- 1Hepatobiliary Pancreatic Surgery, Banan Hospital of Chongqing Medical University, Chongqing, China
- 2Xiangya School of Medicine, Central South University, Changsha, China
Purpose: This study investigates the association between heavy metal exposure and the prevalence of depression, with the mediating role of sleep duration.
Method: Our study investigated the association between heavy metal concentration and depression risk in the NHANES from 2005 to 2020. We used logistic regression analysis, WQS regression model and BKMR model to assess the association. Mediation analysis was performed to explore the role of sleep duration in heavy metal exposure-induced depression.
Result: Of the 10,825 individuals included in this study, 705 (6.85%) were diagnosed with depression. We found a positive association between the cadmium (Cd), cobalt (Co), lead (Pb), antimony (Sb), wolfram (W) concentration and the prevalence of depression. Moreover, heavy metals mixtures were positively correlated with the prevalence of depression, with Cd identified as the main contributor in the WQS regression model (0.4654). Furthermore, sleep duration mediated the association between Cd, molybdenum (Mo), Pb, Sb, W exposure and prevalence of depression, explaining 3.12%, 15.84%, 18.24%, 9.56%, 3.68% of the effect.
Conclusion: The findings affirm that heavy metal exposure is linked to higher depression incidence and that this relationship is partly mediated by sleep duration alterations. The study underscores the importance of environmental health monitoring and interventions aimed at reducing heavy metal exposure to mitigate its psychological impacts.
1 Introduction
Depression, a complex mental disorder, involves persistent sadness and disinterest, affecting daily functioning and increasing the risk of chronic diseases and economic losses (1). It, projected to become the leading global health burden by 2030 (2), affects millions and significantly impacts mortality in specific groups (3) while straining health budgets (4). Given its escalating prevalence, understanding its etiology is crucial (5). Research indicates a potential link between depression and exposure to heavy metals, which can enter the body through various routes and accumulate in organs, leading to damage in the endocrine, cardiovascular, and nervous systems at high levels (6–8). These metals may also induce oxidative stress, affecting neurotransmitter activity and neuron health, ultimately impacting the central nervous system (9). A cohort study confirmed that urinary antimony was positively related to depressive symptoms, especially in female (10). However, there is no study focusing on the association between heavy metal mixture and depression.
Additionally, there is a well-established connection between sleep duration and depression risk, with both insufficient and excessive sleep linked to greater health issues (11, 12). Specific heavy metals, like manganese, are associated with sleep disturbances. Manganese accumulation in the brain’s basal ganglia can disrupt neuronal activity and alter sleep patterns, as demonstrated by changes in sleep stages in animal studies related to manganese toxicity (13, 14). Notably, individuals with obstructive sleep apnea have been observed to have higher manganese levels than healthy controls (15).
Despite the recognized impact of heavy metals on depression, research exploring the simultaneous exposure to various heavy metals and its effects on depression remains sparse. Moreover, while the relationship between sleep duration and depression is understood, systematic studies examining how sleep patterns mediate the relationship between heavy metal exposure and the development of depression are lacking. This study aims to explore these relationships using data from a large-scale cross-sectional study drawn from national health and nutrition examination survey (NHANES).
2 Methodology
2.1 Study population
The National Health and Nutrition Examination Survey (NHANES), managed by the CDC’s National Center for Health Statistics, systematically collects health and nutrition data from U.S. civilians using a stratified, four-stage sampling process. Data are gathered every two years, segmented by demographic factors like age, sex, race/ethnicity, and socioeconomic status. After obtaining informed consent, participants complete questionnaires at home and undergo standardized exams at mobile centers. The NHANES database provides free access to anonymized health data. For our study, we analyzed data from eight cycles covering 2005-2020, ensuring a diverse sample across various U.S. regions.
2.2 Research design
Informed consent was obtained from all participants. NCHS Ethics Review Board (ERB) Approval Protocol #2011-17 and Protocol #2005-06.
Our initial sample comprised 14,367 participants with complete NHANES data from 2004 to 2018. Due to missing information, 4,082 were excluded, leaving 10,285 individuals for the final analysis (Figure 1).
2.3 Measurements
For heavy metals, the method involves using mass spectrometry with an inductively coupled plasma (ICP) ionization source to analyze various metals in urine. Samples are diluted, nebulized into droplets, and carried by argon to the ICP. Ions are directed through a focusing area, a dynamic reaction cell (DRC), and a quadrupole mass filter, allowing for sequential detection and isotope identification of each element (16, 17). All data below the detection limit were included in the study of this paper.
As for the recognition of the depression symptoms, The Patient Health Questionnaire (PHQ-9), consisting of nine items, assessed depression by measuring symptom frequency over the past two weeks, plus an extra question evaluating the symptoms’ overall effect. Responses ranged from “not at all” to “nearly every day,” scored from 0 to 3 (18–20). A PHQ-9 score of 10 or below indicated no depression symptoms, while scores above 10 suggested depression symptoms (18).
As for the measurement of sleep duration, sleep duration was measured by the question “How much sleep do you get (hours)?” (2005–2016 cycle) or the question “Number of hours usually sleep on weekdays or workdays.” (2017–2020 cycle)
2.4 Statistical analysis
We conducted statistical analysis on depression-related characteristics using Kruskal-Wallis and Fisher’s exact tests for continuous and categorical variables, respectively. Heavy metal concentrations were normalized via log-transformation, and correlations were analyzed using Pearson’s correlation. Logistic regression assessed the impact of heavy metals on depression, with adjustments for demographics and health behaviors.
We applied Weighted Quantile Sum (WQS) regression to study both individual and combined effects of metal mixtures, followed by Bayesian Kernel Machine Regression (BKMR) to examine comprehensive impacts at various exposure levels. Relationships between heavy metal exposure and sleep duration were also explored through linear regression and mediation analysis, employing nonparametric bootstrapping.
Our study incorporated various clinically relevant covariates based on previous findings (21, 22), including age, gender, race/ethnicity, educational level, marital status, family poverty income ratio (PIR), alcohol use, smoking status, diabetes, and hypertension.
NHANES categorizes race/ethnicity into groups such as Mexican American, other Hispanic, non-Hispanic white, non-Hispanic black, and other races (including non-Hispanic Asians and multi-racial individuals). Education is classified from below ninth grade to college graduate or higher, and marital status ranges from married to undisclosed. PIR evaluates annual income relative to poverty thresholds by family size.
Alcohol consumption was determined by annual drinking frequency from 2005 to 2016 and lifetime usage from 2017 to 2020. Smoking was assessed by a history of smoking 100 cigarettes or more. Diabetes and hypertension were self-reported and verified by healthcare providers.
All analyses were conducted in R software, considering results with P < 0.05 as statistically significant (23, 24).
3 Results
3.1 Baseline data characteristics
Table 1 illustrates the demographics of the 10,285 participants from the NHANES 2005–2020 dataset. Of these, 705 individuals (percentage: 6.85%) were identified with depression. Significant differences in gender, education, marital status, PIR, diabetes mellitus, hypertension, and smoking habits were observed between those with and without depression (all P < 0.05).
Supplementary Figure 1 displayed Pearson’s correlation coefficients for the heavy metals’ concentrations. Correlation coefficients for Cs and Tl, Mo and W, and Cs and Mo were notably high (value of correlation: 0.77, 0.60, and 0.60), respectively, whereas correlations among other heavy metals generally ranged between 0.40 and 0.60.
3.2 Association between heavy metals and depression
In the logistic regression analyses shown in Table 2, significant associations were observed between depression and several heavy metals: Cd (OR (95%CI) = 5.0711 (3.2718 ~ 7.8600)), Co (OR (95%CI) = 4.1443 (2.0951 ~ 8.1978)), Pb (OR (95%CI) = 2.1941 (1.1460 ~ 4.2007)), Sb (OR (95%CI) = 4.0430 (2.4264 ~ 6.7365)), Tl (OR (95%CI) = 0.5245 (0.2675 ~ 1.0284)), and W (OR (95%CI) = 4.4202 (2.5021 ~ 7.8088)) (OR, odd ratio). However, the significant effects of Pb and Co were diminished after controlling for confounders (P > 0.05).
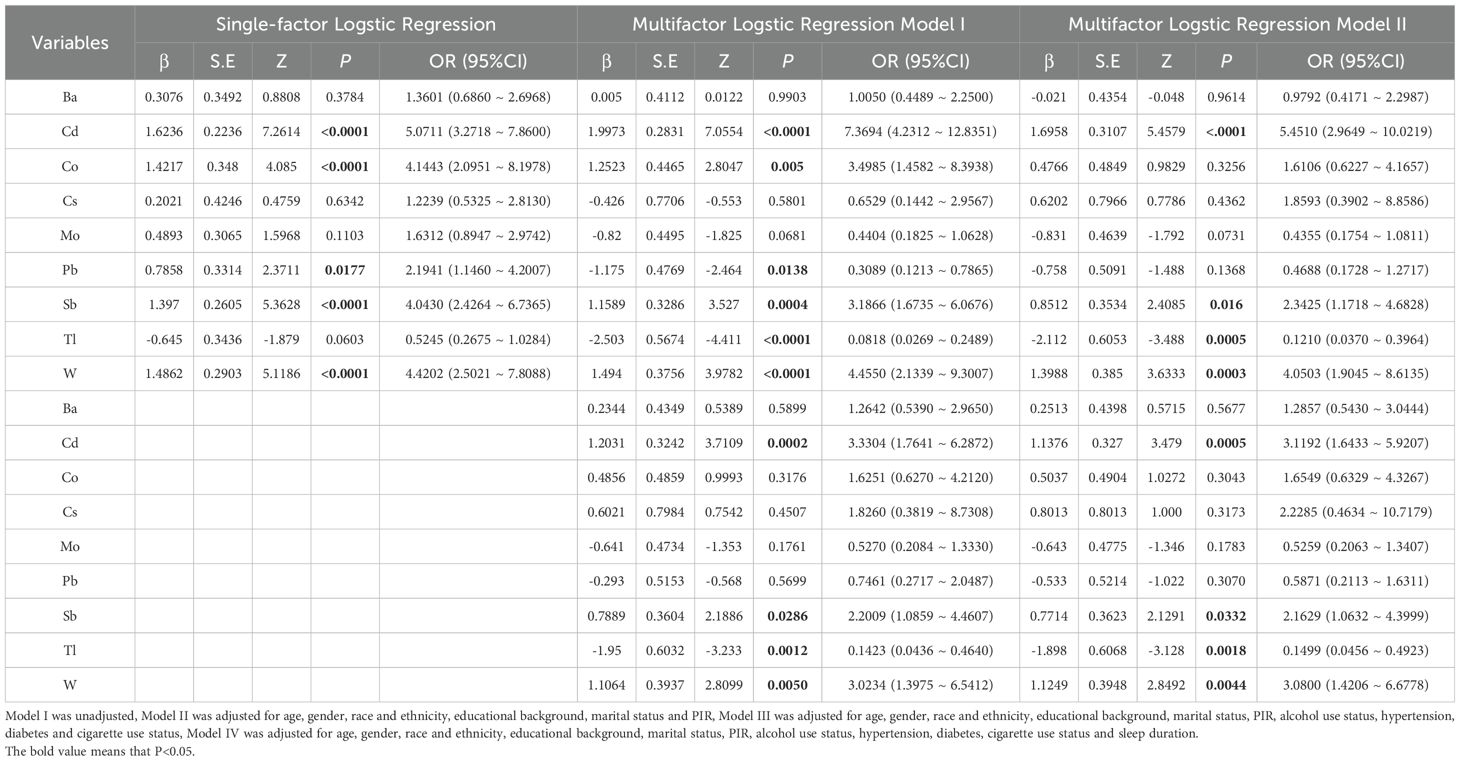
Table 2. Results of a single and multiple logistic regression analysis of the correlation between heavy metal exposure and the prevalence of depression.
The restricted cubic splines model (RCS) is a nonlinear regression model that uses segmented cubic polynomials to fit the data. RCS depicted an increase in depression prevalence with higher exposure to Ba, Cd, Co, Mo, and W (Figures 2A–C, E, I). Conversely, an inverted U-shaped relationship was noted between depression prevalence and Cs, Pb, Sb (Figures 2D, F, G). Tl showed a negative correlation with depression prevalence that persisted even after adjustment (Figure 2H; Supplementary Figure 2).
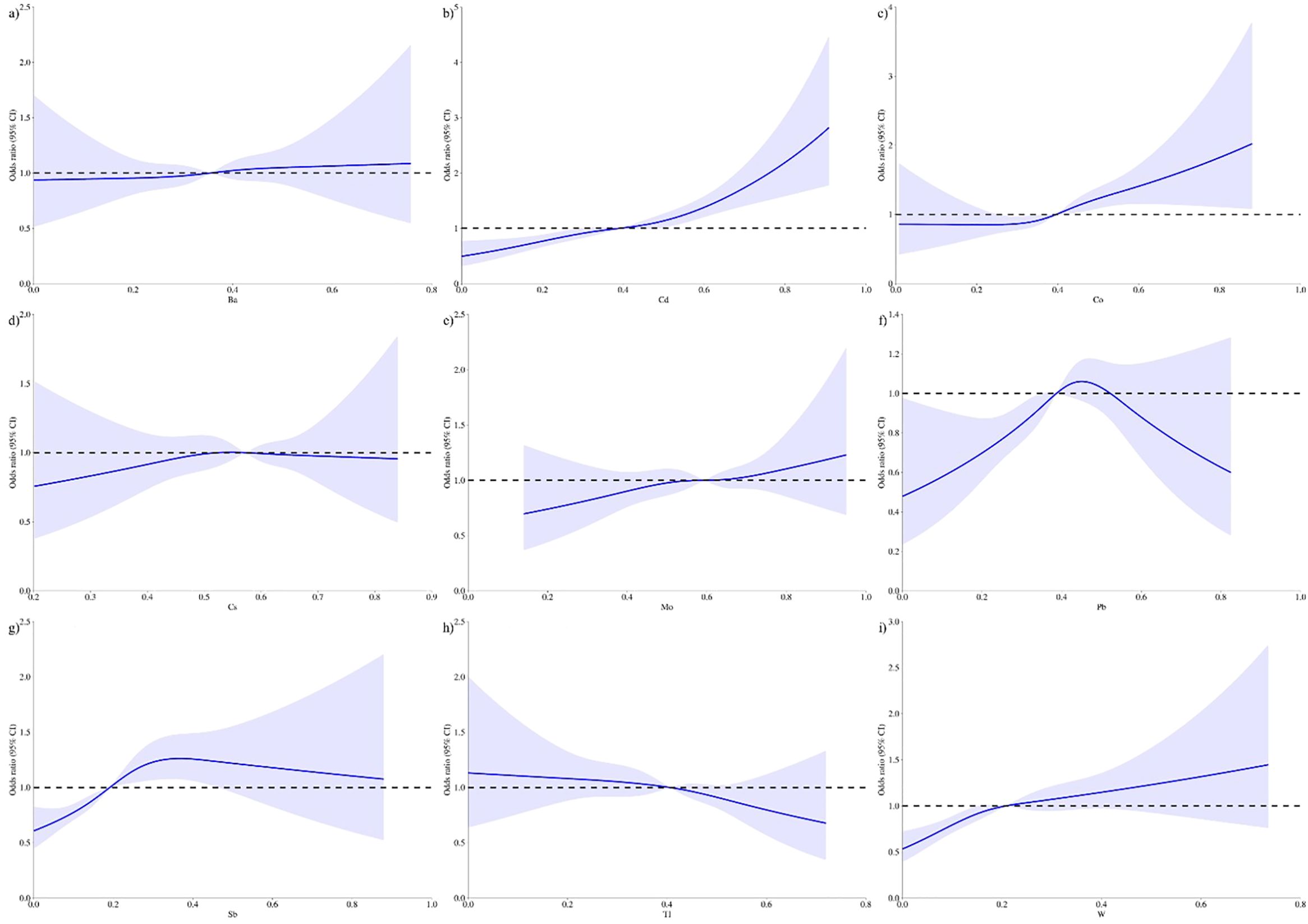
Figure 2. Restricted cubic spline curve describing the dose-response relationship between heavy metal exposure and depression incidence (Unadjusted). : (A) Ba, P = 0.8434; (B) Cd, P<0.001; (C) Co, P = 0.0004; (D) Cs, P = 0.8783; (E) Mo, P = 0.4286; (F) Pb, P = 0.0063; (G) Sb, P<0.001; (H) Tl, P = 0.2696; (I) Ba, P<0.001.
3.3 Subgroup analysis
The correlations between exposure to five heavy metals and both the prevalence and incidence of depression were consistently positive across all analyzed subgroups (Supplementary Figure 3).
In subgroup analyses stratified by factors such as drinking and smoking habits, diabetes, and hypertension, no significant interactions were found for Cd, Co, Pb, Sb, and W as they all showed P-values greater than 0.05 (Supplementary Table 1). However, gender differences were noted where females, compared to males, showed a higher likelihood of depression with excessive exposure to these five metals. Additionally, in the education subgroup analysis, individuals with higher education levels exhibited an increased risk of depression when exposed to higher levels of Cd and Co.
3.4 WQS regression model to assess the association between mixed heavy metals and depression
The WQS model was used to assess the collective impact of nine heavy metals on depression prevalence, as detailed in Supplementary Table 2. The results showed a positive association, with the WQS index revealing significant odds ratios (Unadjusted model: OR=0.4624, 95% CI 0.3159–0.6088, P < 0.001; Model I: OR=1.4842, 95% CI 1.2766–1.7254, P < 0.001). Among the metals, Cd was assigned the highest weight of 0.4654, indicating the most substantial influence on depression risk, and this remained the highest even after adjustment for covariates.
3.5 BKMR model to assess the association between mixed heavy metals and depression
In the BKMR model without adjustments, the risk of depression increased when exposed to a mixture of heavy metals above the 50th percentile, as shown in Figure 3A. In the BKMR model, the PIP value represents the posterior probability of the effect of the variable on the response variable. Supplementary Table 3 summarized the Posterior Inclusion Probabilities (PIP) indicating most heavy metals had a high PIP linked to depression prevalence. Figure 3B illustrated various correlations: a positive correlation with Cd levels, a U-shaped correlation with Ba, Cs, and Tl levels, and an inverted U-shaped correlation with Sb and W levels in depression risk when other metal concentrations were at their median.
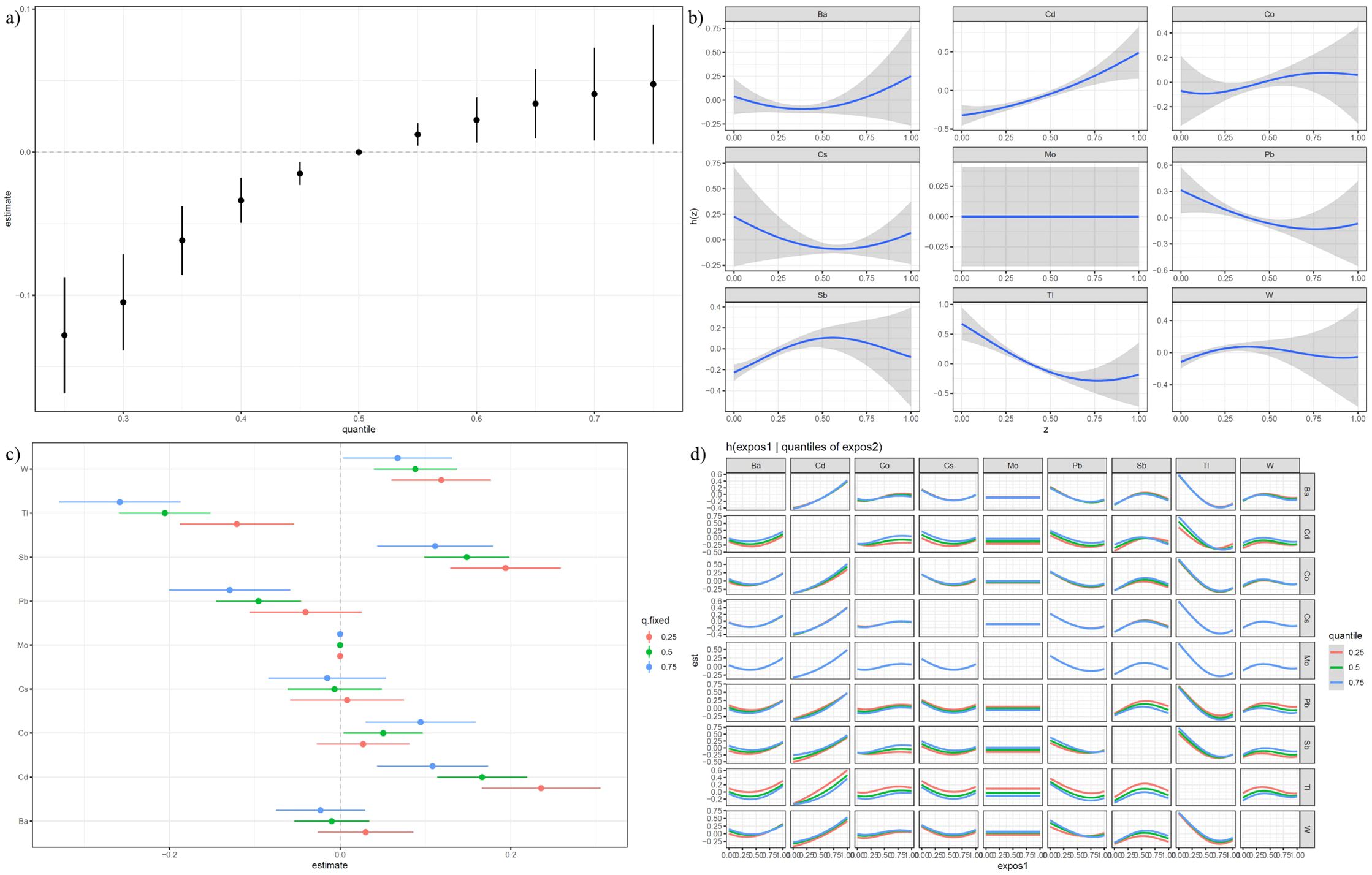
Figure 3. Result of BKMR Model (Unadjusted). (A) is overall effect of heavy metals mixtures on the prevalence of depression in BKMR model where all heavy metals at specific percentiles were compared to their 50th percentile. (B) is univariate exposure–response function between each heavy metal and the prevalence of depression when the other heavy metals were fixed at 50th percentiles. (C) is single exposure-response functions for each heavy metal and prevalence of depression when a single heavy metal was at the 75th compared with the 50th percentile and the concentrations of all the other heavy metals were fixed at either the 25th, 50th, 75th percentile in the BKMR model. (D) is bivariate exposure-response functions for each heavy metal and the prevalence of depression when one heavy metal was fixed at 25th, 50th, 75th percentiles and other heavy metals were fixed at the median in the BKMR model.
Furthermore, Figure 3C showed the impact of heavy metals on depression: W, Sb, Co, and Cd had positive effects, whereas Tl and Pb had negative effects when controlled at the 25th, 50th, and 75th percentiles. Figure 3D indicated no significant interactions among metal concentrations from the 25th to 75th percentiles.
After covariate adjustment, the pattern of increased depression risk for exposures above the 50th percentile persisted (Supplementary Figure 4A). Specific metals showed differing relationships with depression risk when other metals were held at the median: Mo had a negative correlation, while Cs showed a U-shaped and Ba an inverted U-shaped correlation (Supplementary Figure 4B). The trends in the effects of metal exposure on depression incidence remained stable across the 25th, 50th, and 75th percentiles (Supplementary Figure 4C), with no interactions observed between these concentrations (Supplementary Figure 4D).
3.6 Mediating role of sleep duration in the association between heavy metals and prevalence of depression
Concentrations of Cs (Estimate (95%CI) = 0.829 (0.255, 1.402), P = 0.0046), Pb (Estimate (95%CI) = -0.597 (-0.949, -0.245), P = 0.0009), and Sb (Estimate (95%CI) =-0.625 (-0.889, -0.361), P = 0.0000) were notably linked to sleep duration, a relationship that persisted even after adjusting for various covariates. Additionally, Cd’s association with sleep duration became significant with covariate adjustments.
Moreover, sleep duration significantly correlated with the prevalence of depression, presenting an odds ratio (OR) of 0.80 (95% CI: 0.76 – 0.84, P < 0.001). This association remained robust after demographic adjustments (OR = 0.81 (0.78 – 0.85)) and further adjustments for all covariates (OR = 0.82 (0.78 – 0.86), P < 0.001).
According to Figure 5, sleep duration acted as a mediator in the effects of Cd, Mo, Pb, Sb, and W on depression, contributing mediator shares of 2.55%, 11.46%, 16.60%, 7.99%, and 3.68%, respectively. Post-adjustment for covariates, these associations remained significant, with increased mediator shares reported in the model (4.77%, 13.00%, 20.93%, 14.01%, 6.41%) as shown in Supplementary Table 4 and Figure 4.
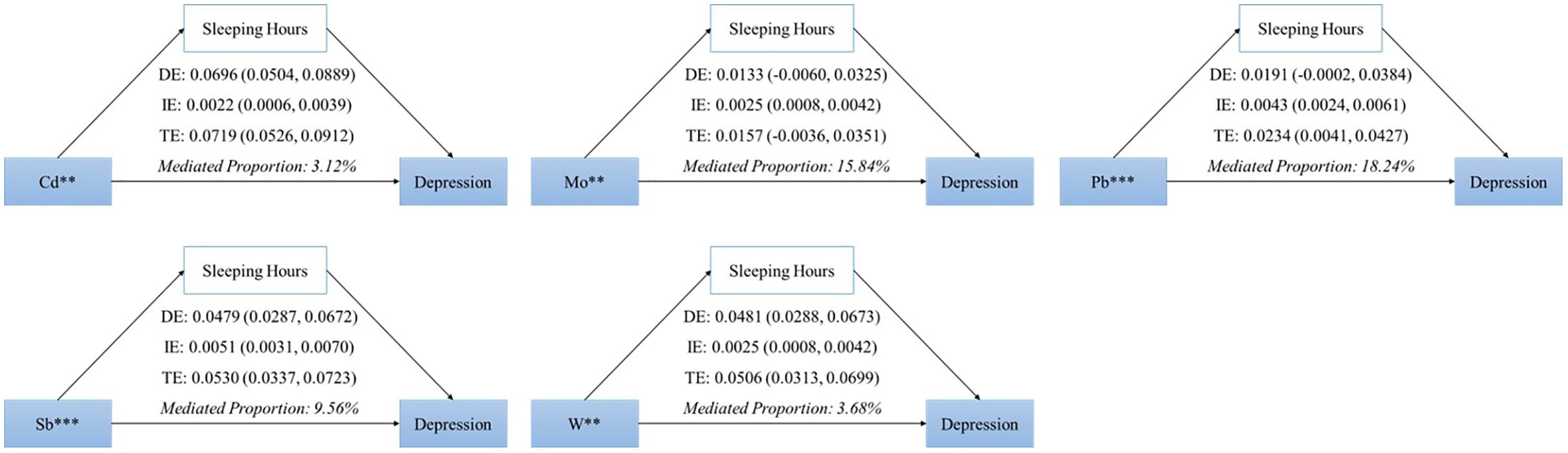
Figure 4. Results of mediation analyses on the association between heavy metal exposure and depression risk mediated by sleep duration (Unadjusted). ** P<0.01, *** P<0.001.
4 Discussion
Depression is characterized by a significant loss of energy, persistent sadness, sleep disturbances, and anhedonia. A worldwide survey in 17 countries found that 1 in 20 people experience depression. Predictions suggest that by 2020, depression will contribute to a 5.7% rise in the global disease burden, ranking it second only to ischemic heart disease (25).
Recent studies have linked heavy metals to a range of health issues, including obesity, metabolic syndrome, and hypertension (26, 27). Concerns about heavy metal contamination are growing globally (28). Research into how individual heavy metals affect different levels of depression is expanding. For example, higher manganese (Mn) levels have been tied to postnatal depression (29), and studies have noted a connection between elevated lead and cadmium levels in the blood and depression (30). There is an increasing focus on investigating how cadmium (Cd) in the blood may relate to depression, highlighting the broader implications of heavy metal exposure on mental health (31).
Previous studies have not considered the combined effects of multiple metals, leaving it unclear if mixed heavy metal exposure is linked to depression prevalence. Our research using WQS and BKMR models showed that exposure to multiple heavy metals significantly raises the risk of depression, with cadmium (Cd) being a key contributor. These results indicate that high levels of mixed heavy metals, especially Cd, are associated with an increased risk of depression.
There is increasing evidence that the length of sleep significantly affects the likelihood of developing depression. Previous research has mostly examined how a lack of sufficient sleep correlates with a greater risk of depression (32, 33). While the negative impacts of inadequate sleep are well established, the potential risks associated with oversleeping have received less scrutiny. Some studies indicate that both insufficient and excessive sleep durations may contribute to a higher risk of depression (34), a finding our research supports. However, there is disagreement among some experts who believe that extended sleep does not increase the risk of depression (35, 36). These variations in research outcomes may be due to different methods of adjusting for potential confounders, diverse definitions of what constitutes long or short sleep, and the age groups of the participants studied.
Excessive sleep may elevate depression risk by disrupting sleep cycles, leading to increased wakefulness and reduced energy (37, 38). It can also decrease physical activity, impairing brain function and reducing factors that prevent depression such as endorphin release and stress diversion (39). Moreover, individuals who sleep excessively often experience heightened vulnerability to mood and anxiety disorders, and increased stress levels, potentially contributing to depression (40). Additionally, long sleep durations might reflect underlying stress, influencing the link between excessive sleep and depression (41).
Recent studies have shown a connection between longer sleep durations and increased exposure to heavy metals. Research indicates that elevated zinc levels or higher selenium intake can either optimize or prolong sleep in American adults (42), while copper levels do not significantly impact sleep duration (42). Additionally, data from the National Health and Nutrition Examination Survey (NHANES) from 2005 to 2008 suggest that low exposure to antimony is associated with poor sleep health, including shorter sleep duration, increased sleep onset latency, obstructive sleep apnea (OSA), and greater daytime sleepiness (43).
Regarding these common mechanisms, it can be supposed that heavy metal can induce the depression, where sleep duration plays a mediating role in this association, which was confirmed by our study.
The subgroup analysis indicated that the connection between exposure to heavy metals and depression varies by gender, with women at higher risk due to biological variations such as hormonal and metabolic differences (44), along with greater psychosocial and environmental challenges (45, 46). Furthermore, individuals with depression may have higher susceptibility to tobacco, a significant source of heavy metals, as evidenced by elevated urinary metabolites in smokers (46, 47). Tobacco uses itself is a known depressive risk factor (48). These insights underscore the necessity of accounting for gender and socio-demographic factors in environmental health studies to devise precise preventive strategies.
Our study presented multiple strengths.
1. It was pioneering in examining the association between mixed heavy metal exposure and depression prevalence.
2. We employed a variety of statistical techniques and made adjustments for potential confounders, thereby enhancing the validity and reliability of our results.
3. The data used in this study came from a large, population-based database with stringent quality controls in place.
However, there were also notable limitations.
1. Due to the cross-sectional nature of our study, it was not possible to infer causality between heavy metal exposure and depression prevalence, highlight the necessity of the perspective randomized control trials in the future.
2. The NHANES database does not include information on certain uncontrollable factors, such as exposure to wastewater and cosmetics, which could influence the accuracy of our findings.
3. The failure to consider cumulative exposure to heavy metals might have affected our conclusions.
5 Conclusion
In summary, our findings show a clear link between exposure to mixed heavy metals and increased depression rates, with cadmium playing a significant role in this association. Additionally, sleep duration serves as a mediator that connects heavy metal exposure to depression prevalence. Future research should focus on further exploring this relationship through prospective studies.
Data availability statement
The raw data supporting the conclusions of this article will be made available by the authors, without undue reservation.
Ethics statement
The studies involving humans were approved by Ethics Review Committee of the American National Health Statistics Center. The studies were conducted in accordance with the local legislation and institutional requirements. The participants provided their written informed consent to participate in this study.
Author contributions
QM: Data curation, Formal analysis, Investigation, Methodology, Software, Visualization, Writing – original draft. XZ: Data curation, Formal analysis, Investigation, Methodology, Visualization, Writing – original draft. YK: Conceptualization, Data curation, Formal analysis, Investigation, Methodology, Project administration, Resources, Software, Supervision, Validation, Visualization, Writing – original draft, Writing – review & editing.
Funding
The author(s) declare that no financial support was received for the research, authorship, and/or publication of this article.
Conflict of interest
The authors declare that the research was conducted in the absence of any commercial or financial relationships that could be construed as a potential conflict of interest.
Publisher’s note
All claims expressed in this article are solely those of the authors and do not necessarily represent those of their affiliated organizations, or those of the publisher, the editors and the reviewers. Any product that may be evaluated in this article, or claim that may be made by its manufacturer, is not guaranteed or endorsed by the publisher.
Supplementary material
The Supplementary Material for this article can be found online at: https://www.frontiersin.org/articles/10.3389/fpsyt.2024.1455896/full#supplementary-material
References
1. Mathers CD, Loncar D. Projections of global mortality and burden of disease from 2002 to 2030. PloS Med. (2006) 3:e442. doi: 10.1371/journal.pmed.0030442
2. Freeman M. The World Mental Health Report: transforming mental health for all. World Psychiatry. (2022) 21:391–2. doi: 10.1002/wps.21018
3. Cuijpers P, Vogelzangs N, Twisk J, Kleiboer A, Li J, Penninx BW. Comprehensive meta-analysis of excess mortality in depression in the general community versus patients with specific illnesses. Am J Psychiatry. (2014) 171:453–62. doi: 10.1176/appi.ajp.2013.13030325
4. Oude Voshaar RC, Aprahamian I, Borges MK, van den Brink RHS, Marijnissen RM, Hoogendijk EO, et al. Excess mortality in depressive and anxiety disorders: The Lifelines Cohort Study. Eur Psychiatry. (2021) 64:e54. doi: 10.1192/j.eurpsy.2021.2229
5. GBD 2019 Diseases and Injuries Collaborators. Global burden of 369 diseases and injuries in 204 countries and territories, 1990-2019: a systematic analysis for the Global Burden of Disease Study 2019. Lancet. (2020) 396:1204–22. doi: 10.1016/S0140-6736(20)30925-9
6. Charkiewicz AE, Backstrand JR. Lead toxicity and pollution in Poland. Int J Environ Res Public Health. (2020) 17:4385. doi: 10.3390/ijerph17124385
7. Wei J, Zhang JJ, Ji JS. Association of environmental exposure to heavy metals and eczema in US population: Analysis of blood cadmium, lead, and mercury. Arch Environ Occup Health. (2019) 74:239–51. doi: 10.1080/19338244.2018.1467874
8. Pemberton R, Fuller Tyszkiewicz MD. Factors contributing to depressive mood states in everyday life: A systematic review. J Affect Disord. (2016) 200:103–10. doi: 10.1016/j.jad.2016.04.023
9. Méndez-Armenta M, Ríos C. Cadmium neurotoxicity. Environ Toxicol Pharmacol. (2007) 23:350–8. doi: 10.1016/j.etap.2006.11.009
10. Zhang T, Luo J, Ge H, Hao K, Wang Z, Zhang D. Relationships between urinary antimony concentrations and depressive symptoms in adults. Chemosphere. (2022) 291. doi: 10.1016/j.chemosphere.2021.133104
11. Vanden Eng JL, Chan A, Abílio AP, Wolkon A, Ponce de Leon G, Gimnig J, et al. Bed net durability assessments: exploring a composite measure of net damage. PloS One. 10:e0128499. doi: 10.1371/journal.pone.0128499
12. Chen P, Bornhorst J, Patton S, Bagai K, Nitin R, Miah M, et al. A potential role for zinc in restless legs syndrome. Sleep. (2021) 44:zsaa236. doi: 10.1093/sleep/zsaa236
13. Roussel B, Renaud B. Effect of chronic manganese intoxication on the sleep-wake cycle in the rat. Neurosci Lett. (1977) 4:55–60. doi: 10.1016/0304-3940(77)90125-2
14. Vetrivelan R, Qiu MH, Chang C, Lu J. Role of Basal Ganglia in sleep-wake regulation: neural circuitry and clinical significance. Front Neuroanat. (2010) 4:145. doi: 10.3389/fnana.2010.00145
15. Asker S, Asker M, Yeltekin AC, Aslan M, Demir H. Serum levels of trace minerals and heavy metals in severe obstructive sleep apnea patients: correlates and clinical implications. Sleep Breath. (2015) 19:547–52. doi: 10.1007/s11325-014-1045-2
16. Caudill SP, Schleicher RL, Pirkle JL. Multi-rule quality control for the age-related eye disease study. Statist Med. (2008) 27:4094–106. doi: 10.1002/sim.3222
17. Westgard JO, Barry PL, Hunt MR, Groth T. A multi-rule Shewhart chart for quality control in clinical chemistry. Clin Chem. (1981) 27:493–501. doi: 10.1093/clinchem/27.3.493
18. Kroenke K, Spitzer RL, William JB. The PHQ-9: validity of a brief depression severity measure. J Gen Intern Med. (2001) 16:1606–13. doi: 10.1046/j.1525-1497.2001.016009606.x
19. Kroenke K, Spitzer RL. The PHQ-9: a new depression and diagnostic severity measure. Psych Ann. (2002) 32:509–21. doi: 10.3928/0048-5713-20020901-06
20. Spitzer RL, Kroenke K, Williams JB. Validation and utility of a self-report version of Prime MD: the PHQ primary care study. JAMA. (1999) 282:1737–44. doi: 10.1001/jama.282.18.1737
21. Leem AY, Kim YS, Chung KS, Park MS, Kang YA, Park YM, et al. Sarcopenia is associated with cardiovascular risk in men with COPD, independent of adiposity. Respir Res. (2022) 23:185. doi: 10.1186/s12931-022-02109-3
22. Kong Y, Xu H, Li C, Yang Y, Zhu X, Zuo Y. Construction of PARI public health education programs for Chinese undergraduates: a Delphi study. Front Public Health. (2024) 12:1390011. doi: 10.3389/fpubh.2024.1390011
23. Kong Y, Zhu X, Yang Y, Xu H, Ma L, Zuo Y. Knowledge, attitudes, practice, and public health education demand regarding PARI prevention: a cross-sectional study among Chinese undergraduates. Front Public Health. (2024) 12:1387789. doi: 10.3389/fpubh.2024.1387789
24. Cheng Z, Kong Y, Yang W, Xu H, Tang D, Zuo Y. Association between serum copper and blood glucose: a mediation analysis of inflammation indicators in the NHANES (2011-2016). Front Public Health. (2024) 12:1401347. doi: 10.3389/fpubh.2024.1401347
25. Kim S, Park T, Kim K, Oh J, Park Y, Kim D, et al. A deep learning algorithm to predict hazardous drinkers and the severity of Alcohol-Related problems using K-NHANES. Front Psychiatry. (2021) 12:684406. doi: 10.3389/fpsyt.2021.684406
26. Peters JL, Fabian MP, Levy JI. Combined impact of lead, cadmium, polychlorinated biphenyls and non-chemical risk factors on blood pressure in NHANES. Environ Res. (2014) 132:93–9. doi: 10.1016/j.envres.2014.03.038
27. Wang X, Mukherjee B, Park SK. Associations of cumulative exposure to heavy metal mixtures with obesity and its comorbidities among U.S. adults in NHANES 2003-2014. Environ Int. (2018) 121:683–94. doi: 10.1016/j.envint.2018.09.035
28. Wu KG, Chang CY, Yen CY, Lai CC. Associations between environmental heavy metal exposure and childhood asthma: A population-based study. J Microbiol Immunol Infect. (2019) 52:352–62. doi: 10.1016/j.jmii.2018.08.001
29. McRae N, Bello G, Svensson K, Solano-González M, Wright RJ, Niedzwiecki MM, et al. Blood manganese levels during pregnancy and postpartum depression: A cohort study among women in Mexico. Neurotoxicology. (2020) 76:183–90. doi: 10.1016/j.neuro.2019.11.005
30. Buser MC, Scinicariello F. Cadmium, lead, and depressive symptoms: analysis of national health and nutrition examination survey 2011-2012. J Clin Psychiatry. (2017) 78:e515–21. doi: 10.4088/JCP.15m10383
31. Tian X, Xue B, Wang B, Lei R, Shan X, Niu J, et al. Physical activity reduces the role of blood cadmium on depression: A cross-sectional analysis with NHANES data. Environ pollut. (2022) 304:119211. doi: 10.1016/j.envpol.2022.119211
32. Berger AT, Wahlstrom KL, Widome R. Relationships between sleep duration and adolescent depression: a conceptual replication. Sleep Health. (2019) 5:175–9. doi: 10.1016/j.sleh.2018.12.003
33. Sun Y, Shi L, Bao Y, Sun Y, Shi J, Lu L. The bidirectional relationship between sleep duration and depression in community-dwelling middle-aged and elderly individuals: evidence from a longitudinal study. Sleep Med. (2018) 52:221–9. doi: 10.1016/j.sleep.2018.03.011
34. Reis C, Dias S, Rodrigues AM, Sousa RD, Gregório MJ, Branco J, et al. Sleep duration, lifestyles and chronic diseases: a cross-sectional population-based study. Sleep Sci. (2018) 11:217–30. doi: 10.5935/1984-0063.20180036
35. Ouyang P, Sun W. Depression and sleep duration: findings from middle-aged and elderly people in China. Public Health. (2019) 166:148–54. doi: 10.1016/j.puhe.2018.10.007
36. Matsui K, Kuriyama K, Yoshiike T, Nagao K, Ayabe N, Komada Y, et al. The effect of short or long sleep duration on quality of life and depression: an internet-based survey in Japan. Sleep Med. (2020) 76:80–5. doi: 10.1016/j.sleep.2020.10.012
37. Youngstedt SD, Kripke DF. Long sleep and mortality: rationale for sleep restriction. Sleep Med Rev. (2004) 8:159–74. doi: 10.1016/j.smrv.2003.10.002
38. Bennett LS, Barbour C, Langford B, Stradling JR, Davies RJ. Health status in obstructive sleep apnea: relationship with sleep fragmentation and daytine sleepiness, and effects of continuous positive airway pressure treatment. Am J Respir Crit Care Med. (1999) 159:1884–90. doi: 10.1164/ajrccm.159.6.9808107
39. Weicker H, Strüder HK. Influence of exercise on serotonergic neuromodulation in the brain. Amino Acids. (2001) 20:35–47. doi: 10.1007/s007260170064
40. Patel SR, Malhotra A, Gottlieb DJ, White DP, Hu FB. Correlates of long sleep duration. Sleep. (2006) 29:881–9. doi: 10.1093/sleep/29.7.881
41. Zhai L, Zhang H, Zhang D. SLEEP DURATION AND DEPRESSION AMONG ADULTS: A META-ANALYSIS OF PROSPECTIVE STUDIES. Depress Anxiety. (2015) 32:664–70. doi: 10.1002/da.22386
42. Jia YN, Sun J, Chen L, Xue Y. Associations of serum zinc, copper, and zinc/copper ratio with sleep duration in adults. Biol Trace Elem Res. (2022) 200:2651–9. doi: 10.1007/s12011-021-02897-7
43. Scinicariello F, Buser MC, Feroe AG, Attanasio R. Antimony and sleep-related disorders: NHANES 2005-2008. Environ Res. (2017) 156:247–52. doi: 10.1016/j.envres.2017.03.036
44. Yamagata AT, Guimarães NC, Santana DF, Gonçalves MR, Souza VC, Barbosa Júnior F, et al. Gender influence on manganese induced depression-like behavior and Mn and Fe deposition in different regions of CNS and excretory organs in intraperitoneally exposed rats. Toxicology. (2017) 376:137–45. doi: 10.1016/j.tox.2016.05.012
45. Hwang S, Shin H. Gender gap in mental health during the COVID-19 pandemic in South Korea: A decomposition analysis. Int J Environ Res Public Health. (2023) 20:2250. doi: 10.3390/ijerph20032250
46. Jiang M, Zhao H. Joint association of heavy metals and polycyclic aromatic hydrocarbons exposure with depression in adults. Environ Res. (2024) 242:117807. doi: 10.1016/j.envres.2023.117807
47. Kim SW, Jung SW, Lee JG, Joo JH, Lee JH, Lee KJ. The exposure level of environmental harmful substances related to the secondhand smoke in Korean non-smoker adults: data from the second Korean National Environmental Health Survey (KoNEHS 2012-2014): a cross-sectional study. Ann Occup Environ Med. (2019) 31:e30. doi: 10.35371/aoem.2019.31.e30
Keywords: sleep duration, heavy metal, depression, mediation analysis, national health and nutrition examination survey
Citation: Mao Q, Zhu X and Kong Y (2024) Sleep duration mediates the association between heavy metals and the prevalence of depression: an integrated approach from the NHANES (2005–2020). Front. Psychiatry 15:1455896. doi: 10.3389/fpsyt.2024.1455896
Received: 27 June 2024; Accepted: 08 August 2024;
Published: 02 September 2024.
Edited by:
Liana Fattore, CNR Neuroscience Institute (IN), ItalyCopyright © 2024 Mao, Zhu and Kong. This is an open-access article distributed under the terms of the Creative Commons Attribution License (CC BY). The use, distribution or reproduction in other forums is permitted, provided the original author(s) and the copyright owner(s) are credited and that the original publication in this journal is cited, in accordance with accepted academic practice. No use, distribution or reproduction is permitted which does not comply with these terms.
*Correspondence: Yuzhe Kong, Y3N1eXV6aGVrb25nQGZveG1haWwuY29t