS, et al. Correlation between voxel based morphometry and manual volumetry in magnetic resonance images of the human brain. Anais da Academia Bras Ciências. (2008) 80:149–56. doi: 10.1590/S0001-37652008000100010
243. Bergouignan L, Chupin M, Czechowska Y, Kinkingnehun S, Lemogne C, Le Bastard G, et al. Can voxel based morphometry, manual segmentation and automated segmentation equally detect hippocampal volume differences in acute depression? Neuroimage. (2009) 45:29–37. doi: 10.1016/j.neuroimage.2008.11.006
244. Radua J, Mataix-Cols D, Phillips ML, El-Hage W, Kronhaus DM, Cardoner N, et al. A new meta-analytic method for neuroimaging studies that combines reported peak coordinates and statistical parametric maps. Eur Psychiatry. (2012) 27:605–11. doi: 10.1016/j.eurpsy.2011.04.001
245. Niso G, Krol LR, Combrisson E, Dubarry AS, Elliott MA, Francois C, et al. Good scientific practice in EEG and MEG research: Progress and perspectives. Neuroimage. (2022) 257:119056. doi: 10.1016/j.neuroimage.2022.119056
246. Coalson TS, Van Essen DC, Glasser MF. The impact of traditional neuroimaging methods on the spatial localization of cortical areas. Proc Natl Acad Sci. (2018) 115:E6356–E65. doi: 10.1073/pnas.1801582115
247. Yen C, Lin C-L, Chiang M-C. Exploring the frontiers of neuroimaging: A review of recent advances in understanding brain functioning and disorders. Life. (2023) 13:1472. doi: 10.3390/life13071472
248. Azhari A, Truzzi A, Neoh MJ-Y, Balagtas JPM, Tan HH, Goh PP, et al. A decade of infant neuroimaging research: What have we learned and where are we going? Infant Behav Dev. (2020) 58:101389. doi: 10.1016/j.infbeh.2019.101389
249. Müller R-A, Shih P, Keehn B, Deyoe JR, Leyden KM, Shukla DK. Underconnected, but how? A survey of functional connectivity MRI studies in autism spectrum disorders. Cereb Cortex. (2011) 21:2233–43. doi: 10.1093/cercor/bhq296
250. Ilioska I, Oldehinkel M, Llera A, Chopra S, Looden T, Chauvin R, et al. Connectome-wide mega-analysis reveals robust patterns of atypical functional connectivity in autism. Biol Psychiatry. (2023) 94:29–39. doi: 10.1016/j.biopsych.2022.12.018
251. Liloia D, Manuello J, Costa T, Keller R, Nani A, Cauda F. Atypical local brain connectivity in pediatric autism spectrum disorder? A coordinate-based meta-analysis of regional homogeneity studies. Eur Arch Psychiatry Clin Neurosci. (2024) 274:3–18. doi: 10.1007/s00406-022-01541-2
252. Hashem S, Nisar S, Bhat AA, Yadav SK, Azeem MW, Bagga P, et al. Genetics of structural and functional brain changes in autism spectrum disorder. Trans Psychiatry. (2020) 10. doi: 10.1038/s41398-020-00921-3
253. Rafiee F, Rezvani Habibabadi R, Motaghi M, Yousem DM, Yousem IJ. Brain MRI in autism spectrum disorder: narrative review and recent advances. J Magnetic Resonance Imaging. (2022) 55:1613–24. doi: 10.1002/jmri.27949
254. Zikopoulos B, Liu X, Tepe J, Trutzer I, John YJ, Barbas H. Opposite development of short- and long-range anterior cingulate pathways in autism. Acta Neuropathol. (2018) 136:759–78. doi: 10.1007/s00401-018-1904-1
255. Zhou Y, Shi L, Cui X, Wang S, Luo X. Functional connectivity of the caudal anterior cingulate cortex is decreased in autism. PloS One. (2016) 11:e0151879. doi: 10.1371/journal.pone.0151879
256. Forde NJ, Naaijen J, Lythgoe DJ, Akkermans SEA, Openneer TJC, Dietrich A, et al. Multi-modal imaging investigation of anterior cingulate cortex cytoarchitecture in neurodevelopment. Eur Neuropsychopharmacol. (2018) 28:13–23. doi: 10.1016/j.euroneuro.2017.11.021
257. Nomi JS, Molnar-Szakacs I, Uddin LQ. Insular function in autism: Update and future directions in neuroimaging and interventions. Prog Neuropsychopharmacol Biol Psychiatry. (2019) 89:412–26. doi: 10.1016/j.pnpbp.2018.10.015
258. Friedman NP, Robbins TW. The role of prefrontal cortex in cognitive control and executive function. Neuropsychopharmacology. (2022) 47:72–89. doi: 10.1038/s41386-021-01132-0
259. Martíez-Sanchis S. Neurobiological foundations of multisensory integration in people with autism spectrum disorders: the role of the medial prefrontal cortex. Front Hum Neurosci. (2014) 8. doi: 10.3389/fnhum.2014.00970
260. Fatemi SH, Eschenlauer A, Aman J, Folsom TD, Chekouo T. Quantitative proteomics of dorsolateral prefrontal cortex reveals an early pattern of synaptic dysmaturation in children with idiopathic autism. Cereb Cortex. (2024) 34:161–71. doi: 10.1093/cercor/bhae044
261. Bachevalier J, Loveland KA. The orbitofrontal-amygdala circuit and self-regulation of social-emotional behavior in autism. Neurosci Biobehav Rev. (2006) 30:97–117. doi: 10.1016/j.neubiorev.2005.07.002
262. Baron-Cohen S, Ring HA, Bullmore ET, Wheelwright S, Ashwin C, Williams SCR. The amygdala theory of autism. Neurosci Behav Rev. (2000) 24:355–64. doi: 10.1016/S0149-7634(00)00011-7
263. Wang S, Li X. A revisit of the amygdala theory of autism: Twenty years after. Neuropsychologia. (2023) 183:108519. doi: 10.1016/j.neuropsychologia.2023.108519
264. Roy DS, Zhang Y, Halassa MM, Feng G. Thalamic subnetworks as units of function. Nat Neurosci. (2022) 25:140–53. doi: 10.1038/s41593-021-00996-1
265. Hampson DR, Blatt GJ. Autism spectrum disorders and neuropathology of the cerebellum. Front Neurosci. (2015) 9:420. doi: 10.3389/fnins.2015.00420
266. Valenti M, Pino MC, Mazza M, Panzarino G, Di Paolantonio C, Verrotti A. Abnormal structural and functional connectivity of the corpus callosum in autism spectrum disorders: a review. Rev J Autism Dev Disord. (2020) 7:46–62. doi: 10.1007/s40489-019-00176-9
267. Van Steensel FJA, Bögels SM, Perrin S. Anxiety disorders in children and adolescents with autistic spectrum disorders: A meta-analysis. Clin Child Family Psychol Review. (2011) 14:302–17. doi: 10.1007/s10567-011-0097-0
268. Hudson CC, Hall L, Harkness KL. Prevalence of depressive disorders in individuals with autism spectrum disorder: a meta-analysis. J Abnormal Child Psychol. (2019) 47:165–75. doi: 10.1007/s10802-018-0402-1
269. Humphreys JS, Gringras P, Blair PS, Scott N, Henderson J, Fleming PJ, et al. Sleep patterns in children with autistic spectrum disorders: a prospective cohort study. Arch Dis Child. (2014) 99:114–8. doi: 10.1136/archdischild-2013-304083
270. Antshel KM, Zhang-James Y, Wagner KE, Ledesma A, Faraone SV. An update on the comorbidity of ADHD and ASD: a focus on clinical management. Expert Rev Neurotherapeutics. (2016) 16:279–93. doi: 10.1586/14737175.2016.1146591
271. Klein F. Optimizing spatial specificity and signal quality in fNIRS: an overview of potential challenges and possible options for improving the reliability of real-time applications. Front Neuroergon. (2024) 5:1286586. doi: 10.3389/fnrgo.2024.1286586
272. Buonocore MH, Maddock RJ. Magnetic resonance spectroscopy of the brain: a review of physical principles and technical methods. Rev Neurosci. (2015) 26:609–32. doi: 10.1515/revneuro-2015-0010
273. Wilson M, Andronesi O, Barker PB, Bartha R, Bizzi A, Bolan PJ, et al. Methodological consensus on clinical proton MRS of the brain: Review and recommendations. Magnetic Resonance Med. (2019) 82:527–50. doi: 10.1002/mrm.27742
274. Dell’Osso B, Camuri G, Castellano F, Vecchi V, Benedetti M, Bortolussi S, et al. Meta-review of metanalytic studies with repetitive transcranial magnetic stimulation (rTMS) for the treatment of major depression. Clin Pract Epidemiol Ment Health. (2011) 7:167–77. doi: 10.2174/1745017901107010167
275. Berlim MT, Van Den Eynde F, Daskalakis ZJ. High-frequency repetitive transcranial magnetic stimulation accelerates and enhances the clinical response to antidepressants in major depression. J Clin Psychiatry. (2013) 74:e122–e9. doi: 10.4088/JCP.12r07996
276. Wieczorek T, Kobylko A, Stramecki F, Fila-Witecka K, Beszlej J, Jakubczyk M, et al. Transcranial magnetic stimulation (TMS) in treatment of psychiatric disorders - review of current studies. Psychiatr Pol. (2021) 55:565–83. doi: 10.12740/PP/OnlineFirst/115556
277. Nunez PL, Srinivasan R. Electric fields of the brain: the neurophysics of EEG. 2nd ed. (2006) (New York: Oxford Academic). doi: 10.1093/acprof:oso/9780195050387.001.0001
278. Oberman LM, Enticott PG, Casanova MF, Rotenberg A, Pascual-Leone A, McCracken JT. Transcranial magnetic stimulation in autism spectrum disorder: Challenges, promise, and roadmap for future research. Autism Res. (2016) 9:184–203. doi: 10.1002/aur.2016.9.issue-2
279. Barahona-Corrêa JB, Velosa A, Chainho A, Lopes R, Oliveira-Maia AJ. Repetitive transcranial magnetic stimulation for treatment of autism spectrum disorder: A systematic review and meta-analysis. Front Integr Neurosci. (2018) 12. doi: 10.3389/fnint.2018.00027
280. Lord C, Leventhal BL, Cook EH. Quantifying the phenotype in autism spectrum disorders. Am J Med Genet. (2001) 105:36–8. doi: 10.1002/1096-8628(20010108)105:1<36::AID-AJMG1053>3.0.CO;2-4
281. Lefort-Besnard J, Vogeley K, Schilbach L, Varoquaux G, Thirion B, Dumas G, et al. Patterns of autism symptoms: hidden structure in the ADOS and ADI-R instruments. Transl Psychiatry. (2020) 10:257. doi: 10.1038/s41398-020-00946-8
Keywords: autism spectrum disorder, neuroimaging, magnetic resonance imaging, diffusion tensor imaging, magnetic resonance spectroscopy, magnetoencephalography, electroencephalography, near infrared spectroscopy
Citation: Halliday AR, Vucic SN, Georges B, LaRoche M, Mendoza Pardo MA, Swiggard LO, McDonald K, Olofsson M, Menon SN, Francis SM, Oberman LM, White T and van der Velpen IF (2024) Heterogeneity and convergence across seven neuroimaging modalities: a review of the autism spectrum disorder literature. Front. Psychiatry 15:1474003. doi: 10.3389/fpsyt.2024.1474003
Received: 31 July 2024; Accepted: 30 September 2024;
Published: 16 October 2024.
Edited by:
Hsiang-Yuan Lin, University of Toronto, CanadaReviewed by:
Iciar Iturmendi, University of Toronto, CanadaCopyright © 2024 Halliday, Vucic, Georges, LaRoche, Mendoza Pardo, Swiggard, McDonald, Olofsson, Menon, Francis, Oberman, White and van der Velpen. This is an open-access article distributed under the terms of the Creative Commons Attribution License (CC BY). The use, distribution or reproduction in other forums is permitted, provided the original author(s) and the copyright owner(s) are credited and that the original publication in this journal is cited, in accordance with accepted academic practice. No use, distribution or reproduction is permitted which does not comply with these terms.
*Correspondence: Isabelle F. van der Velpen, aXNhYmVsbGUudmFuZGVydmVscGVuQG5paC5nb3Y=
†These authors have contributed equally to this work and share first authorship
‡These authors have contributed equally to this work and share last authorship
Disclaimer: All claims expressed in this article are solely those of the authors and do not necessarily represent those of their affiliated organizations, or those of the publisher, the editors and the reviewers. Any product that may be evaluated in this article or claim that may be made by its manufacturer is not guaranteed or endorsed by the publisher.
Research integrity at Frontiers
Learn more about the work of our research integrity team to safeguard the quality of each article we publish.