- 1Department of Psychiatry, University of Illinois Chicago, Chicago, IL, United States
- 2Department of Psychology, Harvard University, Cambridge, MA, United States
This paper discusses the scientific rationale and methodological considerations for incorporating the menstrual cycle as a time-varying intra-individual factor in personalized medicine models, such as Just-In-Time Adaptive Interventions (JITAIs). Among patients, accumulating evidence suggests that the normal hormone fluctuations of the menstrual cycle represent a time-varying factor that can trigger or exacerbate psychiatric symptoms, including but not limited to affective dysregulation, suicidality, and irritability. While only a minority of the general female population experiences significant cyclical changes, this hormone-sensitive response appears to be greater among patients with psychiatric disorders, with studies demonstrating that a majority of patients recruited for past-month suicidal ideation demonstrate worsening of their suicidality around menses. However, no interventions target suicidality during this monthly period of elevated risk despite evidence of a clear recurring biological trigger. This unique and recurrent “biotype” of suicidality is well-suited for JITAIs. In addition to providing a rationale for the inclusion of the cycle in JITAI, we provide illustrative options and examples regarding the measurement and implementation of cycle variables in JITAIs. We discuss how JITAIs might be leveraged to use menstrual cycle data to identify states of vulnerability within people and strategically select and deploy interventions based upon their receptivity at various phases in the cycle. Furthermore, we discuss how to integrate passive measures for tracking the menstrual cycle. Although much research is needed before implementation, we maintain that the menstrual cycle represents a critically understudied time-varying feature that may markedly improve the accuracy of JITAI models for predicting suicidality.
1 Introduction
Despite decades of research, suicide deaths in the United States have not decreased, with mortality rate increasing by 35% between 2000 and 2018 (1). Nonetheless, empirical studies have yielded a critical insight: suicidal ideation (SI) and behaviors (SB) result from highly complex processes that fluctuate over time in person-specific (i.e., idiographic) ways (2, 3). In response, scientists are developing Just-in-Time Adaptive Inventions (JITAIs) for suicide prevention, which model specific time-varying risk factors for each person and intervene in timely, tailored ways (4). In tandem, longitudinal and experimental studies have begun to establish the menstrual cycle as a critical time-varying source of imminent suicide risk, especially in those with chronic suicidality (5–8). Responding to calls from the National Institute of Health to (a) use JITAIs to optimize mental health treatments (9) and (b) focus on female-specific health conditions (10, 11), we argue for integrating the menstrual cycle into JITAI development for suicide prevention. This manuscript discusses why JITAI models may be optimal for treating menstrual cycle-related suicidality, methodological recommendations, and considerations for future research.
1.1 Brief introduction to digital interventions and JITAI
Digital interventions broadly involve identifying vulnerability (e.g., adverse health symptoms) and receptivity [e.g., readiness to use supports (12)] states to deploy personalized interventions aimed at reducing both proximal (i.e., mediator pathways) and distal (i.e., ultimate clinical goals) outcomes. JITAIs tailor treatment type, timing, and intensity based on a patient’s evolving needs, delivering support when it is most effective, and patients are most receptive (13). Typically delivered via smartphone, JITAIs have been studied across various health conditions (14–16). They involve decision points (when interventions can be delivered), intervention options (treatments available at decision points), tailoring variables (when and how to intervene), and decision rules (guidelines for choosing and timing interventions).
1.2 JITAI is a promising method for personalized suicide prevention
There is growing interest in applying JITAI to psychopathology research and practice, including suicidality (4, 17, 18), given the dynamic nature of mental health symptoms (19, 20). JITAIs may enhance evidence-based treatments for mental health conditions (4), for example, by promoting coping skill use during critical moments in between psychotherapy sessions. Specifically, JITAI aims to deliver the best-suited evidence-based intervention for an individual at any given moment based upon the individual’s previous data. While there are no published studies on JITAIs for suicidality, researchers are currently theorizing the best methodology (regarding feasibility, statistics, and ethics) for building these models (2, 4). This work builds on previous suicide research, indicating that suicidality is (a) heterogeneous, with no singular antecedent, content, or function (2, 21), and (b) time-varying (3), and that (c) mobile and internet interventions for suicide prevention show positive treatment effects (22). Thus, JITAI is a promising method in development for suicide prevention.
1.3 The menstrual cycle as a powerful idiographic predictor of suicide risk and associated symptoms for JITAI models
While suicide death is more common among males, females exhibit a greater risk of depression, SI, and suicide attempts—particularly during reproductive years, when ovarian hormones are elevated and fluctuating (23, 24). The menstrual cycle is the primary source of these fluctuations, responsible for a predictable monthly pattern of ovarian hormone change. While most females do not experience significant affective changes in response to the cycle, a substantial minority experience distressing symptoms requiring diagnosis and treatment (25, 26). The menstrual cycle represents a recurring time-varying risk factor for imminent suicide risk in some—but not all—females. Cross-sectional studies demonstrate that patients are more likely to make a suicide attempt just prior to and during menses (27, 28). Among females recruited for past-month SI, most patients demonstrate peak affective symptoms and SI around menses onset, with the cycle accounting for approximately 25% of the within-person variance in daily SI (7, 8). Similarly, suicide risk is elevated among patients with prospectively-confirmed premenstrual dysphoric disorder (PMDD)—a severe form of emotional premenstrual symptoms (29). In a global study of PMDD patients, 71.6% indicated lifetime active SI, 48.58% planning, and 34.72% reported lifetime attempt. A smaller, more tightly controlled study observed high rates (~40%) of current SI in the luteal phase of patients with prospectively-confirmed PMDD (29).
The menstrual cycle is not only a crucial biological predictor of suicide risk but also of suicide-related symptom networks that can guide targeted interventions. Recent work has identified several affective symptoms (e.g., depression, perceived burdensomeness) as mediators of the relationship between the menstrual cycle and suicidality (8). Additionally, distinct hormonal events within the menstrual cycle trigger different symptom clusters associated with suicidality. For instance, progesterone surges in the luteal phase are linked to irritability, interpersonal conflict, and hyperarousal, while estrogen withdrawal before and during menses is associated with depression, anhedonia, and impaired cognitive function (6, 28). These symptom profiles—one driven by progesterone and the other by estrogen—provide a framework for understanding how menstrual cycle phases can correlate with specific patterns of suicidality and pave the way for JITAI models to match hormone-driven suicide-symptom clusters to specific interventions that deploy at the appropriate time in the cycle.
In summary, the luteal/perimenstrual phase of the menstrual cycle may represent the only recurrent, biological, predictable, idiographic variable capable of predicting phases of increased suicide risk and associated symptom clusters. Incorporating the menstrual cycle into JITAI models offers the opportunity to better predict when an individual is most likely to experience suicidality and which hormone-driven affective symptom cluster is most linked to suicidality for the individual, all in service of delivering timely and effective evidence-based treatments (6, 29, 30). In Figure 1 we review a schematic model of how the menstrual cycle may be leverged for JITAI models.
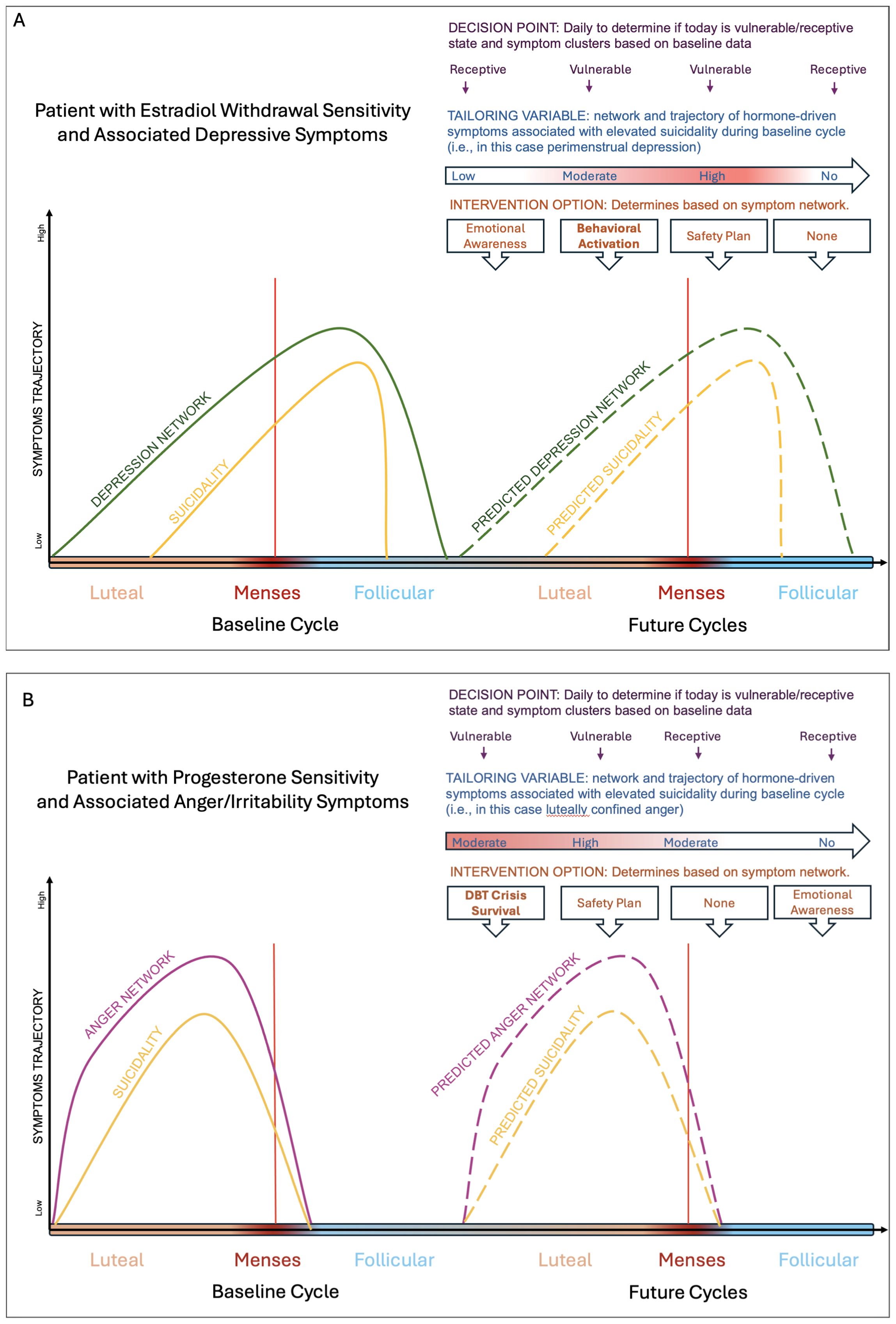
Figure 1. Schematic figure depicting how JITAIs can be used to identify risk states and interventions for patients who experience changes in their suicidal thoughts and behaviors across the cycle from their baseline ratings. Panel (A) is a schematic of a patient experiencing premenstrual exacerbation (i.e., worsening of their symptoms around menses onset) of their psychiatric symptom, with the peak worsening a few days after menses onset. The idiographic driver of their suicidal thoughts and behaviors worsening is depression and hopelessness. In this case, behavioral activation could be deployed to mitigate depression and hopelessness. Panel (B) is a schematic of a patient experiencing luteal confined symptoms, with the symptoms worsening a few days prior to menses onset and completely clearing out a few days post menses. The idiographic driver of their suicidal thoughts and behaviors is anger and interpersonal conflict. In this case, skills from Dialectical Behavioral Therapy may be deployed such as crisis survival skills may be deployed.
1.4 The implementation of JITAI is useful for scientific and treatment research on menstrual cycle-related psychiatric changes
Currently, the only cycle-related psychiatric diagnosis is PMDD, which affects 5.5% of females (31) and is characterized by significant affective, cognitive, and physical symptoms during the week prior to menses that become minimal or absent by the time menses ends. However, similar cyclical changes appear to be more prevalent among psychiatric patient populations, termed premenstrual exacerbation (PME); in which psychiatric symptoms persist throughout the cycle but worsen premenstrually (32). PME has been observed frequently among those with depression (31), suicidality (8), and other psychiatric disorders, including borderline personality disorder, eating disorders, and bipolar disorder (33, 34). However, there is currently no DSM-V diagnosis or specifier for PME. Although these clinical categories (i.e., PMDD/PME) are helpful in specific clinical contexts, there is more variability and dimensionality in symptoms across the cycle than can be captured by these trait-like diagnoses.
Integrating JITAI with the menstrual cycle addresses methodological challenges of high false positive rates in cross-sectional assessments of premenstrual symptom change (34, 35)]. Moreover, JITAI’s reliance on ecological momentary assessment (EMA) allows for a baseline assessment in which symptoms are rated daily across two menstrual cycles to inform the timing of future assessment and intervention. JITAI also allows for a dimensional/continuous approach to modeling affective and suicidal symptoms across the cycle—without being hindered by the need for discrete diagnostic categorization (i.e., PMDD/PME).
Most importantly, JITAI approaches align with the unmet treatment needs of patients with cyclical affective symptoms (i.e., PMDD/PME) and suicidality. JITAI’s person-specific data collection and modeling are well-suited to support understanding the role of the menstrual cycle in patients’ unique symptom trajectories, which is critical given the significant heterogeneity in the timing and content of premenstrual symptoms (30, 36). Therefore, JITAIs seem well-suited to enhance treatment for this population, given the limited number of effective treatment options, limited expert providers, and the strong patient desire for psychosocial interventions (37).
2 Preliminary methodological recommendations for integrating menstrual cycle-related suicidality in JITAI development
Idiographic suicide research has been primarily qualitative (i.e., suicide notes, case studies, and chart reviews) rather than quantitative due to statistical constraints. However, computational advancements (e.g., machine learning) enable more complex modeling that accommodates the heterogeneity of suicide processes across time and unique to each patient (21, 38, 39). We argue that JITAIs can predict how cycle phase drives certain symptoms that exacerbate suicidality and then select the appropriate intervention based on those hormone-driven symptoms. Below we describe how menstrual cycle data can be used in a variety of ways as a digital biomarker (40).
2.1 Selecting patients
JITAI for menstrual cycle-related suicidality is designed for patients experiencing predictable symptom changes across their natural menstrual cycle and patients with PMDD. Thus, the first prerequisite is selecting individuals who are “naturally cycling” without additional hormone variability [e.g., not pregnant, on hormonal birth control, or perimenopausal; for further details see (41)]. Next, this naturally cycling patient must demonstrate menstrual cycle-related suicidality; thus, at least two months of baseline symptom ratings are needed. This will allow for accurate modeling of the typical timing of symptom onset relative to the percent of cycle phase elapsed and symptom clusters. Alternatively, if patients already have prospectively confirmed PMDD or PME of SI diagnoses from a provider, they may benefit from this JITAI as well.
2.2 Statistical models for identifying subgroups of individuals for whom the menstrual cycle significantly impacts suicidality
Currently, algorithms for assessing PMDD and PME are available [C-PASS (42] although they remain strict in their diagnostic categorization, similar methods could be applied flexibly to understand cyclical change. As an initial approach, one might compare the mean of the highest-risk premenstrual days (3 days prior to menses onset to the first two days of menses) to the mean of the lowest-risk postmenstrual days (days 6-12 after menses onset) and evaluate if there is >=15% change in suicidality (8, 42). However, other statistical methods could be applied, as well, see (Table 1).
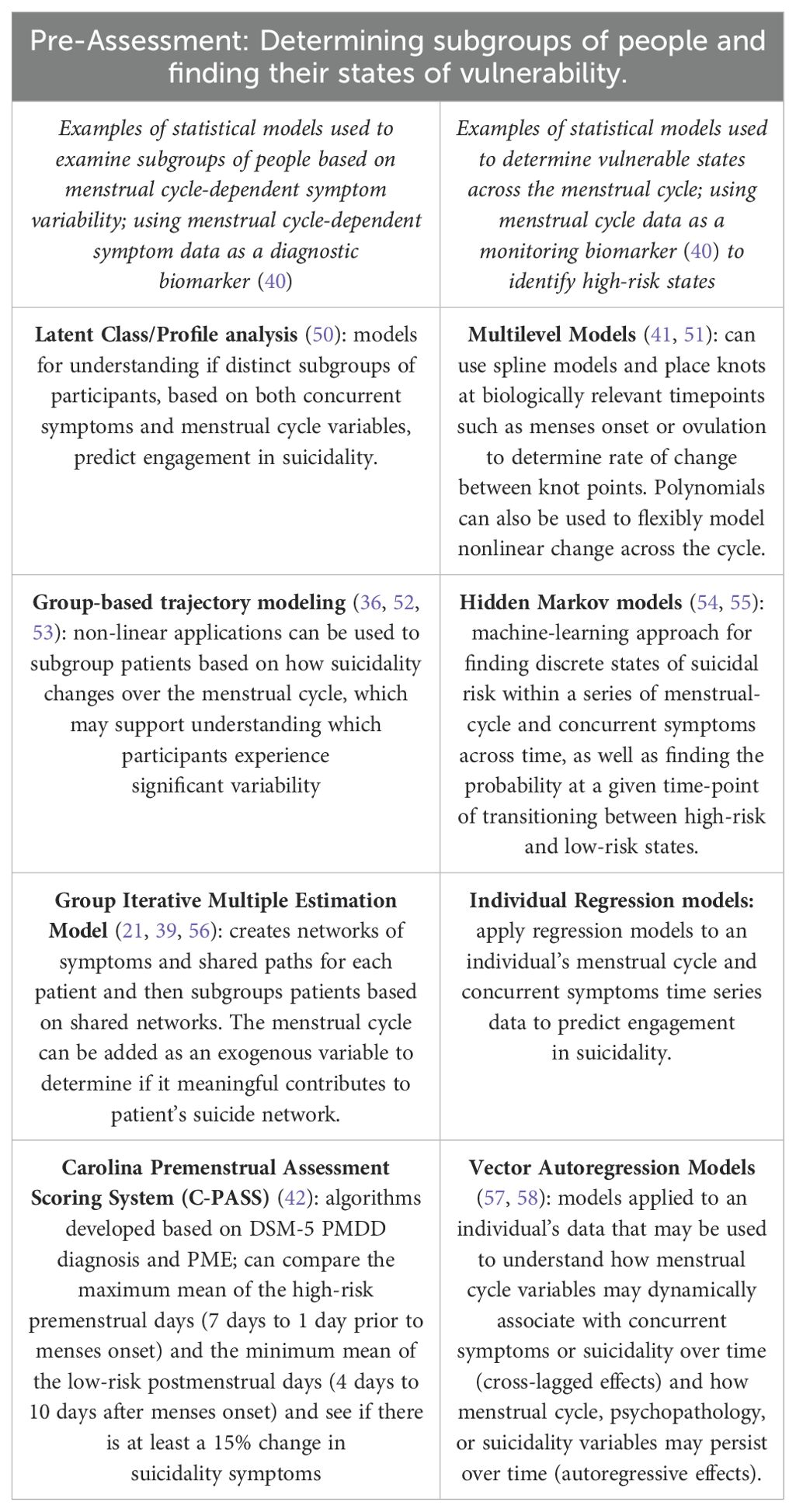
Table 1. Identification of individuals with Menstrual Related Suicidality Changes and their discrete risk states across the menstrual cycle.
2.3 Measuring the menstrual cycle, using wearable technology to detect cycle status, and identifying risk states
There are various ways to measure the menstrual cycle in service of identifying vulnerable states. Given the variability in cycle lengths between and within individuals (43, 44), baseline cycles’ menses onset and ovulation dates can be used to standardize time across the menstrual cycle, providing the percentage of cycle (or cycle phase) elapsed rather than days of the cycle. This approach enhances the precision of continuous modeling strategies in identifying future risk windows, aligning more accurately with ovarian hormones and symptom changes.
When only menses onset dates are available, the duration of each cycle is calculated from one menses onset to the day before the subsequent menses. This duration can then be used to determine the percentage of the cycle elapsed. This percentage is mapped onto subsequent cycles to predict days of increased risk. For example, if a patient has cycles of 32 and 30 days, and symptoms worsening or onsetting at day 28 and 26, respectively, corresponding to 87.5% and 86.7% of their cycle, future cycles may have an estimated duration of 31 days, with symptom onset at approximately 87% of the way through the cycle.
When both menses onset and ovulation dates are available, the luteal phase duration can be calculated from the day after ovulation to the day before the next menses onset, and the follicular phase from menses onset to estimated ovulation (from which percentage of each phase elapsed can be determined for each day). For instance, if a patient’s first cycle has a 14-day follicular phase and a 12-day luteal phase, with symptom onset 10 days into the luteal phase (83.3% elapsed), and the second cycle has a 15-day follicular phase and an 11-day luteal phase with symptoms onset 9 days into the luteal phase (81.8% elapsed), these percentages can inform future risk predictions.
Wearable and smartphone technologies can also be used to track menses dates and identify ovulation. Cycle-tracking applications, such as Apple® Health, collect daily self-reported data on menses status (bleeding vs. not bleeding) and use proprietary algorithms to forecast future menses start dates (45), with a study indicating 40% of the assessed cycles had daily recordings (46). Wearables monitor physiological functions impacted by the cycle, such as thermoregulation and heart rate variability. A high-specificity method for passively detecting ovulation is a wearable tracking basal body temperature (BBT). Progesterone’s thermogenic effect causes an abrupt (surpassing 37°) and sustained temperature elevation in BBT, indicating ovulation. Another potential indicator is heart rate variability (HRV), which is downregulated in the luteal phase, correlating inversely with progesterone (47, 48). Studies are underway to validate passive HRV measurement to determine cycle events (49). Wearables (e.g., Apple Watch®, FitBit®, Oura Rings®) that can passively detect either BBT, HRV, or both would significantly reduce participant burden by passively detecting ovulation. However, for validation of these approaches, it may be prudent for cycle-focused studies to initially include urine luteinizing hormone (LH) testing to evaluate the occurrence and timing of ovulation (LH surges and peaks prior to ovulation), as described in (41).
2.4 Identifying discrete vulnerability states across the menstrual cycle for suicidality based on person-level fluctuations
Once patients are identified as having menstrual cycle-related suicidality (or PMDD/PME), we may pinpoint their vulnerability states throughout the cycle. Group studies suggest peak suicidality occurs three days before and two days after menses onset (7). For JITAI, various statistical models (see Table 1) can identify specific times of suicidality risk based on menstrual cycle time elapsed. These vulnerable states should be stratified into “no”, “low”, “moderate”, and “high” risk days. Interventions can be tailored based on symptom networks exacerbating suicidality.
2.5 Proximal and distal outcomes
We propose person-specific, symptom clusters that mediate the cycle-suicide relationship as the proximal outcome in our example JITAI. The aim of the example JITAI is to deploy interventions that reduce these monthly states of increased hormone-driven symptoms, in order to prevent future suicidality, our distal outcome.
2.6 Decision point
The daily decision point for this JITAI will identify if today is a vulnerable day in the cycle (i.e., a day associated with elevated suicide risk based on baseline cycles). While we anticipate predictable trajectories for SI and SB, we currently recommend daily self-report assessment of suicidal symptoms. However, future JITAI research may move beyond self-report with the development of valid, passively collected prognostic biomarkers of suicide risk, such as smartphone typing patterns (59), fewer phone calls and messages (indicating social withdrawal), and so on.
2.7 Tailoring variables
The tailoring variable for this JITAI is the cluster of symptoms driving perimenstrual worsening of suicidality for the individual. Individual baseline data is used to define cycle days associated with increased suicide risk as well as symptom networks that drive this increase in suicidality (e.g., anger/conflict in response to a progesterone surge, depression/anhedonia in response to estrogen withdrawal).
2.8 Intervention options and personalization
We propose using network models from baseline data to tailor interventions based on individual symptom clusters. JITAI holds the potential to match evidence-based interventions to distinct symptom clusters linked with hormone-driven suicidality within an individual. To illustrate: on risky cycle days, individuals may benefit from receiving a reminder to increase awareness of this vulnerable cycle phase (e.g., “You are at a point in your cycle when depression, hopelessness, and guilt worsen, increasing SI risk”). As risk increases, luteal-phase irritability and affective lability could be targeted with skills from Dialectical Behavior Therapy (e.g., TIP, opposite action to anger). Meanwhile, perimenstrual depression could be targeted with behavioral activation.
Micro-randomized control trials, a type of clinical trial where each relevant decision point is randomly assigned within each person (60), are needed to identify optimal treatments and receptivity states for menstrual cycle-related suicidality and co-occurring affective symptoms. Although current research does not specify receptivity states across the menstrual cycle, we hypothesize that patients may be more receptive to learning and applying skills during non-risky days (e.g., follicular phase). Adapting frameworks such as the multiphase optimization strategy (MOST), which involves a screening, refining, and confirming phase of building a digital interventions, which allows examination of individual components of intervention and delivery (61) may be helpful.
Patients may opt to take their baseline rating to their providers for psychoeducation and diagnosis of PMDD, if applicable. For patients with PMDD, selective serotonin reuptake inhibitors (SSRIs) are an effective treatment option, with some patients seeing symptom reduction within 24-48 hours of taking their medications (62). SSRIs for PMDD patients are prescribed either continuously or to be taken just in the luteal phase (62). For patients who are taking a luteal phase-only SSRI regimen, JITAI can send daily scheduled reminders for medication adherence starting the first day post-ovulation.
2.9 Decision rules
In the schematic decision rule below, we present an example of an operational system that increases participant awareness of their risky phase and deploys interventions well-matched to suicide-related symptoms during their specific vulnerable state.
If the baseline maximum risk score for this menstrual cycle day number is “no risk”:
Then: “No message”
Else if baseline risk score for this menstrual cycle day number is “low risk”:
Then: “Send auto-message that they are entering a vulnerable window in their cycle and recommend non-judgmental awareness of emotions”
Else if baseline risk score for this menstrual cycle day number is “moderate risk” OR “high risk:
Then: “Send patient tailored skill based on the symptom network (e.g., behavioral activation for depression related to estrogen withdrawal or DBT skills for anger/mood swings related to progesterone surge”
3 Discussion
For many patients, the menstrual cycle is a recurring biological predictor of suicide risk. However, there is currently no evidence-based treatment for menstrual cycle-related suicidality. Given the complex, idiographic, and dynamic impact of the menstrual cycle on suicidality, we propose that JITAI are a promising avenue for further exploration. Integrating menstrual cycle variables into JITAI models can leverage a biological variable to predict when and which symptoms are exacerbating suicidality, with the goal of deploying timely, effective interventions. While self-report is a key component of currently proposed models, the advancement of wearable and smartphone-based cycle tracking technologies may allow for low-burden, passive measurement in the future.
3.1 Challenges and future directions
While JITAIs offer promise in addressing menstrual cycle-related suicidality, there are distinct challenges in their development and implementation. First, atypical menstrual cycles, related to anovulation, contraceptive use, peripuberty, or perimenopause (63), pose obstacles to accurate prediction of risky timeframes. Thus, research should account for nuances in cycle variation. Ethical concerns arise regarding intervention timing and methods for high-risk patients. Clear protocols must address imminent risk, balancing intervention efficacy with confidentiality and potential need for hospitalization. Although in this hypothesized model, daily variables were used for tailoring variables, we hope that eventually, suicide and menstrual research will move past daily measurement to more within the moment (i.e, hourly to seconds) detection of states of vulnerability and receptivity, allowing for more timely responses to perimenstrual suicide changes. Finally, before the deployment of JITAI for menstrual cycle-related suicidality, randomized controlled trials are needed to determine (a) which cycle-timed interventions are most acceptable to patients, (b) when patients are most receptive to interventions (12), and (c) whether targeting monthly risk windows with JITAI reduces future suicide attempts. Finally, while our manuscript primarily addresses menstrual cycle-related psychiatric changes, we acknowledge that the menstrual cycle has an impact on broader biological systems [i.e., migraines and epilepsy (64)] and emphasize that the broader digital therapeutic research accounts for menstrual-related changes.
4 Conclusion
We advocate for integrating the menstrual cycle into JITAI models for suicide prevention to address individual variability in suicidality and psychiatric symptoms. Despite implementation challenges, we propose including the menstrual cycle as a variable in JITAIs, particularly for suicide prevention.
Data availability statement
The original contributions presented in the study are included in the article/supplementary material. Further inquiries can be directed to the corresponding author.
Author contributions
HT: Conceptualization, Methodology, Project administration, Resources, Visualization, Writing – original draft, Writing – review & editing. DC: Conceptualization, Writing – original draft, Writing – review & editing, Methodology. AR: Methodology, Writing – original draft, Writing – review & editing. AN: Conceptualization, Writing – review & editing. JR: Supervision, Writing – review & editing. MN: Supervision, Writing – review & editing. TE: Conceptualization, Methodology, Supervision, Writing – review & editing, Writing – original draft.
Funding
The author(s) declare financial support was received for the research, authorship, and/or publication of this article. All funding received for this research was funded by the National Institute of Mental Health (AR: F31 MH132231, AN: F30MH138058, DC: F31MH130055, TE and MK: R01MH122446).
Conflict of interest
MN receives publication royalties from Macmillan, Pearson, and UpToDate. He has been a paid consultant in the past three years for Apple, Microsoft, COMPASS Pathways, and Cambridge Health Alliance, and for legal cases regarding a death by suicide. He has stock options in Cerebral Inc. He is an unpaid scientific advisor for Empatica, Koko, and TalkLife.
The remaining authors declare that the research was conducted in the absence of any commercial or financial relationships that could be construed as a potential conflict of interest.
Publisher’s note
All claims expressed in this article are solely those of the authors and do not necessarily represent those of their affiliated organizations, or those of the publisher, the editors and the reviewers. Any product that may be evaluated in this article, or claim that may be made by its manufacturer, is not guaranteed or endorsed by the publisher.
References
1. Martínez-Alés G, Jiang T, Keyes KM, Gradus JL. The recent rise of suicide mortality in the United States. Annu Rev Public Health. (2022) 43:99–116. doi: 10.1146/annurev-publhealth-051920-123206
2. Sewall CJR, Wright AGC. Personalizing suicidology. Crisis. (2021) 42:405–10. doi: 10.1027/0227-5910/a000834
3. Kleiman EM, Turner BJ, Fedor S, Beale EE, Picard RW, Huffman JC, et al. Digital phenotyping of suicidal thoughts. Depress Anxiety. (2018) 35:601–8. doi: 10.1002/da.2018.35.issue-7
4. Coppersmith DDL, Dempsey W, Kleiman EM, Bentley KH, Murphy SA, Nock MK. Just-in-time adaptive interventions for suicide prevention: promise, challenges, and future directions. Psychiatry. (2022) 85:317–33. doi: 10.1080/00332747.2022.2092828
5. Eisenlohr-Moul TA, Bowers SM, Prinstein MJ, Schmalenberger KM, Walsh EC, Young SL, et al. Effects of acute estradiol and progesterone on perimenstrual exacerbation of suicidal ideation and related symptoms: a crossover randomized controlled trial. Translational psychiatry (2022). 12(1), 528. doi: 10.1038/s41398-022-02294-1
6. Eisenlohr-Moul T, Barone J, Pinna G, Wagner-Schuman M, Almeida FB, Santovito LS, et al. Independent effects of acute estradiol or progesterone on perimenstrual changes in suicidal ideation, affective symptoms, and 3α-reduced progesterone metabolites: A crossover randomized controlled trial. OSF (2023). Available at: https://osf.io/m9sx2.
7. Owens S, Schmalenberger KM, Bowers SM, Rubinow DR, Prinstein MJ, Girdler SS, et al. (2023). Cyclical exacerbation of suicidal ideation in female outpatients: prospective evidence from daily ratings in a transdiagnostic sample. J Psychopathol Clin Sci. In Press. 132(6), 704–15. doi: 10.1037/abn0000838
8. Ross JM, Barone JC, Tauseef H, Schmalenberger KM, Nagpal A, Crane NA, et al. Predicting acute changes in suicidal ideation and planning: A longitudinal study of symptom mediators and the role of the menstrual cycle in female psychiatric outpatients with suicidality. (2024). The American journal of psychiatry. 181(1), 57–67. doi: 10.1176/appi.ajp.20230303
9. RFA-MH-23-170: using just-in-time adaptive interventions to optimize established adolescent mental health treatments (R61/R33 clinical trial required). Available online at: https://grants.nih.gov/grants/guide/rfa-files/RFA-MH-23-170.html. (Accessed May 10, 2024).
10. National Institute of Diabetes and Digestive and Kidney Diseases. Notice of special interest: womens health research - NOT-OD-24-079 - NIDDK. Available online at: https://www.niddk.nih.gov/research-funding/current-opportunities/not-od-24-079. (Accessed May 10, 2024).
11. Arnegard ME, Whitten LA, Hunter C, Clayton JA. Sex as a biological variable: A 5-year progress report and call to action. J Womens Health. (2020) 29:858–64. doi: 10.1089/jwh.2019.8247
12. Keller R, Wangenheim F, Mair J, Kowatsch T. Receptivity to mobile health interventions. In. (2022) p:65–77.
13. Nahum-Shani I, Smith SN, Spring BJ, Collins LM, Witkiewitz K, Tewari A, et al. Just-in-time adaptive interventions (JITAIs) in mobile health: key components and design principles for ongoing health behavior support. Ann Behav Med Publ Soc Behav Med. (2017) 52:446–62. doi: 10.1007/s12160-016-9830-8
14. Wang L, Miller LC. Just-in-the-moment adaptive interventions (JITAI): A meta-analytical review. Health Commun. (2020) 35:1531–44. doi: 10.1080/10410236.2019.1652388
15. Hardeman W, Houghton J, Lane K, Jones A, Naughton F. A systematic review of just-in-time adaptive interventions (JITAIs) to promote physical activity. Int J Behav Nutr Phys Act. (2019) 16:31. doi: 10.1186/s12966-019-0792-7
16. Xu Z, Smit E. Using a complexity science approach to evaluate the effectiveness of just-in-time adaptive interventions: A meta-analysis. Digit Health. (2023) 9:20552076231183543. doi: 10.1177/20552076231183543
17. Carpenter SM, Menictas M, Nahum-Shani I, Wetter DW, Murphy SA. Developments in mobile health just-in-time adaptive interventions for addiction science. Curr Addict Rep. (2020) 7:280–90. doi: 10.1007/s40429-020-00322-y
18. Wang L, Miller L. Assessment and disruption of ruminative episodes to enhance mobile cognitive behavioral therapy just-in-time adaptive interventions in clinical depression: pilot randomized controlled trial. JMIR Form Res. (2023) 7:e37270. doi: 10.2196/37270
19. Mestdagh M, Dejonckheere E. Ambulatory assessment in psychopathology research: Current achievements and future ambitions. Curr Opin Psychol. (2021) 41:1–8. doi: 10.1016/j.copsyc.2021.01.004
20. Olthof M, Hasselman F, Oude Maatman F, Bosman AMT, Lichtwarck-Aschoff A. Complexity theory of psychopathology. J Psychopathol Clin Sci. (2023) 132:314–23. doi: 10.1037/abn0000740
21. Kaurin A, Dombrovski AY, Hallquist MN, Wright AGC. Integrating a functional view on suicide risk into idiographic statistical models. Behav Res Ther. (2022) 150:104012. doi: 10.1016/j.brat.2021.104012
22. Arshad U, Farhat-Ul-Ain null, Gauntlett J, Husain N, Chaudhry N, Taylor PJ. A systematic review of the evidence supporting mobile- and internet-based psychological interventions for self-harm. Suicide Life Threat Behav. (2020) 50:151–79. doi: 10.1111/sltb.12583
23. Nock MK, Green JG, Hwang I, McLaughlin KA, Sampson NA, Zaslavsky AM, et al. Prevalence, correlates, and treatment of lifetime suicidal behavior among adolescents: results from the national comorbidity survey replication adolescent supplement. JAMA Psychiatry. (2013) 70:300–10. doi: 10.1001/2013.jamapsychiatry.55
24. Kessler RC, McGonagle KA, Swartz M, Blazer DG, Nelson CB. Sex and depression in the National Comorbidity Survey I: Lifetime prevalence, chronicity and recurrence. J Affect Disord. (1993) 29:85–96. doi: 10.1016/0165-0327(93)90026-G
25. Eisenlohr-Moul T. Premenstrual disorders: A primer and research agenda for psychologists. Clin Psychol. (2019) 72:5–17. doi: 10.31234/osf.io/tw4bd
26. Epperson CN, Steiner M, Hartlage SA, Eriksson E, Schmidt PJ, Jones I, et al. Premenstrual dysphoric disorder: evidence for a new category for DSM-5. Am J Psychiatry. (2012) 169:465–75. doi: 10.1176/appi.ajp.2012.11081302
27. Saunders KEA, Hawton K. Suicidal behavior and the menstrual cycle. Psychol Med. (2006) 36:901–12. doi: 10.1017/S0033291706007392
28. Owens SA, Eisenlohr-Moul T. Suicide risk and the menstrual cycle: a review of candidate RDoC mechanisms. Curr Psychiatry Rep. (2018) 20:106. doi: 10.1007/s11920-018-0962-3
29. Eisenlohr-Moul T, Divine M, Schmalenberger K, Murphy L, Buchert B, Wagner-Schuman M, et al. Prevalence of lifetime self-injurious thoughts and behaviors in a global sample of 599 patients reporting prospectively confirmed diagnosis with premenstrual dysphoric disorder. BMC psychiatry. (2022) 22(1):199. doi: 10.1186/s12888-022-03851-0
30. Peters J, Schmalenberger KM, Eng A, Stumper A, Martel MM, Eisenlohr-Moul T. Dimensional Affective Sensitivity to Hormones across the Menstrual Cycle (DASH-MC): A Transdiagnostic Framework for Ovarian Steroid Influences on Psychopathology. Molecular psychiatry, (2024). doi: 10.1038/s41380-024-02693-4
31. Hartlage SA, Brandenburg DL, Kravitz HM. Premenstrual exacerbation of depressive disorders in a community-based sample in the United States. Psychosom Med. (2004) 66:698–706. doi: 10.1097/01.psy.0000138131.92408.b9
32. Consensus Group of the International Society for Premenstrual Disorders, Nevatte T, O’Brien PMS, Bäckström T, Brown C, Dennerstein L, et al. ISPMD consensus on the management of premenstrual disorders. Arch Womens Ment Health. (2013) 16:279–91. doi: 10.1007/s00737-013-0346-y
33. Eisenlohr-Moul TA, Schmalenberger KM, Owens SA, Peters JR, Dawson DN, Girdler SS. Perimenstrual exacerbation of symptoms in borderline personality disorder: evidence from multilevel models and the Carolina premenstrual assessment scoring system. Psychol Med. (2018) 48:2085–95. doi: 10.1017/S0033291718001253
34. Eisenlohr-Moul TA. Commentary on Joyce et al.: Studying menstrual cycle effects on behavior requires within-person designs and attention to individual differences in hormone sensitivity. Addiction. (2021) 116(10):2759–60. doi: 10.1111/add.15576
35. Roy-Byrne PP, Rubinow DR, Hoban MC, Parry BL, Rosenthal NE, Nurnberger JI, et al. Premenstrual changes: a comparison of five populations. Psychiatry Res. (1986) 17:77–85. doi: 10.1016/0165-1781(86)90062-4
36. Eisenlohr-Moul TA, Kaiser G, Weise C, Schmalenberger KM, Kiesner J, Ditzen B, et al. Are there temporal subtypes of premenstrual dysphoric disorder?: Using group-based trajectory modeling to identify individual differences in symptom change. Psychol Med. (2020) 50:964–72. doi: 10.1017/S0033291719000849
37. PMDD Community Coalition (PCC) Roundtable. PCORI (2022). Available at: https://www.pcori.org/resources/pmdd-community-coalition-pcc-roundtable.
38. Kusuma K, Larsen M, Quiroz JC, Gillies M, Burnett A, Qian J, et al. The performance of machine learning models in predicting suicidal ideation, attempts, and deaths: A meta-analysis and systematic review. J Psychiatr Res. (2022) 155:579–88. doi: 10.1016/j.jpsychires.2022.09.050
39. Yin Q, Hughes CD, Rizvi SL. Using GIMME to model the emotional context of suicidal ideation based on clinical data: From research to clinical practice. Behav Res Ther. (2023) 171:104427. doi: 10.1016/j.brat.2023.104427
40. Coravos A, Khozin S, Mandl KD. Developing and adopting safe and effective digital biomarkers to improve patient outcomes. NPJ Digit Med. (2019) 2:1–5. doi: 10.1038/s41746-019-0090-4
41. Schmalenberger KM, Tauseef HA, Barone JC, Owens SA, Lieberman L, Jarczok MN, et al. How to study the menstrual cycle: Practical tools and recommendations. Psychoneuroendocrinology. (2021) 123:104895. doi: 10.1016/j.psyneuen.2020.104895
42. Eisenlohr-Moul TA, Girdler SS, Schmalenberger KM, Dawson DN, Surana P, Johnson JL, et al. Toward the reliable diagnosis of DSM-5 premenstrual dysphoric disorder: the Carolina premenstrual assessment scoring system (C-PASS). Am J Psychiatry. (2017) 174:51–9. doi: 10.1176/appi.ajp.2016.15121510
43. Fehring RJ, Schneider M, Raviele K. Variability in the phases of the menstrual cycle. J Obstet Gynecol Neonatal Nurs JOGNN. (2006) 35:376–84. doi: 10.1111/j.1552-6909.2006.00051.x
44. Bull JR, Rowland SP, Scherwitzl EB, Scherwitzl R, Danielsson KG, Harper J. Real-world menstrual cycle characteristics of more than 600,000 menstrual cycles | npj Digital Medicine. NPJ Digit Med. (2019) 2:83. doi: 10.1038/s41746-019-0152-7
45. Simmons RG, Shattuck DC, Jennings VH. Assessing the efficacy of an app-based method of family planning: the dot study protocol. JMIR Res Protoc. (2017) 6:e5. doi: 10.2196/resprot.6886
46. Karasneh RA, Al-Azzam SI, Alzoubi KH, Muflih SM, Hawamdeh SS. Smartphone applications for period tracking: rating and behavioral change among women users. Obstet Gynecol Int. (2020) 2020:2192387. doi: 10.1155/2020/2192387
47. Schmalenberger KM, Eisenlohr-Moul TA, Würth L, Schneider E, Thayer JF, Ditzen B, et al. A systematic review and meta-analysis of within-person changes in cardiac vagal activity across the menstrual cycle: implications for female health and future studies. J Clin Med. (2019) 8:1946. doi: 10.3390/jcm8111946
48. Schmalenberger KM, Eisenlohr-Moul TA, Jarczok MN, Eckstein M, Schneider E, Brenner IG, et al. Menstrual cycle changes in vagally-mediated heart rate variability are associated with progesterone: evidence from two within-person studies. J Clin Med. (2020) 9:617. doi: 10.3390/jcm9030617
49. Alzueta E, de Zambotti M, Javitz H, Dulai T, Albinni B, Simon KC, et al. Tracking sleep, temperature, heart rate, and daily symptoms across the menstrual cycle with the oura ring in healthy women. Int J Womens Health. (2022) 14:491–503. doi: 10.2147/IJWH.S341917
50. Williams GA, Kibowski F. Latent class analysis and latent profile analysis. In: Handbook of methodological approaches to community-based research: Qualitative, quantitative, and mixed methods. Oxford University Press, New York, NY, US (2016). p. 143–51.
51. Grimm KJ, Ram N, Hamagami F. Nonlinear growth curves in developmental research. Child Dev. (2011) 82:1357–71. doi: 10.1111/j.1467-8624.2011.01630.x
52. Nagin DS, Odgers CL. Group-based trajectory modeling in clinical research. Annu Rev Clin Psychol. (2010) 6:109–38. doi: 10.1146/annurev.clinpsy.121208.131413
53. Ram N, Grimm KJ. Growth mixture modeling: A method for identifying differences in longitudinal change among unobserved groups. Int J Behav Dev. (2009) 33:565–76. doi: 10.1177/0165025409343765
54. Eddy SR. What is a hidden Markov model? Nat Biotechnol. (2004) 22(10):1315–6. doi: 10.1038/nbt1004-1315
55. Jiang X, Chen Y, Ao N, Xiao Y, Du F. A depression-risk mental pattern identified by hidden Markov model in undergraduates. Int J Environ Res Public Health. (2022) 19:14411. doi: 10.3390/ijerph192114411
56. Gates KM, Molenaar PCM. Group search algorithm recovers effective connectivity maps for individuals in homogeneous and heterogeneous samples. NeuroImage. (2012) 63:310–9. doi: 10.1016/j.neuroimage.2012.06.026
57. Wright AGC, Woods WC. Personalized models of psychopathology. Annu Rev Clin Psychol. (2020) 16:49–74. doi: 10.1146/annurev-clinpsy-102419-125032
58. Brandt P, Williams JT. Multiple time series models Vol. xiii. . Thousand Oaks, CA, US: Sage Publications, Inc (2007). 99 p. (Multiple time series models).
59. Knol L, Nagpal A, Leaning IE, Idda E, Hussain F, Ning E, et al. Smartphone keyboard dynamics predict affect in suicidal ideation. NPJ Digit Med. (2024) 7:1–10. doi: 10.1038/s41746-024-01048-1
60. Klasnja P, Hekler EB, Shiffman S, Boruvka A, Almirall D, Tewari A, et al. Microrandomized trials: An experimental design for developing just-in-time adaptive interventions. Health Psychol. (2015) 34:1220–8. doi: 10.1037/hea0000305
61. Collins LM, Murphy SA, Strecher V. The multiphase optimization strategy (MOST) and the sequential multiple assignment randomized trial (SMART): new methods for more potent eHealth interventions. Am J Prev Med. (2007) 32:S112–8. doi: 10.1016/j.amepre.2007.01.022
62. Marjoribanks J, Brown J, O’Brien PMS, Wyatt K. Selective serotonin reuptake inhibitors for premenstrual syndrome. Cochrane Database Syst Rev. (2013) 2013:CD001396. doi: 10.1002/14651858.CD001396.pub3
63. Klusmann H, Eisenlohr-Moul T, Baresich K, Schmalenberger KM, Girdler S, Andersen E. Analyzing the atypical - Methods for studying the menstrual cycle in adolescents. Psychoneuroendocrinology. (2023) 158:106389. doi: 10.1016/j.psyneuen.2023.106389
64. Barone JC, Butler MP, Ross A, Patterson A, Wagner-Schuman M, Eisenlohr-Moul TA. A scoping review of hormonal clinical trials in menstrual cycle-related brain disorders: Studies in premenstrual mood disorder, menstrual migraine, and catamenial epilepsy. Front Neuroendocrinol. (2023) 71:101098. doi: 10.1016/j.yfrne.2023.101098
Keywords: just-in-time adaptative intervention, menstrual cycle, suicide, idiographic modeling, mobile health, digital phenotyping, passive measures, self-injury
Citation: Tauseef HA, Coppersmith DDL, Reid-Russell AJ, Nagpal A, Ross J, Nock MK and Eisenlohr-Moul T (2024) A call to integrate menstrual cycle influences into just-in-time adaptive interventions for suicide prevention. Front. Psychiatry 15:1434499. doi: 10.3389/fpsyt.2024.1434499
Received: 17 May 2024; Accepted: 23 September 2024;
Published: 05 December 2024.
Edited by:
Liisa Hantsoo, Johns Hopkins University, United StatesReviewed by:
Anastasio Tini, Marche Polytechnic University, ItalyMarcia Nißen, University of St. Gallen, Switzerland
Copyright © 2024 Tauseef, Coppersmith, Reid-Russell, Nagpal, Ross, Nock and Eisenlohr-Moul. This is an open-access article distributed under the terms of the Creative Commons Attribution License (CC BY). The use, distribution or reproduction in other forums is permitted, provided the original author(s) and the copyright owner(s) are credited and that the original publication in this journal is cited, in accordance with accepted academic practice. No use, distribution or reproduction is permitted which does not comply with these terms.
*Correspondence: Hafsah A. Tauseef, aGFmc2FoQHVpYy5lZHU=