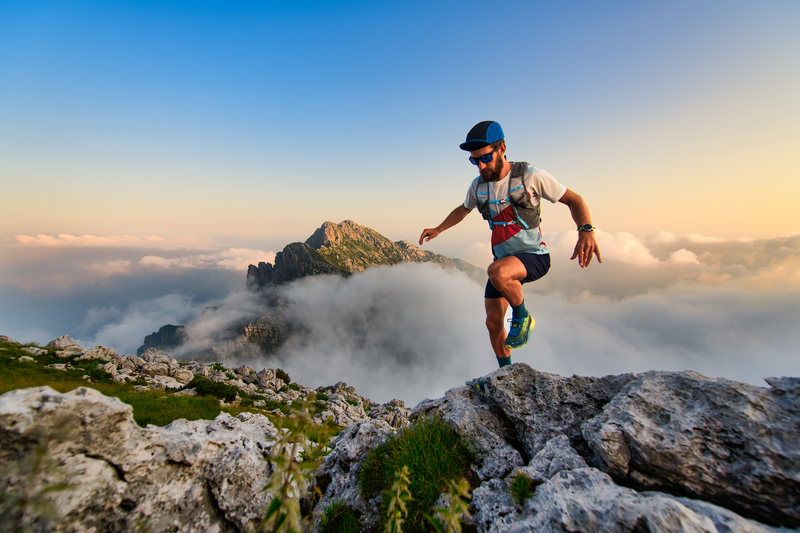
94% of researchers rate our articles as excellent or good
Learn more about the work of our research integrity team to safeguard the quality of each article we publish.
Find out more
EDITORIAL article
Front. Psychiatry , 12 June 2024
Sec. Forensic Psychiatry
Volume 15 - 2024 | https://doi.org/10.3389/fpsyt.2024.1435219
This article is part of the Research Topic Applications of Artificial Intelligence in Forensic Mental Health: Opportunities and Challenges View all 5 articles
Editorial on the Research Topic
Applications of artificial intelligence in forensic mental health: opportunities and challenges
In recent years, growing attention has been paid to the possible application of artificial intelligence (AI) techniques and machine learning (ML) in clinical and forensic settings.
AI methods based on Knowledge Representation and Automated Reasoning (KR&R), Model Checking (MC) as well as Machine (deep-)Learning (ML) have been employed for the development of predictive quantitative models for, e.g., biochemical reactions, human pathophysiology, and many other domains.
In the forensic field, discriminative AI has been applied for predicting risk of aggression (Kirchebner et al., 2020; Gou et al., 2021; Parmigiani et al., 2022; Watts et al., 2021), crime recidivism (Tollenaar and van der Heijden, 2019), and forecasting future offences (Watts et al., 2021). In addition, AI has been used to inform decisions about sentencing, parole, probation or pretrial risk assessment, raising several legal and ethical concerns regarding, among others, fairness, accountability and transparency (Tortora et al., 2020). These concerns stem, for instance, from the finding that some algorithms contained race and gender bias (Barabas et al., 2018), the fact that the scores may be misapplied and misinterpreted by judges and practitioners (Hannah-Moffat, 2015), and proprietary issues which can contribute to a lack of transparency (Barabas et al., 2018).
This Research Topic aims to present cutting-edge studies on the application of AI techniques in the forensic mental health field, including research on ethical challenges such as those related to the need to ensure non-discrimination, the “fair process” and the values of transparency and comprehensibility of decision-making processes.
The contributing works are two original articles (Bender et al. Machetanz et al.), one perspective article (Starke et al.) and one conceptual analysis article (Tortora).
Bender et al. employed supervised machine learning algorithms in order to identify distinguishing factors between 269 offenders and 184 non-offenders affected by both schizophrenia spectrum disorders and substance use disorder. The ML model, with an AUC of 0.88, found the most influential distinguishing factors to be: failures during opening (defined as rule violations during a permitted temporally leave), nonadherence to treatment, social isolation, and the absence of antipsychotic prescription and no outpatient psychiatric treatments before the current hospitalization.
Similarly, Machetanz et al. used discriminative AI (through the use of supervised ML algorithms), to build a model, with an AUC of 0.86, able to investigate the clinical key factors discriminating 370 offenders and 370 non-offenders diagnosed with a schizophrenia spectrum disorder. The most influential predictors were: olanzapine equivalent at discharge, history of antipsychotic prescription, a history of antidepressant, benzodiazepine or mood stabilizer prescription, medication compliance, outpatient treatment(s) in the past, and the necessity of compulsory measures.
Starke et al. wrote a perspective article focused on the ethical challenges of using AI methods in forensic psychiatry. The authors argue that, given the importance and influence of environment and social circumstances on the onset and course of psychiatric disorders, we should not overlook these factors when developing predictive algorithms (from the collection of data in the training set, to the selection of ML methods, and the formulation of the explainability requirements).
Finally, Tortora in her conceptual analysis article, examined the potential of Generative AI in reshaping forensic mental health practice in comparison with the Discriminative AI paradigm. Next, she addresses ethical and legal issues associated with Generative AI application, such as, biased and stereotyped outputs, lack of transparency, ‘hallucinations’ and facts fabrication, highlighting the risks of the spread of misinformation and the reinforcement of discriminatory and criminalizing narratives and stereotypes.
This Research Topic provides a collection of studies on the application of AI techniques in the forensic mental health setting. While underlining the potential of both discriminative and generative AI for this domain, it also emphasizes associated risks and ethical concerns. We hope this Research Topic will be a helpful reference for forensic practitioners who must deal with the increasing role of IA in their work, and that it will provide a stimulus for much-needed further research on this fascinating theme.
GP: Writing – original draft, Writing – review & editing. GM: Writing – review & editing. TM: Writing – review & editing. SF: Writing – review & editing.
The authors declare that the research was conducted in the absence of any commercial or financial relationships that could be construed as a potential conflict of interest.
The author(s) declared that they were an editorial board member of Frontiers, at the time of submission. This had no impact on the peer review process and the final decision.
All claims expressed in this article are solely those of the authors and do not necessarily represent those of their affiliated organizations, or those of the publisher, the editors and the reviewers. Any product that may be evaluated in this article, or claim that may be made by its manufacturer, is not guaranteed or endorsed by the publisher.
Barabas C., Dinakar K., Ito J., Virza M., Zittrain J. (2018). Interventions over predictions: reframing the ethical debate for actuarial risk assessment. Proc. Mach. Learn. Res. 81, 1–15.
Gou N., Xiang Y., Zhou J., Zhang S., Zhong S., Lu J., et al. (2021). Identification of violent patients with schizophrenia using a hybrid machine learning approach at the individual level. Psychiatry Res. 306, 114294. doi: 10.1016/j.psychres.2021.114294
Hannah-Moffat K. (2015). The uncertainties of risk assessment: partiality, transparency, and just decisions. Federal Sentencing Rep. 27 (4), 244–247. doi: 10.1525/fsr.2015.27.4.244
Kirchebner J., Gunther M. P., Sonnweber M., King A., Lau S. (2020). Factors and predictors of length of stay in offenders diagnosed with schizophrenia - a machine-learning-based approach. BMC Psychiatry 20 (1), 201. doi: 10.1186/s12888-020-02612-1
Parmigiani G., Barchielli B., Casale S., Mancini T., Ferracuti S. (2022). The impact of machine learning in predicting risk of violence: a systematic review. Front. Psychiatry 13, 1015914. doi: 10.3389/fpsyt.2022.1015914
Tollenaar N., van der Heijden P. G. M. (2019). Optimizing predictive performance of criminal recidivism models using registration data with binary and survival outcomes. PloS One 14 (3), e0213245. doi: 10.1371/journal.pone.0213245
Tortora L., Meynen G., Bijlsma J., Tronci E., Ferracuti S. (2020). Neuroprediction and A.I. in forensic psychiatry and criminal justice: a neurolaw perspective. Front. Psychol. 11, 220. doi: 10.3389/fpsyg.2020.00220
Keywords: artificial intelligence, forensic psychiatry, machine learning (ML), offenders, ethical challenges
Citation: Parmigiani G, Meynen G, Mancini T and Ferracuti S (2024) Editorial: Applications of artificial intelligence in forensic mental health: opportunities and challenges. Front. Psychiatry 15:1435219. doi: 10.3389/fpsyt.2024.1435219
Received: 19 May 2024; Accepted: 31 May 2024;
Published: 12 June 2024.
Edited and Reviewed by:
Birgit Angela Völlm, University of Rostock, GermanyCopyright © 2024 Parmigiani, Meynen, Mancini and Ferracuti. This is an open-access article distributed under the terms of the Creative Commons Attribution License (CC BY). The use, distribution or reproduction in other forums is permitted, provided the original author(s) and the copyright owner(s) are credited and that the original publication in this journal is cited, in accordance with accepted academic practice. No use, distribution or reproduction is permitted which does not comply with these terms.
*Correspondence: Giovanna Parmigiani, Z2lvdmFubmEucGFybWlnaWFuaUB1bmlyb21hMS5pdA==
Disclaimer: All claims expressed in this article are solely those of the authors and do not necessarily represent those of their affiliated organizations, or those of the publisher, the editors and the reviewers. Any product that may be evaluated in this article or claim that may be made by its manufacturer is not guaranteed or endorsed by the publisher.
Research integrity at Frontiers
Learn more about the work of our research integrity team to safeguard the quality of each article we publish.